NumPy arrays are essential for applications requiring efficient numerical computations and data manipulation. They are crucial in data science, machine learning, physics, engineering, and finance due to their ability to handle large-scale data efficiently. For example, in financial analysis, NumPy can calculate moving averages using np.convolve. However, users must be mindful of memory usage and balance performance with code readability.
NumPy arrays are commonly used in a wide range of applications, particularly those that require efficient numerical computations and data manipulation. Let's dive into some of these applications and explore why NumPy is so essential.
NumPy arrays are the backbone of many scientific and numerical computing tasks. If you're into data science, machine learning, or any field that deals with large datasets, you've probably used NumPy without even realizing it. Why? Because libraries like Pandas, Scikit-learn, and TensorFlow all rely heavily on NumPy under the hood.
Imagine you're working on a machine learning project. You need to preprocess your data, perhaps normalize it or perform feature scaling. NumPy arrays make these operations lightning fast and memory-efficient. They're designed to handle large-scale numerical data with ease, which is crucial when you're dealing with datasets that can easily reach millions of rows.
But it's not just about data science. NumPy arrays are also a go-to tool in fields like physics, engineering, and finance. For instance, in physics simulations, you might need to perform complex calculations on large matrices. NumPy's vectorized operations allow you to do this efficiently, without writing cumbersome loops.
Let's look at a practical example. Suppose you're analyzing stock prices over time. You could use NumPy to calculate moving averages, which is a common technique in financial analysis. Here's how you might do it:
import numpy as np # Sample stock prices prices = np.array([100, 102, 104, 103, 105, 107, 106, 108, 110, 112]) # Calculate a 3-day moving average window_size = 3 moving_averages = np.convolve(prices, np.ones(window_size), 'valid') / window_size print(moving_averages)
This code snippet demonstrates how effortlessly NumPy handles array operations. The np.convolve
function is used here to calculate the moving average, showcasing NumPy's ability to perform complex operations in a concise manner.
Now, let's talk about some of the challenges and considerations when using NumPy arrays. One common pitfall is memory management. While NumPy arrays are incredibly efficient, they can also consume a lot of memory, especially for large datasets. It's crucial to be mindful of your system's memory constraints and consider using memory-mapped arrays or chunking data when necessary.
Another aspect to consider is the trade-off between readability and performance. NumPy's vectorized operations are fast, but they can sometimes be less intuitive than traditional loops. It's important to strike a balance, ensuring your code is both efficient and readable.
In terms of best practices, always try to use NumPy's built-in functions whenever possible. For example, instead of writing a custom function to calculate the mean of an array, use np.mean()
. These functions are often optimized and can significantly improve performance.
To wrap up, NumPy arrays are indispensable in applications that require high-performance numerical computing. Whether you're analyzing financial data, running scientific simulations, or building machine learning models, NumPy's efficiency and versatility make it a cornerstone of modern data processing. Just remember to be mindful of memory usage and strive for a balance between performance and code readability. With these insights, you're well-equipped to harness the power of NumPy in your next project.
The above is the detailed content of In what types of applications are NumPy arrays commonly used?. For more information, please follow other related articles on the PHP Chinese website!
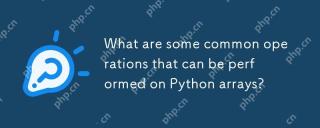
Pythonarrayssupportvariousoperations:1)Slicingextractssubsets,2)Appending/Extendingaddselements,3)Insertingplaceselementsatspecificpositions,4)Removingdeleteselements,5)Sorting/Reversingchangesorder,and6)Listcomprehensionscreatenewlistsbasedonexistin
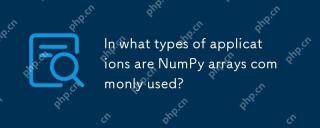
NumPyarraysareessentialforapplicationsrequiringefficientnumericalcomputationsanddatamanipulation.Theyarecrucialindatascience,machinelearning,physics,engineering,andfinanceduetotheirabilitytohandlelarge-scaledataefficiently.Forexample,infinancialanaly
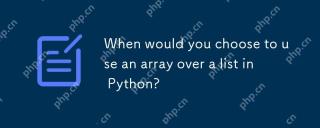
Useanarray.arrayoveralistinPythonwhendealingwithhomogeneousdata,performance-criticalcode,orinterfacingwithCcode.1)HomogeneousData:Arrayssavememorywithtypedelements.2)Performance-CriticalCode:Arraysofferbetterperformancefornumericaloperations.3)Interf
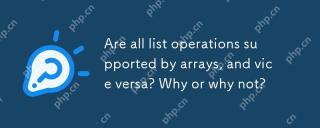
No,notalllistoperationsaresupportedbyarrays,andviceversa.1)Arraysdonotsupportdynamicoperationslikeappendorinsertwithoutresizing,whichimpactsperformance.2)Listsdonotguaranteeconstanttimecomplexityfordirectaccesslikearraysdo.
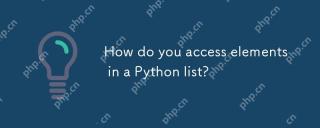
ToaccesselementsinaPythonlist,useindexing,negativeindexing,slicing,oriteration.1)Indexingstartsat0.2)Negativeindexingaccessesfromtheend.3)Slicingextractsportions.4)Iterationusesforloopsorenumerate.AlwayschecklistlengthtoavoidIndexError.
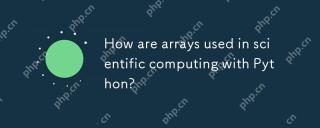
ArraysinPython,especiallyviaNumPy,arecrucialinscientificcomputingfortheirefficiencyandversatility.1)Theyareusedfornumericaloperations,dataanalysis,andmachinelearning.2)NumPy'simplementationinCensuresfasteroperationsthanPythonlists.3)Arraysenablequick
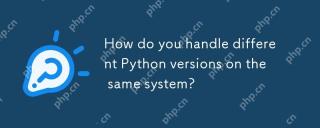
You can manage different Python versions by using pyenv, venv and Anaconda. 1) Use pyenv to manage multiple Python versions: install pyenv, set global and local versions. 2) Use venv to create a virtual environment to isolate project dependencies. 3) Use Anaconda to manage Python versions in your data science project. 4) Keep the system Python for system-level tasks. Through these tools and strategies, you can effectively manage different versions of Python to ensure the smooth running of the project.
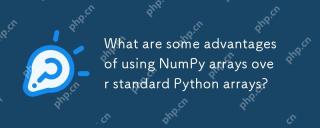
NumPyarrayshaveseveraladvantagesoverstandardPythonarrays:1)TheyaremuchfasterduetoC-basedimplementation,2)Theyaremorememory-efficient,especiallywithlargedatasets,and3)Theyofferoptimized,vectorizedfunctionsformathematicalandstatisticaloperations,making


Hot AI Tools

Undresser.AI Undress
AI-powered app for creating realistic nude photos

AI Clothes Remover
Online AI tool for removing clothes from photos.

Undress AI Tool
Undress images for free

Clothoff.io
AI clothes remover

Video Face Swap
Swap faces in any video effortlessly with our completely free AI face swap tool!

Hot Article

Hot Tools

Notepad++7.3.1
Easy-to-use and free code editor

PhpStorm Mac version
The latest (2018.2.1) professional PHP integrated development tool

SAP NetWeaver Server Adapter for Eclipse
Integrate Eclipse with SAP NetWeaver application server.

MinGW - Minimalist GNU for Windows
This project is in the process of being migrated to osdn.net/projects/mingw, you can continue to follow us there. MinGW: A native Windows port of the GNU Compiler Collection (GCC), freely distributable import libraries and header files for building native Windows applications; includes extensions to the MSVC runtime to support C99 functionality. All MinGW software can run on 64-bit Windows platforms.
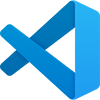
VSCode Windows 64-bit Download
A free and powerful IDE editor launched by Microsoft
