


You can use your brain, never your hands.
In the future, you may be able to ask a robot to help you with housework just by thinking about it. The NOIR system recently proposed by the team of Wu Jiajun and Li Feifei of Stanford University allows users to control robots to complete daily tasks through non-invasive electroencephalography devices.
NOIR can decode your EEG signals into a robot skill library. It can now complete tasks such as cooking sukiyaki, ironing clothes, grating cheese, playing tic-tac-toe, and even petting a robot dog. This modular system has powerful learning capabilities and can handle complex and varied tasks in daily life.

The Brain and Robot Interface (BRI) is a masterpiece of human art, science and engineering. We have seen it in countless science fiction works and creative arts, such as "The Matrix" and "Avatar"; but truly realizing BRI is not easy and requires breakthrough scientific research to create a device that can perfectly coordinate with humans. functioning robotic system.
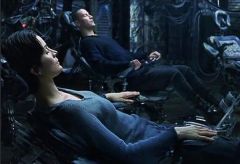
One key component for such a system is the ability of machines to communicate with humans. In the process of human-machine collaboration and robot learning, the ways humans communicate their intentions include actions, button presses, gaze, facial expressions, language, etc. Communicating directly with robots through neural signals is the most exciting but also most challenging prospect.
Recently, a multidisciplinary joint team led by Wu Jiajun and Li Feifei of Stanford University proposed a universal intelligent BRI system NOIR (Neural Signal Operated Intelligent Robots/Neural Signal Operated Intelligent Robots).
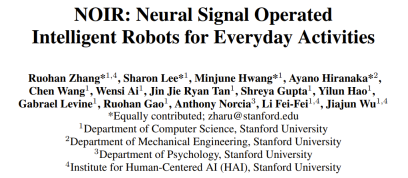
Paper address: https://openreview.net/pdf?id=eyykI3UIHa
Project website: https://noir-corl.github.io/
The system is based on non-invasive electroencephalography (EEG) technology. According to reports, the main principle based on this system is hierarchical shared autonomy, that is, humans define high-level goals, and robots achieve their goals by executing low-level movement instructions. The system incorporates new advances in neuroscience, robotics, and machine learning to achieve improvements over previous methods. The team summarizes the contributions made.
First of all, NOIR is versatile, can be used for diverse tasks, and is easy to use by different communities. Research shows that NOIR can complete up to 20 daily activities; in comparison, previous BRI systems were often designed for one or a few tasks, or were simply simulation systems. Additionally, the NOIR system can be used by the general population with minimal training.
Secondly, the I in NOIR means that the robot system is intelligent and has adaptive capabilities. The robot is equipped with a diverse repertoire of skills that allow it to perform low-level actions without intensive human supervision. Using parameterized skill primitives such as Pick (obj-A) or MoveTo (x,y), robots can naturally acquire, interpret, and execute human behavioral goals.
In addition, the NOIR system also has the ability to learn what humans want to achieve during the collaboration process. Research shows that by leveraging recent advances in underlying models, the system can adapt to even very limited data. This can significantly improve the efficiency of the system.
NOIR’s key technical contributions include a modular workflow for decoding neural signals to understand human intent. You know, decoding human intended goals from neural signals is extremely challenging. To do this, the team's approach is to break down human intention into three major components: the object to be manipulated (What), the way to interact with the object (How), and the location of the interaction (Where). Their research shows that these signals can be decoded from different types of neural data. These decomposed signals can naturally correspond to parameterized robot skills and can be effectively communicated to the robot.
Three human subjects successfully used the NOIR system in 20 home activities involving desktop or mobile operations (including making sukiyaki, ironing clothes, playing tic-tac-toe, petting a robot dog, etc.), That is, completing these tasks through their brain signals!
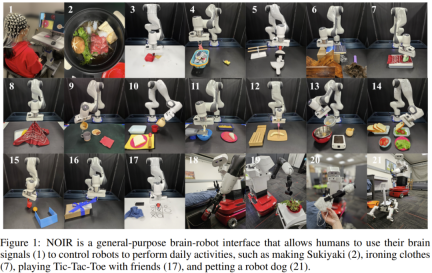
Experiments show that by using humans as teachers for few-shot robot learning, the efficiency of the NOIR system can be significantly improved. This method of using human brain signals to collaborate to build intelligent robotic systems has great potential to develop vital assistive technologies for people, especially those with disabilities, to improve their quality of life.
NOIR System
The challenges this research seeks to solve include: 1. How to build a universal BRI system suitable for various tasks? 2. How to decode relevant communication signals from the human brain? 3. How to improve the intelligence and adaptability of robots to achieve more efficient collaboration? Figure 2 gives an overview of the system.
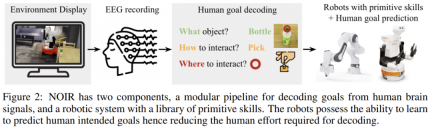
In this system, humans, as planning agents, perceive, plan, and communicate behavioral goals to the robot; while the robot uses predefined primitive skills to achieve these goals.
To achieve the overall goal of creating a universal BRI system, these two designs need to be collaboratively integrated. To this end, the team proposed a new brain signal decoding workflow and equipped the robot with a set of parameterized original skill libraries. Finally, the team used few-sample imitation learning technology to give the robot more efficient learning capabilities.
Brain: Modular decoding workflow
As shown in Figure 3, human intention will be decomposed into three components: the object to be manipulated (What), the way to interact with the object (How), and the interaction Where.
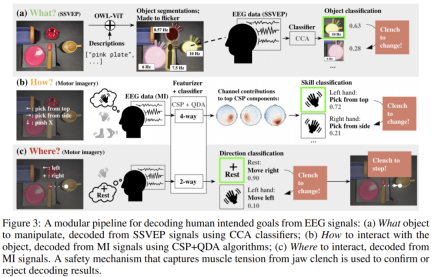
Decoding specific user intentions from EEG signals is not easy, but it can be accomplished through steady-state visual evoked potentials (SSVEP) and motor imagery. Briefly, the process includes:
Select an object with a Steady State Visual Evoked Potential (SSVEP)
Select skills and parameters via Motor Imagery (MI)
Select via muscle tightening to confirm or Interrupt
Robot: Parameterized primitive skills
Parameterized primitive skills can be combined and reused for different tasks to achieve complex and diverse operations. Furthermore, these skills are very intuitive to humans. Neither humans nor agents need to understand the control mechanisms of these skills, so people can implement these skills through any method as long as they are robust and adaptable to diverse tasks.
The team used two robots in the experiment: one is a Franka Emika Panda robotic arm for desktop operation tasks, and the other is a PAL Tiago robot for mobile operation tasks. The following table gives the primitive skills of these two robots.
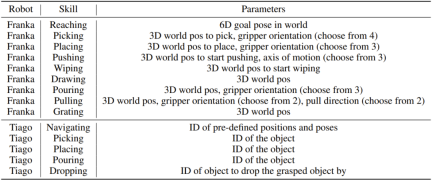
Using Robot Learning for Efficient BRI
The modular decoding workflow and primitive skill library described above lay the foundation for NOIR. However, the efficiency of such systems can be improved further. The robot should be able to learn the user's items, skills, and parameter selection preferences during the collaboration process, so that in the future it can predict the goals the user wants to achieve, achieve better automation, and make decoding simpler and easier. Since the position, pose, arrangement, and instance of the items may differ each time it is executed, learning and generalization capabilities are required. Additionally, learning algorithms should be highly sample efficient because collecting human data is expensive.
The team adopted two methods for this: retrieval-based few-sample item and skill selection, and single-sample skill parameter learning.
Retrieval-based few-sample item and skill selection. This method can learn implicit representations of the observed states. Given a new observed state, it finds the most similar state and corresponding action in the hidden space. Figure 4 gives an overview of the method.
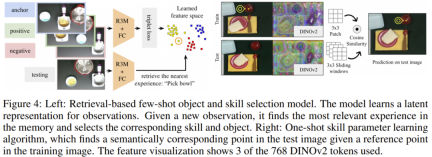
During mission execution, data points consisting of images and human-selected "item-skill" pairs are recorded. These images are first encoded by a pre-trained R3M model to extract features useful for robot manipulation tasks, and then passed through a number of trainable fully connected layers. These layers are trained using contrastive learning with a triplet loss, which encourages images with the same "item-skill" label to be closer together in the hidden space. The learned image embeddings and "item-skill" labels are stored in memory.
During testing, the model retrieves the nearest data point in the hidden space and then suggests the item-skill pair associated with that data point to the human.
Single sample skill parameter learning. Parameter selection requires extensive human involvement, as the process requires precise cursor operation through motor imagery (MI). To reduce human effort, the team proposed a learning algorithm that predicts parameters given an item-skill pair used as the starting point for cursor control. Assuming that the user has successfully located the precise key point of picking up a cup handle, does it need to specify this parameter again in the future? Recently, basic models such as DINOv2 have made a lot of progress, and the corresponding semantic key points can be found, eliminating the need to specify parameters again.
Compared with previous work, the new algorithm proposed here is single-sample and predicts specific 2D points rather than semantic fragments. As shown in Figure 4, given a training image (360 × 240) and parameter selection (x, y), the model predicts semantically corresponding points in different test images. Specifically, the team used the pre-trained DINOv2 model to obtain semantic features.
Experiments and results
missions. The tasks selected for the experiment come from the BEHAVIOR and Activities of Daily Living benchmarks, which can reflect human daily needs to a certain extent. Figure 1 shows the experimental tasks, which include 16 desktop tasks and 4 mobile operation tasks.
Examples of experimental processes for making sandwiches and caring for COVID-19 patients are shown below.


Experimental process. During the experiment, the user stayed in an isolated room, remained still, watched the robot on the screen, and relied solely on brain signals to communicate with the robot.
System performance. Table 1 summarizes the system performance under two metrics: the number of attempts before success and the time to complete the task upon success.
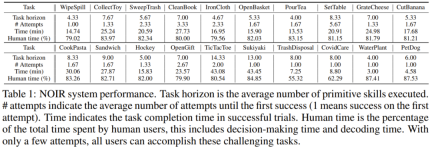
Despite the long span and difficulty of these tasks, NOIR achieved very encouraging results: on average, it only took 1.83 attempts to complete the tasks.
Decoding accuracy. The accuracy with which brain signals are decoded is a key to the success of the NOIR system. Table 2 summarizes the decoding accuracy at different stages. It can be seen that the CCA (canonical correlation analysis) based on SSVEP can achieve a high accuracy of 81.2%, which means that the item selection is generally accurate.

Item and skill selection results. So, can the newly proposed robot learning algorithm improve the efficiency of NOIR? The researchers first assessed item and skill selection learning. To do this, they collected an offline dataset for the MakePasta task, with 15 training samples for each item-skill pair. Given an image, when the correct item and skill are predicted simultaneously, the prediction is considered correct. The results are shown in Table 3.
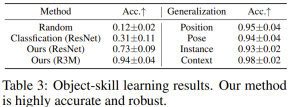
A simple image classification model using ResNet can achieve an average accuracy of 0.31, while the new method based on pre-trained ResNet backbone network can achieve a significantly higher 0.73, which highlights the contrastive learning and retrieval-based The importance of learning.
Results of single-sample parameter learning. The researchers compared the new algorithm against multiple benchmarks based on pre-collected data sets. Table 4 gives the MSE values of the predicted results.
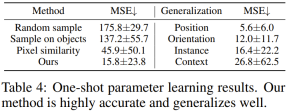
They also demonstrated the effectiveness of the parameter learning algorithm in actual task execution on the SetTable task. Figure 5 shows the human effort saved in controlling cursor movement.
The above is the detailed content of Li Feifei's team's new work: brain-controlled robots do housework, giving brain-computer interfaces the ability to learn with few samples. For more information, please follow other related articles on the PHP Chinese website!
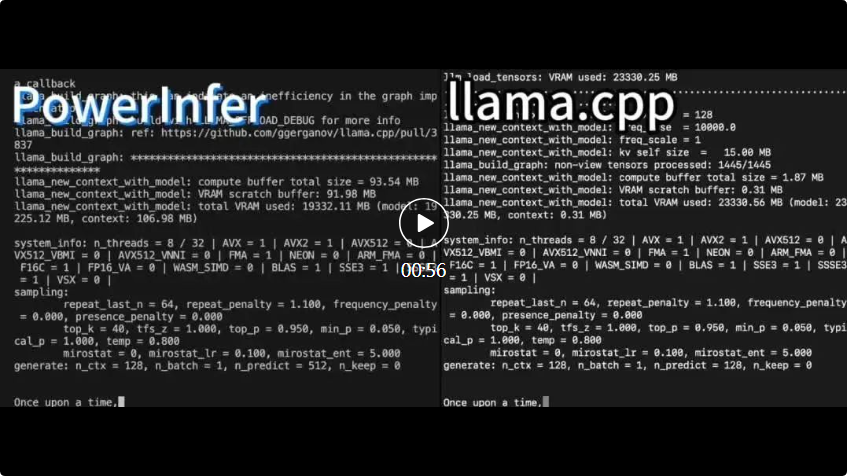
PowerInfer提高了在消费级硬件上运行AI的效率上海交大团队最新推出了超强CPU/GPULLM高速推理引擎PowerInfer。PowerInfer和llama.cpp都在相同的硬件上运行,并充分利用了RTX4090上的VRAM。这个推理引擎速度有多快?在单个NVIDIARTX4090GPU上运行LLM,PowerInfer的平均token生成速率为13.20tokens/s,峰值为29.08tokens/s,仅比顶级服务器A100GPU低18%,可适用于各种LLM。PowerInfer与
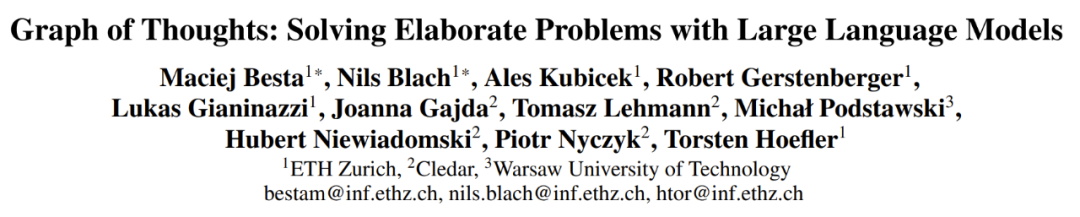
要让大型语言模型(LLM)充分发挥其能力,有效的prompt设计方案是必不可少的,为此甚至出现了promptengineering(提示工程)这一新兴领域。在各种prompt设计方案中,思维链(CoT)凭借其强大的推理能力吸引了许多研究者和用户的眼球,基于其改进的CoT-SC以及更进一步的思维树(ToT)也收获了大量关注。近日,苏黎世联邦理工学院、Cledar和华沙理工大学的一个研究团队提出了更进一步的想法:思维图(GoT)。让思维从链到树到图,为LLM构建推理过程的能力不断得到提升,研究者也通
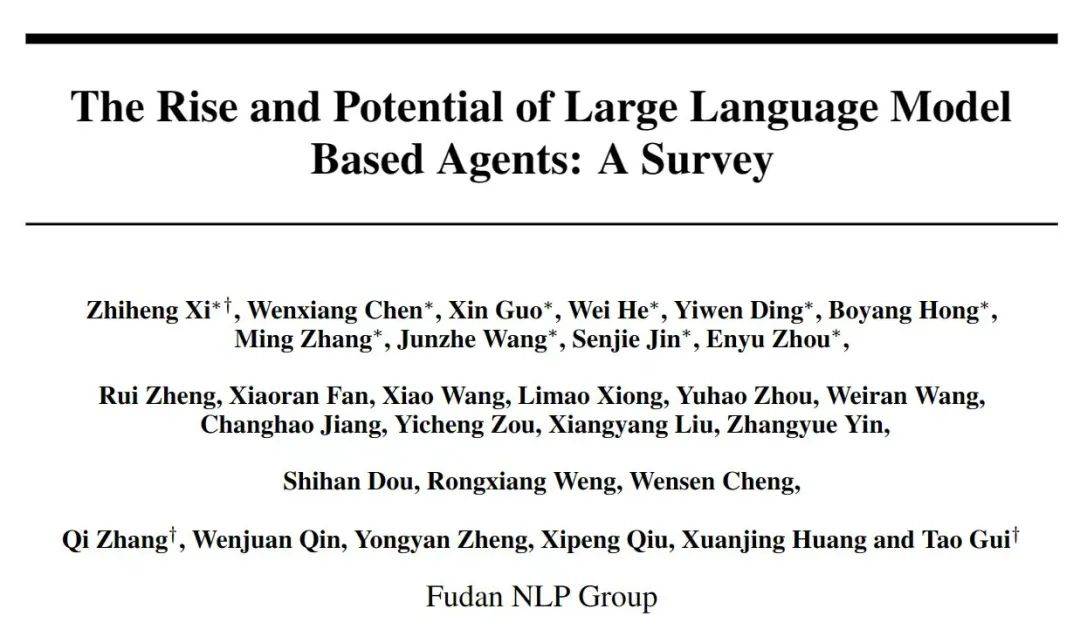
近期,复旦大学自然语言处理团队(FudanNLP)推出LLM-basedAgents综述论文,全文长达86页,共有600余篇参考文献!作者们从AIAgent的历史出发,全面梳理了基于大型语言模型的智能代理现状,包括:LLM-basedAgent的背景、构成、应用场景、以及备受关注的代理社会。同时,作者们探讨了Agent相关的前瞻开放问题,对于相关领域的未来发展趋势具有重要价值。论文链接:https://arxiv.org/pdf/2309.07864.pdfLLM-basedAgent论文列表:
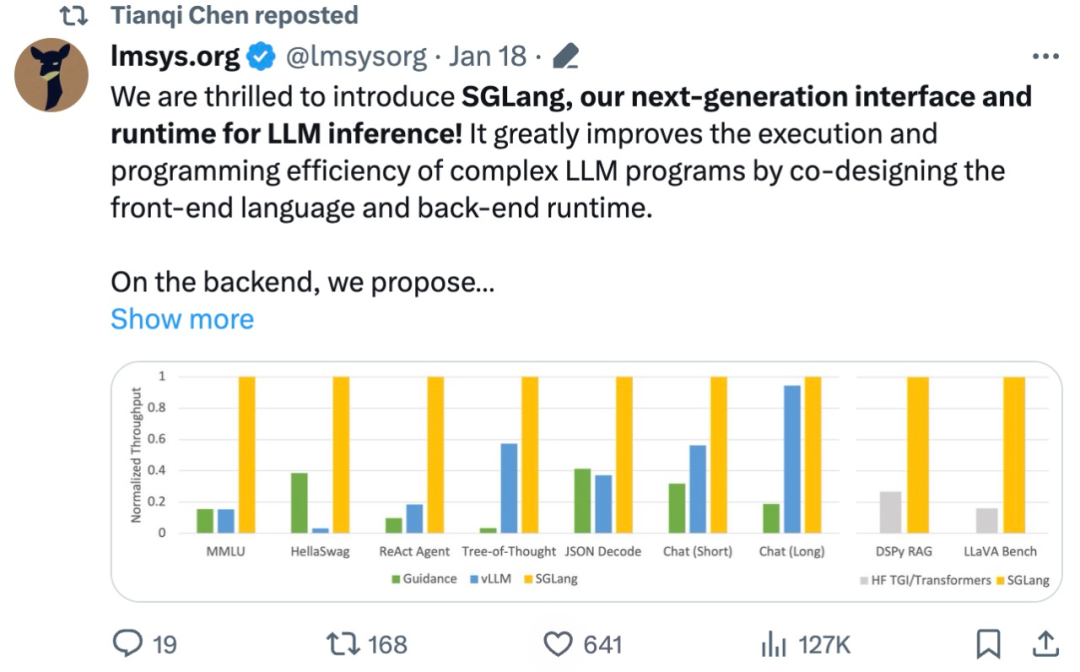
大型语言模型(LLM)被广泛应用于需要多个链式生成调用、高级提示技术、控制流以及与外部环境交互的复杂任务。尽管如此,目前用于编程和执行这些应用程序的高效系统却存在明显的不足之处。研究人员最近提出了一种新的结构化生成语言(StructuredGenerationLanguage),称为SGLang,旨在改进与LLM的交互性。通过整合后端运行时系统和前端语言的设计,SGLang使得LLM的性能更高、更易控制。这项研究也获得了机器学习领域的知名学者、CMU助理教授陈天奇的转发。总的来说,SGLang的
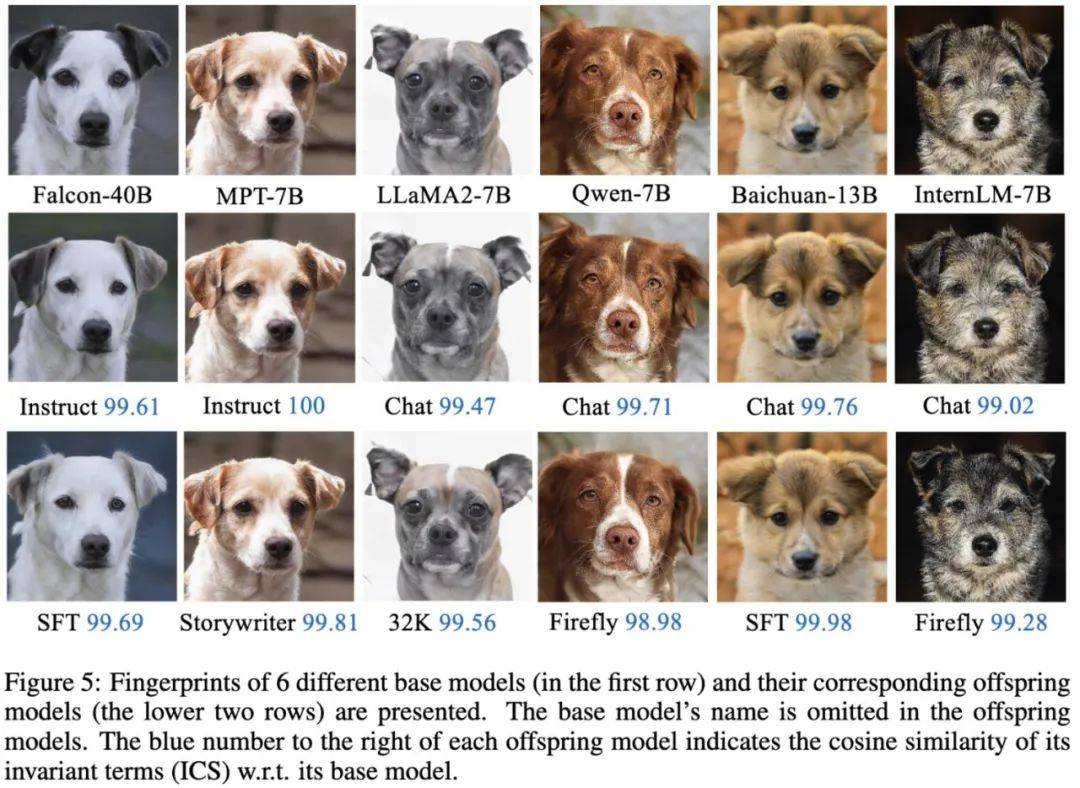
将不同的基模型象征为不同品种的狗,其中相同的「狗形指纹」表明它们源自同一个基模型。大模型的预训练需要耗费大量的计算资源和数据,因此预训练模型的参数成为各大机构重点保护的核心竞争力和资产。然而,与传统软件知识产权保护不同,对预训练模型参数盗用的判断存在以下两个新问题:1)预训练模型的参数,尤其是千亿级别模型的参数,通常不会开源。预训练模型的输出和参数会受到后续处理步骤(如SFT、RLHF、continuepretraining等)的影响,这使得判断一个模型是否基于另一个现有模型微调得来变得困难。无
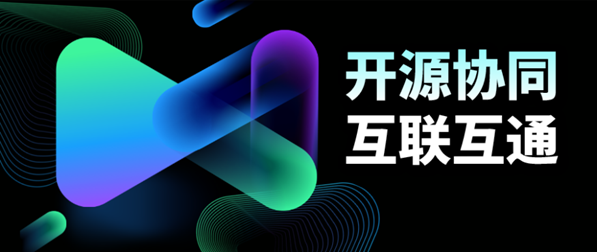
FATE2.0全面升级,推动隐私计算联邦学习规模化应用FATE开源平台宣布发布FATE2.0版本,作为全球领先的联邦学习工业级开源框架。此次更新实现了联邦异构系统之间的互联互通,持续增强了隐私计算平台的互联互通能力。这一进展进一步推动了联邦学习与隐私计算规模化应用的发展。FATE2.0以全面互通为设计理念,采用开源方式对应用层、调度、通信、异构计算(算法)四个层面进行改造,实现了系统与系统、系统与算法、算法与算法之间异构互通的能力。FATE2.0的设计兼容了北京金融科技产业联盟的《金融业隐私计算
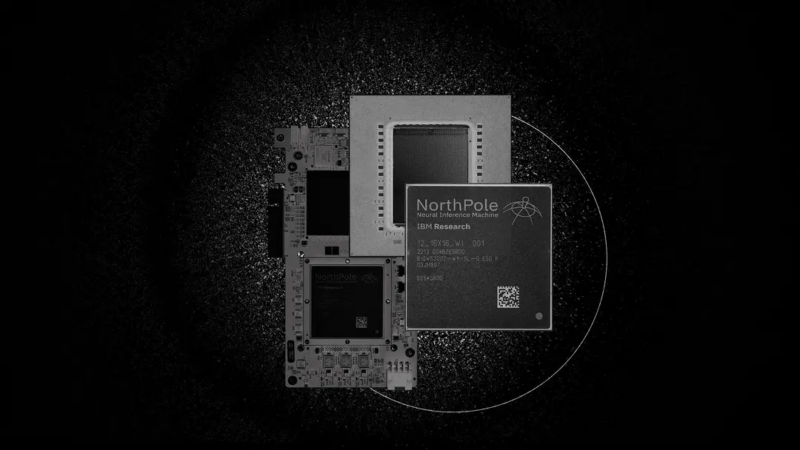
IBM再度发力。随着AI系统的飞速发展,其能源需求也在不断增加。训练新系统需要大量的数据集和处理器时间,因此能耗极高。在某些情况下,执行一些训练好的系统,智能手机就能轻松胜任。但是,执行的次数太多,能耗也会增加。幸运的是,有很多方法可以降低后者的能耗。IBM和英特尔已经试验过模仿实际神经元行为设计的处理器。IBM还测试了在相变存储器中执行神经网络计算,以避免重复访问RAM。现在,IBM又推出了另一种方法。该公司的新型NorthPole处理器综合了上述方法的一些理念,并将其与一种非常精简的计算运行
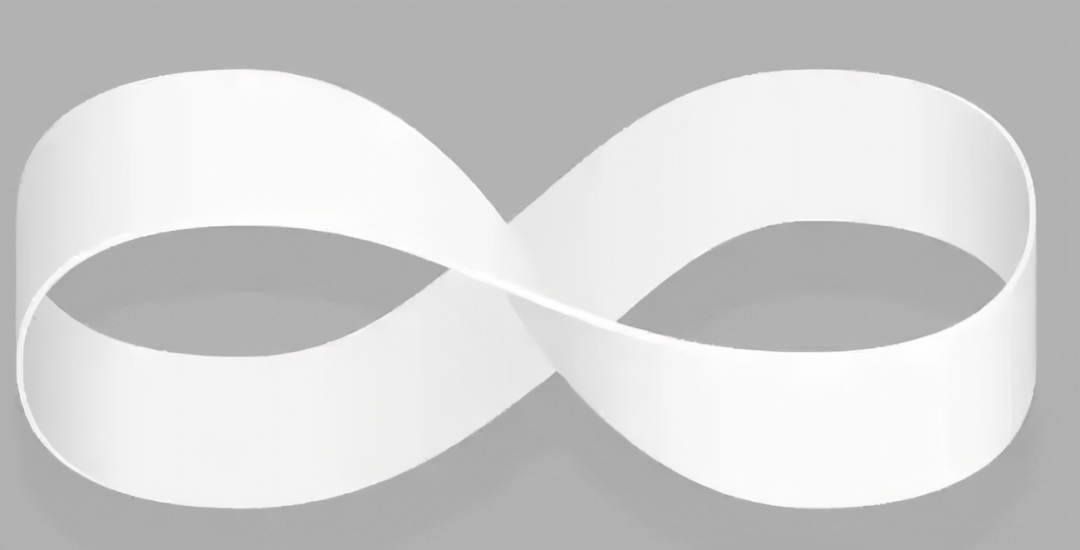
自己动手做过莫比乌斯带吗?莫比乌斯带是一种奇特的数学结构。要构造一个这样美丽的单面曲面其实非常简单,即使是小孩子也可以轻松完成。你只需要取一张纸带,扭曲一次,然后将两端粘在一起。然而,这样容易制作的莫比乌斯带却有着复杂的性质,长期吸引着数学家们的兴趣。最近,研究人员一直被一个看似简单的问题困扰着,那就是关于制作莫比乌斯带所需纸带的最短长度?布朗大学RichardEvanSchwartz谈到,对于莫比乌斯带来说,这个问题没有解决,因为它们是「嵌入的」而不是「浸入的」,这意味着它们不会相互渗透或自我


Hot AI Tools

Undresser.AI Undress
AI-powered app for creating realistic nude photos

AI Clothes Remover
Online AI tool for removing clothes from photos.

Undress AI Tool
Undress images for free

Clothoff.io
AI clothes remover

AI Hentai Generator
Generate AI Hentai for free.

Hot Article

Hot Tools
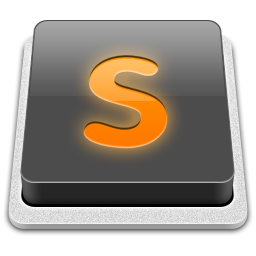
SublimeText3 Mac version
God-level code editing software (SublimeText3)

MinGW - Minimalist GNU for Windows
This project is in the process of being migrated to osdn.net/projects/mingw, you can continue to follow us there. MinGW: A native Windows port of the GNU Compiler Collection (GCC), freely distributable import libraries and header files for building native Windows applications; includes extensions to the MSVC runtime to support C99 functionality. All MinGW software can run on 64-bit Windows platforms.

Atom editor mac version download
The most popular open source editor
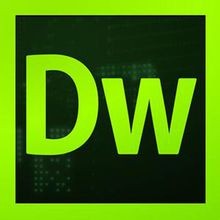
Dreamweaver CS6
Visual web development tools
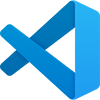
VSCode Windows 64-bit Download
A free and powerful IDE editor launched by Microsoft