The AIxiv column is a column where this site publishes academic and technical content. In the past few years, the AIxiv column of this site has received more than 2,000 reports, covering top laboratories from major universities and companies around the world, effectively promoting academic exchanges and dissemination. If you have excellent work that you want to share, please feel free to contribute or contact us for reporting. Submission email: liyazhou@jiqizhixin.com; zhaoyunfeng@jiqizhixin.com
3D generation is one of the most eye-catching topics in the field of generative artificial intelligence and computer graphics. 3D generation that meets film, television and game standards It is especially concerned by the industry. In the production process, 3D assets of general categories are often produced through manual modeling or scanning. However, as an important category of 3D assets, clothing assets are often derived from processes such as flat patterns and physical simulation, rather than directly modeling in 3D. Shanghai University of Science and Technology, Yingmo Technology and the University of Pennsylvania jointly proposed DressCode. It is the first 3D clothing generation framework that fully supports CG operations and is compatible with industrial processes. It can automatically generate high-quality clothing through text guidance. Quality rendering, editable, driveable, simulated 3D clothing.
Dresscode has been accepted by Transactions on Graphics, the top international journal in the field of computer graphics, and will be presented at SIGGRAPH 2024, the top international conference on computer graphics.
- Project link: https://sites.google.com/view/projectpage-dresscode
- Paper link: https://arxiv.org/abs/2401.16465
Clothing plays an important role in human appearance, which highlights the importance of clothing digitization for the creation of digital humans. Recent dramatic advances in 3D content creation are critical to the creation of digital humans.
However, there is still a vacancy in the work on clothing generation. Since the mesh or neural field generated by the general generation method is incompatible with the actual digital clothing production process, the direct application of this type of method to clothing categories has not achieved much success. Good results.
In order to solve these problems, the R&D team from Yingmo Technology and Shanghai University of Science and Technology proposed a text-driven 3D clothing generation framework-DressCode, aiming to simplify the design of digital clothing for beginners and improve the efficiency in fashion design. , virtual try-on and digital human creation offer huge potential.
This framework can generate clothing models that are friendly to the CG process through natural language interaction, and also facilitates pattern completion and texture editing, simplifying the design process through user-friendly interaction. This article will introduce the main functions of DressCode in detail and explore its application prospects in actual design scenarios.
SewingGPT Framework OverviewFor the pattern generation module, the paper proposes SewingGPT, which is an autoregressive model based on GPT for generating sewing patterns through text prompts. It first converts the sewing pattern parameters into a series of quantified tokens and trains a Decoder-only Transformer. And by integrating the cross-attention of text prompt words in the Transformer, the text can be used to guide the generated results. After the training is completed, the model can autoregressively generate a token sequence according to user conditions, and the generated sequence is reverse quantized to obtain the generated sewing pattern parameters. DressCode Framework OverviewWith SewingGPT, the framework is able to generate diverse sewing patterns directly from text prompts. Since material mapping is so critical in the CG pipeline, the framework generates corresponding physically based textures for these patterns, aligning more closely with the costume design workflow. The DressCode framework proposed by the authors uses SewingGPT and PBR texture generators to generate the geometric parts and texture parts of 3D clothing, and further utilizes large language models to create customized clothing for users through natural language interaction. After realizing the generation of sewing patterns and textures through text prompts, in order to enable designers to use natural language to interact with the generator in actual scenarios instead of relying on prompts in the dataset format, the authors adopted GPT- 4 Perform content learning to interpret the user’s natural language input and generate geometric prompt words and texture prompt words. After these prompt words are input into SewingGPT and PBR texture generator, the generated sewing patterns and textures are obtained, and the final results are obtained through simulation and rendering. At the same time, the generated clothing and PBR textures can be seamlessly integrated into industrial software, can be animated and driven with human models, and rendered under various lighting to ensure vivid and realistic effects. 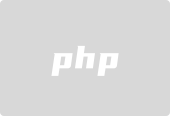
Pattern completion capabilityThanks to the autoregressive model, SewingGPT can complete the entire sewing pattern after receiving partial pattern information by utilizing the probability prediction provided by the model. In addition, entering a text prompt can guide the model to complete the sewing pattern. For example, after a sleeve is given, the model can complete various sewing patterns according to different prompts. This enables users to manually design partial patterns, use SewingGPT for inspiration and complete garments based on text prompts. Texture editing capabilityIn most recent 3D generation tasks, the inability to generate structured UV mapping is a major obstacle to practical application scenarios, especially when generating clothing, making it It cannot be used in actual design scenarios. The generative method proposed in this paper, utilizing sewing pattern representation, enables the creation of unique and structured UV maps for each pattern. This also enables users to easily edit textures at specific locations, supporting efficient post-processing of texture maps. DressCode framework has achieved excellent results in comparison with other methods. As the first garment generation work based on sewing patterns, the framework can enable beginners to Both designers and professional designers can generate high-quality sewing patterns and PBR textures through simple text prompts, greatly simplifying the clothing design process. DressCode’s ease of use and innovative approach promise to drive the future of digital clothing. This kind of generation task based on sewing patterns will promote the development of virtual try-on, fashion design and digital clothing created by digital people, which we look forward to together. The above is the detailed content of SIGGRAPH2024|Shanghai University of Science and Technology and Yingmu jointly proposed DressCode: generating 3D clothing patterns from text. For more information, please follow other related articles on the PHP Chinese website!
Statement:The content of this article is voluntarily contributed by netizens, and the copyright belongs to the original author. This site does not assume corresponding legal responsibility. If you find any content suspected of plagiarism or infringement, please contact admin@php.cn