Use examples to introduce how to import the pandas library
Pandas library import practical guide
Introduction:
In the fields of data analysis and machine learning, the pandas library is a very powerful tool. It provides rich functionality for data reading, processing and analysis. This article will provide you with a practical guide to importing the pandas library and present some specific code examples to help readers better understand and use the pandas library.
1. Install the pandas library
To use the pandas library, you first need to install it. There are many ways to install the pandas library, the most common way is to use the pip command. Enter the following command on the command line to install the pandas library:
pip install pandas
After the installation is complete, you can start using the pandas library.
2. Import the pandas library
Before using the pandas library, you first need to import it into the Python environment. The usual approach is to import the pandas library using the import statement, as shown below:
import pandas as pd
In this example, we import the pandas library and reference it with the alias "pd". This is a common practice because "pd" is more concise than "pandas" and easier to use in code.
3. Reading data
One of the most commonly used functions of the pandas library is to read various data files. We can use the read_xxx() function provided by the pandas library to read different types of files, such as CSV files, Excel files, SQL databases, etc.
-
Read CSV file
The following example shows how to read a CSV file and store the data in a DataFrame object.data = pd.read_csv("data.csv")
In this example, we read a CSV file named "data.csv" into a DataFrame object named "data".
-
Reading Excel files
If you want to read Excel files, you can use the read_excel() function of the pandas library. The following example shows how to read an Excel file.data = pd.read_excel("data.xlsx")
In this example, we read an Excel file named "data.xlsx" into a DataFrame object named "data".
-
Read SQL database
If you want to read data in a SQL database, you can use the read_sql() function of the pandas library. The following example shows how to connect to a SQLite database named "mydb" and read a table named "customers" in it.import sqlite3 con = sqlite3.connect("mydb.db") data = pd.read_sql("SELECT * FROM customers", con)
In this example, we first use the sqlite3 library to connect to the SQLite database and assign the connection object to the variable "con". Then, we executed a SELECT query using the read_sql() function of the pandas library and stored the query results in a DataFrame object "data".
4. Data processing and analysis
The pandas library provides a wealth of functions to perform various processing operations on data, such as filtering, sorting, grouping, calculation, etc.
-
Data filtering
To filter the data in the DataFrame, you can use conditional statements. The following example shows how to filter out data for people older than 30 years old.selected_data = data[data['age'] > 30]
In this example, we use the conditional statement "data['age'] > 30" to filter the data in the DataFrame object "data" and store the data that meets the conditions in a new DataFrame object "selected_data".
-
Data sorting
To sort the data in the DataFrame, you can use the sort_values() function. The following example shows how to sort data from smallest to largest age.sorted_data = data.sort_values('age')
In this example, we use the sort_values() function to sort the data in the DataFrame object "data" according to the column name "age", and store the sorting result in a new DataFrame object "sorted_data" "middle.
-
Data Grouping
To group the data in the DataFrame, you can use the groupby() function. The following example shows how to group data by gender and perform statistical calculations.grouped_data = data.groupby('gender').mean()
In this example, we use the groupby() function to group the data in the DataFrame object "data" according to the column name "gender", and use the mean() function to calculate the average of each grouping.
-
Data calculation
The pandas library supports a variety of calculation operations, such as addition, subtraction, multiplication, division, etc. The following example shows how to calculate a new column "total_sales" whose value is equal to the product of the "quantity" column and the "price" column.data['total_sales'] = data['quantity'] * data['price']
In this example, we use the ordinary operator "*" to multiply the elements of the "quantity" column and the "price" column one by one, and assign the operation result to a new column "total_sales".
Conclusion:
This article provides a practical guide to importing the pandas library and presents some specific code examples. By reading this article and practicing the sample code, readers can better understand and use the pandas library to perform data analysis and machine learning tasks more efficiently. Hope this article is helpful to readers!
The above is the detailed content of Use examples to introduce how to import the pandas library. For more information, please follow other related articles on the PHP Chinese website!
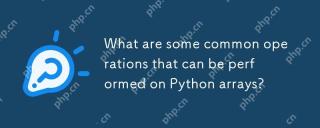
Pythonarrayssupportvariousoperations:1)Slicingextractssubsets,2)Appending/Extendingaddselements,3)Insertingplaceselementsatspecificpositions,4)Removingdeleteselements,5)Sorting/Reversingchangesorder,and6)Listcomprehensionscreatenewlistsbasedonexistin
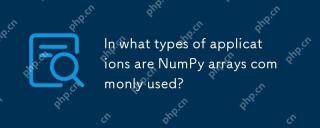
NumPyarraysareessentialforapplicationsrequiringefficientnumericalcomputationsanddatamanipulation.Theyarecrucialindatascience,machinelearning,physics,engineering,andfinanceduetotheirabilitytohandlelarge-scaledataefficiently.Forexample,infinancialanaly
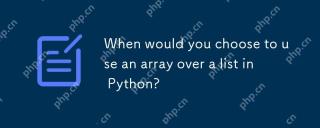
Useanarray.arrayoveralistinPythonwhendealingwithhomogeneousdata,performance-criticalcode,orinterfacingwithCcode.1)HomogeneousData:Arrayssavememorywithtypedelements.2)Performance-CriticalCode:Arraysofferbetterperformancefornumericaloperations.3)Interf
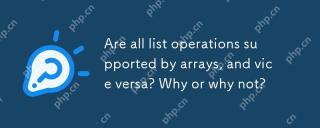
No,notalllistoperationsaresupportedbyarrays,andviceversa.1)Arraysdonotsupportdynamicoperationslikeappendorinsertwithoutresizing,whichimpactsperformance.2)Listsdonotguaranteeconstanttimecomplexityfordirectaccesslikearraysdo.
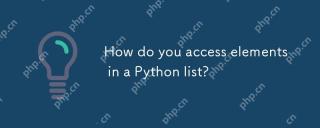
ToaccesselementsinaPythonlist,useindexing,negativeindexing,slicing,oriteration.1)Indexingstartsat0.2)Negativeindexingaccessesfromtheend.3)Slicingextractsportions.4)Iterationusesforloopsorenumerate.AlwayschecklistlengthtoavoidIndexError.
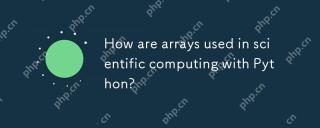
ArraysinPython,especiallyviaNumPy,arecrucialinscientificcomputingfortheirefficiencyandversatility.1)Theyareusedfornumericaloperations,dataanalysis,andmachinelearning.2)NumPy'simplementationinCensuresfasteroperationsthanPythonlists.3)Arraysenablequick
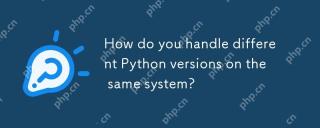
You can manage different Python versions by using pyenv, venv and Anaconda. 1) Use pyenv to manage multiple Python versions: install pyenv, set global and local versions. 2) Use venv to create a virtual environment to isolate project dependencies. 3) Use Anaconda to manage Python versions in your data science project. 4) Keep the system Python for system-level tasks. Through these tools and strategies, you can effectively manage different versions of Python to ensure the smooth running of the project.
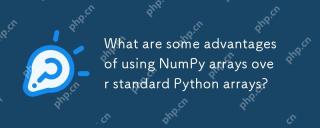
NumPyarrayshaveseveraladvantagesoverstandardPythonarrays:1)TheyaremuchfasterduetoC-basedimplementation,2)Theyaremorememory-efficient,especiallywithlargedatasets,and3)Theyofferoptimized,vectorizedfunctionsformathematicalandstatisticaloperations,making


Hot AI Tools

Undresser.AI Undress
AI-powered app for creating realistic nude photos

AI Clothes Remover
Online AI tool for removing clothes from photos.

Undress AI Tool
Undress images for free

Clothoff.io
AI clothes remover

Video Face Swap
Swap faces in any video effortlessly with our completely free AI face swap tool!

Hot Article

Hot Tools

MinGW - Minimalist GNU for Windows
This project is in the process of being migrated to osdn.net/projects/mingw, you can continue to follow us there. MinGW: A native Windows port of the GNU Compiler Collection (GCC), freely distributable import libraries and header files for building native Windows applications; includes extensions to the MSVC runtime to support C99 functionality. All MinGW software can run on 64-bit Windows platforms.

PhpStorm Mac version
The latest (2018.2.1) professional PHP integrated development tool

SublimeText3 Linux new version
SublimeText3 Linux latest version

mPDF
mPDF is a PHP library that can generate PDF files from UTF-8 encoded HTML. The original author, Ian Back, wrote mPDF to output PDF files "on the fly" from his website and handle different languages. It is slower than original scripts like HTML2FPDF and produces larger files when using Unicode fonts, but supports CSS styles etc. and has a lot of enhancements. Supports almost all languages, including RTL (Arabic and Hebrew) and CJK (Chinese, Japanese and Korean). Supports nested block-level elements (such as P, DIV),
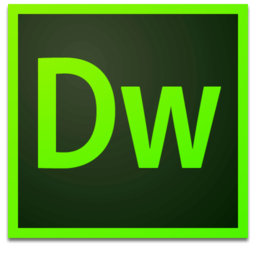
Dreamweaver Mac version
Visual web development tools
