


Using Azure Semantic Search and OpenAI to build a cognitive search system
In today’s digital age, having powerful, scalable and efficient systems is more than just a competitive advantage; it's necessary. Whether you're working to optimize user input processing to simplify document searches, a combination of services and platforms is the key to unparalleled performance. In this article, we'll explore a holistic approach that combines the power of Azure Cognitive Services with the capabilities of OpenAI. By delving into intent recognition, document filtering, domain-specific algorithms, and text summarization, you'll learn to create a system that not only understands user intent but also processes and presents information efficiently.
We will build this:
Setting up the environment
Before we dive in, let’s make sure we have it installed Download the necessary packages and set the environment variables:
!pip show azure-search-documents %pip install azure-search-documents --pre %pip show azure-search-documents !pip install python-dotenv !pip install openai import os import requests import json import openai openai.api_key = os.getenv("AZURE_OPENAI_KEY") openai.api_base = os.getenv("AZURE_OPENAI_ENDPOINT") openai.api_type = 'azure' openai.api_version = '2023-05-15' # Look in Azure OpenAI Studio > Deployments deployment_name = 'gpt-35-turbo'
Here, we set up the OpenAI environment with the necessary API keys, endpoints and types.
Set up Azure Search
To use Azure Semantic Search, we need to import the necessary modules and set up the environment.
import os from azure.core.credentials import AzureKeyCredential from azure.search.documents.indexes import SearchIndexClient from azure.search.documents import SearchClient from azure.search.documents.indexes.models import ( ComplexField, CorsOptions, SearchIndex, ScoringProfile, SearchFieldDataType, SimpleField, SearchableField )
After importing the module, we can now set up the Azure Search Service endpoint and API key:
# 从环境中设置服务端点和 API 密钥 service_name = "xxxxx" admin_key ="xxxxx" # 如果您共享密钥 - 请确保您的 index_name 是唯一的! index_name = "hotels-quickstart" # 创建 SDK 客户 endpoint = "https://{}.search.windows.net/".format(service_name) admin_client = SearchIndexClient(endpoint=endpoint, index_name=index_name, credential=AzureKeyCredential(admin_key)) search_client = SearchClient(endpoint=endpoint, index_name=index_name, credential=AzureKeyCredential(admin_key))
(Note: Be sure to mask or hide your key before sharing any code. )
Preparing indexes for Azure Semantic Search
Before adding data to Azure Search, we need to define an index that describes the structure of the data:
# 删除索引(如果存在) try: result = admin_client.delete_index(index_name) print ('Index', index_name, 'Deleted') except Exception as ex: print (ex)
This code snippet ensures that if the index already exists, it is deleted. This is useful when rerunning code or changing indexes.
Now, let us specify the schema of the index:
# 指定索引模式 name = index_name fields = [ SimpleField(name= "HotelId" , type=SearchFieldDataType.String, key= True ), SearchableField(name= "HotelName" , type=SearchFieldDataType.String, sortable= True ), SearchableField (名称= “描述”,类型=SearchFieldDataType.String,analyzer_name= “en.lucene”), SearchableField(名称= “Description_fr”,类型=SearchFieldDataType.String,analyzer_name= “fr.lucene”), SearchableField(名称= “类别”、 type=SearchFieldDataType.String、facetable= True、filterable= True、sortable= True )、 SearchableField(name= "Tags"、collection= True、type=SearchFieldDataType.String、facetable= True、filterable= True )、 SimpleField(name = “ParkingInincluded”,类型=SearchFieldDataType.Boolean,facetable= True,filterable= True,sortable= True), SimpleField(name= “LastRenovationDate”,type=SearchFieldDataType.DateTime关闭设置,facetable= True,filterable=True、sortable= True )、 SimpleField(name= "Rating"、 type=SearchFieldDataType.Double、facetable= True、filterable= True、sortable= True )、 ComplexField(name= "地址"、 fields=[ SearchableField(name= " StreetAddress"、 type=SearchFieldDataType.String)、 SearchableField(name= "City"、 type=SearchFieldDataType.String、facetable= True、 filterable= True、 sortable= True )、 SearchableField(name= "StateProvince"、 type=SearchFieldDataType.String、facetable= True、filterable= True、sortable= True )、 SearchableField(name= "邮政编码"、 type=SearchFieldDataType.String、facetable= True、filterable= True、sortable= True )、 SearchableField(name = “国家”,类型= SearchFieldDataType.String,facetable = True,filterable = True,sortable = True), ]) ] cors_options = CorsOptions(allowed_origins = [ “*” ],max_age_in_seconds = 60) Scoring_profiles = [] suggester = [{ 'name' : 'sg' , 'source_fields' : [ '标签' , '地址/城市' , '地址/国家' ]}]
Next, you have to create this index on Azure:
index = SearchIndex( name=name, fields=fields, scoring_profiles=scoring_profiles, suggesters = suggester, cors_options=cors_options) try: result = admin_client.create_index(index) print ('Index', result.name, 'created') except Exception as ex: print (ex)
After creating the index, we need to populate it with documents it. It’s important to point out that it can be any type of document! I just manually add the documents that will persist in the blob storage here:
文档 = [ { "@search.action": "上传", "@search.action" : "上传" , "HotelId" : "1" , "HotelName" : "秘密点汽车旅馆" , "Description" : "酒店地理位置优越,位于纽约市中心的城市主要商业干道上。几分钟即可到达时代广场和城市的历史中心,以及使纽约成为美国最具吸引力的城市之一的其他名胜古迹和国际大都市。” , “Description_fr”:“L'hôtel est idéalement situé sur la prime artère Commerciale de la ville en plein cœur de New York.A insi que d'autres lieux d'intérêt qui font纽约的城市充满魅力和美国的国际化。” , "Category" : "精品店" , "Tags" : [ "游泳池" , "空调" , "礼宾服务" ], "ParkingInincluded" : "false" , "LastRenovationDate" : "1970-01-18T00:00:00Z ”, "Rating" : 3.60 , "Address" : { “StreetAddress”:“677 第五大道”, “City”:“纽约”, “StateProvince”:“纽约” , “PostalCode”:“10022”, “Country”:“美国” } }, { “@search. action" : "上传" , "HotelId" : "2" , "HotelName" : "双圆顶汽车旅馆" , "Description" :“该酒店坐落在一座十九世纪的广场上,该广场已按照最高建筑标准进行扩建和翻新,打造出一座现代化、实用的一流酒店,艺术和独特的历史元素与最现代的舒适设施共存。” , "Description_fr" : "L'hôtel 位于十九世纪的地方,是一座现代化酒店的高级规范建筑,在艺术和历史独特方面具有一流的功能和一流的设计舒适与现代共存。” , "Category" : "精品店" , "Tags" : [ "泳池" ,], "ParkingInincluded" : "false" , "LastRenovationDate" : "1979-02-18T00:00:00Z" , "Rating" : 3.60 , "Address" : { "StreetAddress" : "140 大学城中心" , "City”:“萨拉索塔”, “StateProvince”:“佛罗里达州”, “PostalCode”:“34243”, “Country”:“美国” } }, { "@search.action" : "上传" , "HotelId" :"3" , "HotelName" : "三重景观酒店" , "Description" : "该酒店在 William Dough 的管理下以其卓越的美食脱颖而出,他为酒店的所有餐厅服务提供建议并监督。" , "Description_fr" : "L'hôtel 位于十九世纪的地方,是一座现代化酒店的高级规范建筑,在艺术和历史独特方面具有一流的功能和一流的设计舒适与现代共存。” , "Category" : "度假村中心" , "Tags" : [ "酒吧" , "欧陆式早餐" ], "ParkingInincluded" : "true" , "LastRenovationDate" : "2015-09-20T00:00:00Z" , "Rating" : 4.80 , "Address" : { "StreetAddress" : “3393 Peachtree Rd”、 “City”:“亚特兰大”、 “StateProvince”:“GA”、 “PostalCode”:“30326”、 “Country”:“美国” } } ]
Now push these documents to the semantic search index.
try: result = search_client.upload_documents(documents=documents) print("Upload of new document succeeded: {}".format(result[0].succeeded)) except Exception as ex: print (ex.message)
Integration with OpenAI
Let’s establish a connection to OpenAI:
question="What is the address of ChatGpt Hotel?"
Then, add the Azure OpenAI connection:
### import os import requests import json import openai os.environ["AZURE_OPENAI_KEY"] = "xxxx" os.environ["AZURE_OPENAI_ENDPOINT"] = "xxxx" openai.api_key = os.getenv("AZURE_OPENAI_KEY") openai.api_base = os.getenv("AZURE_OPENAI_ENDPOINT") openai.api_type = 'azure' openai.api_version = '2023-05-15' # 在 Azure OpenAI Studio > 部署中查找 deployment_name = 'gpt-35-turbo' ### # 定义一个函数,根据系统消息和消息创建提示 def create_prompt(system_message, messages): prompt = system_message message_template = "\n<|im_start|>{}\n{}\n<|im_end|>" for message in messages: prompt += message_template.format(message['sender'], message['text']) prompt += "\n<|im_start|>assistant\n" return prompt # 定义系统消息 system_message_template = "<|im_start|>system\n{}\n<|im_end|>" system_message = system_message_template.format("") print(system_message)
At this point, you can use semantic search and Azure OpenAI. Let’s query Semantic Search:
import json results = search_client.search(search_text=question, include_total_count=True, select='HotelId,HotelName,Tags,Address')= search_client.search(search_text=question, include_total_count=True, select='HotelId,HotelName,Tags,Address') json_results="" print ('Total Documents Matching Query:', results.get_count()) for result in results: #print("{}: {}: {}".format(result["HotelId"], result["HotelName"], result["Tags"],results["Address"])) json_results+=str(result) print(json_results)
With the search results in hand, we can now leverage Azure OpenAI to interpret or further process the results.
# 创建消息列表来跟踪对话 messages = [{"sender": "user", "text": "Hello, take into account the following information "+json_results}, {"sender": "user", "text": question}, ] response = openai.Completion.create( engine=deployment_name, prompt= create_prompt(system_message, messages), temperature=0.7, max_tokens=800, top_p=0.95, frequency_penalty=0, presence_penalty=0, stop=["<|im_end|>"]) print(response)
This code prompts the OpenAI model with search results and our original question, allowing it to process and provide meaningful information based on the data.
Conclusion
In this article, we learned how to combine the power of Azure Semantic Search with the capabilities of OpenAI. By integrating these two powerful tools, we can provide users with rich, intelligent search results in our applications.
The above is the detailed content of Using Azure Semantic Search and OpenAI to build a cognitive search system. For more information, please follow other related articles on the PHP Chinese website!
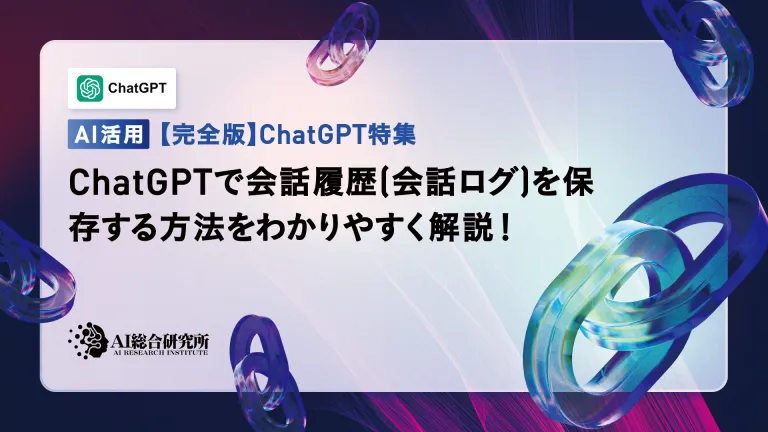
Various ways to efficiently save ChatGPT dialogue records Have you ever thought about saving a ChatGPT-generated conversation record? This article will introduce a variety of saving methods in detail, including official functions, Chrome extensions and screenshots, etc., to help you make full use of ChatGPT conversation records. Understand the characteristics and steps of various methods and choose the one that suits you best. [Introduction to the latest AI proxy "OpenAI Operator" released by OpenAI] (The link to OpenAI Operator should be inserted here) Table of contents Save conversation records using ChatGPT Export Steps to use the official export function Save ChatGPT logs using Chrome extension ChatGP
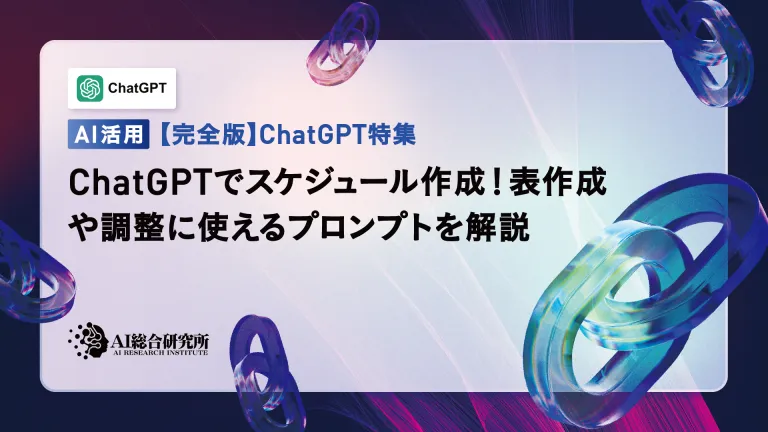
Modern society has a compact pace and efficient schedule management is crucial. Work, life, study and other tasks are intertwined, and prioritization and schedules are often a headache. Therefore, intelligent schedule management methods using AI technology have attracted much attention. In particular, ChatGPT's powerful natural language processing capabilities can automate tedious schedules and task management, significantly improving productivity. This article will explain in-depth how to use ChatGPT for schedule management. We will combine specific cases and steps to demonstrate how AI can improve daily life and work efficiency. In addition, we will discuss things to note when using ChatGPT to ensure safe and effective use of this technology. Experience ChatGPT now and get your schedule
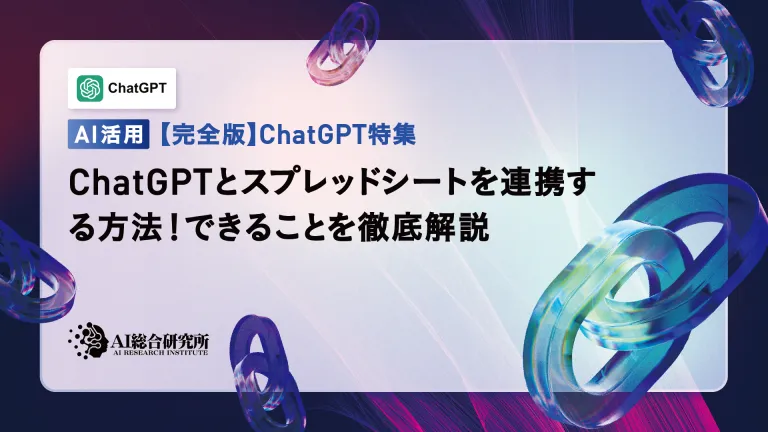
We will explain how to link Google Sheets and ChatGPT to improve business efficiency. In this article, we will explain in detail how to use the add-on "GPT for Sheets and Docs" that is easy for beginners to use. No programming knowledge is required. Increased business efficiency through ChatGPT and spreadsheet integration This article will focus on how to connect ChatGPT with spreadsheets using add-ons. Add-ons allow you to easily integrate ChatGPT features into your spreadsheets. GPT for Shee
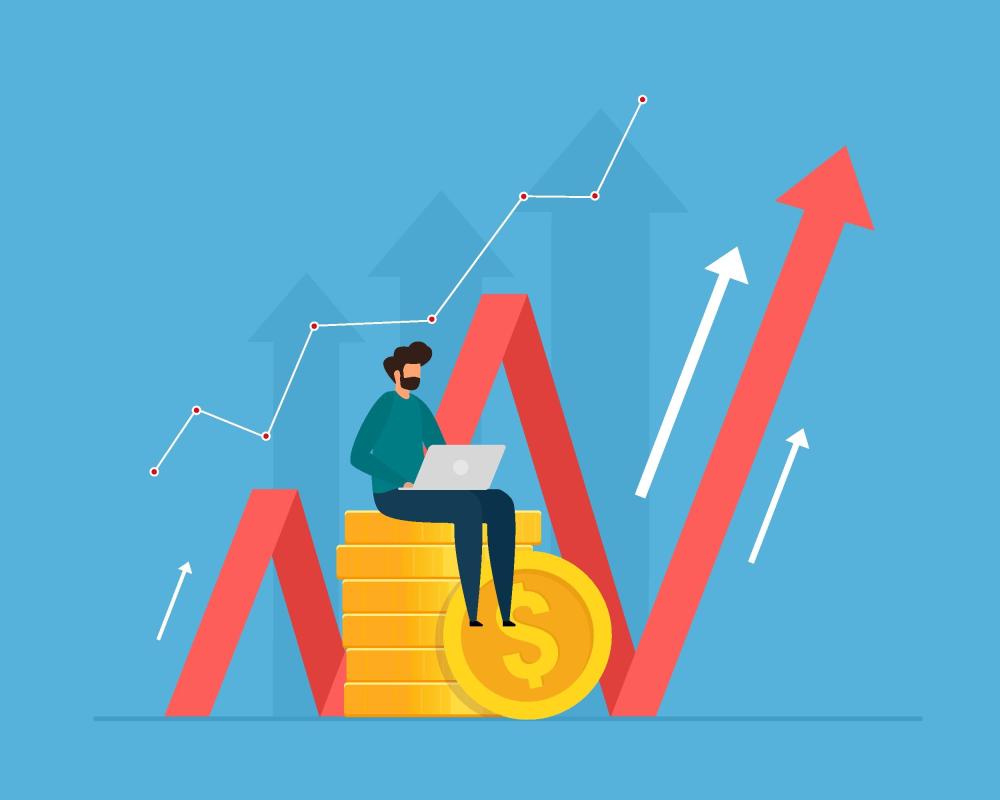
There are overarching trends and patterns that experts are highlighting as they forecast the next few years of the AI revolution. For instance, there's a significant demand for data, which we will discuss later. Additionally, the need for energy is d
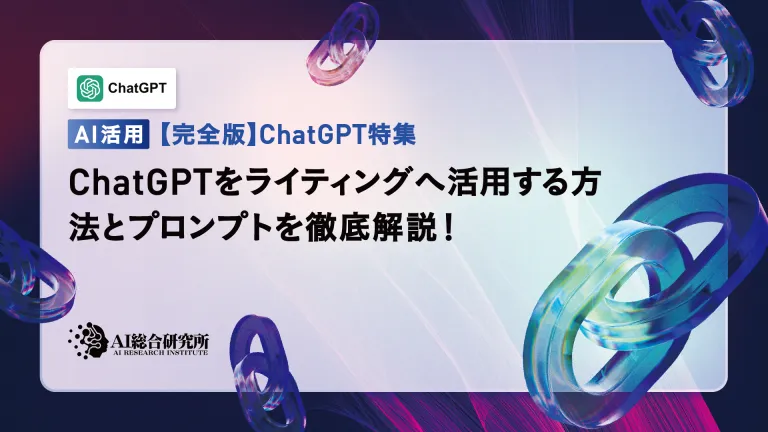
ChatGPT is not just a text generation tool, it is a true partner that dramatically increases writers' creativity. By using ChatGPT for the entire writing process, such as initial manuscript creation, ideation ideas, and stylistic changes, you can simultaneously save time and improve quality. This article will explain in detail the specific ways to use ChatGPT at each stage, as well as tips for maximizing productivity and creativity. Additionally, we will examine the synergy that combines ChatGPT with grammar checking tools and SEO optimization tools. Through collaboration with AI, writers can create originality with free ideas
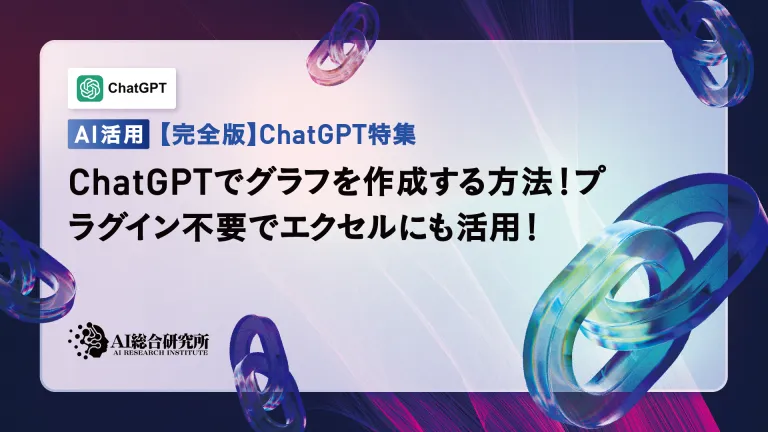
Data visualization using ChatGPT: From graph creation to data analysis Data visualization, which conveys complex information in an easy-to-understand manner, is essential in modern society. In recent years, due to the advancement of AI technology, graph creation using ChatGPT has attracted attention. In this article, we will explain how to create graphs using ChatGPT in an easy-to-understand manner even for beginners. We will introduce the differences between the free version and the paid version (ChatGPT Plus), specific creation steps, and how to display Japanese labels, along with practical examples. Creating graphs using ChatGPT: From basics to advanced use ChatG
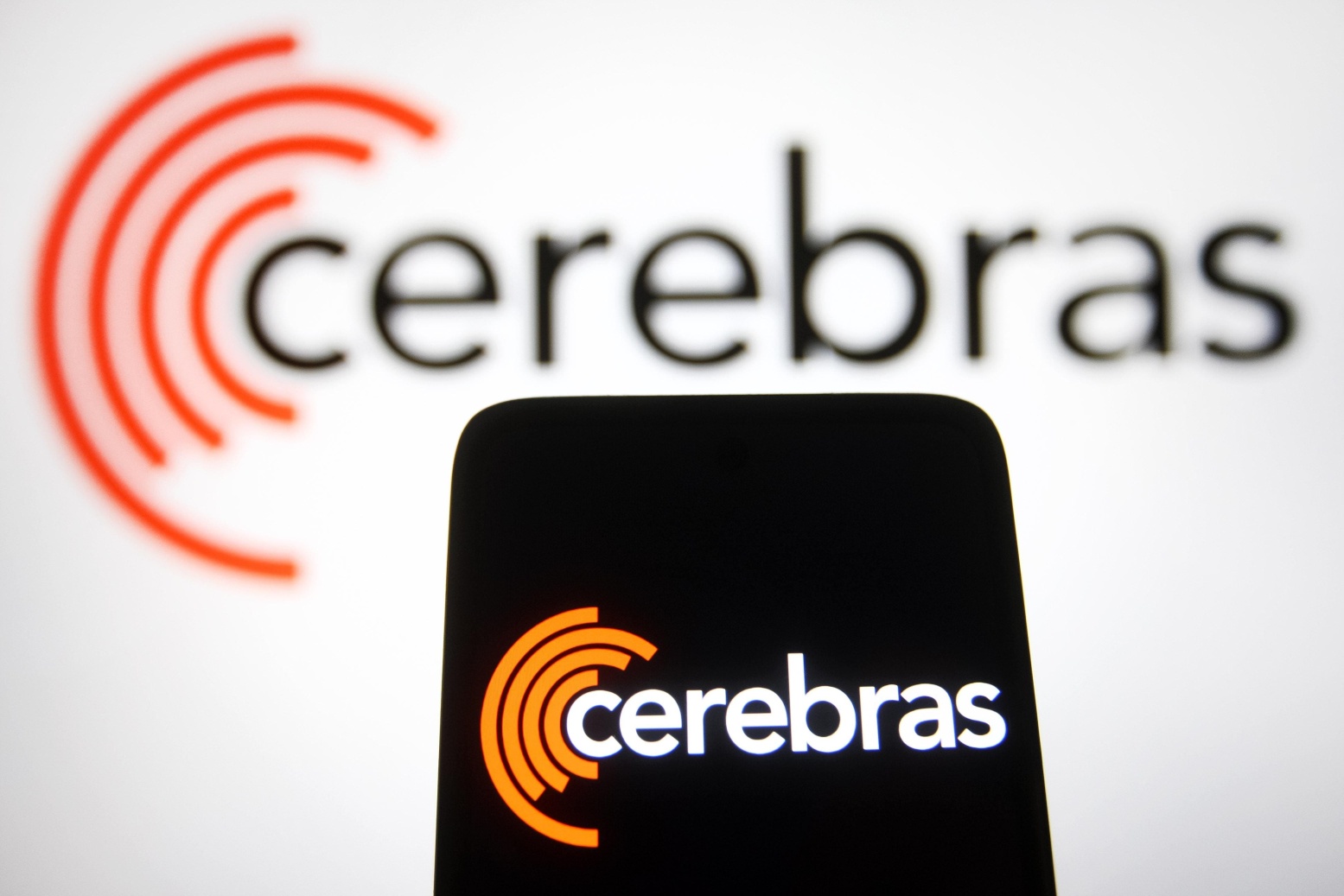
In general, we know that AI is big, and getting bigger. It’s fast, and getting faster. Specifically, though, not everyone’s familiar with some of the newest hardware and software approaches in the industry, and how they promote better results. Peopl
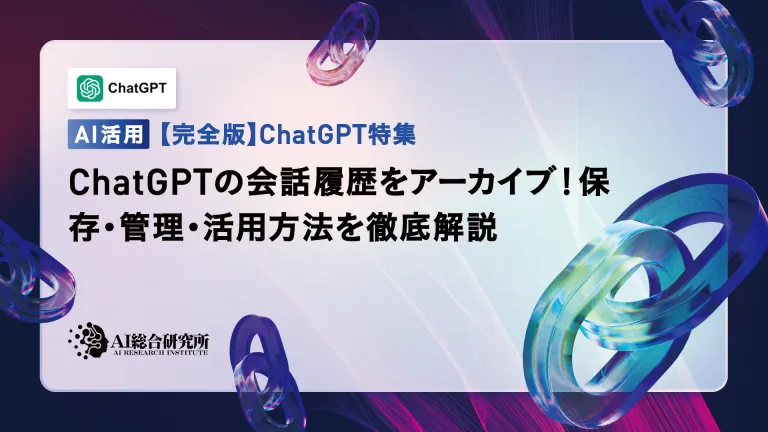
ChatGPT Dialogue Record Management Guide: Efficiently organize and make full use of your treasure house of knowledge! ChatGPT dialogue records are a source of creativity and knowledge, but how can growing records be effectively managed? Is it time-consuming to find important information? don’t worry! This article will explain in detail how to effectively "archive" (save and manage) your ChatGPT conversation records. We will cover official archive functions, data export, shared links, and data utilization and considerations. Table of contents Detailed explanation of ChatGPT's "archive" function How to use ChatGPT archive function Save location and viewing method of ChatGPT archive records Cancel and delete methods for ChatGPT archive records Cancel archive Delete the archive Summarize Ch


Hot AI Tools

Undresser.AI Undress
AI-powered app for creating realistic nude photos

AI Clothes Remover
Online AI tool for removing clothes from photos.

Undress AI Tool
Undress images for free

Clothoff.io
AI clothes remover

Video Face Swap
Swap faces in any video effortlessly with our completely free AI face swap tool!

Hot Article

Hot Tools

Zend Studio 13.0.1
Powerful PHP integrated development environment
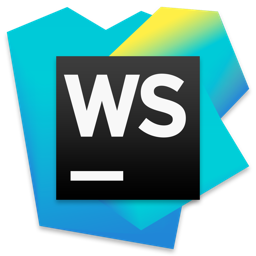
WebStorm Mac version
Useful JavaScript development tools

SublimeText3 English version
Recommended: Win version, supports code prompts!

SublimeText3 Chinese version
Chinese version, very easy to use

PhpStorm Mac version
The latest (2018.2.1) professional PHP integrated development tool
