


With just a few tweaks, the large model support context size can be extended from 16,000 tokens to 1 million? !
Still on LLaMA 2 which has only 7 billion parameters.
You must know that even the most popular Claude 2 and GPT-4 support context lengths of only 100,000 and 32,000. Beyond this range, large models will start to talk nonsense and be unable to remember things.
Now, a new study from Fudan University and Shanghai Artificial Intelligence Laboratory has not only found a way to increase the length of the context window for a series of large models, but also discovered the rules.
According to this rule, only need to adjust 1 hyperparameter, can ensure the output effect while stably improving the large modelExtrapolation performance.
Extrapolation refers to the change in output performance when the input length of the large model exceeds the length of the pre-trained text. If the extrapolation ability is not good, once the input length exceeds the length of the pre-trained text, the large model will "talk nonsense".
So, what exactly can it improve the extrapolation capabilities of large models, and how does it do it?
"Mechanism" to improve the extrapolation ability of large models
This method of improving the extrapolation ability of large models is the same as the position coding in the Transformer architecture. module related.
In fact, the simple attention mechanism (Attention) module cannot distinguish tokens in different positions. For example, "I eat apples" and "apples eat me" have no difference in its eyes. Therefore, position coding needs to be added to allow it to understand the word order information and truly understand the meaning of a sentence. The current Transformer position encoding methods include absolute position encoding (integrating position information into the input), relative position encoding (writing position information into attention score calculation) and rotation position encoding. Among them, the most popular one is the rotational position encoding, which isRoPE.
RoPE achieves the effect of relative position encoding through absolute position encoding, but compared with relative position encoding, it can better improve the extrapolation potential of large models. How to further stimulate the extrapolation capabilities of large models using RoPE position encoding has become a new direction of many recent studies. These studies are mainly divided into two major schools:Limiting attention and Adjusting rotation angle.
Representative research on limiting attention includes ALiBi, xPos, BCA, etc. The StreamingLLM recently proposed by MIT can allow large models to achieve infinite input length (but does not increase the context window length), which belongs to the type of research in this direction.There is more work to adjust the rotation angle, typical representatives such as linear interpolation, Giraffe, Code LLaMA, LLaMA2 Long et al. all belong to this type of research.
32,000 tokens.
This hyperparameter is exactly the"switch" found by Code LLaMA and LLaMA2 Long et al.——
rotation angle base(base ).
Just fine-tune it to ensure that the extrapolation performance of large models is improved. But whether it is Code LLaMA or LLaMA2 Long, they are only fine-tuned on a specific base and continued training length to enhance their extrapolation capabilities. Is it possible to find a pattern to ensure thatall large models using RoPE position encoding can steadily improve the extrapolation performance?
Master this rule, the context is easy 100w Researchers from Fudan University and Shanghai AI Research Institute conducted experiments on this problem. They first analyzed several parameters that affect the RoPE extrapolation ability, and proposed a concept calledCritical Dimension (Critical Dimension). Then based on this concept, they concluded A set of RoPE extrapolation scaling laws (Scaling Laws of RoPE-based Extrapolation).
Just apply thisrule to ensure that any large model based on RoPE positional encoding can improve extrapolation capabilities.
Let’s first look at what the critical dimension is.From the definition, it is related to the pre-training text length Ttrain, the number of self-attention head dimensions d and other parameters. The specific calculation method is as follows:
Among them, 10000 is the "initial value" of the hyperparameter and rotation angle base.
The author found that whether the base is enlarged or reduced, the extrapolation ability of the large model based on RoPE can be enhanced in the end. In contrast, when the base of the rotation angle is 10000, the extrapolation ability of the large model is the best. Poor.
This paper believes that a smaller base of the rotation angle can allow more dimensions to perceive position information, and a larger base of the rotation angle, then Can express longer location information.
In this case, when facing continued training corpus of different lengths, how much rotation angle base should be reduced and enlarged to ensure that the extrapolation ability of the large model is maximized? To what extent?
The paper gives a scaling rule for extended RoPE extrapolation, which is related to parameters such as critical dimensions, continued training text length and pre-training text length of large models:
Based on this rule, the extrapolation performance of the large model can be directly calculated based on different pre-training and continued training text lengths. In other words, the context length supported by the large model is predicted.
On the contrary, using this rule, we can also quickly deduce how to best adjust the base of the rotation angle, thereby improving the extrapolation performance of large models.
The author tested this series of tasks and found that currently inputting 100,000, 500,000, or even 1 million tokens lengths can ensure that extrapolation can be achieved without additional attention restrictions.
At the same time, work on enhancing the extrapolation capabilities of large models, including Code LLaMA and LLaMA2 Long, has proven that this rule is indeed reasonable and effective.
In this way, you only need to "adjust a parameter" according to this rule, and you can easily expand the context window length of the large model based on RoPE and enhance the extrapolation capability.
Liu Xiaoran, the first author of the paper, said that this research is still improving the downstream task effect by improving the continued training corpus. After completion, the code and model will be open source. You can look forward to it~
Paper address:
https://arxiv.org/abs/2310.05209
Github repository:
https://github.com/OpenLMLab/scaling-rope
Paper analysis blog:
https:// zhuanlan.zhihu.com/p/660073229
The above is the detailed content of The LLaMA2 context length skyrockets to 1 million tokens, with only one hyperparameter need to be adjusted.. For more information, please follow other related articles on the PHP Chinese website!
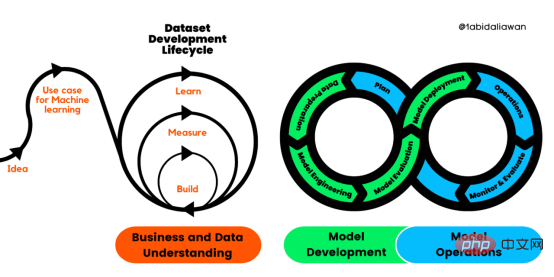
译者 | 布加迪审校 | 孙淑娟目前,没有用于构建和管理机器学习(ML)应用程序的标准实践。机器学习项目组织得不好,缺乏可重复性,而且从长远来看容易彻底失败。因此,我们需要一套流程来帮助自己在整个机器学习生命周期中保持质量、可持续性、稳健性和成本管理。图1. 机器学习开发生命周期流程使用质量保证方法开发机器学习应用程序的跨行业标准流程(CRISP-ML(Q))是CRISP-DM的升级版,以确保机器学习产品的质量。CRISP-ML(Q)有六个单独的阶段:1. 业务和数据理解2. 数据准备3. 模型
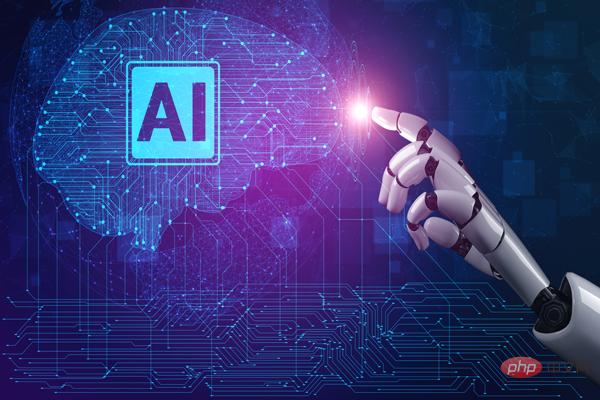
人工智能(AI)在流行文化和政治分析中经常以两种极端的形式出现。它要么代表着人类智慧与科技实力相结合的未来主义乌托邦的关键,要么是迈向反乌托邦式机器崛起的第一步。学者、企业家、甚至活动家在应用人工智能应对气候变化时都采用了同样的二元思维。科技行业对人工智能在创建一个新的技术乌托邦中所扮演的角色的单一关注,掩盖了人工智能可能加剧环境退化的方式,通常是直接伤害边缘人群的方式。为了在应对气候变化的过程中充分利用人工智能技术,同时承认其大量消耗能源,引领人工智能潮流的科技公司需要探索人工智能对环境影响的
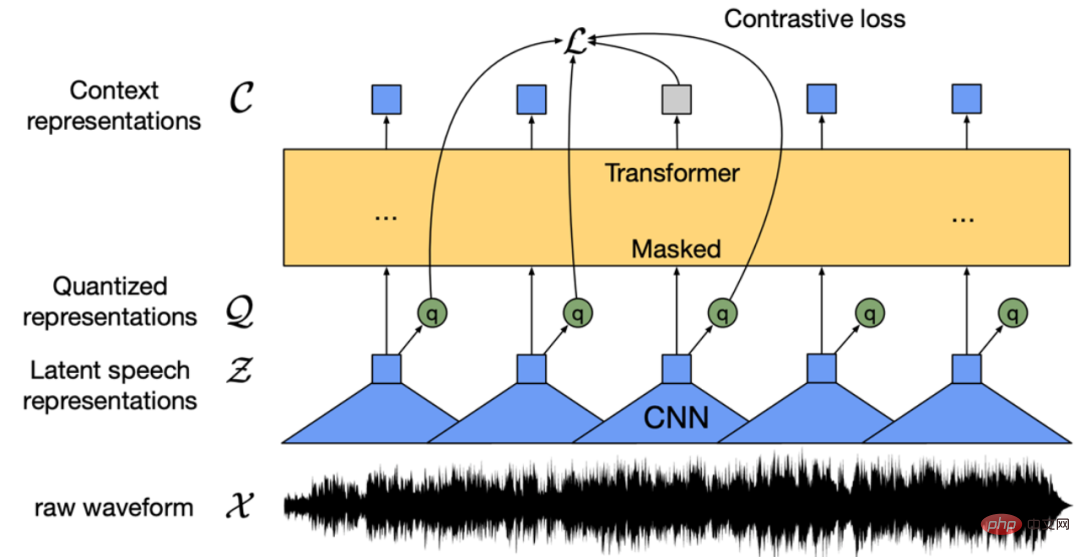
Wav2vec 2.0 [1],HuBERT [2] 和 WavLM [3] 等语音预训练模型,通过在多达上万小时的无标注语音数据(如 Libri-light )上的自监督学习,显著提升了自动语音识别(Automatic Speech Recognition, ASR),语音合成(Text-to-speech, TTS)和语音转换(Voice Conversation,VC)等语音下游任务的性能。然而这些模型都没有公开的中文版本,不便于应用在中文语音研究场景。 WenetSpeech [4] 是
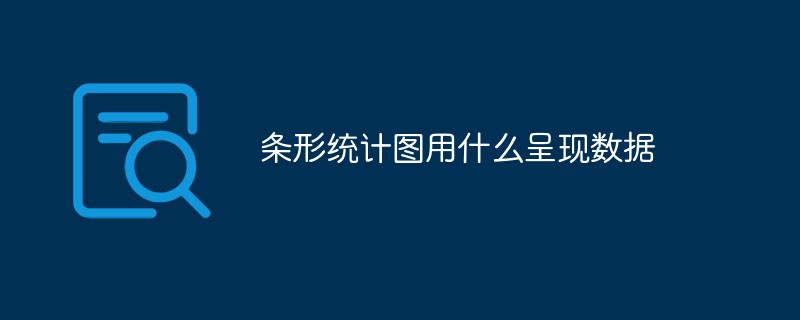
条形统计图用“直条”呈现数据。条形统计图是用一个单位长度表示一定的数量,根据数量的多少画成长短不同的直条,然后把这些直条按一定的顺序排列起来;从条形统计图中很容易看出各种数量的多少。条形统计图分为:单式条形统计图和复式条形统计图,前者只表示1个项目的数据,后者可以同时表示多个项目的数据。

arXiv论文“Sim-to-Real Domain Adaptation for Lane Detection and Classification in Autonomous Driving“,2022年5月,加拿大滑铁卢大学的工作。虽然自主驾驶的监督检测和分类框架需要大型标注数据集,但光照真实模拟环境生成的合成数据推动的无监督域适应(UDA,Unsupervised Domain Adaptation)方法则是低成本、耗时更少的解决方案。本文提出对抗性鉴别和生成(adversarial d
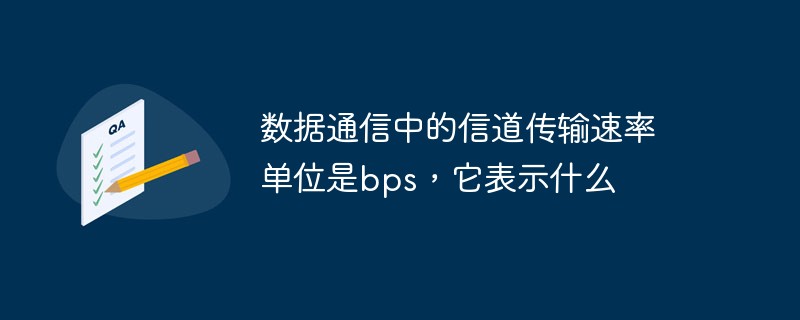
数据通信中的信道传输速率单位是bps,它表示“位/秒”或“比特/秒”,即数据传输速率在数值上等于每秒钟传输构成数据代码的二进制比特数,也称“比特率”。比特率表示单位时间内传送比特的数目,用于衡量数字信息的传送速度;根据每帧图像存储时所占的比特数和传输比特率,可以计算数字图像信息传输的速度。
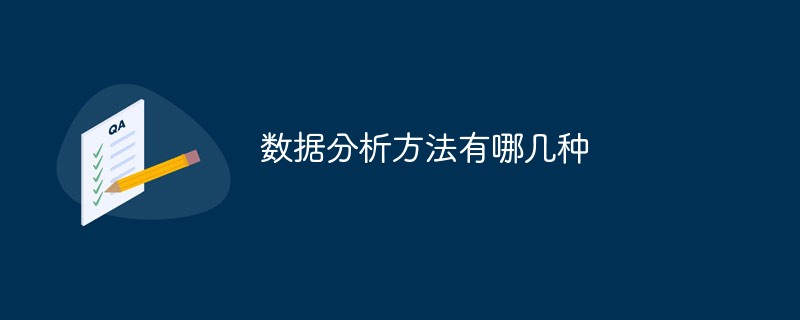
数据分析方法有4种,分别是:1、趋势分析,趋势分析一般用于核心指标的长期跟踪;2、象限分析,可依据数据的不同,将各个比较主体划分到四个象限中;3、对比分析,分为横向对比和纵向对比;4、交叉分析,主要作用就是从多个维度细分数据。
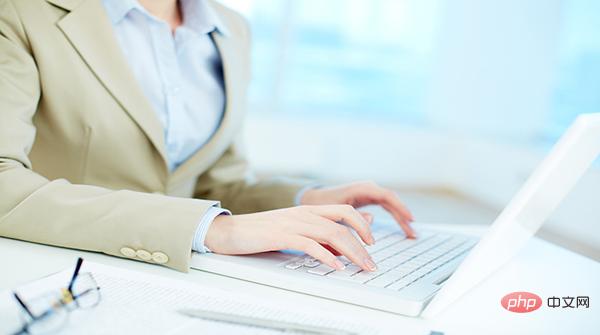
在日常开发中,对数据进行序列化和反序列化是常见的数据操作,Python提供了两个模块方便开发者实现数据的序列化操作,即 json 模块和 pickle 模块。这两个模块主要区别如下:json 是一个文本序列化格式,而 pickle 是一个二进制序列化格式;json 是我们可以直观阅读的,而 pickle 不可以;json 是可互操作的,在 Python 系统之外广泛使用,而 pickle 则是 Python 专用的;默认情况下,json 只能表示 Python 内置类型的子集,不能表示自定义的


Hot AI Tools

Undresser.AI Undress
AI-powered app for creating realistic nude photos

AI Clothes Remover
Online AI tool for removing clothes from photos.

Undress AI Tool
Undress images for free

Clothoff.io
AI clothes remover

AI Hentai Generator
Generate AI Hentai for free.

Hot Article

Hot Tools

MantisBT
Mantis is an easy-to-deploy web-based defect tracking tool designed to aid in product defect tracking. It requires PHP, MySQL and a web server. Check out our demo and hosting services.

mPDF
mPDF is a PHP library that can generate PDF files from UTF-8 encoded HTML. The original author, Ian Back, wrote mPDF to output PDF files "on the fly" from his website and handle different languages. It is slower than original scripts like HTML2FPDF and produces larger files when using Unicode fonts, but supports CSS styles etc. and has a lot of enhancements. Supports almost all languages, including RTL (Arabic and Hebrew) and CJK (Chinese, Japanese and Korean). Supports nested block-level elements (such as P, DIV),

Zend Studio 13.0.1
Powerful PHP integrated development environment
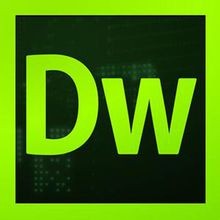
Dreamweaver CS6
Visual web development tools

Safe Exam Browser
Safe Exam Browser is a secure browser environment for taking online exams securely. This software turns any computer into a secure workstation. It controls access to any utility and prevents students from using unauthorized resources.
