Selecting the Optimal Multilingual Embedding Model for Your Retrieval Augmented Generation (RAG) System
In today's interconnected world, building effective multilingual AI systems is paramount. Robust multilingual embedding models are crucial for Retrieval Augmented Generation (RAG) systems, which combine the power of large language models with external knowledge retrieval. This guide helps you choose the best multilingual embedding model for your RAG system.
Understanding Multilingual Embeddings and RAG
Before model selection, grasp the concepts of multilingual embeddings and their role in RAG.
Multilingual embeddings are vector representations of words or sentences that capture semantic meaning across multiple languages. This cross-lingual semantic understanding is vital for multilingual AI, enabling cross-lingual information retrieval and comparison.
RAG systems integrate a retrieval component and a generative model. The retrieval component, using embeddings, finds relevant information from a knowledge base to enhance the generative model's input. For multilingual RAG, efficient cross-lingual representation and comparison are essential.
Key Factors in Multilingual Embedding Model Selection
Consider these factors when choosing a multilingual embedding model:
-
Language Support: The model must support all languages needed by your application. Some models cover many languages, while others focus on specific language families.
-
Embedding Dimensionality: Higher dimensionality offers richer semantic representation but demands more computational resources. Balance performance with resource constraints.
-
Training Data and Domain: The model's performance depends heavily on the quality and diversity of its training data. For specific domains (e.g., legal, medical), consider domain-specific models or fine-tuning options.
-
Licensing and Usage Rights: Check the model's license. Some are open-source, while others require commercial licenses. Ensure the license aligns with your usage plans.
-
Integration Ease: Choose models easily integrated into your existing RAG architecture, preferably with clear APIs and documentation.
-
Community Support and Updates: Active community support and regular updates ensure long-term model maintenance and improvement.
Popular Multilingual Embedding Models
Several models stand out for their performance and versatility. The following table compares several popular options (note that performance metrics may not be directly comparable across all models due to variations in tasks and benchmarks):
Model Performance Overview
A summary of the performance of several models:
- XLM-RoBERTa: Excellent performance in cross-lingual natural language inference (XNLI).
- mBERT: Good zero-shot performance on cross-lingual transfer tasks.
- LaBSE: High accuracy in cross-lingual semantic retrieval.
- GPT-3.5: Strong zero-shot and few-shot learning capabilities across multiple languages.
- LASER: High accuracy in cross-lingual document classification.
- Multilingual Universal Sentence Encoder: Good performance in cross-lingual semantic similarity.
- (Other models listed in the original text are also strong contenders, with varying strengths across different tasks.)
Evaluation Methods
Effective evaluation is critical:
- Benchmark Datasets: Use established multilingual benchmarks like XNLI or PAWS-X.
- Task-Specific Evaluation: Test models on tasks relevant to your RAG system (e.g., cross-lingual information extraction).
- Domain-Specific Testing: Create a test set from your domain for accurate performance assessment.
- Computational Efficiency: Measure the time and resources required for embedding generation and similarity searches.
Best Practices for Implementation
After model selection:
- Fine-tuning: Fine-tune the model on your domain-specific data.
- Caching: Cache embeddings for frequently accessed content.
- Dimensionality Reduction: Reduce embedding dimensions if resources are limited.
- Hybrid Approaches: Combine multiple models or use language-specific models for high-priority languages.
- Regular Evaluation: Monitor model performance and adjust as needed.
- Fallback Mechanisms: Have backup strategies for languages or contexts where the primary model underperforms.
Conclusion
Choosing the right multilingual embedding model significantly impacts your RAG system's performance, resource usage, and scalability. Careful consideration of language coverage, computational requirements, domain relevance, and rigorous evaluation will lead to optimal results. The field is constantly evolving, so stay updated on new models and techniques. With the right model, your RAG system can overcome language barriers and deliver powerful multilingual capabilities.
Frequently Asked Questions
-
Q1: What are multilingual embedding models and their importance in RAG? A1: They represent text from multiple languages in a shared vector space, enabling cross-lingual information retrieval and understanding within RAG systems.
-
Q2: How do I evaluate multilingual embedding models for my specific needs? A2: Use a diverse test set, measure retrieval accuracy (MRR, NDCG), assess cross-lingual semantic preservation, and test with real-world queries in various languages.
-
Q3: What are some popular multilingual embedding models for RAG? A3: mBERT, XLM-RoBERTa, LaBSE, LASER, and the Multilingual Universal Sentence Encoder are good starting points. The best choice depends on your specific requirements.
-
Q4: How do I balance model performance and computational requirements? A4: Consider hardware limitations, use quantized or distilled versions of models, evaluate different model sizes, and benchmark on your infrastructure.
The above is the detailed content of How to Find the Best Multilingual Embedding Model for Your RAG?. For more information, please follow other related articles on the PHP Chinese website!
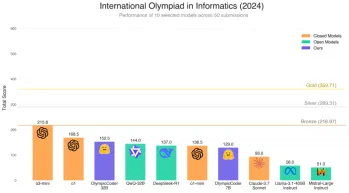
Hugging Face's OlympicCoder-7B: A Powerful Open-Source Code Reasoning Model The race to develop superior code-focused language models is intensifying, and Hugging Face has joined the competition with a formidable contender: OlympicCoder-7B, a product
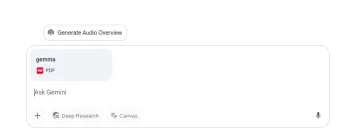
How many of you have wished AI could do more than just answer questions? I know I have, and as of late, I’m amazed by how it’s transforming. AI chatbots aren’t just about chatting anymore, they’re about creating, researchin
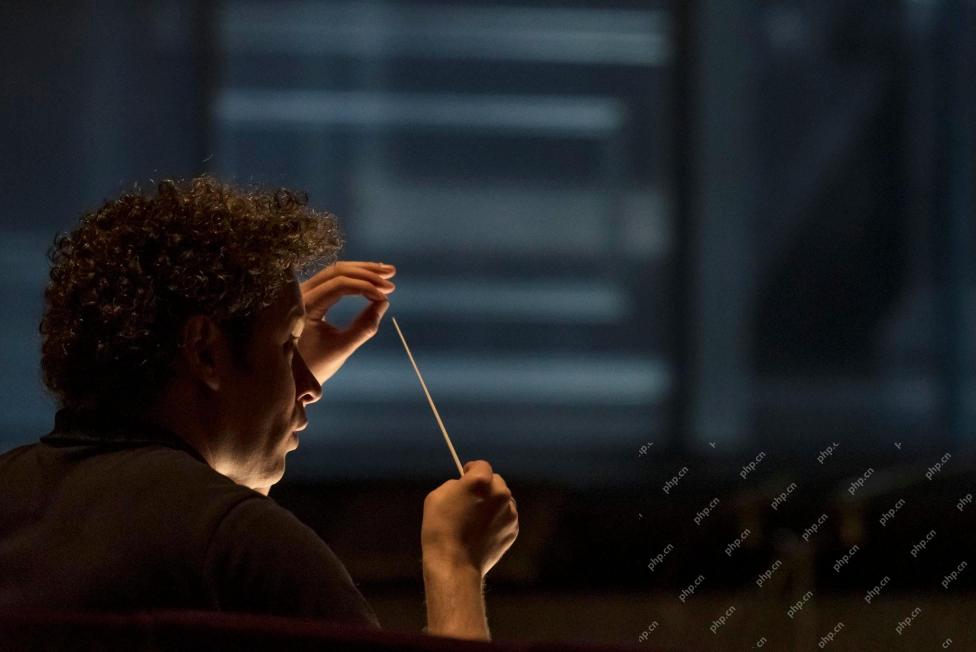
As smart AI begins to be integrated into all levels of enterprise software platforms and applications (we must emphasize that there are both powerful core tools and some less reliable simulation tools), we need a new set of infrastructure capabilities to manage these agents. Camunda, a process orchestration company based in Berlin, Germany, believes it can help smart AI play its due role and align with accurate business goals and rules in the new digital workplace. The company currently offers intelligent orchestration capabilities designed to help organizations model, deploy and manage AI agents. From a practical software engineering perspective, what does this mean? The integration of certainty and non-deterministic processes The company said the key is to allow users (usually data scientists, software)
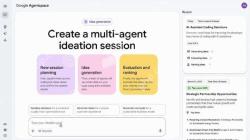
Attending Google Cloud Next '25, I was keen to see how Google would distinguish its AI offerings. Recent announcements regarding Agentspace (discussed here) and the Customer Experience Suite (discussed here) were promising, emphasizing business valu
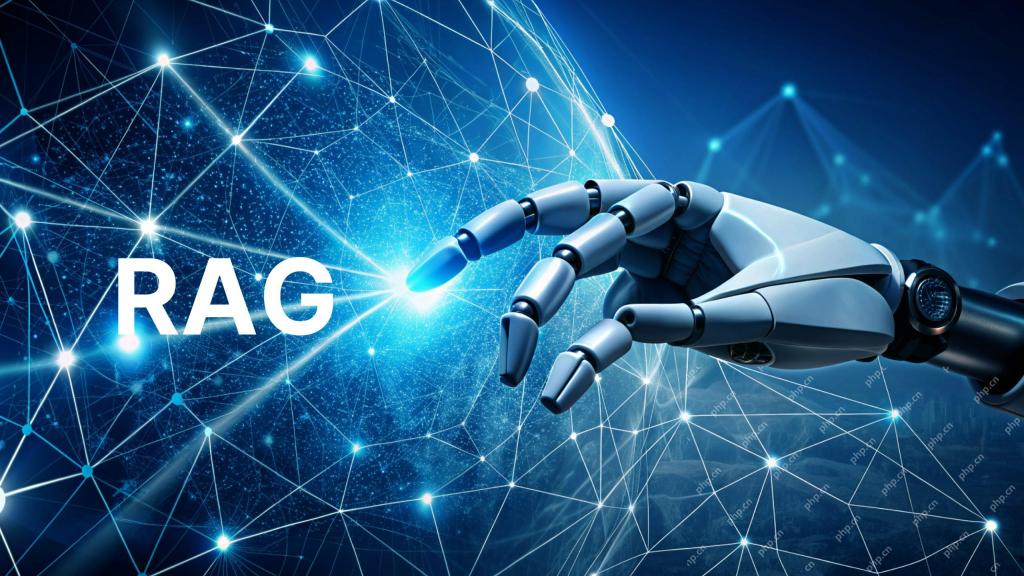
Selecting the Optimal Multilingual Embedding Model for Your Retrieval Augmented Generation (RAG) System In today's interconnected world, building effective multilingual AI systems is paramount. Robust multilingual embedding models are crucial for Re
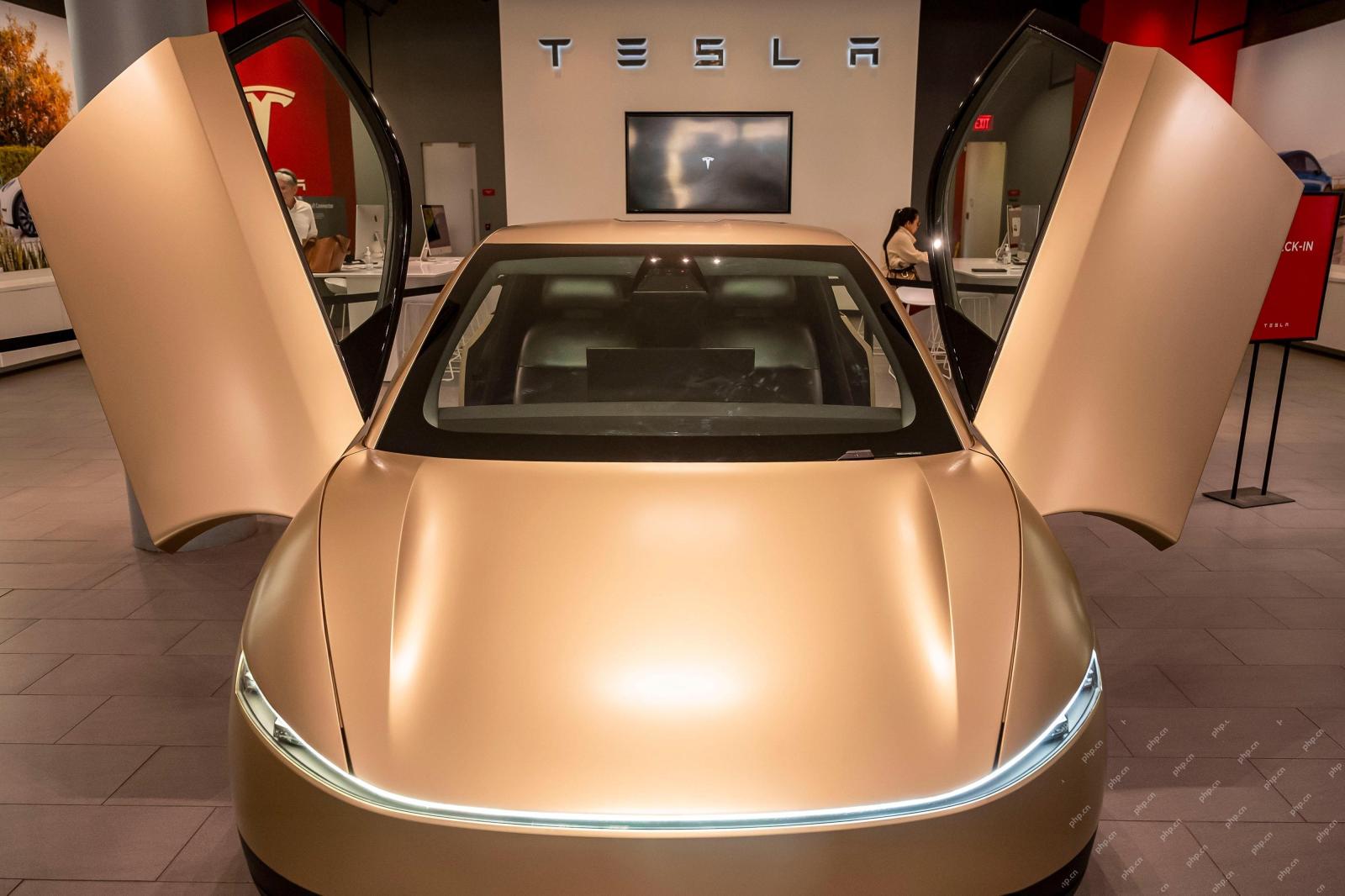
Tesla's Austin Robotaxi Launch: A Closer Look at Musk's Claims Elon Musk recently announced Tesla's upcoming robotaxi launch in Austin, Texas, initially deploying a small fleet of 10-20 vehicles for safety reasons, with plans for rapid expansion. H
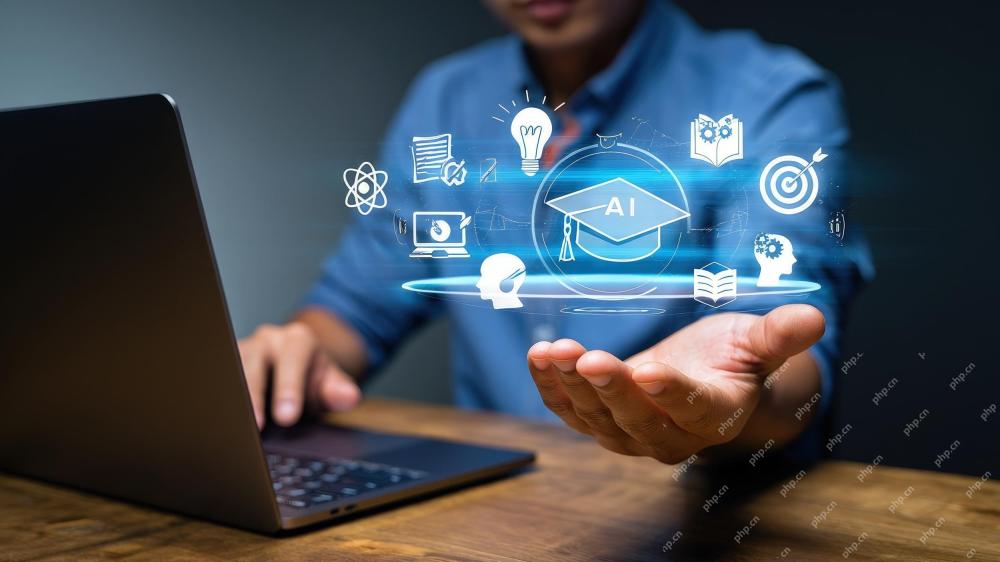
The way artificial intelligence is applied may be unexpected. Initially, many of us might think it was mainly used for creative and technical tasks, such as writing code and creating content. However, a recent survey reported by Harvard Business Review shows that this is not the case. Most users seek artificial intelligence not just for work, but for support, organization, and even friendship! The report said that the first of AI application cases is treatment and companionship. This shows that its 24/7 availability and the ability to provide anonymous, honest advice and feedback are of great value. On the other hand, marketing tasks (such as writing a blog, creating social media posts, or advertising copy) rank much lower on the popular use list. Why is this? Let's see the results of the research and how it continues to be
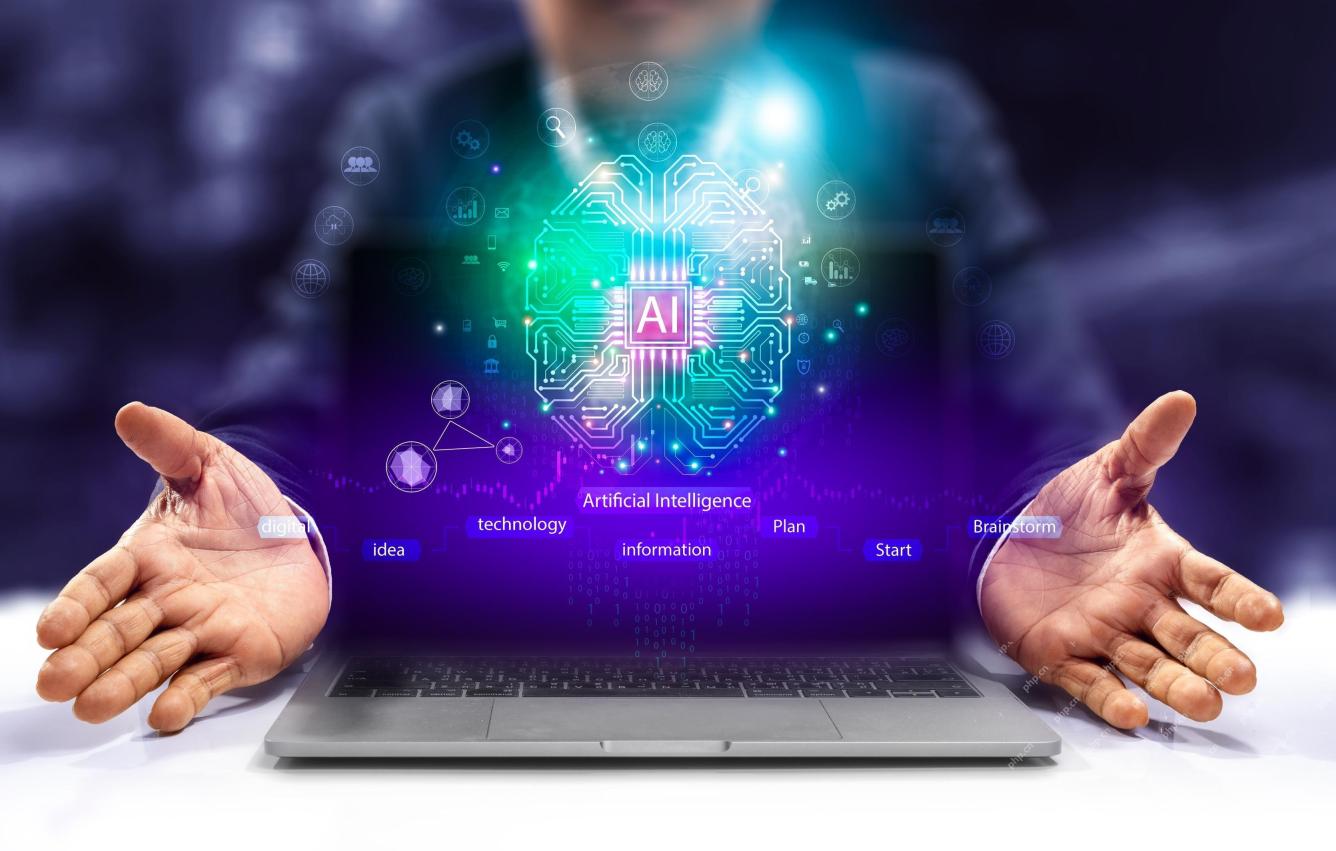
The rise of AI agents is transforming the business landscape. Compared to the cloud revolution, the impact of AI agents is predicted to be exponentially greater, promising to revolutionize knowledge work. The ability to simulate human decision-maki


Hot AI Tools

Undresser.AI Undress
AI-powered app for creating realistic nude photos

AI Clothes Remover
Online AI tool for removing clothes from photos.

Undress AI Tool
Undress images for free

Clothoff.io
AI clothes remover

Video Face Swap
Swap faces in any video effortlessly with our completely free AI face swap tool!

Hot Article

Hot Tools

Zend Studio 13.0.1
Powerful PHP integrated development environment

SublimeText3 Chinese version
Chinese version, very easy to use

MinGW - Minimalist GNU for Windows
This project is in the process of being migrated to osdn.net/projects/mingw, you can continue to follow us there. MinGW: A native Windows port of the GNU Compiler Collection (GCC), freely distributable import libraries and header files for building native Windows applications; includes extensions to the MSVC runtime to support C99 functionality. All MinGW software can run on 64-bit Windows platforms.

PhpStorm Mac version
The latest (2018.2.1) professional PHP integrated development tool
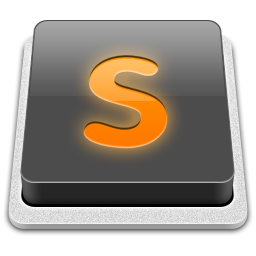
SublimeText3 Mac version
God-level code editing software (SublimeText3)