


Title: Python problems encountered in parallel programming and solution strategies
Abstract:
With the continuous development of computer technology, for data processing and computing capabilities The demand is growing. Parallel programming has become one of the important ways to improve computing efficiency. In Python, we can use multi-threading, multi-process and asynchronous programming to achieve parallel computing. However, parallel programming also brings a series of problems, such as the management of shared resources, thread safety and performance issues. This article will introduce common Python problems in parallel programming, and provide corresponding solution strategies and specific code examples.
1. Global Interpreter Lock (GIL) in Python
In Python, the Global Interpreter Lock (GIL) is a controversial issue. The existence of GIL makes Python's multi-threading not really capable of parallel execution. When multiple threads need to perform CPU-intensive tasks simultaneously, the GIL can become a performance bottleneck. In order to solve this problem, we can consider using multi-process instead of multi-thread, and use inter-process communication to achieve data sharing.
The following is a sample code that uses multi-process instead of multi-thread:
from multiprocessing import Process def worker(num): print(f'Worker {num} started') # 执行耗时任务 print(f'Worker {num} finished') if __name__ == '__main__': processes = [] for i in range(5): process = Process(target=worker, args=(i,)) process.start() processes.append(process) for process in processes: process.join()
2. Management of shared resources
In parallel programming, multiple threads or processes may access shared resources at the same time , such as database connections, files, etc. This can lead to problems such as resource contention and data corruption. In order to solve this problem, we can use thread lock (Lock) or process lock (Lock) to achieve synchronous access to shared resources.
The following is a sample code for using thread lock:
import threading counter = 0 lock = threading.Lock() def worker(): global counter for _ in range(1000000): lock.acquire() counter += 1 lock.release() threads = [] for _ in range(4): thread = threading.Thread(target=worker) thread.start() threads.append(thread) for thread in threads: thread.join() print(f'Counter value: {counter}')
3. Thread safety
In a multi-threaded environment, multiple threads may access the same object or data structure at the same time. question. If thread safety is not handled correctly, data errors or crashes can result. In order to solve this problem, we can use thread-safe data structures or use thread locks (Lock) to ensure data consistency.
The following is a sample code that uses a thread-safe queue (Queue) to implement the producer-consumer model:
import queue import threading q = queue.Queue() def producer(): for i in range(10): q.put(i) def consumer(): while True: item = q.get() if item is None: break print(f'Consumed: {item}') threads = [] threads.append(threading.Thread(target=producer)) threads.append(threading.Thread(target=consumer)) for thread in threads: thread.start() for thread in threads: thread.join()
4. Performance issues
Parallel programming may cause performance issues, For example, the overhead of creating and destroying threads or processes, the overhead of data communication, etc. In order to solve this problem, we can use connection pools to reuse threads or processes to reduce the overhead of creation and destruction; use shared memory or shared files to reduce the overhead of data communication, etc.
The following is a sample code for using the connection pool:
from multiprocessing.pool import ThreadPool def worker(num): # 执行任务 pool = ThreadPool(processes=4) results = [] for i in range(10): result = pool.apply_async(worker, (i,)) results.append(result) for result in results: result.get()
Conclusion:
Through the specific code examples introduced in this article, we have learned about common Python problems and solution strategies in parallel programming. By rationally using technologies such as multi-processing, thread locks, thread-safe data structures, and connection pools, we can better leverage Python's advantages in parallel computing and improve computing efficiency and performance. However, in practical applications, we also need to flexibly apply these strategies according to specific problem scenarios to achieve the best performance and effects.
The above is the detailed content of Python problems encountered in parallel programming and solution strategies. For more information, please follow other related articles on the PHP Chinese website!
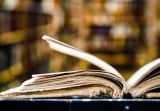
This tutorial demonstrates how to use Python to process the statistical concept of Zipf's law and demonstrates the efficiency of Python's reading and sorting large text files when processing the law. You may be wondering what the term Zipf distribution means. To understand this term, we first need to define Zipf's law. Don't worry, I'll try to simplify the instructions. Zipf's Law Zipf's law simply means: in a large natural language corpus, the most frequently occurring words appear about twice as frequently as the second frequent words, three times as the third frequent words, four times as the fourth frequent words, and so on. Let's look at an example. If you look at the Brown corpus in American English, you will notice that the most frequent word is "th
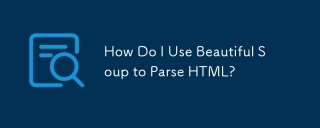
This article explains how to use Beautiful Soup, a Python library, to parse HTML. It details common methods like find(), find_all(), select(), and get_text() for data extraction, handling of diverse HTML structures and errors, and alternatives (Sel
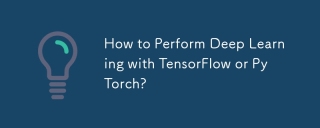
This article compares TensorFlow and PyTorch for deep learning. It details the steps involved: data preparation, model building, training, evaluation, and deployment. Key differences between the frameworks, particularly regarding computational grap
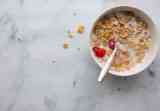
Serialization and deserialization of Python objects are key aspects of any non-trivial program. If you save something to a Python file, you do object serialization and deserialization if you read the configuration file, or if you respond to an HTTP request. In a sense, serialization and deserialization are the most boring things in the world. Who cares about all these formats and protocols? You want to persist or stream some Python objects and retrieve them in full at a later time. This is a great way to see the world on a conceptual level. However, on a practical level, the serialization scheme, format or protocol you choose may determine the speed, security, freedom of maintenance status, and other aspects of the program
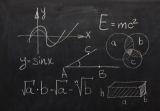
Python's statistics module provides powerful data statistical analysis capabilities to help us quickly understand the overall characteristics of data, such as biostatistics and business analysis. Instead of looking at data points one by one, just look at statistics such as mean or variance to discover trends and features in the original data that may be ignored, and compare large datasets more easily and effectively. This tutorial will explain how to calculate the mean and measure the degree of dispersion of the dataset. Unless otherwise stated, all functions in this module support the calculation of the mean() function instead of simply summing the average. Floating point numbers can also be used. import random import statistics from fracti
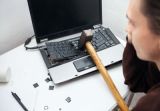
In this tutorial you'll learn how to handle error conditions in Python from a whole system point of view. Error handling is a critical aspect of design, and it crosses from the lowest levels (sometimes the hardware) all the way to the end users. If y
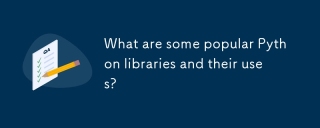
The article discusses popular Python libraries like NumPy, Pandas, Matplotlib, Scikit-learn, TensorFlow, Django, Flask, and Requests, detailing their uses in scientific computing, data analysis, visualization, machine learning, web development, and H
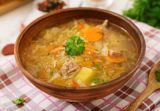
This tutorial builds upon the previous introduction to Beautiful Soup, focusing on DOM manipulation beyond simple tree navigation. We'll explore efficient search methods and techniques for modifying HTML structure. One common DOM search method is ex


Hot AI Tools

Undresser.AI Undress
AI-powered app for creating realistic nude photos

AI Clothes Remover
Online AI tool for removing clothes from photos.

Undress AI Tool
Undress images for free

Clothoff.io
AI clothes remover

AI Hentai Generator
Generate AI Hentai for free.

Hot Article

Hot Tools
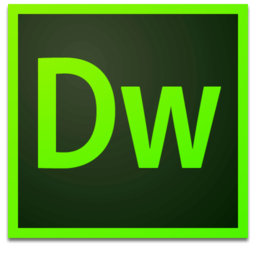
Dreamweaver Mac version
Visual web development tools
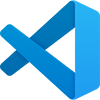
VSCode Windows 64-bit Download
A free and powerful IDE editor launched by Microsoft

MinGW - Minimalist GNU for Windows
This project is in the process of being migrated to osdn.net/projects/mingw, you can continue to follow us there. MinGW: A native Windows port of the GNU Compiler Collection (GCC), freely distributable import libraries and header files for building native Windows applications; includes extensions to the MSVC runtime to support C99 functionality. All MinGW software can run on 64-bit Windows platforms.

PhpStorm Mac version
The latest (2018.2.1) professional PHP integrated development tool

SAP NetWeaver Server Adapter for Eclipse
Integrate Eclipse with SAP NetWeaver application server.
