Llama 3.1 finally appeared, but the source is not Meta official. Today, news of the leak of the new Llama large model went viral on Reddit. In addition to the base model, it also includes benchmark results of 8B, 70B and the maximum parameter of 405B. 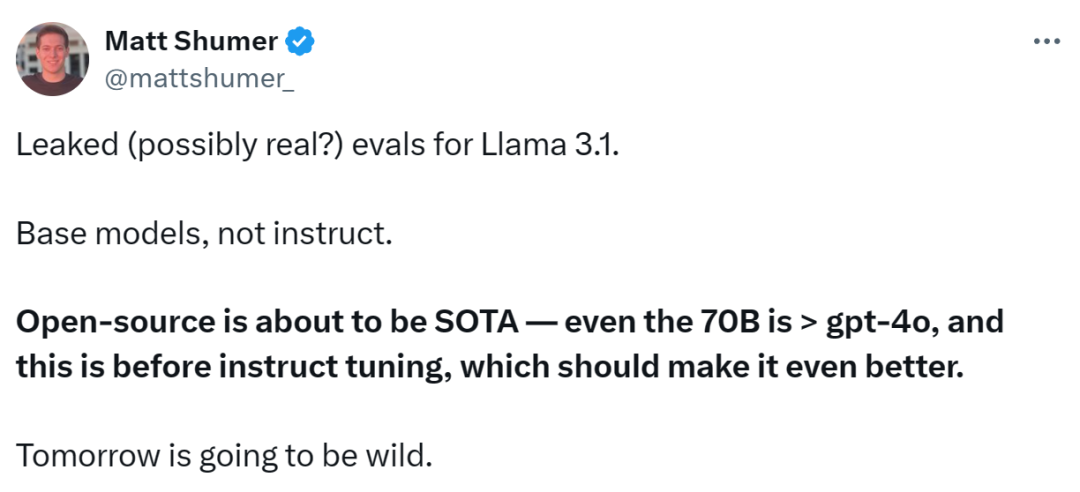
The picture below shows the comparison results of each version of Llama 3.1 with OpenAI GPT-4o and Llama 3 8B/70B. As you can see, even the 70B version surpasses GPT-4o on multiple benchmarks. 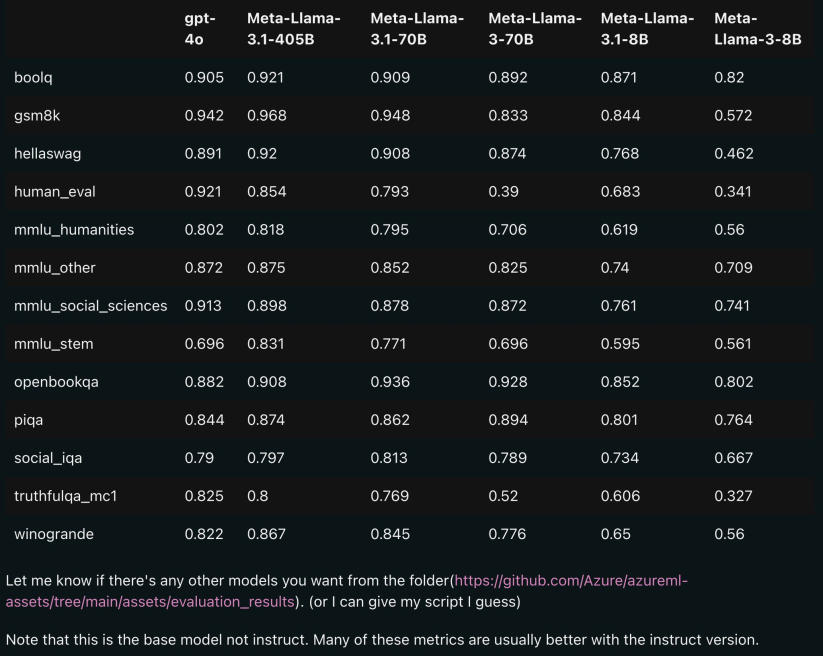
, the 8B and 70B models of version 3.1 are distilled from 405B, so compared to The previous generation had significant performance improvements.
Some netizens said that this is the first time that an open source model has surpassed closed source models such as GPT4o and Claude Sonnet 3.5 and reached SOTA on multiple benchmarks.
At the same time, the model card of Llama 3.1 leaked and the details were leaked (the date marked in the model card indicates that it is based on the July 23rd release).
Someone summarized the following highlights: 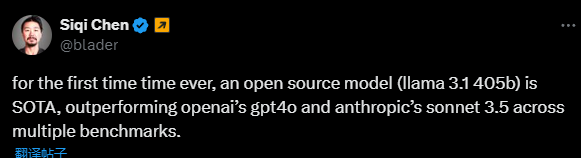
The model uses 15T+ tokens from public sources for training, and the pre-training data deadline is December 2023;
Fine-tuning data includes public Available instruction fine-tuning dataset (unlike Llama 3) and 15 million synthetic samples;
- Model supports multiple languages, including English, French, German, Hindi, Italian, Portuguese, Spanish and Thai.
-
Although the leaked Github link is currently 404, some netizens have given download links ( However, for the sake of safety, it is recommended to wait for the official channel announcement tonight): 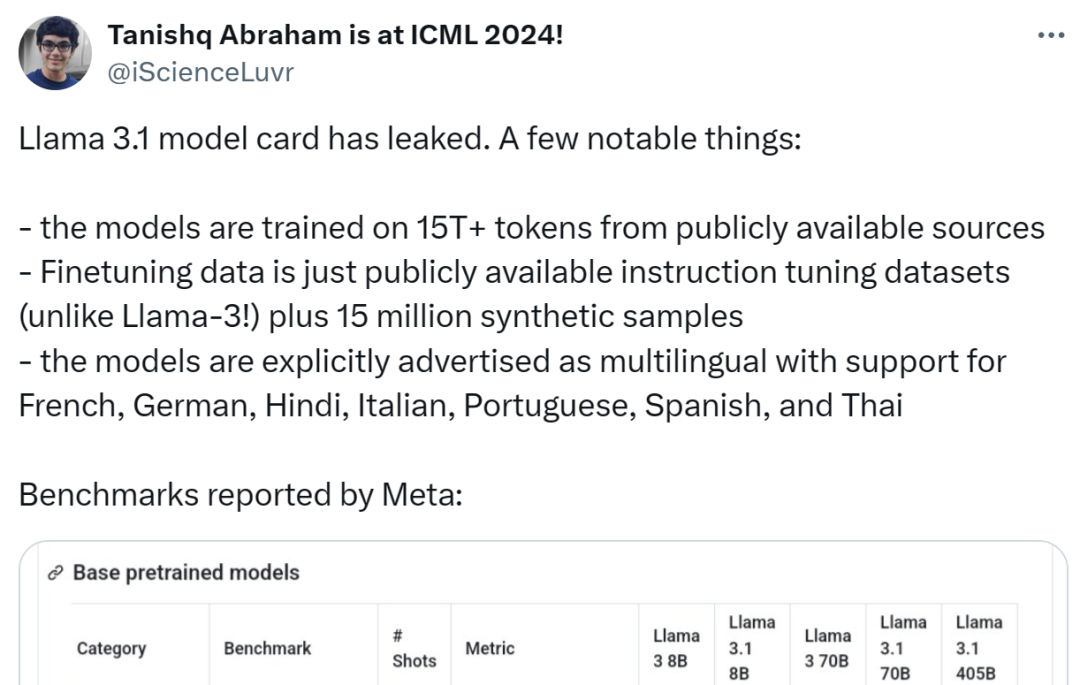
But this is a 100 billion-level model after all, please prepare enough hard disk space before downloading:
The following is the Llama 3.1 model Important content in the card: 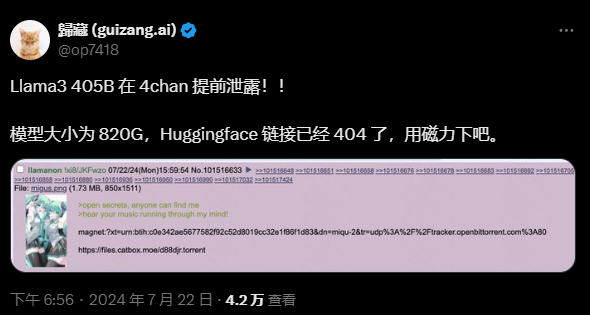
Basic model information
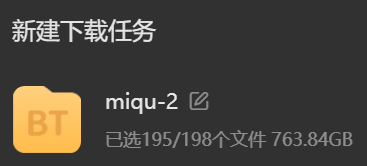
Meta Llama 3.1 Multilingual Large Language Model (LLM) collection is a set of pre-trained and instruction fine-tuned generative models, each 8B in size , 70B and 405B (text input/text output). Llama 3.1 command-fine-tuned text-only models (8B, 70B, 405B) are optimized for multilingual conversation use cases and outperform many available open and closed source chat models on common industry benchmarks. Model architecture: Llama 3.1 is an optimized Transformer architecture autoregressive language model. The fine-tuned version uses SFT and RLHF to align usability and security preferences.
Supported languages: English, German, French, Italian, Portuguese, Hindi, Spanish and Thai. It can be inferred from the model card information that the context length of the
Llama 3.1 series model is 128k. All model versions use Grouped Query Attention (GQA) to improve inference scalability.
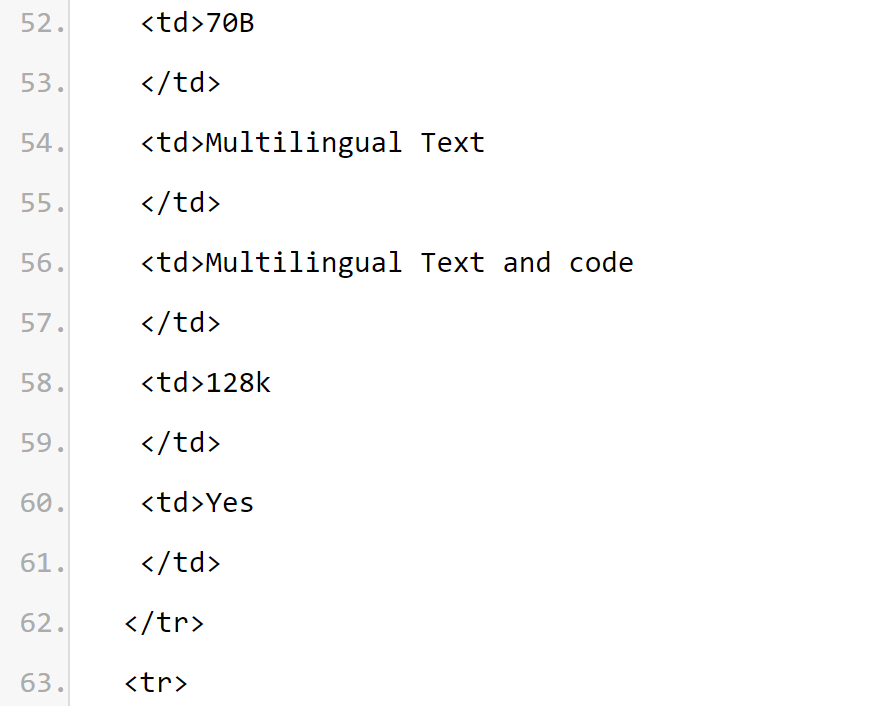
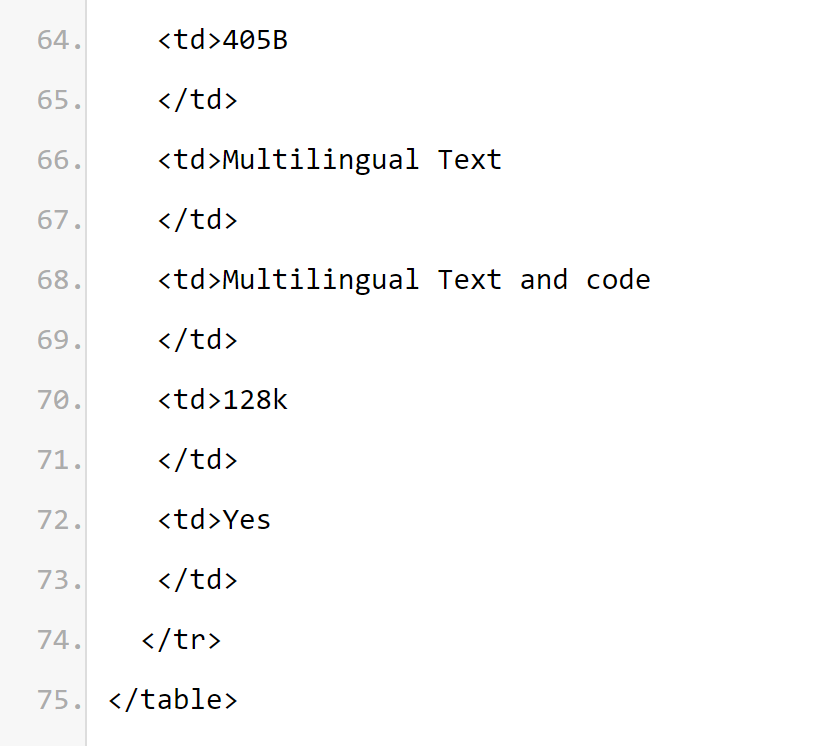
INTENDED USE CASE. Llama 3.1 is intended for multilingual business applications and research. Instruction-tuned text-only models are suitable for assistant-like chat, while pre-trained models can be adapted to a variety of natural language generation tasks. The Llama 3.1 model set also supports the ability to leverage its model output to improve other models, including synthetic data generation and distillation. The Llama 3.1 Community License allows these use cases. Llama 3.1 trains on a wider set of languages than the 8 supported languages. Developers may fine-tune Llama 3.1 models for languages other than the 8 supported languages, provided they comply with the Llama 3.1 Community License Agreement and Acceptable Use Policy, and are responsible in such cases for ensuring that other languages are used in a safe and responsible manner Language Llama 3.1. Software and hardware infrastructureThe first is the training element. Llama 3.1 uses a custom training library, Meta-customized GPU cluster and production infrastructure for pre-training, and is also fine-tuned on the production infrastructure. , annotation and evaluation. The second is the training energy consumption. Llama 3.1 training uses a total of 39.3 M GPU hours of calculation on H100-80GB (TDP is 700W) type hardware. Here training time is the total GPU time required to train each model, and power consumption is the peak power capacity of each GPU device, adjusted for power efficiency. Training on greenhouse gas emissions. Total greenhouse gas emissions during the Llama 3.1 training period based on a geographical baseline are estimated at 11,390 tonnes of CO2e. Since 2020, Meta has maintained net-zero greenhouse gas emissions across its global operations and matched 100% of its electricity use with renewable energy, resulting in total market-based greenhouse gas emissions of 0 tonnes of CO2e during the training period . The methods used to determine training energy use and greenhouse gas emissions can be found in the following paper. Because Meta releases these models publicly, others do not need to bear the burden of training energy usage and greenhouse gas emissions. Paper address: https://arxiv.org/pdf/2204.05149Overview: Llama 3.1 was conducted using approximately 1.5 trillion token data from public sources. Pre-training. Fine-tuning data includes publicly available instruction datasets, and over 25 million synthetically generated examples. Data freshness: The deadline for pre-training data is December 2023. In this section, Meta reports the scoring results of the Llama 3.1 model on the annotation benchmark. For all evaluations, Meta uses internal evaluation libraries. 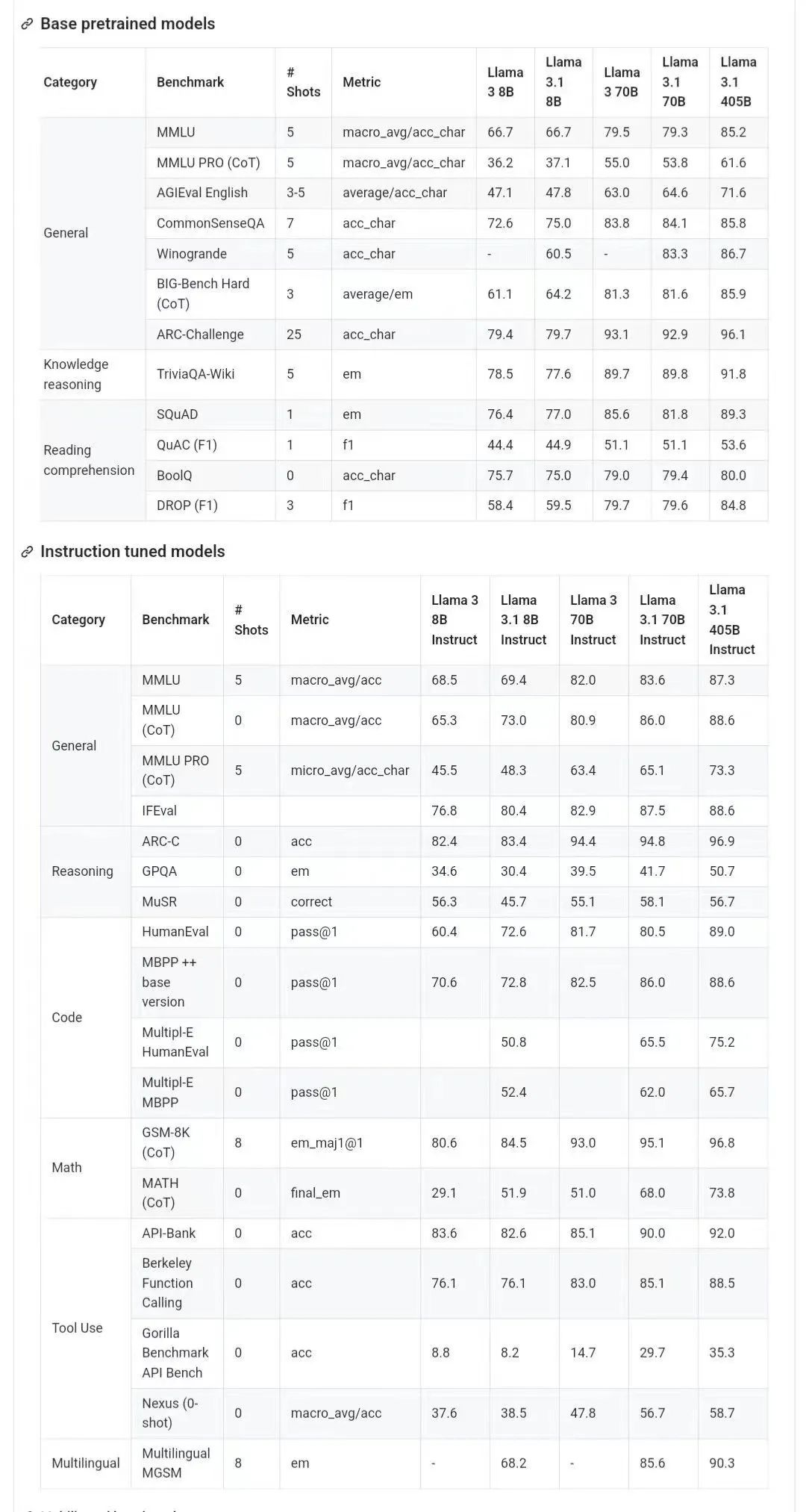
Llama 研究團隊致力於為研究界提供寶貴的資源來研究安全微調的穩健性,並為開發人員提供適用於各種應用的安全且強大的現成模型,以減少部署安全人工智慧系統的開發人員的工作量。 研究團隊採用多方面資料收集方法,將供應商的人工產生資料與合成資料結合,以減輕潛在的安全風險。研究團隊開發了許多基於大型語言模型 (LLM) 的分類器,以深思熟慮地選擇高品質的 prompt 和回應,從而增強資料品質控制。 值得一提的是,Llama 3.1 非常重視模型拒絕良性 prompt 以及拒絕語氣。研究團隊在安全資料策略中引入了邊界 prompt 和對抗性 prompt,並修改了安全資料回應以遵循語氣指南。 Llama 3.1 模型並非設計為單獨部署,而是應作為整個人工智慧系統的一部分進行部署,並根據需要提供額外的「安全護欄」。開發人員在建置智能體系統時應部署系統安全措施。 請注意,該版本引入了新功能,包括更長的上下文視窗、多語言輸入和輸出,以及開發人員與第三方工具的可能整合。使用這些新功能進行建置時,除了需要考慮一般適用於所有生成式人工智慧用例的最佳實踐外,還需要特別注意以下問題: 工具使用:與標準軟體開發一樣,由開發人員負責將LLM 與他們選擇的工具和服務整合。他們應為自己的使用案例制定明確的政策,並評估所使用的第三方服務的完整性,以了解使用此功能時的安全和安保限制。 多語言:Lama 3.1 除英語外還支援 7 種語言:法語、德語、印地語、義大利語、葡萄牙語、西班牙語和泰語。 Llama 可能可以輸出其他語言的文本,但這些文本可能不符合安全性和幫助性表現閾值。 Llama 3.1 的核心價值是開放、包容和樂於助人。它旨在服務每個人,並適用於各種使用情況。因此,Llama 3.1 的設計宗旨是讓不同背景、經驗和觀點的人都能使用。 Llama 3.1 以使用者及其需求為本,沒有插入不必要的評判或規範,同時也反映了這樣一種認識,即即使在某些情況下看似有問題的內容,在其他情況下也能達到有價值的目的。 Llama 3.1 尊重所有使用者的尊嚴和自主權,特別是尊重為創新和進步提供動力的自由思想和表達價值。 但 Llama 3.1 是一項新技術,與任何新技術一樣,其使用也存在風險。迄今為止進行的測試尚未涵蓋也不可能涵蓋所有情況。因此,與所有 LLM 一樣,Llama 3.1 的潛在輸出無法事先預測,在某些情況下,模型可能會對使用者提示做出不準確、有偏差或其他令人反感的反應。因此,在部署 Llama 3.1 模型的任何應用之前,開發人員應針對模型的特定應用進行安全測試和微調。 模型卡來源:https://pastebin.com/9jGkYbXY參考資訊:https://x.com/op74185001874720387418520374185203743720372727203838372370383838383838 https: //x.com/iScienceLuvr/status/1815519917715730702https://x.com/mattshumer_/status/1815444612414087294The above is the detailed content of The first open source model to surpass GPT4o level! Llama 3.1 leaked: 405 billion parameters, download links and model cards are available. For more information, please follow other related articles on the PHP Chinese website!
Statement:The content of this article is voluntarily contributed by netizens, and the copyright belongs to the original author. This site does not assume corresponding legal responsibility. If you find any content suspected of plagiarism or infringement, please contact admin@php.cn