背景
1、引言
知識圖譜的概念,最早由Google 在2012 年提出, 旨在實現更聰明的搜尋引擎,並在2013年後開始在學術界和工業級普及。目前,隨著人工智慧技術的高速發展,知識圖譜已廣泛應用於搜尋、推薦、廣告、風控、智慧調度、語音辨識、機器人等多個領域。
2、發展現況
知識圖譜作為人工智慧的核心技術驅動力,能緩解深度學習依賴海量訓練資料、大規模算力的問題,它能夠廣泛適配不同的下游任務,且具有良好的解釋性,因此,全球大型網路公司都在積極部署本企業的知識圖譜。
例如2013年Facebook發表Open Graph,應用於社群網路智慧搜尋;2014年百度推出的知識圖譜,主要應用於搜尋、助理、及toB商業場景;2015年阿里推出的商品知識圖譜,在前端導購、平台治理和智慧問答等業務上發揮關鍵作用;騰訊於2017年推出的騰訊雲知識圖譜,有效助力於金融搜尋、實體風險預測等場景;美團於2018年推出的美團大腦知識圖譜,已經在智慧搜尋推薦、智慧商家營運等多個業務中落地。
3、目標及效益
目前,領域圖譜主要集中在電商、醫療、金融等商業領域,而汽車知識的語意網絡及知識圖譜建構缺乏系統性的指導方法。本文以汽車領域知識為例,圍繞車系、車型、經銷商、廠商、品牌等實體及相互關係,提供一種從零搭建領域圖譜的思路,並對搭建知識圖譜中的步驟及方法進行了詳細說明,以及介紹了基於本圖譜的幾個典型落地應用。
其中,資料來源採用汽車之家網站,汽車之家是由導購、資訊、評測、口碑等多個板塊組成的汽車服務類平台,在看、買、用等維度積累了大量的汽車數據,透過建構知識圖譜把以汽車為核心的內容進行組織和挖掘,提供豐富的知識信息,結構化精準刻畫興趣,支持推薦用戶冷啟、召回、排序、展示等多個維度,給業務提升帶來效果。
二、圖譜建構
1、建構的挑戰
知識圖譜為真實世界的語意表示,,其基本組成單位為【實體-關係-實體】, 【實體-屬性-屬性值】的三元組(Triplet),實體之間透過關係相互聯結,從而構成語意網路。圖譜建構中會面臨較大的挑戰,但建構之後,可在資料分析、建議計算、可解釋性等多個場景展現豐富的應用價值。
建構挑戰:
- schema難定義:目前尚無統一成熟的本體建構流程,且特定領域本體定義通常需專家參與;
- 資料型態異質:通常情況下,一個知識圖譜建構中面對的資料來源不會是單一型,包含結構化、半結構化,及非結構化資料,面對結構各異的資料,知識轉模及挖掘的難度較高;
- 依賴專業知識:領域知識圖譜通常依賴較強的專業知識,例如車型對應的維修方法,涉及機械、電工、材料、力學等多個領域知識,且此類關係對於準確度的要求較高,需要保證知識足夠正確,因此也需要較好的專家和演算法相結合的方式來進行高效的圖譜構建;
- 數據品質無保證:挖掘或抽取資訊需要知識融合或人工校驗,才能作為知識助力下游應用。
收益:
- 知識圖譜統一知識表示:透過整合多源異質數據,形成統一視圖;
- 語意資訊豐富:透過關係推理可以發現新關係邊,獲得更豐富的語意資訊;
- 可解釋性強:顯式的推理路徑對比深度學習結果具有更強的解釋性;
- 高品質且能持續累積:根據業務場景設計合理的知識儲存方案,實現知識更新和累積。
2、圖譜架構設計
技術架構主要分為建構層、儲存層及應用層三大層,架構圖如下:
- 建構層:包括schema定義,結構化資料轉模,非結構化資料探勘,以及知識融合;
- 儲存層:包括知識的儲存和索引,知識更新,元數據管理,以及支援基本的知識查詢;
- 服務層:包含智慧推理、結構化查詢等業務相關的下游應用層。
3、具體建置步驟及流程
依據架構圖,具體建構流程可分為四個步驟:本體設計、知識獲取、知識入庫,以及應用服務設計及使用。
3.1 本體建構
本體(Ontology)是公認的概念集合,本體的建構是指依據本體的定義,建構出知識圖譜的本體結構和知識架構。
基於本體建立圖譜的原因主要有以下幾點:
- #明確專業術語、關係及其領域公理,當一條資料必須滿足Schema預先定義好的實體物件和類型後,才允許被更新到知識圖譜中。
- 將領域知識與操作性知識分離,透過Schema可以宏觀了解圖譜架構及相關定義,無須再從三元組中歸納整理。
- 實現一定程度的領域知識重複使用。在建構本體之前,可以先研究是否有相關本體已經被建構出來了,這樣可以基於已有本體進行改進和擴展,達到事半功倍的效果。
- 基於本體的定義,可以避免圖譜與應用脫節,或修改圖譜schema比重新建構成本還要高的情況。例如將「BMWx3」、「2022寶馬x3」都當作汽車類實體來儲存,在應用時都可能造成實例關係混亂、可用性差的問題,這種情況可以在設本體計階段,透過將「汽車類實體」進行「車系」、「車型」子類細分的方法來避免。
依照知識的覆蓋率來看,知識圖譜可以劃分為通用知識圖譜和領域知識圖譜,目前通用知識圖譜已有較多案例,例如Google的Knowledge Graph、微軟的Satori和Probase等,領域圖譜則為金融、電商等具體產業圖譜。通用圖譜較注重廣度,強調融合較多的實體數量,但對精確度的要求不高,很難藉助本體庫對公理、規則及約束條件進行推理和使用;而領域圖譜的知識覆蓋範圍較小,但知識深度更深,往往是某一專業領域的建構。
考慮準確率的要求,領域本體建構多傾向於手工建構的方式,例如代表性的七步法、IDEF5方法等[1],該類方法的核心思想是,基於已有結構化數據,進行本體分析,將符合應用目的和範圍的本體進行歸納及構建,再對本體進行優化和驗證,從而獲取初步版本體定義。若想取得更大範疇的領域本體,則可以從非結構化語料中補充,考慮手工構建過程較大,本文以汽車領域為例,提供一種半自動本體構建的方式,詳細步驟如下:
- 首先收集大量汽車非結構化語料(例如車系諮詢、新車導購文章等),作為初始個體概念集,利用統計方法或無監督模型(TF-IDF、 BERT等)取得字特徵與字特徵;
- 其次利用BIRCH聚類演算法對概念間層次劃分,初步建構起概念間層級關係,並對聚類結果進行人工概念校驗與歸納,取得本體的等價、上下位概念;
- 最後使用卷積神經網路結合遠端監督的方法,抽取本體屬性的實體關係,並輔以人工識別本體中的類別及屬性的概念,建構起汽車領域本體。
上述方法可有效利用BERT等深度學習的技術,更好地捕捉語料間的內部關係,使用聚類分層次對本體各模組進行構建,輔以人工幹預,能夠快速、準確的完成初步本體建構。下圖為半自動化本體建構示意圖:
利用Protégé本體建構工具[2],可以進行本體概念類別、關係、屬性和實例的構建,下圖為本體構建視覺化範例圖:
#本文將汽車領域的頂層本體概念劃分為三類,實體、事件及標籤體系:
1)實體類別代表特定意義的概念類別實體,包括詞彙實體和汽車類別實體,其中汽車類別實體又包括組織機構和汽車概念等子實體類型;
2)標籤體系代表各個維度的標籤體系,包括內容分類、概念標籤、興趣標籤等以物料維度刻畫的標籤;
#3)事件類別代表一個或多個角色的客觀事實,不同類型事件間具有演變關係。
Protégé可以匯出不同類型的Schema設定文件,其中owl.xml結構設定檔如下圖所示。此設定檔可直接在MYSQL、JanusGraph中載入使用,實現自動化的建立Schema。
3.2 知識獲取
知識圖譜的資料來源通常包含三類資料結構,分別為結構化資料、半結構化資料、非結構化資料。 面向不同類型的資料來源,知識擷取所涉及的關鍵技術和需要解決的技術難度有所不同。
3.2.1 結構化知識轉模
結構化資料是圖譜最直接的知識來源,基本上透過初步轉換就可以使用,相較其他類型資料成本最低,所以一般圖譜資料優先考慮結構化資料。結構化資料可能涉及多個資料庫來源,通常需要使用ETL方法轉模,ETL即Extract(抽取)、Transform(轉換)、Load(裝載),抽取是將資料從各種原始的業務系統中讀取出來,這是所有工作的前提;轉換是按照預先設計好的規則將抽取的數據進行轉換,使本來異質的數據格式可以統一起來;裝載是將轉換完的數據按計劃增量或全部導入到數據倉庫中。
透過上述ETL流程可將不同來源資料落到中間表,以便於後續的知識入庫。下圖為車系實體屬性、關係表示例圖:
車系與品牌關係表:
3.2.2 非結構化知識抽取-三元組抽取
除了結構化數據,非結構化資料中也存在著海量的知識(三元組)資訊。一般來說企業的非結構化資料量遠大於結構化數據,挖掘非結構化知識能夠大幅拓展和豐富知識圖譜。
三元組抽取演算法的挑戰
問題1:單一領域內,⽂檔內容和格式多樣,需要⼤量的標註數據,成本⾼
問題2:領域之間遷移的效果不夠好,跨領域的可規模化拓展的代價⼤
模型基本上都是針對特定⾏業特定場景,換⼀個場景,效果會出現明顯下降。
解決思路,Pre-train Finetune的範式,預訓練:重量級底座讓模型「⻅多識⼴」充分利⽤⼤規模多⾏業的⽆標⽂檔,訓練⼀個統⼀的預訓練底座,增強模型對各類⽂檔的表示與理解能⼒。
微調:輕量⽂檔結構化演算法。在預訓練基礎上,建構輕量級的⾯向⽂檔結構化的演算法,降低標註成本。
針對⽂檔的預訓練⽅法
現有關於⽂檔的預訓練模型,如果文字較短的類型,Bert可以完全編碼整篇⽂檔;⽽我們實際的⽂檔通常⽐較⻓,需要抽取的屬性值有很多是超過1024個字的,Bert進⾏編碼會造成屬性值截斷。
針對長文本預訓練方法優點和不足
Sparse Attention的⽅法透過優化Self-Attention,將O(n2)的計算優化⾄O(n ),⼤⼤提⾼了輸⼊⽂本⻓度。雖然普通模型的⽂本⻓度從512提升到4096,但是依舊不能完全解決截斷⽂本的碎⽚化問題。百度提出了ERNIE-DOC[3]使用了Recurrence Transformer方法,理論上可以建模⽆限⻓的⽂本。由於建模要輸⼊所有的⽂本訊息,耗時⾮常⾼。
上述兩種基於⻓⽂本的預訓練⽅法,都沒有考慮⽂檔特性,如空間(Spartial)、視覺(Visual)等資訊。並且基於⽂本設計的PretrainTask,整體是針對純⽂本進⾏的設計,⽽沒有針對⽂檔的邏輯結構設計。
針對上述不足這裡介紹一種⻓⽂檔預訓練模型DocBert[4],DocBert模型設計:
使⽤⼤規模(百萬)⽆標註⽂檔資料進⾏預訓練,基於⽂檔的⽂本語意(Text)、版⾯資訊(Layout)、視覺特徵(Visual)建構⾃監督學習任務,使模型更能理解⽂檔語義和結構資訊。
1.Layout-Aware MLM:在Mask語⾔模型中考慮⽂本的位置、字體⼤⼩訊息,實現⽂檔佈局感知的語義理解。
2.Text-Image Alignment:融合⽂檔視覺特徵,重建影像中被Mask的⽂字,幫助模型學習⽂本、版⾯、影像不同模態間的對⻬關係。
3.Title Permutation:以⾃監督的⽅式建構標題重建任務,增強模型對⽂檔邏輯結構的理解能⼒。
4.Sparse Transformer Layers:⽤Sparse Attention的⽅法,增強模型對⻓⽂檔的處理能⼒。
3.2.3 挖掘概念,興趣詞標籤,關聯到車系、實體
除了結構化和非結構化文字中取得三元組,汽車之家也挖掘物料所包含的分類、概念標籤和興趣關鍵字標籤,並建立物料和車實體之間的關聯,為汽車知識圖譜帶來新的知識。以下從分類、概念標籤、興趣詞標籤來介紹汽車之家所做的內容理解部分工作以及思考。
分類體係作為內容刻畫基礎,對物料進行粗粒度的劃分。建立的統一的內容體系更多的是基於人工定義的方式,透過AI模型進行劃分。在分類方法上我們我們採用了主動學習,對於比較難分的資料進行標註,同時採用資料增強,對抗訓練,以及關鍵字融合方式提高分類的效果。
概念標籤粒度介於分類和興趣詞標籤之間,比分類粒度更細,同時比興趣詞對於興趣點刻畫更加完整,我們建立了車視野、人視野、內容視野三個維度,豐富了標籤維度,細化了標籤粒度。豐富且具體的物料標籤,更方便搜尋推薦基於標籤的模型優化,且可用於標籤外展起到吸引用戶及二次引流等作用。概念標籤的挖掘,結合在query等重要資料上採用機器挖掘方式,並對概括性進行分析,透過人工review,拿到概念標籤集合,採用多標籤模型分類。
興趣詞標籤是最細粒度的標籤,映射為使用者興趣,根據不同使用者興趣偏好進可以更好的進行行個人化推薦。關鍵字的挖掘採用多種興趣詞挖掘相結合的方式,包括Keybert提取關鍵子串,並結合TextRank、positionRank、singlerank、TopicRank、MultipartiteRank等 句法分析多種方法,產生興趣詞候選。
挖掘出來的詞,相似度比較高,需要對同義詞進行識別,需要提升人工的效率,因此我們也透過聚類進行自動化語義相似識別。用於聚類的特徵有word2vec,bert embding等其他人工特徵。然後使用聚類方法,最後經過人工矯正我們離線產生了一批高品質的關鍵字。
對於不同粒度的標籤還是在物料層面的,我們需要把標籤和車建立起關聯,首先我們分別計算出標題文章的所屬標籤,然後識別出標題文章內的實體,得到若干標籤-實體偽標籤,最後根據大量的語料,共現機率高的標籤就會標記為該實體的標籤。透過以上三個任務,我們在獲得了豐富且大量的標籤。對車系、實體關聯上這些標籤,會大大豐富我們的汽車圖譜,建立了媒體和使用者的關注車標籤。
3.2.4人效提升:
伴隨著更大規模的訓練樣本,如何獲得更好的模型質量,如何解決標註成本高,標註週期長成為亟待解決的問題。首先我們可以使用半監督式學習,並利用海量未標註資料進行預訓練。再採用主動學習方式,最大化標註資料的價值,迭代選擇高資訊量樣本標註。最後可以利用遠端監督,發揮現有知識的價值,發覺任務之間的相關性。例如有了圖譜和標題後,可以用遠端監督的方法基於圖譜來建構NER訓練資料。
3.3 知識入庫
知識圖譜中的知識是透過RDF結構來進行表示的,其基本單元是事實。每個事實是一個三元組(S, P, O),在實際系統中,依照儲存方式的不同,知識圖譜的儲存可以分為基於RDF表結構的儲存和基於屬性圖結構的儲存。圖庫更多是採用屬性圖結構的存儲,常見的存儲系統有Neo4j、JanusGraph、OritentDB、InfoGrid等。
圖資料庫選擇
透過JanusGraph 與Neo4J、ArangoDB、OrientDB 這幾種主流圖資料庫的對比,我們最後選擇JanusGraph 作為專案的圖資料庫,之所以選擇JanusGraph,主要有以下原因:
- 基於Apache 2 授權協定開放原始碼,開放性佳。
- 支援使用 Hadoop 框架進行全域圖分析和批次圖處理。
- 支援很大的並發事務處理和圖操作處理。透過新增機器橫向擴展 JanusGraph 的事務 處理能力,可以在毫秒層級對應大圖的複雜查詢。
- 原生支援 Apache TinkerPop 所描述的目前流行的屬性圖資料模型。
- 原生支援圖遍歷語言 Gremlin。
- 下圖是主流圖資料庫比較:
#Janusgraph介紹
JanusGraph[5]是一個圖形資料庫引擎。其本身專注於緊湊圖序列化、豐富圖資料建模、高效的查詢執行。圖庫schema 構成可以用下面一個公式來表示:
janusgraph schema = vertex label edge label property keys
#這裡值得注意的是property key通常用於graph index。
為了更好的圖查詢效能janusgraph建立了索引,索引分為Graph Index,Vertex-centric Indexes。 Graph Index包含組合索引(Composite Index)和混合索引(Mixed Index).
#組合索引只限相等查找。 (組合索引不需要配置外部索引後端,透過主儲存後端支援(當然也可以配置hbase,Cassandra,Berkeley))
舉例:
<span style="color: rgb(89, 89, 89); margin: 0px; padding: 0px; background: none 0% 0% / auto repeat scroll padding-box border-box rgba(0, 0, 0, 0);">mgmt</span>.<span style="color: rgb(89, 89, 89); margin: 0px; padding: 0px; background: none 0% 0% / auto repeat scroll padding-box border-box rgba(0, 0, 0, 0);">buildIndex</span>(<span style="color: rgb(102, 153, 0); margin: 0px; padding: 0px; background: none 0% 0% / auto repeat scroll padding-box border-box rgba(0, 0, 0, 0);">'byNameAndAgeComposite'</span>, <span style="color: rgb(89, 89, 89); margin: 0px; padding: 0px; background: none 0% 0% / auto repeat scroll padding-box border-box rgba(0, 0, 0, 0);">Vertex</span>.<span style="color: rgb(215, 58, 73); margin: 0px; padding: 0px; background: none 0% 0% / auto repeat scroll padding-box border-box rgba(0, 0, 0, 0);">class</span>).<span style="color: rgb(89, 89, 89); margin: 0px; padding: 0px; background: none 0% 0% / auto repeat scroll padding-box border-box rgba(0, 0, 0, 0);">addKey</span>(<span style="color: rgb(89, 89, 89); margin: 0px; padding: 0px; background: none 0% 0% / auto repeat scroll padding-box border-box rgba(0, 0, 0, 0);">name</span>).<span style="color: rgb(89, 89, 89); margin: 0px; padding: 0px; background: none 0% 0% / auto repeat scroll padding-box border-box rgba(0, 0, 0, 0);">addKey</span>(<span style="color: rgb(89, 89, 89); margin: 0px; padding: 0px; background: none 0% 0% / auto repeat scroll padding-box border-box rgba(0, 0, 0, 0);">age</span>).<span style="color: rgb(89, 89, 89); margin: 0px; padding: 0px; background: none 0% 0% / auto repeat scroll padding-box border-box rgba(0, 0, 0, 0);">buildCompositeIndex</span>() <span style="color: rgb(89, 89, 89); margin: 0px; padding: 0px; background: none 0% 0% / auto repeat scroll padding-box border-box rgba(0, 0, 0, 0);">#构建一个组合索引“name</span><span style="color: rgb(215, 58, 73); margin: 0px; padding: 0px; background: none 0% 0% / auto repeat scroll padding-box border-box rgba(0, 0, 0, 0);">-</span><span style="color: rgb(89, 89, 89); margin: 0px; padding: 0px; background: none 0% 0% / auto repeat scroll padding-box border-box rgba(0, 0, 0, 0);">age”</span><br><span style="color: rgb(89, 89, 89); margin: 0px; padding: 0px; background: none 0% 0% / auto repeat scroll padding-box border-box rgba(0, 0, 0, 0);">g</span>.<span style="color: rgb(89, 89, 89); margin: 0px; padding: 0px; background: none 0% 0% / auto repeat scroll padding-box border-box rgba(0, 0, 0, 0);">V</span>().<span style="color: rgb(89, 89, 89); margin: 0px; padding: 0px; background: none 0% 0% / auto repeat scroll padding-box border-box rgba(0, 0, 0, 0);">has</span>(<span style="color: rgb(102, 153, 0); margin: 0px; padding: 0px; background: none 0% 0% / auto repeat scroll padding-box border-box rgba(0, 0, 0, 0);">'age'</span>, <span style="color: rgb(0, 92, 197); margin: 0px; padding: 0px; background: none 0% 0% / auto repeat scroll padding-box border-box rgba(0, 0, 0, 0);">30</span>).<span style="color: rgb(89, 89, 89); margin: 0px; padding: 0px; background: none 0% 0% / auto repeat scroll padding-box border-box rgba(0, 0, 0, 0);">has</span>(<span style="color: rgb(102, 153, 0); margin: 0px; padding: 0px; background: none 0% 0% / auto repeat scroll padding-box border-box rgba(0, 0, 0, 0);">'name'</span>, <span style="color: rgb(102, 153, 0); margin: 0px; padding: 0px; background: none 0% 0% / auto repeat scroll padding-box border-box rgba(0, 0, 0, 0);">'小明'</span>)<span style="color: rgb(89, 89, 89); margin: 0px; padding: 0px; background: none 0% 0% / auto repeat scroll padding-box border-box rgba(0, 0, 0, 0);">#查找</span> <span style="color: rgb(89, 89, 89); margin: 0px; padding: 0px; background: none 0% 0% / auto repeat scroll padding-box border-box rgba(0, 0, 0, 0);">名字为小明年龄30的节点</span>
混合索引需要ES作為後端索引以支援除相等以外的多條件查詢(也支援相等查詢,但相等查詢,組合索引更快)。根據是否需要分詞分為full-text search,和string search
JanusGraph資料儲存模型
了解Janusgraph儲存資料的方式,有助於我們更好的利用該圖庫。 JanusGraph 以鄰接清單格式儲存圖形,這表示圖形儲存為頂點及其鄰接清單的集合。
頂點的鄰接清單包含頂點的所有入射邊(和屬性)。
JanusGraph 將每個鄰接清單作為一行儲存在底層儲存後端。 (64 位元)頂點 ID(JanusGraph 唯一指派給每個頂點)是指向包含頂點鄰接清單的行的鍵。
每個邊和屬性都儲存為行中的一個單獨的儲存格,允許有效的插入和刪除。因此,特定儲存後端中每行允許的最大單元數也是JanusGraph 可以針對該後端支援的頂點的最大度數。
如果儲存後端支援key-order,則鄰接表將按頂點 id 排序,JanusGraph可以指派頂點 id,以便對圖進行有效分區。分配 id 使得經常共同訪問的頂點具有絕對差異小的 id。
3.4 圖譜查詢服務
Janusgraph進行圖搜尋用的是gremlin語言,我們提供了統一的圖譜查詢服務,外部使用不用關心gremlin語言的具體實現,採用通用的介面進行查詢。我們分為三個接口:條件搜尋接口,以節點為中心向外查詢,和節點間路徑查詢接口。以下是幾個gremlin實現的範例:
- 條件搜尋:查詢10萬左右,銷售量最高的車:
<span style="color: rgb(89, 89, 89); margin: 0px; padding: 0px; background: none 0% 0% / auto repeat scroll padding-box border-box rgba(0, 0, 0, 0);">g</span>.<span style="color: rgb(89, 89, 89); margin: 0px; padding: 0px; background: none 0% 0% / auto repeat scroll padding-box border-box rgba(0, 0, 0, 0);">V</span>().<span style="color: rgb(89, 89, 89); margin: 0px; padding: 0px; background: none 0% 0% / auto repeat scroll padding-box border-box rgba(0, 0, 0, 0);">has</span>(<span style="color: rgb(102, 153, 0); margin: 0px; padding: 0px; background: none 0% 0% / auto repeat scroll padding-box border-box rgba(0, 0, 0, 0);">'price'</span>,<span style="color: rgb(89, 89, 89); margin: 0px; padding: 0px; background: none 0% 0% / auto repeat scroll padding-box border-box rgba(0, 0, 0, 0);">gt</span>(<span style="color: rgb(0, 92, 197); margin: 0px; padding: 0px; background: none 0% 0% / auto repeat scroll padding-box border-box rgba(0, 0, 0, 0);">8</span>)).<span style="color: rgb(89, 89, 89); margin: 0px; padding: 0px; background: none 0% 0% / auto repeat scroll padding-box border-box rgba(0, 0, 0, 0);">has</span>(<span style="color: rgb(102, 153, 0); margin: 0px; padding: 0px; background: none 0% 0% / auto repeat scroll padding-box border-box rgba(0, 0, 0, 0);">'price'</span>,<span style="color: rgb(89, 89, 89); margin: 0px; padding: 0px; background: none 0% 0% / auto repeat scroll padding-box border-box rgba(0, 0, 0, 0);">lt</span>(<span style="color: rgb(0, 92, 197); margin: 0px; padding: 0px; background: none 0% 0% / auto repeat scroll padding-box border-box rgba(0, 0, 0, 0);">12</span>)).<span style="color: rgb(89, 89, 89); margin: 0px; padding: 0px; background: none 0% 0% / auto repeat scroll padding-box border-box rgba(0, 0, 0, 0);">order</span>().<span style="color: rgb(89, 89, 89); margin: 0px; padding: 0px; background: none 0% 0% / auto repeat scroll padding-box border-box rgba(0, 0, 0, 0);">by</span>(<span style="color: rgb(102, 153, 0); margin: 0px; padding: 0px; background: none 0% 0% / auto repeat scroll padding-box border-box rgba(0, 0, 0, 0);">'sales'</span>,<span style="color: rgb(89, 89, 89); margin: 0px; padding: 0px; background: none 0% 0% / auto repeat scroll padding-box border-box rgba(0, 0, 0, 0);">desc</span>).<span style="color: rgb(89, 89, 89); margin: 0px; padding: 0px; background: none 0% 0% / auto repeat scroll padding-box border-box rgba(0, 0, 0, 0);">valueMap</span>().<span style="color: rgb(89, 89, 89); margin: 0px; padding: 0px; background: none 0% 0% / auto repeat scroll padding-box border-box rgba(0, 0, 0, 0);">limit</span>(<span style="color: rgb(0, 92, 197); margin: 0px; padding: 0px; background: none 0% 0% / auto repeat scroll padding-box border-box rgba(0, 0, 0, 0);">1</span>)
輸出:
<span style="color: rgb(215, 58, 73); margin: 0px; padding: 0px; background: none 0% 0% / auto repeat scroll padding-box border-box rgba(0, 0, 0, 0);">==></span>{<span style="color: rgb(89, 89, 89); margin: 0px; padding: 0px; background: none 0% 0% / auto repeat scroll padding-box border-box rgba(0, 0, 0, 0);">name</span><span style="color: rgb(215, 58, 73); margin: 0px; padding: 0px; background: none 0% 0% / auto repeat scroll padding-box border-box rgba(0, 0, 0, 0);">=</span>[<span style="color: rgb(89, 89, 89); margin: 0px; padding: 0px; background: none 0% 0% / auto repeat scroll padding-box border-box rgba(0, 0, 0, 0);">xuanyi</span>], <span style="color: rgb(89, 89, 89); margin: 0px; padding: 0px; background: none 0% 0% / auto repeat scroll padding-box border-box rgba(0, 0, 0, 0);">price</span><span style="color: rgb(215, 58, 73); margin: 0px; padding: 0px; background: none 0% 0% / auto repeat scroll padding-box border-box rgba(0, 0, 0, 0);">=</span>[<span style="color: rgb(0, 92, 197); margin: 0px; padding: 0px; background: none 0% 0% / auto repeat scroll padding-box border-box rgba(0, 0, 0, 0);">10</span>], <span style="color: rgb(89, 89, 89); margin: 0px; padding: 0px; background: none 0% 0% / auto repeat scroll padding-box border-box rgba(0, 0, 0, 0);">sales</span><span style="color: rgb(215, 58, 73); margin: 0px; padding: 0px; background: none 0% 0% / auto repeat scroll padding-box border-box rgba(0, 0, 0, 0);">=</span>[<span style="color: rgb(0, 92, 197); margin: 0px; padding: 0px; background: none 0% 0% / auto repeat scroll padding-box border-box rgba(0, 0, 0, 0);">45767</span>]}
軒逸銷售量最高,為45767
- 以節點為中心向外查詢:查詢以小明為中心, 2度的節點
<span style="color: rgb(89, 89, 89); margin: 0px; padding: 0px; background: none 0% 0% / auto repeat scroll padding-box border-box rgba(0, 0, 0, 0);">g</span>.<span style="color: rgb(89, 89, 89); margin: 0px; padding: 0px; background: none 0% 0% / auto repeat scroll padding-box border-box rgba(0, 0, 0, 0);">V</span>(<span style="color: rgb(89, 89, 89); margin: 0px; padding: 0px; background: none 0% 0% / auto repeat scroll padding-box border-box rgba(0, 0, 0, 0);">xiaoming</span>).<span style="color: rgb(89, 89, 89); margin: 0px; padding: 0px; background: none 0% 0% / auto repeat scroll padding-box border-box rgba(0, 0, 0, 0);">repeat</span>(<span style="color: rgb(89, 89, 89); margin: 0px; padding: 0px; background: none 0% 0% / auto repeat scroll padding-box border-box rgba(0, 0, 0, 0);">out</span>()).<span style="color: rgb(89, 89, 89); margin: 0px; padding: 0px; background: none 0% 0% / auto repeat scroll padding-box border-box rgba(0, 0, 0, 0);">times</span>(<span style="color: rgb(0, 92, 197); margin: 0px; padding: 0px; background: none 0% 0% / auto repeat scroll padding-box border-box rgba(0, 0, 0, 0);">2</span>).<span style="color: rgb(89, 89, 89); margin: 0px; padding: 0px; background: none 0% 0% / auto repeat scroll padding-box border-box rgba(0, 0, 0, 0);">valueMap</span>()
- #節點間路徑查詢:薦給小明推薦兩篇文章,這兩篇文章分別介紹的是卡羅拉和軒逸,查詢小明和這兩篇文章的路徑:
<span style="color: rgb(89, 89, 89); margin: 0px; padding: 0px; background: none 0% 0% / auto repeat scroll padding-box border-box rgba(0, 0, 0, 0);">g</span>.<span style="color: rgb(89, 89, 89); margin: 0px; padding: 0px; background: none 0% 0% / auto repeat scroll padding-box border-box rgba(0, 0, 0, 0);">V</span>(<span style="color: rgb(89, 89, 89); margin: 0px; padding: 0px; background: none 0% 0% / auto repeat scroll padding-box border-box rgba(0, 0, 0, 0);">xiaoming</span>).<span style="color: rgb(89, 89, 89); margin: 0px; padding: 0px; background: none 0% 0% / auto repeat scroll padding-box border-box rgba(0, 0, 0, 0);">repeat</span>(<span style="color: rgb(89, 89, 89); margin: 0px; padding: 0px; background: none 0% 0% / auto repeat scroll padding-box border-box rgba(0, 0, 0, 0);">out</span>().<span style="color: rgb(89, 89, 89); margin: 0px; padding: 0px; background: none 0% 0% / auto repeat scroll padding-box border-box rgba(0, 0, 0, 0);">simplePath</span>()).<span style="color: rgb(89, 89, 89); margin: 0px; padding: 0px; background: none 0% 0% / auto repeat scroll padding-box border-box rgba(0, 0, 0, 0);">until</span>(<span style="color: rgb(89, 89, 89); margin: 0px; padding: 0px; background: none 0% 0% / auto repeat scroll padding-box border-box rgba(0, 0, 0, 0);">or</span>(<span style="color: rgb(89, 89, 89); margin: 0px; padding: 0px; background: none 0% 0% / auto repeat scroll padding-box border-box rgba(0, 0, 0, 0);">has</span>(<span style="color: rgb(102, 153, 0); margin: 0px; padding: 0px; background: none 0% 0% / auto repeat scroll padding-box border-box rgba(0, 0, 0, 0);">"car"</span>,<span style="color: rgb(102, 153, 0); margin: 0px; padding: 0px; background: none 0% 0% / auto repeat scroll padding-box border-box rgba(0, 0, 0, 0);">'name'</span>, <span style="color: rgb(102, 153, 0); margin: 0px; padding: 0px; background: none 0% 0% / auto repeat scroll padding-box border-box rgba(0, 0, 0, 0);">'kaluola'</span>),<span style="color: rgb(89, 89, 89); margin: 0px; padding: 0px; background: none 0% 0% / auto repeat scroll padding-box border-box rgba(0, 0, 0, 0);">has</span>(<span style="color: rgb(102, 153, 0); margin: 0px; padding: 0px; background: none 0% 0% / auto repeat scroll padding-box border-box rgba(0, 0, 0, 0);">"car"</span>, <span style="color: rgb(102, 153, 0); margin: 0px; padding: 0px; background: none 0% 0% / auto repeat scroll padding-box border-box rgba(0, 0, 0, 0);">'name'</span>,<span style="color: rgb(102, 153, 0); margin: 0px; padding: 0px; background: none 0% 0% / auto repeat scroll padding-box border-box rgba(0, 0, 0, 0);">'xuanyi'</span>))).<span style="color: rgb(89, 89, 89); margin: 0px; padding: 0px; background: none 0% 0% / auto repeat scroll padding-box border-box rgba(0, 0, 0, 0);">path</span>().<span style="color: rgb(89, 89, 89); margin: 0px; padding: 0px; background: none 0% 0% / auto repeat scroll padding-box border-box rgba(0, 0, 0, 0);">by</span>(<span style="color: rgb(102, 153, 0); margin: 0px; padding: 0px; background: none 0% 0% / auto repeat scroll padding-box border-box rgba(0, 0, 0, 0);">"name"</span>)
輸出
<span style="color: rgb(215, 58, 73); margin: 0px; padding: 0px; background: none 0% 0% / auto repeat scroll padding-box border-box rgba(0, 0, 0, 0);">==></span><span style="color: rgb(89, 89, 89); margin: 0px; padding: 0px; background: none 0% 0% / auto repeat scroll padding-box border-box rgba(0, 0, 0, 0);">path</span>[<span style="color: rgb(89, 89, 89); margin: 0px; padding: 0px; background: none 0% 0% / auto repeat scroll padding-box border-box rgba(0, 0, 0, 0);">xiaoming</span>, <span style="color: rgb(89, 89, 89); margin: 0px; padding: 0px; background: none 0% 0% / auto repeat scroll padding-box border-box rgba(0, 0, 0, 0);">around</span> <span style="color: rgb(0, 92, 197); margin: 0px; padding: 0px; background: none 0% 0% / auto repeat scroll padding-box border-box rgba(0, 0, 0, 0);">10</span><span style="color: rgb(89, 89, 89); margin: 0px; padding: 0px; background: none 0% 0% / auto repeat scroll padding-box border-box rgba(0, 0, 0, 0);">w</span>, <span style="color: rgb(89, 89, 89); margin: 0px; padding: 0px; background: none 0% 0% / auto repeat scroll padding-box border-box rgba(0, 0, 0, 0);">kaluola</span>]<br><span style="color: rgb(215, 58, 73); margin: 0px; padding: 0px; background: none 0% 0% / auto repeat scroll padding-box border-box rgba(0, 0, 0, 0);">==></span><span style="color: rgb(89, 89, 89); margin: 0px; padding: 0px; background: none 0% 0% / auto repeat scroll padding-box border-box rgba(0, 0, 0, 0);">path</span>[<span style="color: rgb(89, 89, 89); margin: 0px; padding: 0px; background: none 0% 0% / auto repeat scroll padding-box border-box rgba(0, 0, 0, 0);">xiaoming</span>, <span style="color: rgb(89, 89, 89); margin: 0px; padding: 0px; background: none 0% 0% / auto repeat scroll padding-box border-box rgba(0, 0, 0, 0);">around</span> <span style="color: rgb(0, 92, 197); margin: 0px; padding: 0px; background: none 0% 0% / auto repeat scroll padding-box border-box rgba(0, 0, 0, 0);">10</span><span style="color: rgb(89, 89, 89); margin: 0px; padding: 0px; background: none 0% 0% / auto repeat scroll padding-box border-box rgba(0, 0, 0, 0);">w</span>, <span style="color: rgb(89, 89, 89); margin: 0px; padding: 0px; background: none 0% 0% / auto repeat scroll padding-box border-box rgba(0, 0, 0, 0);">xuanyi</span>]
發現小明和這兩篇文章之間有個節點「10萬左右」
三、知識圖譜在推薦的應用
知識圖譜中存在大量的非歐式數據,基於KG的推薦應用有效利用非歐式數據提升推薦系統準確度,進而讓推薦系統能達到傳統系統所無法達到的效果。基於KG的推薦可以分成以三類,基於KG表徵技術(KGE)、基於路徑的方法、圖神經網路。 本章將從KG在推薦系統中冷開機、理由、排序三方面的應用和論文來介紹。
3.1 知識圖譜在推薦冷啟動的應用
知識圖譜能夠從user-item交互中建模KG中隱藏的高階關係,很好地解決了因用戶呼叫有限數量的行為而導致的資料稀疏性,進而可以應用在解決冷啟動問題。這一問題業界也有相關研究。
Sang 等[6]提出了一種雙通道神經交互作用方法,稱為知識圖增強的殘差遞歸神經協同過濾(KGNCF-RRN),該方法利用KG上下文的長期關係依賴性和使用者項交互進行推薦。
(1)對於KG上下文互動通道,提出了殘差遞歸網路(RRN)來建構基於上下文的路徑嵌入,將殘差學習融入傳統的遞歸神經網路(RNN)中,以有效地編碼KG的長期關係依賴。然後將自關注網路應用於路徑嵌入,以捕捉各種使用者互動行為的多義。
(2)對於使用者項目互動通道,使用者和項目嵌入被輸入到新設計的二維互動圖中。
(3)最後,在雙通道神經交互矩陣之上,使用卷積神經網路來學習使用者和項目之間的複雜相關性。此方法能捕捉豐富的語意訊息,也能捕捉使用者與專案之間複雜的隱含關係,用於推薦。
Du Y等[7]提出了一個新的基於元學習框架的冷啟動問題解決方案MetaKG,包括collaborative-aware meta learner和knowledge-aware meta learner,捕捉使用者的偏好和實體冷啟動知識。 collaborative-aware meta learner學習任務旨在聚合每個使用者的偏好知識表示。相反,knowledge-aware meta learner學習任務要在全域泛化不同的使用者偏好知識表示。在兩個learner的指導下,MetaKG可以有效地捕捉高階的協作關係關係和語意表示,可以輕鬆適應冷啟動場景。此外,作者還設計了一種自適應任務,可以自適應地選擇KG資訊進行學習,以防止模型被噪音資訊幹擾。 MetaKG架構如下圖所示。
3.2 知識圖譜在推薦理由產生的應用
推薦理由能提升推薦系統的可解釋性,讓使用者理解產生推薦結果的計算過程,同時也能解釋item受歡迎的原因。使用者透過推薦理由了解推薦結果的產生原理,可以增強使用者對系統推薦結果的信心,並且在推薦失誤的情況下對錯誤結果更加寬容。
最早可解釋推薦是以模板為主,模板的好處是保證了可讀性和高準確率。但是需要人工整理模板,泛華性不強,給人一種重複的感覺。後來發展不需要預設的free-form形式,並且加以知識圖譜,以其中的一條路徑作為解釋,配合標註還有一些結合KG路徑的生成式的方法,模型中選擇的每個點或邊都是一個推理過程,可以向使用者展示。最近Chen Z [8]等人提出一種增量多任務學習框架ECR,可以實現推薦預測、解釋生成和用戶回饋整合之間的緊密協作。它由兩大部分組成。第一部分,增量交叉知識建模,學習推薦任務和解釋任務中轉移的交叉知識,並說明如何使用交叉知識透過使用增量學習進行更新。第二部分,增量多任務預測,闡述如何基於交叉知識生成解釋,以及如何根據交叉知識和使用者回饋預測推薦分數。
3.3 知識圖譜在推薦排序的應用
KG可以透過給item用不同的屬性進行鏈接,建立user- item之間interaction,將uesr-item graph和KG結合成一張大圖,可以捕捉item間的高階連結。傳統的推薦方法是將問題建模為一個監督學習任務,這種方式會忽略item之間的內在聯繫(例如凱美瑞和雅閣的競品關係),並且無法從user行為中獲取協同信號。以下介紹兩篇KG應用在推薦排序的論文。
Wang[9]等人設計了KGAT演算法,首先利用GNN迭代對embedding進行傳播、更新,從而能夠在快速捕捉高階聯繫;其次,在aggregation時使用attention機制,傳播過程中學習到每個neighbor的weight,反應高階連結的重要程度;最後,透過N階傳播更新得到user-item的N個隱式表示,不同layer表示不同階數的連接訊息。 KGAT可以捕捉更豐富、不特定的高階連結。
Zhang[20]等人提出RippleNet模型,其關鍵思想是興趣傳播:RippleNet將使用者的歷史興趣作為KG中的種子集合(seed set),然後沿著KG的連結向外擴展用戶興趣,形成用戶在KG上的興趣分佈。 RippleNet最大的優勢在於它可以自動地挖掘從使用者歷史點擊過的物品到候選物品的可能路徑,不需要任何人工設計元路徑或元圖。
RippleNet將使用者U和專案V作為輸入,並輸出使用者U點選專案V的預測機率。對於使用者U,將其歷史興趣V_{u}作為種子,在圖中可以看到最初的起點是兩個,之後不斷向周圍擴散。給定itemV和用戶U的1跳ripple集合V_{u_{}^{1}}中的每個三元組left( h_{i},r_{i},t_{i}right),透過比較V與三元組中的節點h_{i}和關係r_{i}分配相關機率。
在得到相關機率後,將V_{u_{}^{1}}中三元組的尾部乘以對應的相關機率進行加權求和,得到使用者U的歷史興趣關於V的一階響應,使用者興趣由V_{u}轉移到o_{u}^{1},可以計算得到o_{u}^{2}、o_{u}^{3} ...o_{u}^{n},進而計算得到U關於item V的特徵可以被計算為融合他的所有階數響應。
四、總結
綜上,我們主要圍繞著推薦,介紹了圖譜建立詳細流程,對其中的困難和挑戰做出了分析。同時也綜述了許多重要的工作,以及給了具體的解決方案,思路以及建議。最後介紹了包含知識圖譜的應用,特別在推薦領域中冷起、可解釋性、召回排序介紹了知識圖譜的作用與使用。
引用:
[1] Kim S,Oh S G. Extracting and Applying Evaluation Criteria for Ontology Quality Assessment[J]. Library Hi Tech,2019.
[2]Protege: #https://www.php.cn/link/9d405c24be657bbf7a5244815a908922
[3] Ding S , Shang J , Wang S , et al. ERNIE-DOC: The Retrospective Long-Document Modeling Transformer[J]. 2020.[4]DocBert,[1] Adhikari A , Ram A , Tang R ,et al. DocBERT: BERT for Document Classification[J]. 2019.[5]JanusGraph,#https://www.php.cn /link/fc0de4e0396fff257ea362983c2dda5a
[6] Sang L, Xu M, Qian S, et al. Knowledge graph enhanced neural collaborative filtering with residual recurrenting.com , 454: 417-429.[7] Du Y , Zhu X , Chen L , et al. MetaKG: Meta-learning on Knowledge Graph for Cold-start Recommendation[J]. arXiv e-prints, 2022.[8] Chen Z , Wang X , Xie X , et al. Towards Explainable Conversational Recommendation[C]// Twenty-Ninth International Joint Conference on Artificial Intelligence and Seventeenth Pacific Rim International Conference int Conference on Artificial Intelligence and Seventeenth Pacific Rim International Conference int {IJCAI-PRICAI-20. 2020.[9] Wang X , He X , Cao Y , et al. KGAT: 諾##[10]Wang H , Zhang F , Wang J , et al. RippleNet: Propagating User Preferences on the Knowledge Graph for Recommender Systems[J]. ACM, 2018.以上是面向推薦的汽車知識圖譜構建的詳細內容。更多資訊請關注PHP中文網其他相關文章!
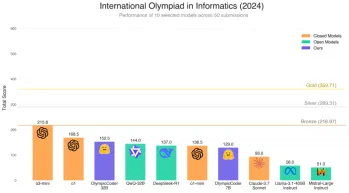
擁抱Face的OlympicCoder-7B:強大的開源代碼推理模型 開發以代碼為中心的語言模型的競賽正在加劇,擁抱面孔與強大的競爭者一起參加了比賽:OlympicCoder-7B,一種產品
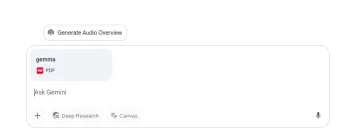
你們當中有多少人希望AI可以做更多的事情,而不僅僅是回答問題?我知道我有,最近,我對它的變化感到驚訝。 AI聊天機器人不僅要聊天,還關心創建,研究
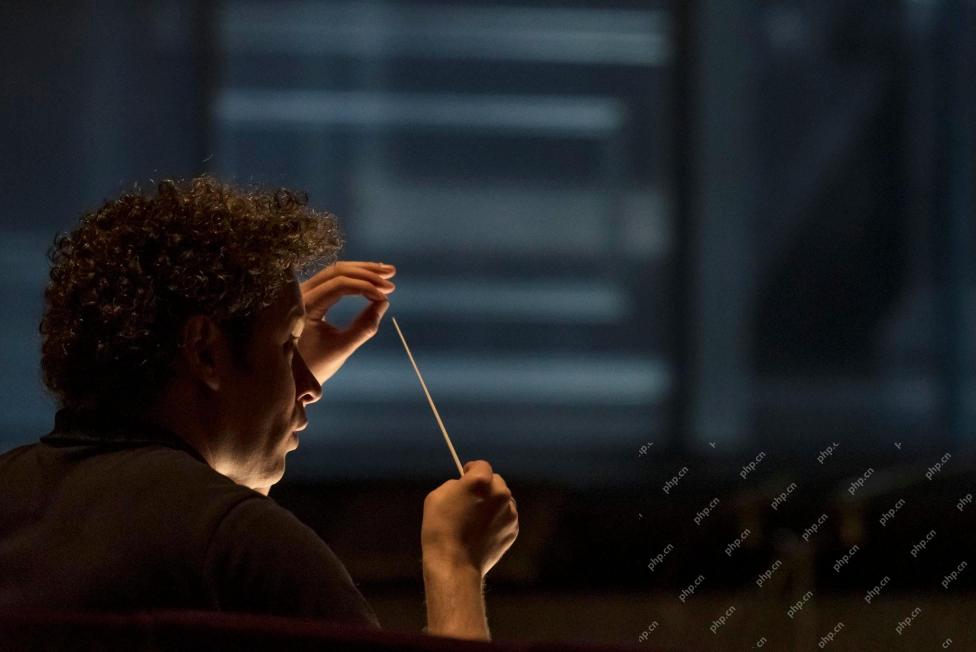
隨著智能AI開始融入企業軟件平台和應用程序的各個層面(我們必須強調的是,既有強大的核心工具,也有一些不太可靠的模擬工具),我們需要一套新的基礎設施能力來管理這些智能體。 總部位於德國柏林的流程編排公司Camunda認為,它可以幫助智能AI發揮其應有的作用,並與新的數字工作場所中的準確業務目標和規則保持一致。該公司目前提供智能編排功能,旨在幫助組織建模、部署和管理AI智能體。 從實際的軟件工程角度來看,這意味著什麼? 確定性與非確定性流程的融合 該公司表示,關鍵在於允許用戶(通常是數據科學家、軟件
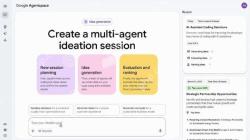
參加Google Cloud Next '25,我渴望看到Google如何區分其AI產品。 有關代理空間(此處討論)和客戶體驗套件(此處討論)的最新公告很有希望,強調了商業價值
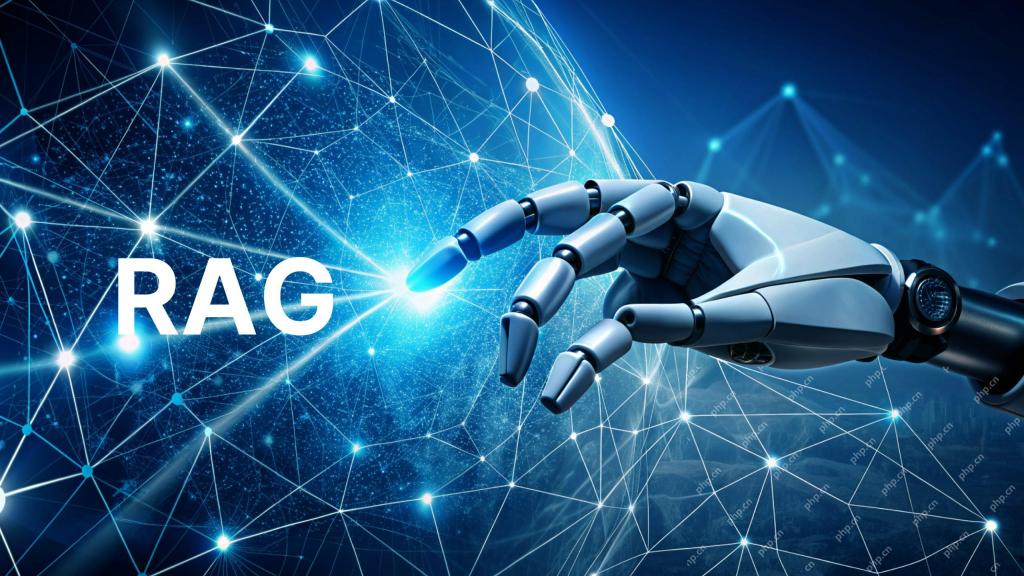
為您的檢索增強發電(RAG)系統選擇最佳的多語言嵌入模型 在當今的相互聯繫的世界中,建立有效的多語言AI系統至關重要。 強大的多語言嵌入模型對於RE至關重要
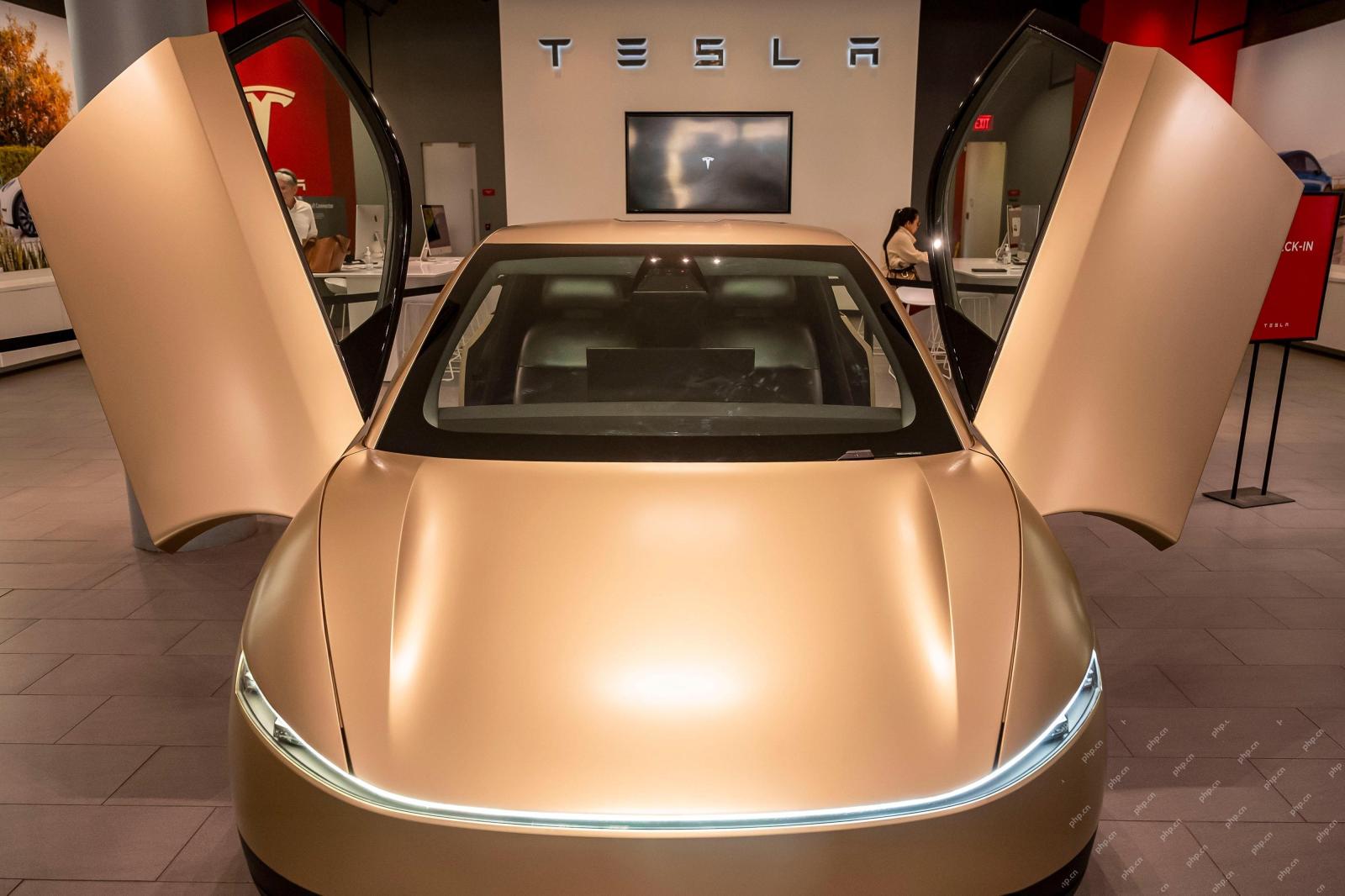
特斯拉的Austin Robotaxi發射:仔細觀察Musk的主張 埃隆·馬斯克(Elon Musk)最近宣布,特斯拉即將在德克薩斯州奧斯汀推出的Robotaxi發射,最初出於安全原因部署了一支小型10-20輛汽車,並有快速擴張的計劃。 h
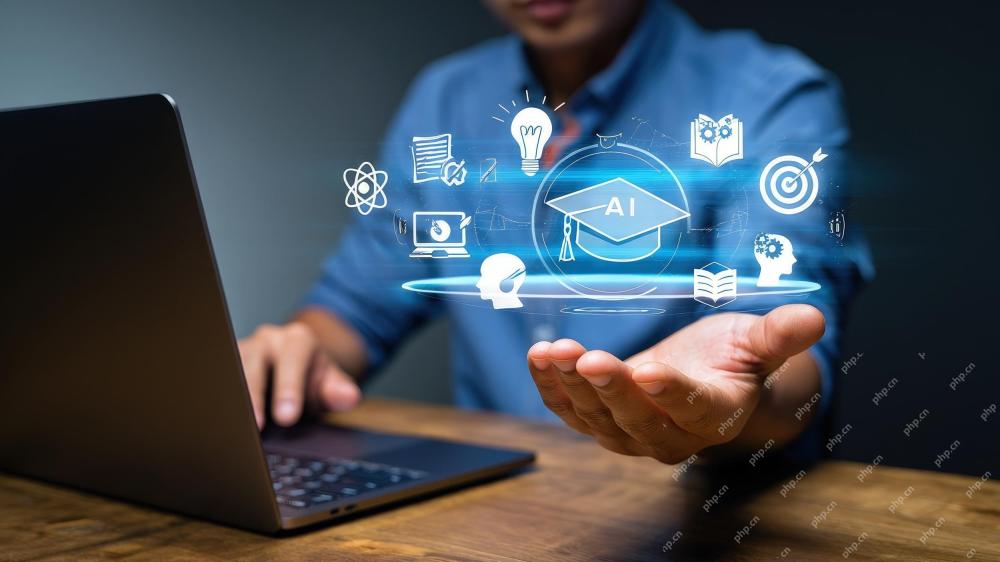
人工智能的應用方式可能出乎意料。最初,我們很多人可能認為它主要用於代勞創意和技術任務,例如編寫代碼和創作內容。 然而,哈佛商業評論最近報導的一項調查表明情況並非如此。大多數用戶尋求人工智能的並非是代勞工作,而是支持、組織,甚至是友誼! 報告稱,人工智能應用案例的首位是治療和陪伴。這表明其全天候可用性以及提供匿名、誠實建議和反饋的能力非常有價值。 另一方面,營銷任務(例如撰寫博客、創建社交媒體帖子或廣告文案)在流行用途列表中的排名要低得多。 這是為什麼呢?讓我們看看研究結果及其對我們人類如何繼續將


熱AI工具

Undresser.AI Undress
人工智慧驅動的應用程序,用於創建逼真的裸體照片

AI Clothes Remover
用於從照片中去除衣服的線上人工智慧工具。

Undress AI Tool
免費脫衣圖片

Clothoff.io
AI脫衣器

Video Face Swap
使用我們完全免費的人工智慧換臉工具,輕鬆在任何影片中換臉!

熱門文章

熱工具

MantisBT
Mantis是一個易於部署的基於Web的缺陷追蹤工具,用於幫助產品缺陷追蹤。它需要PHP、MySQL和一個Web伺服器。請查看我們的演示和託管服務。
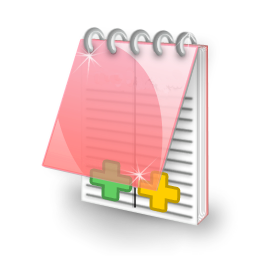
EditPlus 中文破解版
體積小,語法高亮,不支援程式碼提示功能
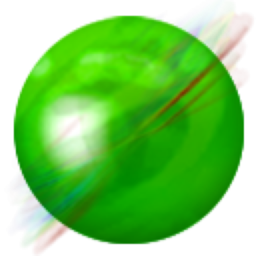
ZendStudio 13.5.1 Mac
強大的PHP整合開發環境

Safe Exam Browser
Safe Exam Browser是一個安全的瀏覽器環境,安全地進行線上考試。該軟體將任何電腦變成一個安全的工作站。它控制對任何實用工具的訪問,並防止學生使用未經授權的資源。
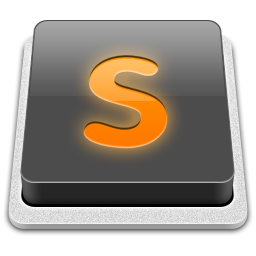
SublimeText3 Mac版
神級程式碼編輯軟體(SublimeText3)