Adaptive RAG: A Smarter Approach to Question Answering
Large language models (LLMs) excel at answering questions based on their training data, but this fixed knowledge base limits their ability to provide current or highly specific information. Retrieval-Augmented Generation (RAG) addresses this by allowing LLMs to access external data, but even RAG struggles with complex queries. Adaptive RAG offers a superior solution.
Adaptive RAG dynamically selects the optimal strategy for answering a question, choosing from a direct LLM response, a single data lookup, multiple data lookups, or even a web search. A classifier assesses the question's complexity to determine the most appropriate method.
Adaptive RAG employs three core answering strategies:
- Straightforward Response: For simple questions, the LLM utilizes its internal knowledge, providing rapid answers without external data retrieval.
- Single-step Approach: For moderately complex questions, the system retrieves information from a single external source, balancing speed and accuracy.
- Multi-step Approach: For the most intricate questions, the system consults multiple sources, constructing a comprehensive and nuanced response.
(Image showing Adaptive RAG's superior speed and performance compared to other RAG methods)
Why Adaptive RAG Excels:
Adaptive RAG's flexibility allows it to efficiently allocate resources, optimizing processing power and delivering precise answers swiftly. Its adaptive nature, based on question complexity, minimizes the risk of outdated or generalized responses, ensuring current, specific, and reliable answers. This adaptability significantly enhances accuracy.
Adaptive RAG Architecture:
Adaptive RAG is a sophisticated RAG architecture designed to enhance LLM query handling. It dynamically adjusts its answering strategy based on query complexity. A classifier evaluates the query and selects the best approach: straightforward, single-step retrieval, or multi-step retrieval.
(Image illustrating Adaptive RAG's decision-making process)
(Image comparing Adaptive RAG with single-step and multi-step approaches)
Building an Adaptive RAG System with LangGraph:
This section details building an Adaptive RAG application using the LangGraph framework. The application routes user questions to the most appropriate data source (vector database or web search). Key components include:
- Document Indexing: Documents are indexed using ChromaDB, a vector database within LangChain, enabling efficient retrieval of relevant information.
- Routing: A question router directs each query to the optimal data source.
- Retrieval Grader: Evaluates the relevance of retrieved documents.
- Generation: Combines prompts, LLMs, and retrieved data to generate answers.
- Hallucination Grader: Verifies the factual accuracy of the generated response.
- Answer Grader: Assesses whether the response fully answers the question.
- Question Rewriter: Refines the question for improved retrieval.
- Web Search: Performs web searches using a tool like Tavily Search.
- Graph Representation: LangGraph visualizes the application's workflow.
(Image depicting the system architecture)
(Image illustrating the workflow)
Implementation Steps (Detailed in the original text, omitted here for brevity).
Conclusion:
Adaptive RAG systems represent a significant advancement in question-answering AI. By dynamically adapting to question complexity, they achieve superior speed and accuracy compared to traditional methods. The flexibility and adaptability of Adaptive RAG, combined with frameworks like LangGraph, pave the way for more effective and user-friendly AI applications. As AI continues to evolve, Adaptive RAG will play a crucial role in building systems capable of understanding and responding to complex human queries with greater precision and efficiency.
The above is the detailed content of Guide to Adaptive RAG Systems with LangGraph. For more information, please follow other related articles on the PHP Chinese website!
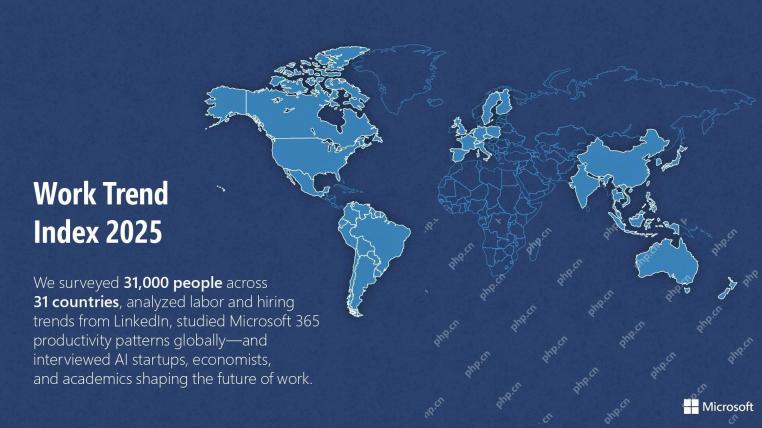
The burgeoning capacity crisis in the workplace, exacerbated by the rapid integration of AI, demands a strategic shift beyond incremental adjustments. This is underscored by the WTI's findings: 68% of employees struggle with workload, leading to bur
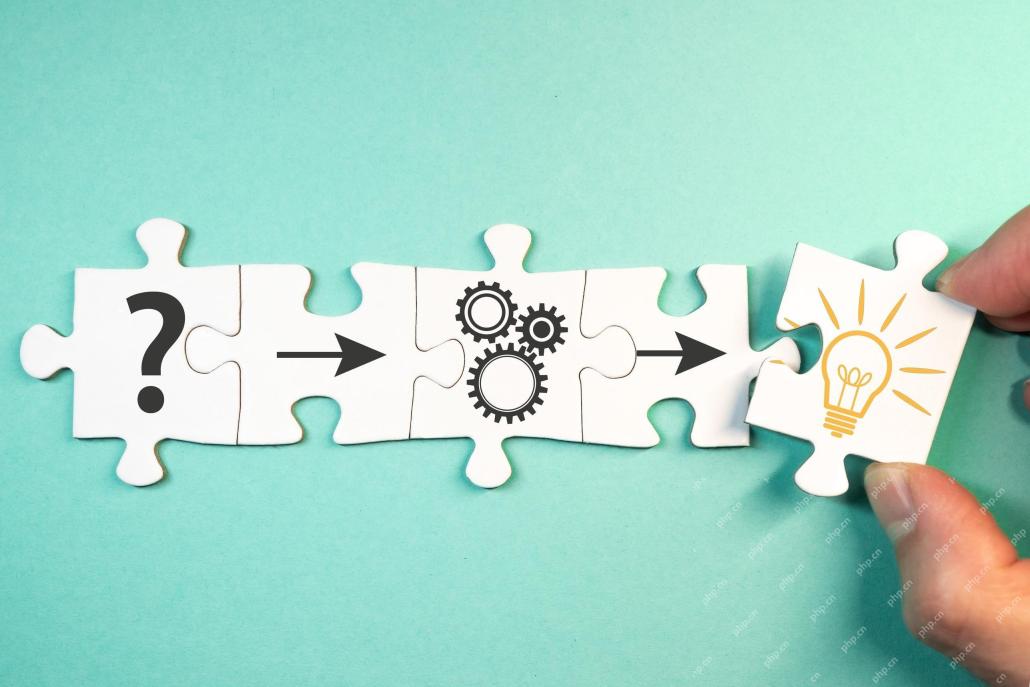
John Searle's Chinese Room Argument: A Challenge to AI Understanding Searle's thought experiment directly questions whether artificial intelligence can genuinely comprehend language or possess true consciousness. Imagine a person, ignorant of Chines
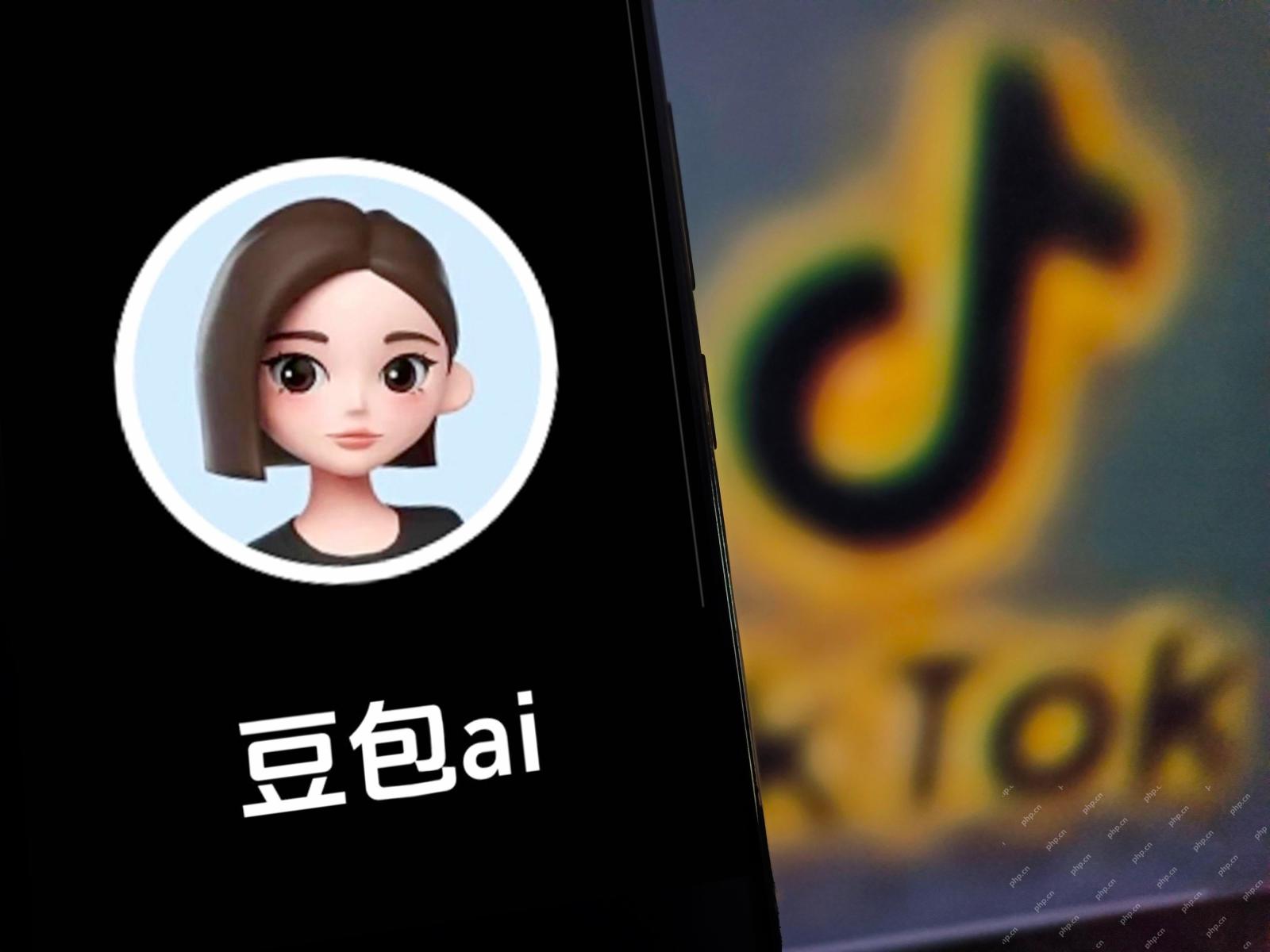
China's tech giants are charting a different course in AI development compared to their Western counterparts. Instead of focusing solely on technical benchmarks and API integrations, they're prioritizing "screen-aware" AI assistants – AI t
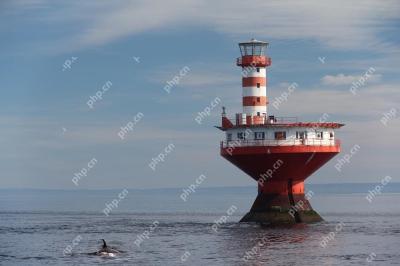
MCP: Empower AI systems to access external tools Model Context Protocol (MCP) enables AI applications to interact with external tools and data sources through standardized interfaces. Developed by Anthropic and supported by major AI providers, MCP allows language models and agents to discover available tools and call them with appropriate parameters. However, there are some challenges in implementing MCP servers, including environmental conflicts, security vulnerabilities, and inconsistent cross-platform behavior. Forbes article "Anthropic's model context protocol is a big step in the development of AI agents" Author: Janakiram MSVDocker solves these problems through containerization. Doc built on Docker Hub infrastructure
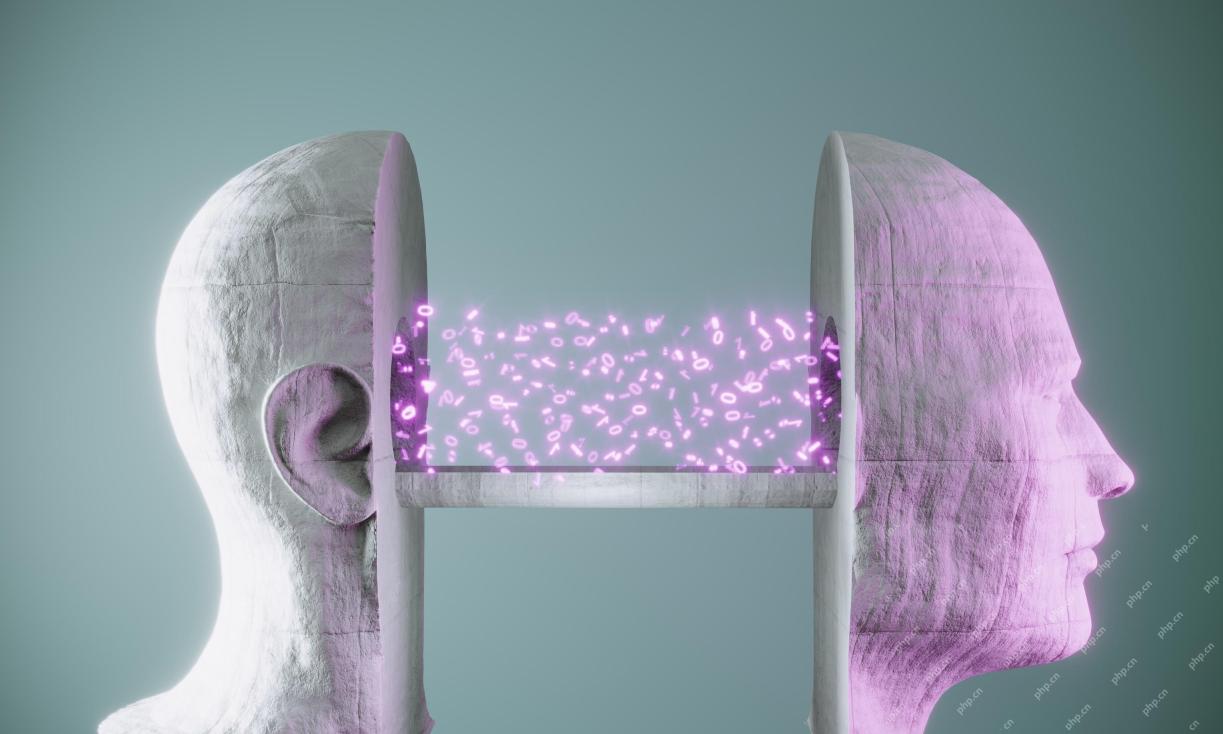
Six strategies employed by visionary entrepreneurs who leveraged cutting-edge technology and shrewd business acumen to create highly profitable, scalable companies while maintaining control. This guide is for aspiring entrepreneurs aiming to build a
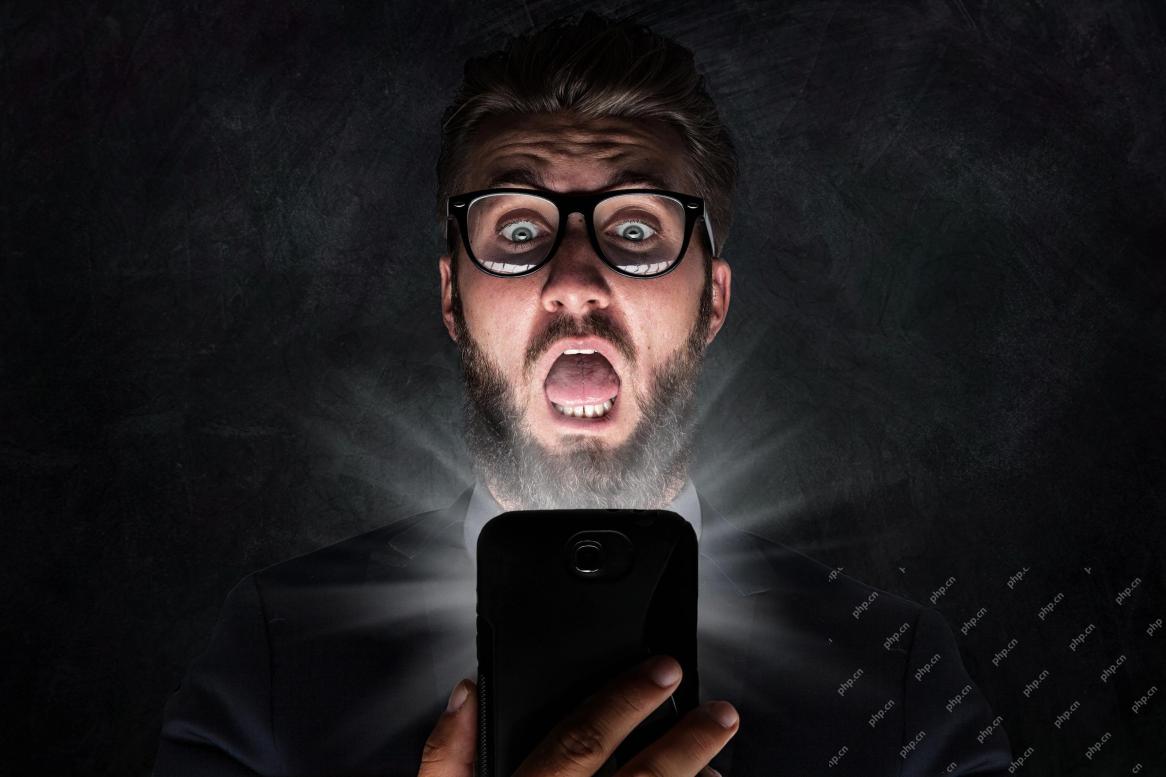
Google Photos' New Ultra HDR Tool: A Game Changer for Image Enhancement Google Photos has introduced a powerful Ultra HDR conversion tool, transforming standard photos into vibrant, high-dynamic-range images. This enhancement benefits photographers a
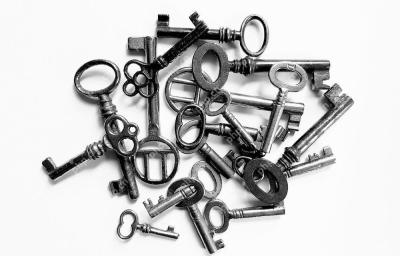
Technical Architecture Solves Emerging Authentication Challenges The Agentic Identity Hub tackles a problem many organizations only discover after beginning AI agent implementation that traditional authentication methods aren’t designed for machine-
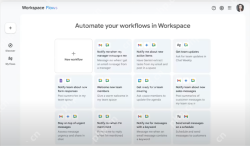
(Note: Google is an advisory client of my firm, Moor Insights & Strategy.) AI: From Experiment to Enterprise Foundation Google Cloud Next 2025 showcased AI's evolution from experimental feature to a core component of enterprise technology, stream


Hot AI Tools

Undresser.AI Undress
AI-powered app for creating realistic nude photos

AI Clothes Remover
Online AI tool for removing clothes from photos.

Undress AI Tool
Undress images for free

Clothoff.io
AI clothes remover

Video Face Swap
Swap faces in any video effortlessly with our completely free AI face swap tool!

Hot Article

Hot Tools
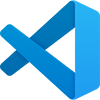
VSCode Windows 64-bit Download
A free and powerful IDE editor launched by Microsoft

Atom editor mac version download
The most popular open source editor
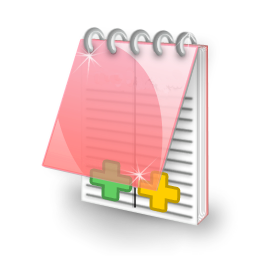
EditPlus Chinese cracked version
Small size, syntax highlighting, does not support code prompt function
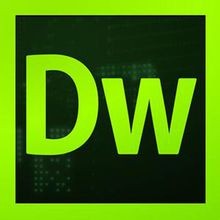
Dreamweaver CS6
Visual web development tools

SublimeText3 English version
Recommended: Win version, supports code prompts!
