Use Python to draw cool gif animations that amaze everyone
In a previous article, the editor shared how to use gif## in Python
#Module to makegif
format charts, is awesome, use Python to draw dynamic visualization charts, and save them in gif format. Today I will introduce it to you. A new method of making
format charts, calling the relevant modules of matplotlib
, the steps and methods are also quite simple and easy to understand. .
The data set we used this time is the data set that comes with the
bokehmodule, through The following line of code can directly download <pre class='brush:php;toolbar:false;'>import bokeh
bokeh.sampledata.download()
</pre>
and then import the data set to be used later. We selected the data on the proportion of the population of different age groups in the specified country from 1950 to the present
from bokeh.sampledata.population import data import numpy as np data = filter_loc('United States of America') data.head()
output
##First draw several static charts
We can draw several static charts first, and then draw these Just combine several charts into one animation in the format of It's very simple. We want to filter the data based on the year, and then draw a chart based on the filtered data. The charts are different for each year
import seaborn as sns import matplotlib.pyplot as plt import matplotlib.patheffects as fx # 绘制图表的函数 def make_plot(year): # 根据年份来筛选出数据 df = data[data.Year == year] # 制作图表 fig, (ax1, ax2) = plt.subplots(1, 2, sharey = True) ax1.invert_xaxis() fig.subplots_adjust(wspace = 0) ax1.barh(df[df.Sex == 'Male'].AgeGrp, df[df.Sex == 'Male'].percent, label = 'Male') ax2.barh(df[df.Sex == 'Female'].AgeGrp, df[df.Sex == 'Female'].percent, label = 'Female', color = 'C1') country = df.Location.iloc[0] if country == 'United States of America': country == 'US' fig.suptitle(f'......') fig.supxlabel('......') fig.legend(bbox_to_anchor = (0.9, 0.88), loc = 'upper right') ax1.set_ylabel('Age Groups') return fig
output
gif
format. The code is as followsyears = [i for i in set(data.Year) if i < 2022] years.sort() for year in years: fig = make_plot(year) fig.savefig(f'{year}.jpeg',bbox_inches = 'tight')
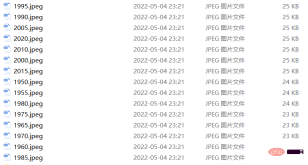
Maybe after seeing this, some people will find the method mentioned above a little troublesome. After all, we Dozens of static charts need to be generated first. If the computer disk space is a little tight, or there is no such place to store these dozens of charts. So you will wonder if it can be done in one step. Of course it is possible. For example, if we plan to draw the distribution of population proportions at different ages from 1950 to 2020, the first step is to draw the distribution of population proportions at different ages in 1950, which is the starting year. Chart, the code is as follows
import matplotlib.animation as animation fig, ax = plt.subplots() ims = [] for year in years: im = ax.imshow(plt.imread(f'{year}.jpeg'), animated = True) ims.append([im]) ani = animation.ArtistAnimation(fig, ims, interval=600) ani.save('us_population.gif')
output
Then we customize a function to draw the chart, where the parameter is the year, the purpose is to filter out the phase by year Corresponding data and draw corresponding chartsdef run(year): # 通过年份来筛选出数据 df = data[data.Year == year] # 针对不同地性别来绘制 total_pop = df.Value.sum() df['percent'] = df.Value / total_pop * 100 male.remove() y_pos = [i for i in range(len(df[df.Sex == 'Male']))] male.patches = ax1.barh(y_pos, df[df.Sex == 'Male'].percent, label = 'Male', color = 'C0', tick_label = df[df.Sex == 'Male'].AgeGrp) female.remove() female.patches = ax2.barh(y_pos, df[df.Sex == 'Female'].percent, label = 'Female', color = 'C1', tick_label = df[df.Sex == 'Female'].AgeGrp) text.set_text(year) return male#, female
然后我们调用animation.FuncAnimation()
方法,
ani = animation.FuncAnimation(fig, run, years, blit = True, repeat = True, interval = 600) ani.save('文件名.gif')
output
这样就可以一步到位生成gif
格式的图表,避免生成数十张繁多地静态图片了。
将若干张<span style="color: #2b2b2b;">gif</span>
动图放置在一张大图当中
最后我们可以将若干张gif
动图放置在一张大的图表当中,代码如下
import matplotlib.animation as animation # 创建一个新的画布 fig, (ax, ax2, ax3) = plt.subplots(1, 3, figsize = (10, 3)) ims = [] for year in years: im = ax.imshow(plt.imread(f'文件1{year}.jpeg'), animated = True) im2 = ax2.imshow(plt.imread(f'文件2{year}.jpeg'), animated = True) im3 = ax3.imshow(plt.imread(f'文件3{year}.jpeg'), animated = True) ims.append([im, im2, im3]) ani = animation.ArtistAnimation(fig, ims, interval=600) ani.save('comparison.gif')
output
The above is the detailed content of Use Python to draw cool gif animations that amaze everyone. For more information, please follow other related articles on the PHP Chinese website!
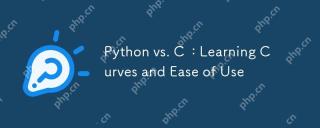
Python is easier to learn and use, while C is more powerful but complex. 1. Python syntax is concise and suitable for beginners. Dynamic typing and automatic memory management make it easy to use, but may cause runtime errors. 2.C provides low-level control and advanced features, suitable for high-performance applications, but has a high learning threshold and requires manual memory and type safety management.
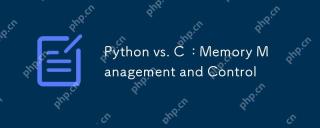
Python and C have significant differences in memory management and control. 1. Python uses automatic memory management, based on reference counting and garbage collection, simplifying the work of programmers. 2.C requires manual management of memory, providing more control but increasing complexity and error risk. Which language to choose should be based on project requirements and team technology stack.
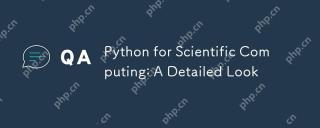
Python's applications in scientific computing include data analysis, machine learning, numerical simulation and visualization. 1.Numpy provides efficient multi-dimensional arrays and mathematical functions. 2. SciPy extends Numpy functionality and provides optimization and linear algebra tools. 3. Pandas is used for data processing and analysis. 4.Matplotlib is used to generate various graphs and visual results.
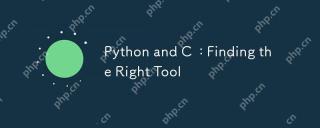
Whether to choose Python or C depends on project requirements: 1) Python is suitable for rapid development, data science, and scripting because of its concise syntax and rich libraries; 2) C is suitable for scenarios that require high performance and underlying control, such as system programming and game development, because of its compilation and manual memory management.
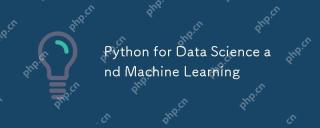
Python is widely used in data science and machine learning, mainly relying on its simplicity and a powerful library ecosystem. 1) Pandas is used for data processing and analysis, 2) Numpy provides efficient numerical calculations, and 3) Scikit-learn is used for machine learning model construction and optimization, these libraries make Python an ideal tool for data science and machine learning.
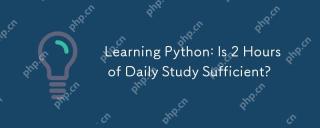
Is it enough to learn Python for two hours a day? It depends on your goals and learning methods. 1) Develop a clear learning plan, 2) Select appropriate learning resources and methods, 3) Practice and review and consolidate hands-on practice and review and consolidate, and you can gradually master the basic knowledge and advanced functions of Python during this period.
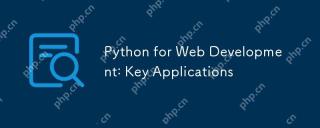
Key applications of Python in web development include the use of Django and Flask frameworks, API development, data analysis and visualization, machine learning and AI, and performance optimization. 1. Django and Flask framework: Django is suitable for rapid development of complex applications, and Flask is suitable for small or highly customized projects. 2. API development: Use Flask or DjangoRESTFramework to build RESTfulAPI. 3. Data analysis and visualization: Use Python to process data and display it through the web interface. 4. Machine Learning and AI: Python is used to build intelligent web applications. 5. Performance optimization: optimized through asynchronous programming, caching and code
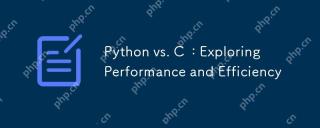
Python is better than C in development efficiency, but C is higher in execution performance. 1. Python's concise syntax and rich libraries improve development efficiency. 2.C's compilation-type characteristics and hardware control improve execution performance. When making a choice, you need to weigh the development speed and execution efficiency based on project needs.


Hot AI Tools

Undresser.AI Undress
AI-powered app for creating realistic nude photos

AI Clothes Remover
Online AI tool for removing clothes from photos.

Undress AI Tool
Undress images for free

Clothoff.io
AI clothes remover

Video Face Swap
Swap faces in any video effortlessly with our completely free AI face swap tool!

Hot Article

Hot Tools

Atom editor mac version download
The most popular open source editor

SublimeText3 Linux new version
SublimeText3 Linux latest version
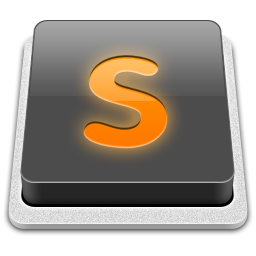
SublimeText3 Mac version
God-level code editing software (SublimeText3)

SublimeText3 English version
Recommended: Win version, supports code prompts!

SAP NetWeaver Server Adapter for Eclipse
Integrate Eclipse with SAP NetWeaver application server.