Python是一门弱类型语言,很多从C/C++转过来的朋友起初不是很适应。比如,在声明一个函数时,不能指定参数的类型。用C做类比,那就是所有参数都是void*类型!void类型强制转换在C++中被广泛地认为是个坏习惯,不到万不得已是不会使用的。
Python自然没有类型强制转换一说了,因为它是动态语言。首先,所有对象都从Object继承而来,其次,它有强大的内省,如果调用某个不存在的方法会有异常抛出。大多数情况,我们都不需要做参数类型栓查,除了一些特殊情况。例如,某个函数接受一个str类型,结果在实际调用时传入的是unicode,测试过程中又没有代码覆盖到,这样问题就比较严重了。解决方法也很简单,借助Python的内省,很容易就能判断出参数的类型。但是每个地方都写检查代码会很累赘,何况它带来的实际价值并不高。一个好的解决方法是使用装饰器。
''' >>> NONE, MEDIUM, STRONG = 0, 1, 2 >>> >>> @accepts(int, int, int) ... def average(x, y, z): ... return (x + y + z) / 2 ... >>> average(5.5, 10, 15.0) TypeWarning: 'average' method accepts (int, int, int), but was given (float, int, float) 15.25 ''' def accepts(*types, **kw): """ Function decorator. Checks that inputs given to decorated function are of the expected type. Parameters: types -- The expected types of the inputs to the decorated function. Must specify type for each parameter. kw -- Optional specification of 'debug' level (this is the only valid keyword argument, no other should be given). debug = ( 0 | 1 | 2 ) """ if not kw: # default level: MEDIUM debug = 1 else: debug = kw['debug'] try: def decorator(f): def newf(*args): if debug == 0: return f(*args) assert len(args) == len(types) argtypes = tuple(map(type, args)) if argtypes != types: msg = info(f.__name__, types, argtypes, 0) if debug == 1: print >> sys.stderr, 'TypeWarning: ', msg elif debug == 2: raise TypeError, msg return f(*args) newf.__name__ = f.__name__ return newf return decorator except KeyError, key: raise KeyError, key + "is not a valid keyword argument" except TypeError, msg: raise TypeError, msg def info(fname, expected, actual, flag): """ Convenience function returns nicely formatted error/warning msg. """ format = lambda types: ', '.join([str(t).split("'")[1] for t in types]) expected, actual = format(expected), format(actual) msg = "'%s' method " % fname \ + ("accepts", "returns")[flag] + " (%s), but " % expected\ + ("was given", "result is")[flag] + " (%s)" % actual return msg
本质上讲,这也是一种运行时检查,但效果已经不错了。
更多有趣的装饰器的使用,可以参考这篇文章http://wiki.python.org/moin/PythonDecoratorLibrary
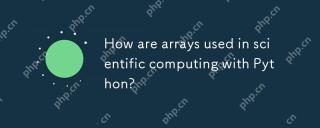
ArraysinPython,especiallyviaNumPy,arecrucialinscientificcomputingfortheirefficiencyandversatility.1)Theyareusedfornumericaloperations,dataanalysis,andmachinelearning.2)NumPy'simplementationinCensuresfasteroperationsthanPythonlists.3)Arraysenablequick
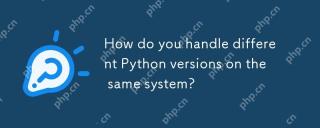
You can manage different Python versions by using pyenv, venv and Anaconda. 1) Use pyenv to manage multiple Python versions: install pyenv, set global and local versions. 2) Use venv to create a virtual environment to isolate project dependencies. 3) Use Anaconda to manage Python versions in your data science project. 4) Keep the system Python for system-level tasks. Through these tools and strategies, you can effectively manage different versions of Python to ensure the smooth running of the project.
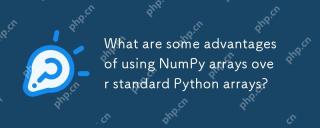
NumPyarrayshaveseveraladvantagesoverstandardPythonarrays:1)TheyaremuchfasterduetoC-basedimplementation,2)Theyaremorememory-efficient,especiallywithlargedatasets,and3)Theyofferoptimized,vectorizedfunctionsformathematicalandstatisticaloperations,making
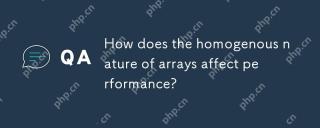
The impact of homogeneity of arrays on performance is dual: 1) Homogeneity allows the compiler to optimize memory access and improve performance; 2) but limits type diversity, which may lead to inefficiency. In short, choosing the right data structure is crucial.
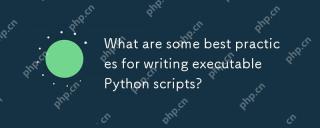
TocraftexecutablePythonscripts,followthesebestpractices:1)Addashebangline(#!/usr/bin/envpython3)tomakethescriptexecutable.2)Setpermissionswithchmod xyour_script.py.3)Organizewithacleardocstringanduseifname=="__main__":formainfunctionality.4
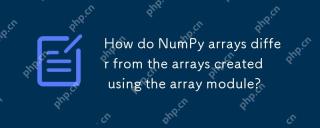
NumPyarraysarebetterfornumericaloperationsandmulti-dimensionaldata,whilethearraymoduleissuitableforbasic,memory-efficientarrays.1)NumPyexcelsinperformanceandfunctionalityforlargedatasetsandcomplexoperations.2)Thearraymoduleismorememory-efficientandfa
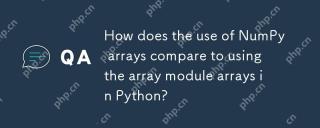
NumPyarraysarebetterforheavynumericalcomputing,whilethearraymoduleismoresuitableformemory-constrainedprojectswithsimpledatatypes.1)NumPyarraysofferversatilityandperformanceforlargedatasetsandcomplexoperations.2)Thearraymoduleislightweightandmemory-ef
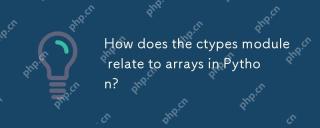
ctypesallowscreatingandmanipulatingC-stylearraysinPython.1)UsectypestointerfacewithClibrariesforperformance.2)CreateC-stylearraysfornumericalcomputations.3)PassarraystoCfunctionsforefficientoperations.However,becautiousofmemorymanagement,performanceo


Hot AI Tools

Undresser.AI Undress
AI-powered app for creating realistic nude photos

AI Clothes Remover
Online AI tool for removing clothes from photos.

Undress AI Tool
Undress images for free

Clothoff.io
AI clothes remover

Video Face Swap
Swap faces in any video effortlessly with our completely free AI face swap tool!

Hot Article

Hot Tools

SecLists
SecLists is the ultimate security tester's companion. It is a collection of various types of lists that are frequently used during security assessments, all in one place. SecLists helps make security testing more efficient and productive by conveniently providing all the lists a security tester might need. List types include usernames, passwords, URLs, fuzzing payloads, sensitive data patterns, web shells, and more. The tester can simply pull this repository onto a new test machine and he will have access to every type of list he needs.
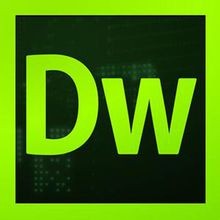
Dreamweaver CS6
Visual web development tools

SAP NetWeaver Server Adapter for Eclipse
Integrate Eclipse with SAP NetWeaver application server.

SublimeText3 Linux new version
SublimeText3 Linux latest version
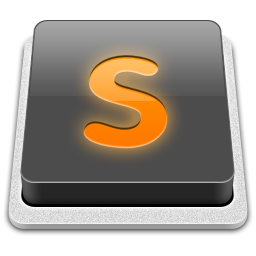
SublimeText3 Mac version
God-level code editing software (SublimeText3)
