GMAC stands for "Giga Multiply-Add Operations per Second" and is an indicator used to measure the computational efficiency of deep learning models. This metric represents the computational speed of the model in terms of one billion multiplication and addition operations per second.
The multiply-accumulate (MAC) operation is fundamental in many mathematical calculations, including matrix multiplication, convolution, and other tensor operations commonly used in deep learning. Each MAC operation involves multiplying two numbers and adding the result to an accumulator.
The GMAC indicator can be calculated using the following formula:
<code>GMAC =(乘法累加运算次数)/(10⁹)</code>
The number of multiply-add operations is usually determined by analyzing the network architecture and the dimensions of the model parameters, such as weights and biases.
With the GMAC metric, researchers and practitioners can make informed decisions about model selection, hardware requirements, and optimization strategies for efficient and effective deep learning computations.
GFLOPS is a measure of computing performance of a computer system or a specific operation, representing one billion floating-point operations per second. It is the number of floating point operations per second, expressed in billions (giga).
Floating point arithmetic refers to performing arithmetic calculations on real numbers represented in IEEE 754 floating point format. These operations typically include addition, subtraction, multiplication, division, and other mathematical operations.
GFLOPS is commonly used in high-performance computing (HPC) and benchmarking, especially in areas that require heavy computational tasks, such as scientific simulations, data analysis, and deep learning.
Calculate the GFLOPS formula as follows:
<code>GFLOPS =(浮点运算次数)/(以秒为单位的运行时间)/ (10⁹)</code>
GFLOPS is an effective measure of the computing power of different computer systems, processors, or specific operations. It helps evaluate the speed and efficiency of hardware or algorithms that perform floating point calculations. GFLOPS is a measure of theoretical peak performance and may not reflect the actual performance achieved in real-world scenarios because it does not take into account factors such as memory access, parallelization, and other system limitations.
The relationship between GMAC and GFLOPS
<code>1 GFLOP = 2 GMAC</code>
If we want to calculate these two indicators, it will be more troublesome to write the code manually, but Python already has a ready-made library for us to use:
ptflops library can calculate GMAC and GFLOPs
<code>pip install ptflops</code>
It is also very simple to use:
<code>import torchvision.models as models import torch from ptflops import get_model_complexity_info import re #Model thats already available net = models.densenet161() macs, params = get_model_complexity_info(net, (3, 224, 224), as_strings=True, print_per_layer_stat=True, verbose=True) # Extract the numerical value flops = eval(re.findall(r'([\d.]+)', macs)[0])*2 # Extract the unit flops_unit = re.findall(r'([A-Za-z]+)', macs)[0][0] print('Computational complexity: {:</code>
The results are as follows:
<code>Computational complexity: 7.82 GMac Computational complexity: 15.64 GFlops Number of parameters: 28.68 M</code>
We can customize a model to take a look Is the result correct?
<code>import os import torch from torch import nn class NeuralNetwork(nn.Module): def __init__(self): super().__init__() self.flatten = nn.Flatten() self.linear_relu_stack = nn.Sequential( nn.Linear(28*28, 512), nn.ReLU(), nn.Linear(512, 512), nn.ReLU(), nn.Linear(512, 10),) def forward(self, x): x = self.flatten(x) logits = self.linear_relu_stack(x) return logits custom_net = NeuralNetwork() macs, params = get_model_complexity_info(custom_net, (28, 28), as_strings=True, print_per_layer_stat=True, verbose=True) # Extract the numerical value flops = eval(re.findall(r'([\d.]+)', macs)[0])*2 # Extract the unit flops_unit = re.findall(r'([A-Za-z]+)', macs)[0][0] print('Computational complexity: {:</code>
The result is as follows:
<code>Computational complexity: 670.73 KMac Computational complexity: 1341.46 KFlops Number of parameters: 669.71 k</code>
For the convenience of demonstration, we only write the fully connected layer code to manually calculate GMAC. Iterating over the model weight parameters and calculating the shape of the number of multiplication and addition operations depends on the weight parameters, which is the key to calculating GMAC. The formula for calculating the fully connected layer weight required by GMAC is 2 x (input dimension x output dimension). The total GMAC value is obtained by multiplying and accumulating the shapes of the weight parameters of each linear layer, a process based on the structure of the model.
<code>import torch import torch.nn as nn def compute_gmac(model): gmac_count = 0 for param in model.parameters(): shape = param.shape if len(shape) == 2:# 全连接层的权重 gmac_count += shape[0] * shape[1] * 2 gmac_count = gmac_count / 1e9# 转换为十亿为单位 return gmac_count</code>
According to the model given above, the result of calculating GMAC is as follows:
<code>0.66972288</code>
Since the result of GMAC is in billions, it is not much different from the result we calculated using the class library above . Finally, calculating the GMAC of convolution is a little complicated. The formula is ((input channel x convolution kernel height x convolution kernel width) x output channel) x 2. Here is a simple code, which may not be completely correct. Reference
<code>def compute_gmac(model): gmac_count = 0 for param in model.parameters(): shape = param.shape if len(shape) == 2:# 全连接层的权重 gmac_count += shape[0] * shape[1] * 2 elif len(shape) == 4:# 卷积层的权重 gmac_count += shape[0] * shape[1] * shape[2] * shape[3] * 2 gmac_count = gmac_count / 1e9# 转换为十亿为单位 return gmac_count</code>
The above is the detailed content of A brief analysis of calculating GMAC and GFLOPS. For more information, please follow other related articles on the PHP Chinese website!
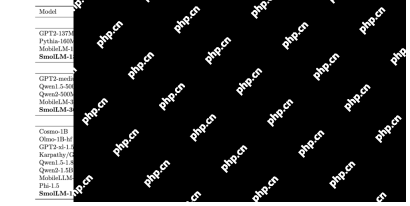
Harness the Power of On-Device AI: Building a Personal Chatbot CLI In the recent past, the concept of a personal AI assistant seemed like science fiction. Imagine Alex, a tech enthusiast, dreaming of a smart, local AI companion—one that doesn't rely
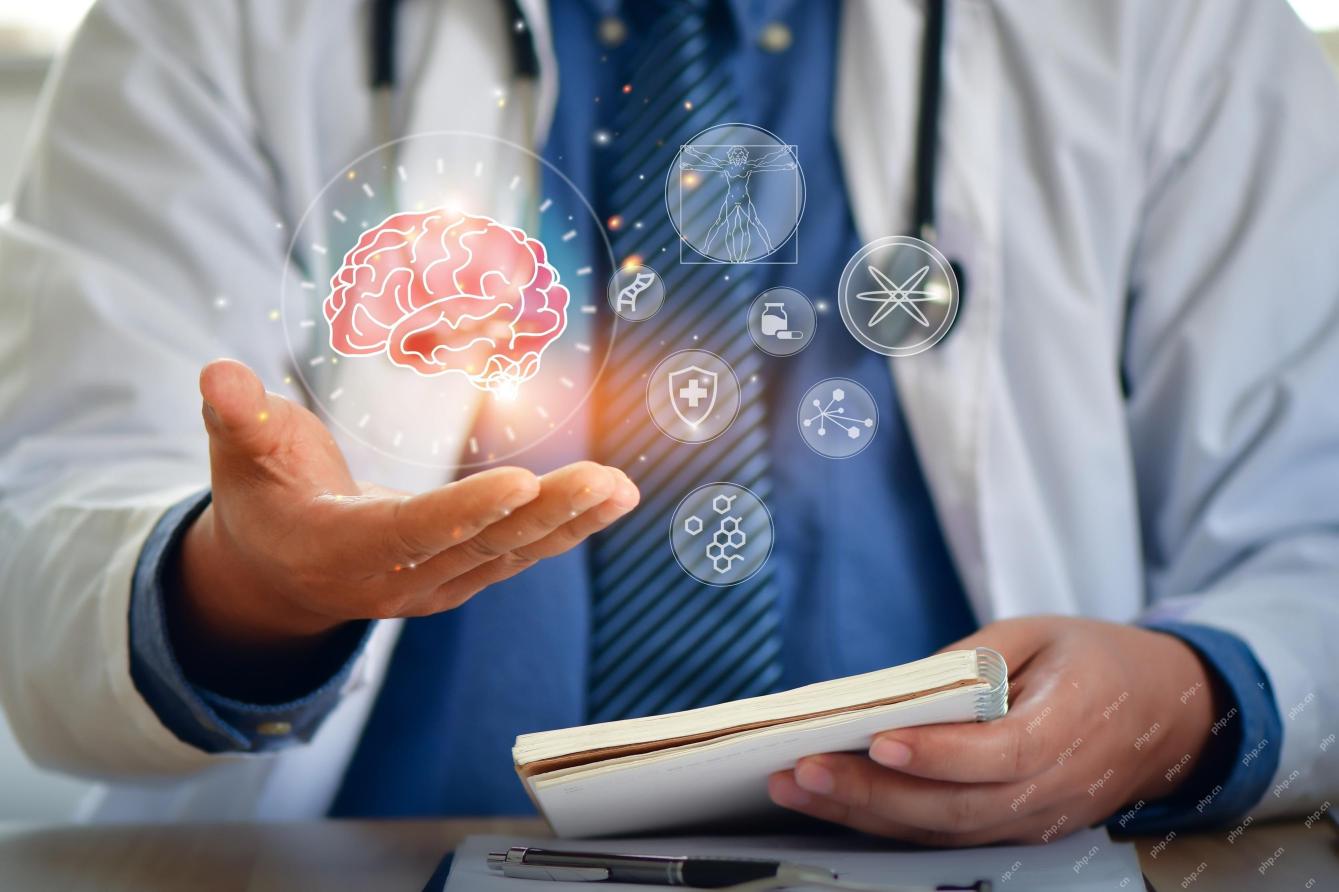
Their inaugural launch of AI4MH took place on April 15, 2025, and luminary Dr. Tom Insel, M.D., famed psychiatrist and neuroscientist, served as the kick-off speaker. Dr. Insel is renowned for his outstanding work in mental health research and techno
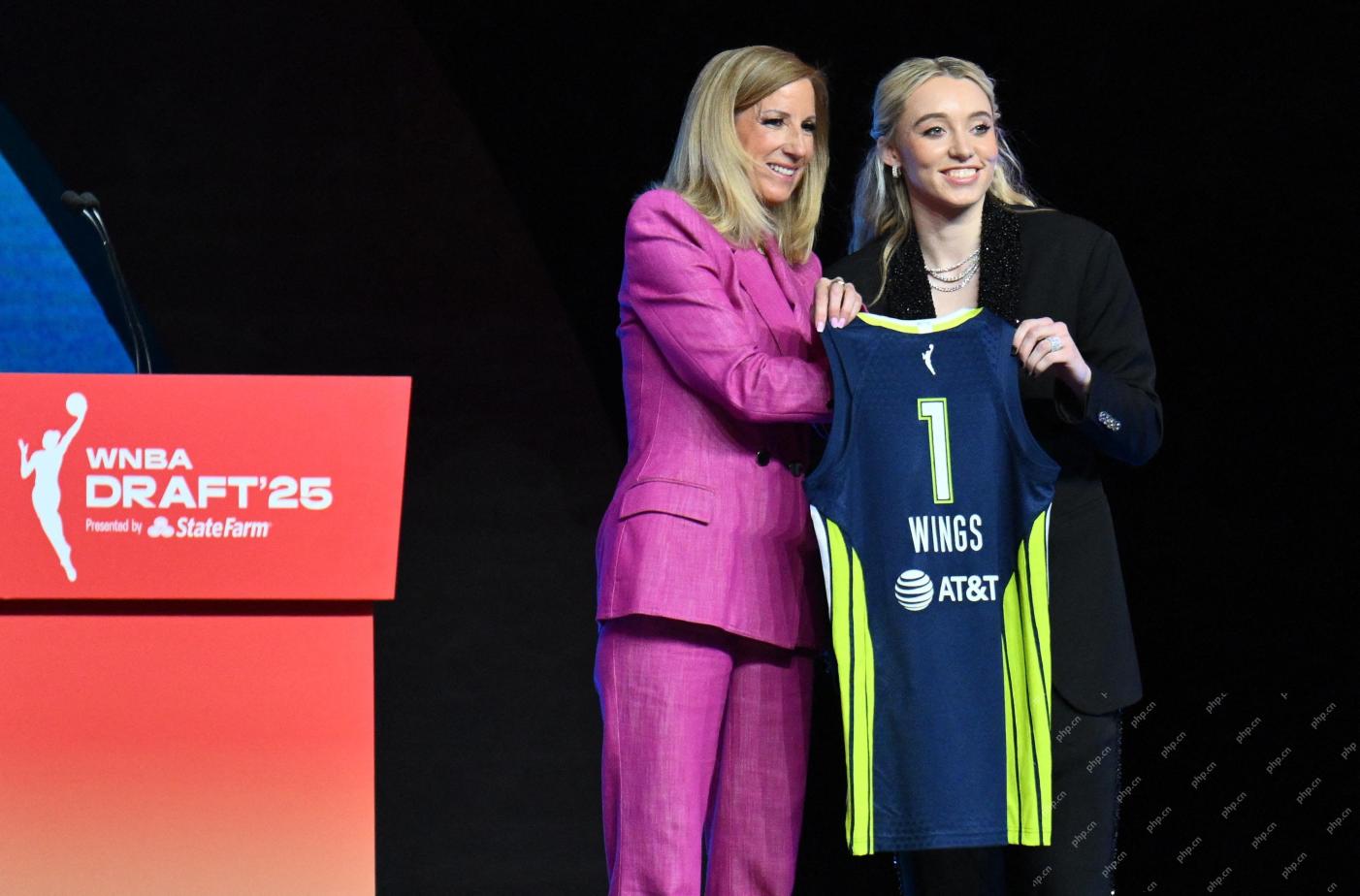
"We want to ensure that the WNBA remains a space where everyone, players, fans and corporate partners, feel safe, valued and empowered," Engelbert stated, addressing what has become one of women's sports' most damaging challenges. The anno
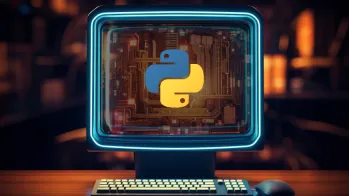
Introduction Python excels as a programming language, particularly in data science and generative AI. Efficient data manipulation (storage, management, and access) is crucial when dealing with large datasets. We've previously covered numbers and st
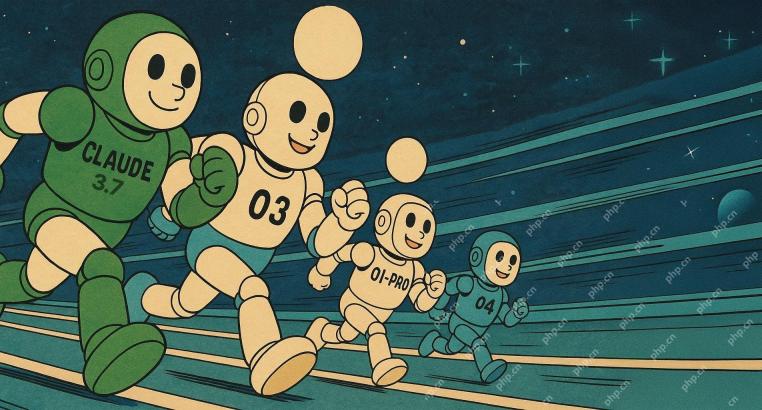
Before diving in, an important caveat: AI performance is non-deterministic and highly use-case specific. In simpler terms, Your Mileage May Vary. Don't take this (or any other) article as the final word—instead, test these models on your own scenario
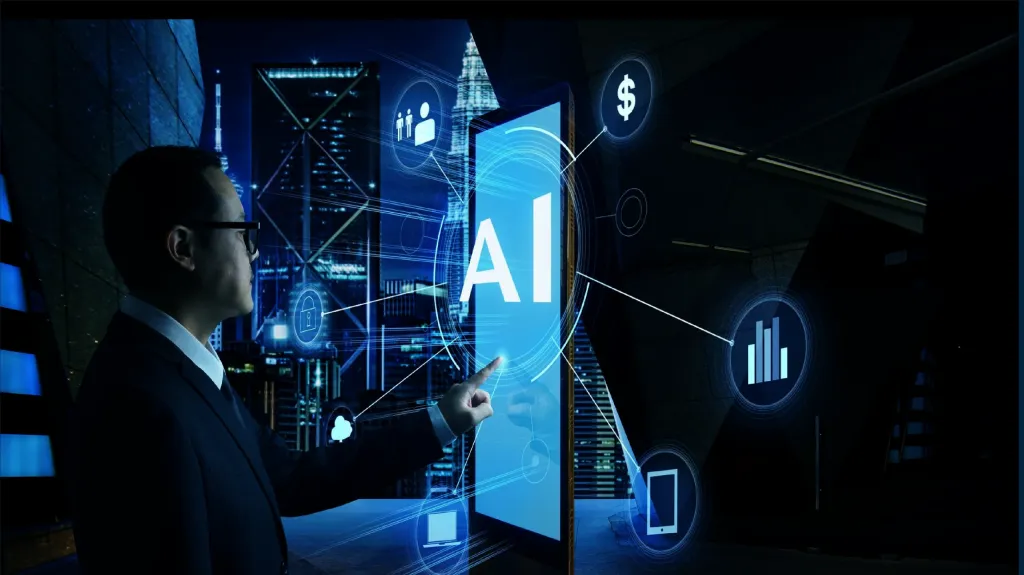
Building a Standout AI/ML Portfolio: A Guide for Beginners and Professionals Creating a compelling portfolio is crucial for securing roles in artificial intelligence (AI) and machine learning (ML). This guide provides advice for building a portfolio
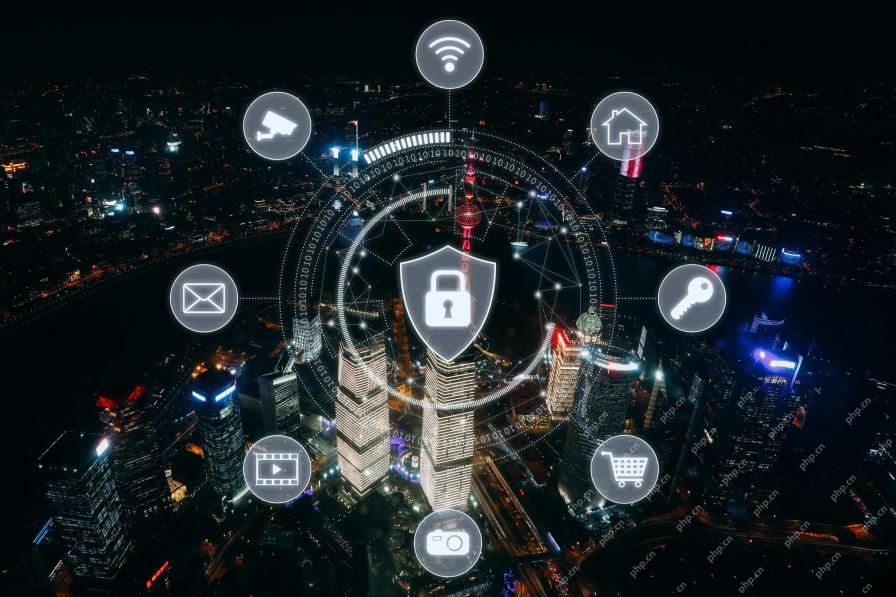
The result? Burnout, inefficiency, and a widening gap between detection and action. None of this should come as a shock to anyone who works in cybersecurity. The promise of agentic AI has emerged as a potential turning point, though. This new class
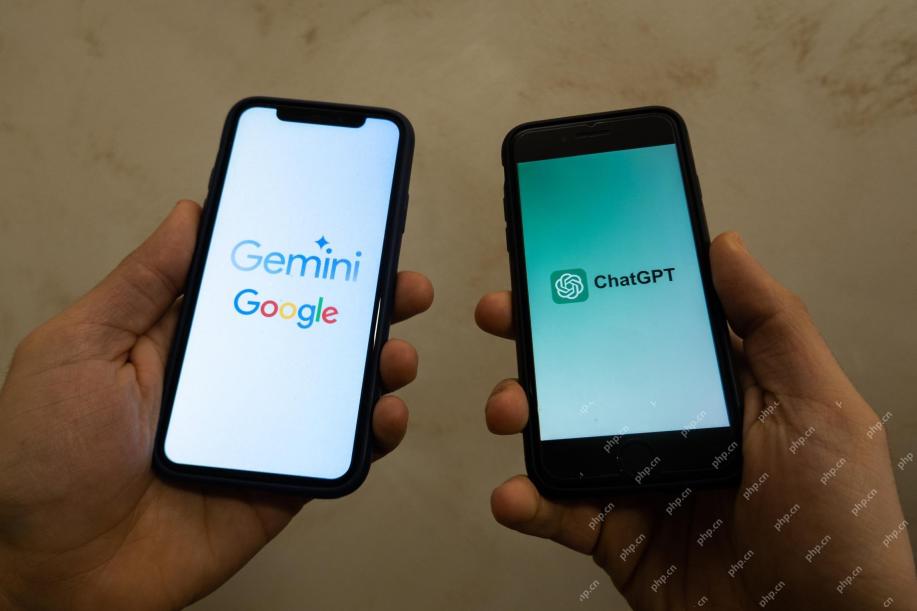
Immediate Impact versus Long-Term Partnership? Two weeks ago OpenAI stepped forward with a powerful short-term offer, granting U.S. and Canadian college students free access to ChatGPT Plus through the end of May 2025. This tool includes GPT‑4o, an a


Hot AI Tools

Undresser.AI Undress
AI-powered app for creating realistic nude photos

AI Clothes Remover
Online AI tool for removing clothes from photos.

Undress AI Tool
Undress images for free

Clothoff.io
AI clothes remover

AI Hentai Generator
Generate AI Hentai for free.

Hot Article

Hot Tools

MinGW - Minimalist GNU for Windows
This project is in the process of being migrated to osdn.net/projects/mingw, you can continue to follow us there. MinGW: A native Windows port of the GNU Compiler Collection (GCC), freely distributable import libraries and header files for building native Windows applications; includes extensions to the MSVC runtime to support C99 functionality. All MinGW software can run on 64-bit Windows platforms.

DVWA
Damn Vulnerable Web App (DVWA) is a PHP/MySQL web application that is very vulnerable. Its main goals are to be an aid for security professionals to test their skills and tools in a legal environment, to help web developers better understand the process of securing web applications, and to help teachers/students teach/learn in a classroom environment Web application security. The goal of DVWA is to practice some of the most common web vulnerabilities through a simple and straightforward interface, with varying degrees of difficulty. Please note that this software

SecLists
SecLists is the ultimate security tester's companion. It is a collection of various types of lists that are frequently used during security assessments, all in one place. SecLists helps make security testing more efficient and productive by conveniently providing all the lists a security tester might need. List types include usernames, passwords, URLs, fuzzing payloads, sensitive data patterns, web shells, and more. The tester can simply pull this repository onto a new test machine and he will have access to every type of list he needs.
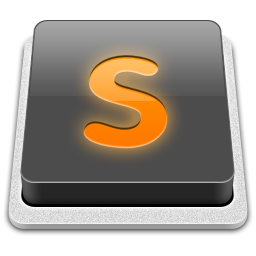
SublimeText3 Mac version
God-level code editing software (SublimeText3)

Notepad++7.3.1
Easy-to-use and free code editor