


Do you need to ask about language models when dating your boyfriend? Nature: Propose ideas and summarize notes. GPT-3 has become a contemporary 'scientific research worker'
Let a monkey press the keys randomly on a typewriter. As long as you give it long enough, it can type out the complete works of Shakespeare.
What if it was a monkey that understood grammar and semantics? The answer is that even scientific research can be done for you!
The development momentum of language models is very rapid. A few years ago, it could only automatically complete the next word to be input on the input method. Today, it can already help researchers analyze and writing scientific papers and generating code.
The training of large language models (LLM) generally requires massive text data for support.
In 2020, OpenAI released the GPT-3 model with 175 billion parameters. It can write poems, do math problems, and almost everything the generative model can do. GPT-3 has already achieved the ultimate. Even today, GPT-3 is still the baseline for many language models to be compared and surpassed.
After the release of GPT-3, it quickly sparked heated discussions on Twitter and other social media. A large number of researchers were surprised by this weird "human-like writing" method.
After GPT-3 releases online services, users can enter text at will and let the model return the following. The minimum charge per 750 words processed is only $0.0004, which is very affordable. .
Recently, an article was published in the Nature column on science and technology. Unexpectedly, in addition to helping you write essays, these language models can also help you "do scientific research"!
Let the machine think for you
Hafsteinn Einarsson, a computer scientist at the University of Iceland in Reykjavik, said: I use it almost every day GPT-3, such as revising the abstract of a paper.
When Einarsson was preparing the copy at a meeting in June, although GPT-3 made many useless modification suggestions, there were also some helpful ones, such as "Make the research question in the abstract" "The beginning of the manuscript is more clear", and you won't realize this kind of problem when you read the manuscript yourself, unless you ask someone else to read it for you. And why can't this other person be "GPT-3"?
Language models can even help you improve your experimental design!
In another project, Einarsson wanted to use the Pictionary game to collect language data among participants.
After giving a description of the game, GPT-3 gave some suggestions for game modifications. In theory, researchers could also request new attempts at experimental protocols.
#Some researchers also use language models to generate paper titles or make text more readable.
The method used by Mina Lee, a doctoral student who is a professor of computer science at Stanford University, is to enter "Use these keywords to generate a paper title" into GPT-3 as a prompt, and the model will We will help you come up with several titles.
If some chapters need to be rewritten, she will also use Wordtune, an artificial intelligence writing assistant released by the AI21 laboratory in Tel Aviv, Israel. She only needs to click "Rewrite" to convert Just rewrite passages in multiple versions and then choose carefully.
Lee will also ask GPT-3 to provide advice on some things in life. For example, when asking "How to introduce her boyfriend to her parents," GPT-3 suggested going to the beach. A restaurant.
Domenic Rosati, a computer scientist at Scite, a technology startup located in Brooklyn, New York, used the Generate language model to reorganize his ideas.
Link: https://cohere.ai/generate
Generate is run by a Canadian NLP Developed by the company Cohere, the workflow of the model is very similar to GPT-3.
You only need to enter notes, or just talk about some ideas, and finally add "summarize" or "turn it into an abstract concept", and the model will automatically organize it for you. ideas.
Why write the code yourself?
OpenAI researchers trained GPT-3 on a large set of text, including books, news stories, Wikipedia entries, and software code.
Later, the team noticed that GPT-3 could complete code just like normal text.
The researchers created a fine-tuned version of the algorithm called Codex, trained on more than 150G of text from the code sharing platform GitHub; GitHub has now integrated Codex into Copilot In the service, users can be assisted in writing code.
Luca Soldaini, a computer scientist at AI2 at the Allen Institute for Artificial Intelligence in Seattle, Washington, says at least half the people in their office are using Copilot
Soldaini said that Copilot is most suitable for repetitive programming scenarios. For example, one of his projects involved writing template code for processing PDFs, and Copilot directly completed it.
However, Copilot's completion content often makes mistakes, so it is best to use it in languages you are familiar with.
Literature retrieval
Perhaps the most mature application scenario of language models is searching and summarizing documents.
The Semantic Scholar search engine developed by AI2 uses the TLDR language model to give a Twitter-like description of each paper.
The search engine covers approximately 200 million papers, mostly from biomedicine and computer science.
TLDR was developed based on the BART model released earlier by Meta, and then AI2 researchers fine-tuned the model based on human-written summaries.
By today's standards, TLDR is not a large language model, as it only contains about 400 million parameters, while the largest version of GPT-3 contains 175 billion.
TLDR is also used in Semantic Reader, an extended scientific paper application developed by AI2.
When users use in-text citations in Semantic Reader, an information box containing a summary of the TLDR will pop up.
Dan Weld, chief scientist of Semantic Scholar, said that the idea is to use language models to improve the reading experience.
When a language model generates a text summary, the model may generate some facts that do not exist in the article. Researchers call this problem "illusion," but in fact language The model is simply making it up or lying.
TLDR performed well in the authenticity test. The author of the paper rated TLDR’s accuracy as 2.5 points (out of 3 points).
Weld said TLDR is more realistic because the summary is only about 20 words long, and possibly because the algorithm doesn't put words into the summary that don't appear in the text.
In terms of search tools, Ought, a machine learning nonprofit in San Francisco, California, launched Elicit in 2021. If users ask it, “What is the impact of mindfulness on decision-making?” it will Output a table containing ten papers.
Users can ask the software to fill in columns with things like summaries and metadata, as well as information about study participants, methods and results information, which are then extracted or generated from the paper using tools including GPT-3.
Joel Chan of the University of Maryland, College Park, studies human-computer interaction. Whenever he starts a new project, he uses Elicit to search for relevant papers.
Gustav Nilsonne, a neuroscientist at the Karolinska Institute in Stockholm, also used Elicit to find papers with data that could be added to the pooled analysis, using the tool to find papers not found in other searches. Found files.
Evolving Model
AI2’s prototype gives LLM a futuristic feel.
Sometimes researchers have questions after reading the abstract of a scientific paper but have not yet had time to read the full text.
A team at AI2 has also developed a tool that can answer these questions in the field of NLP.
The model first requires researchers to read the abstract of an NLP paper and then asks relevant questions (such as "What five conversational attributes were analyzed?")
The research team then asked other researchers to answer these questions after reading the entire paper.
AI2 trained another version of the Longformer language model as input as a complete paper, and then used the collected The dataset generates answers to different questions about other papers.
The ACCoRD model can generate definitions and analogies for 150 scientific concepts related to NLP.
MS2 is a dataset containing 470,000 medical documents and 20,000 multi-document summaries. After fine-tuning BART with MS2, researchers were able to pose a question and a set of documents and generate A brief meta-analysis summary.
In 2019, AI2 fine-tuned the language model BERT created by Google in 2018 and created SciBERT with 110 million parameters based on Semantic Scholar's paper
Scite used artificial intelligence to create a scientific search engine, further fine-tuning SciBERT so that when its search engine lists papers that cite a target paper, it categorizes those papers as supporting, contrasting, or otherwise way to refer to the paper.
Rosati said this nuance helps people identify limitations or gaps in the scientific literature.
AI2’s SPECTER model is also based on SciBERT, which simplifies papers into compact mathematical representations.
Conference organizers use SPECTER to match submitted papers to peer reviewers, and Semantic Scholar uses it to recommend papers based on users’ libraries, Weld said.
Tom Hope, a computer scientist at Hebrew University and AI2, said they have research projects fine-tuning language models to identify effective drug combinations, links between genes and diseases, and scientific challenges in COVID-19 research and direction.
But, can language models provide deeper insights and even discovery capabilities?
In May, Hope and Weld co-authored a commentary with Microsoft Chief Science Officer Eric Horvitz outlining the challenges of achieving this goal, including teaching models to "infer ) is the result of reorganizing two concepts."
Hope said that this is basically the same thing as OpenAI's DALL · E 2 image generation model "generating a picture of a cat flying into space", but how can we move towards combined abstraction What about highly complex scientific concepts?
This is an open question.
To this day, large language models have had a real impact on research, and if people haven't started using these large language models to assist their work, they will miss these opportunities.
Reference materials:
https://www.nature.com/articles/d41586-022-03479-w
The above is the detailed content of Do you need to ask about language models when dating your boyfriend? Nature: Propose ideas and summarize notes. GPT-3 has become a contemporary 'scientific research worker'. For more information, please follow other related articles on the PHP Chinese website!
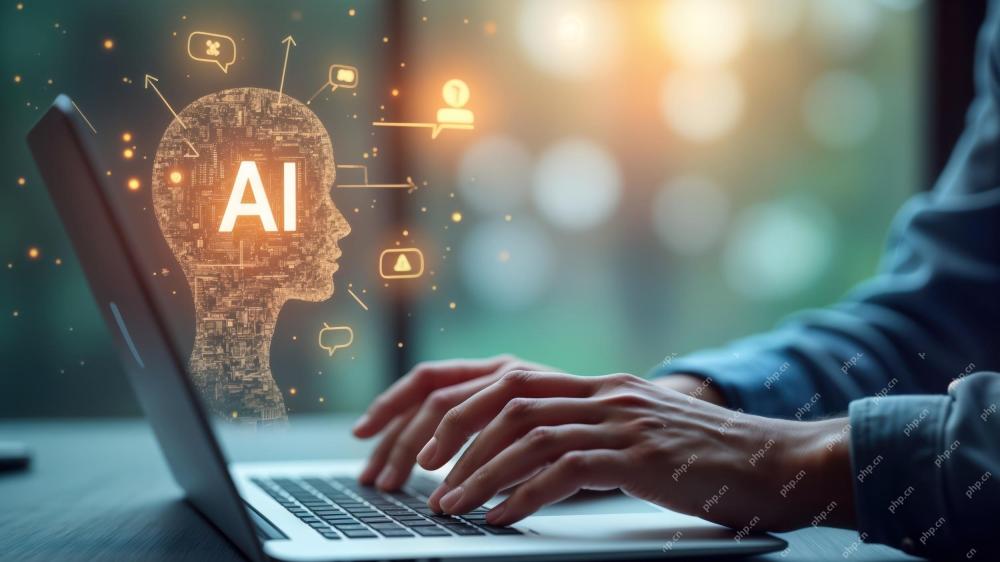
Generative AI, exemplified by chatbots like ChatGPT, offers project managers powerful tools to streamline workflows and ensure projects stay on schedule and within budget. However, effective use hinges on crafting the right prompts. Precise, detail
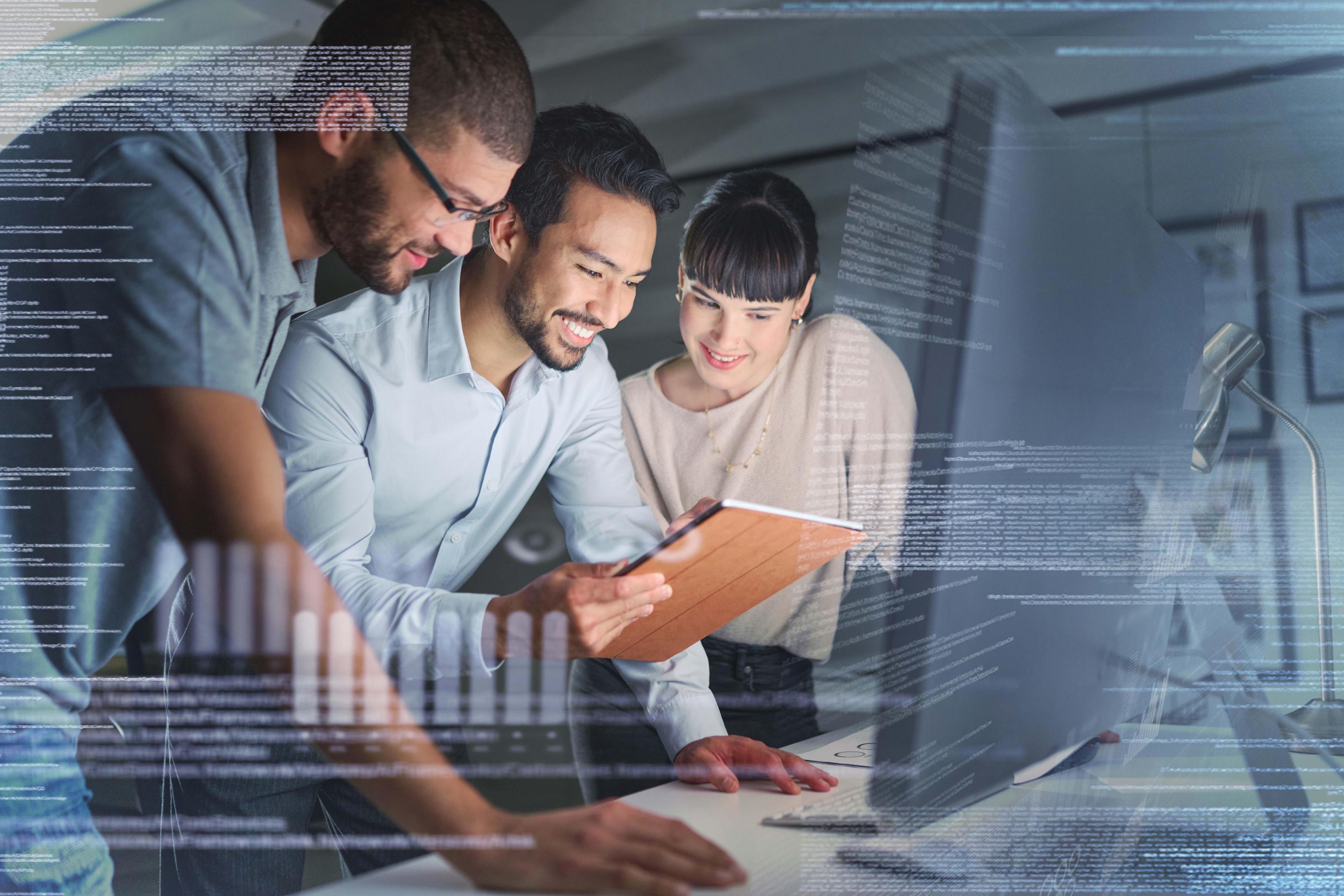
The challenge of defining Artificial General Intelligence (AGI) is significant. Claims of AGI progress often lack a clear benchmark, with definitions tailored to fit pre-determined research directions. This article explores a novel approach to defin
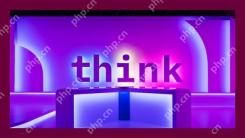
IBM Watsonx.data: Streamlining the Enterprise AI Data Stack IBM positions watsonx.data as a pivotal platform for enterprises aiming to accelerate the delivery of precise and scalable generative AI solutions. This is achieved by simplifying the compl
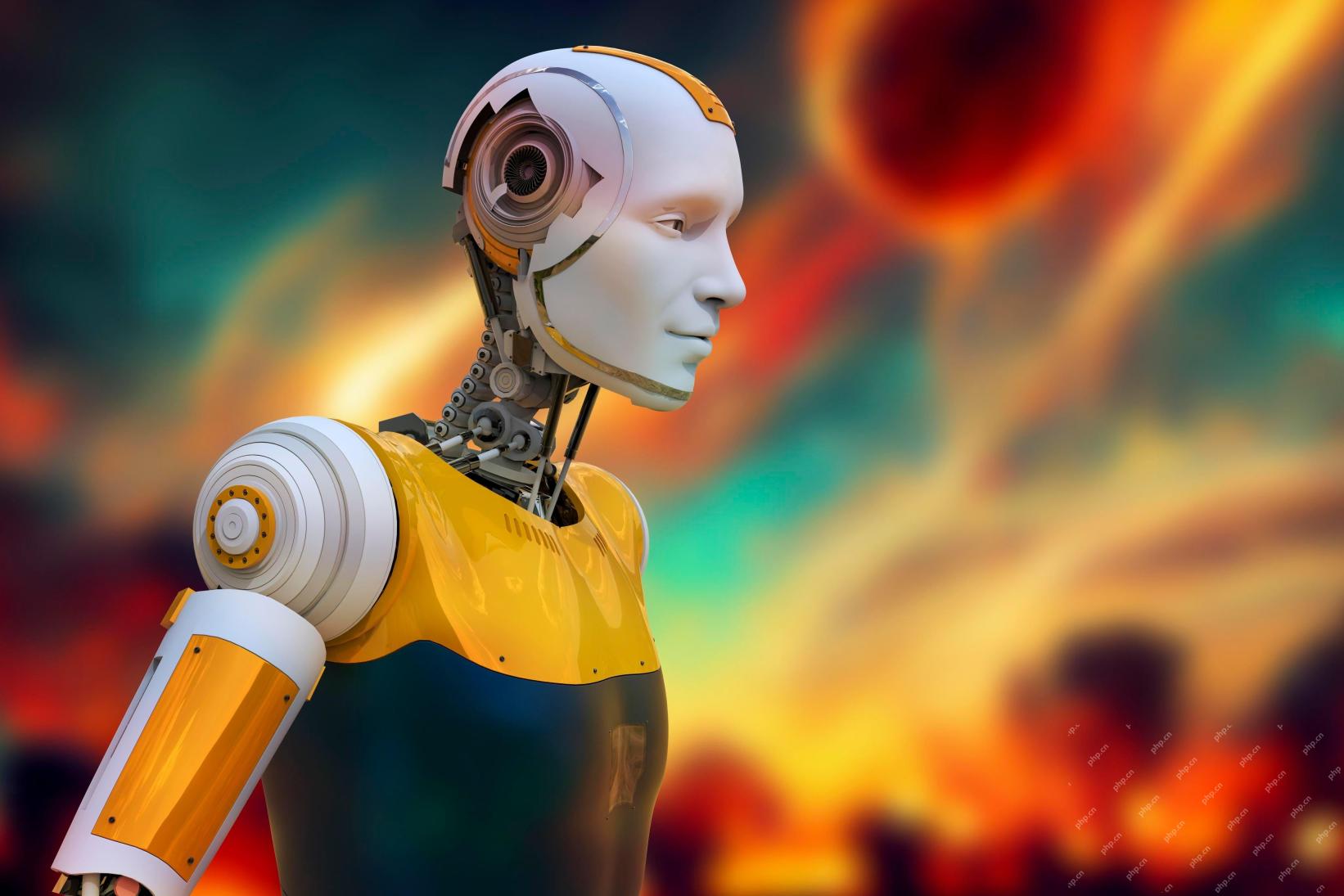
The rapid advancements in robotics, fueled by breakthroughs in AI and materials science, are poised to usher in a new era of humanoid robots. For years, industrial automation has been the primary focus, but the capabilities of robots are rapidly exp
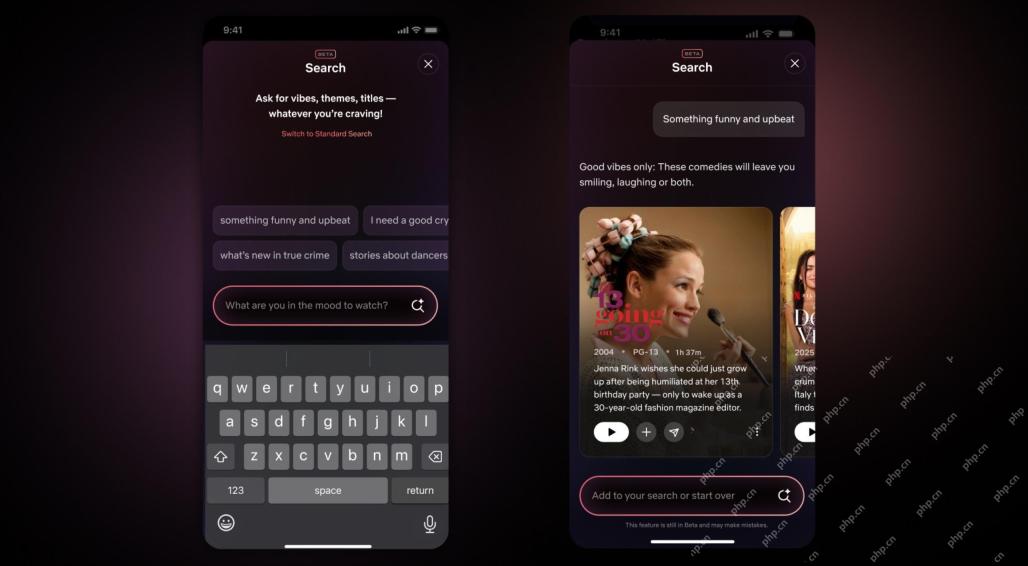
The biggest update of Netflix interface in a decade: smarter, more personalized, embracing diverse content Netflix announced its largest revamp of its user interface in a decade, not only a new look, but also adds more information about each show, and introduces smarter AI search tools that can understand vague concepts such as "ambient" and more flexible structures to better demonstrate the company's interest in emerging video games, live events, sports events and other new types of content. To keep up with the trend, the new vertical video component on mobile will make it easier for fans to scroll through trailers and clips, watch the full show or share content with others. This reminds you of the infinite scrolling and very successful short video website Ti
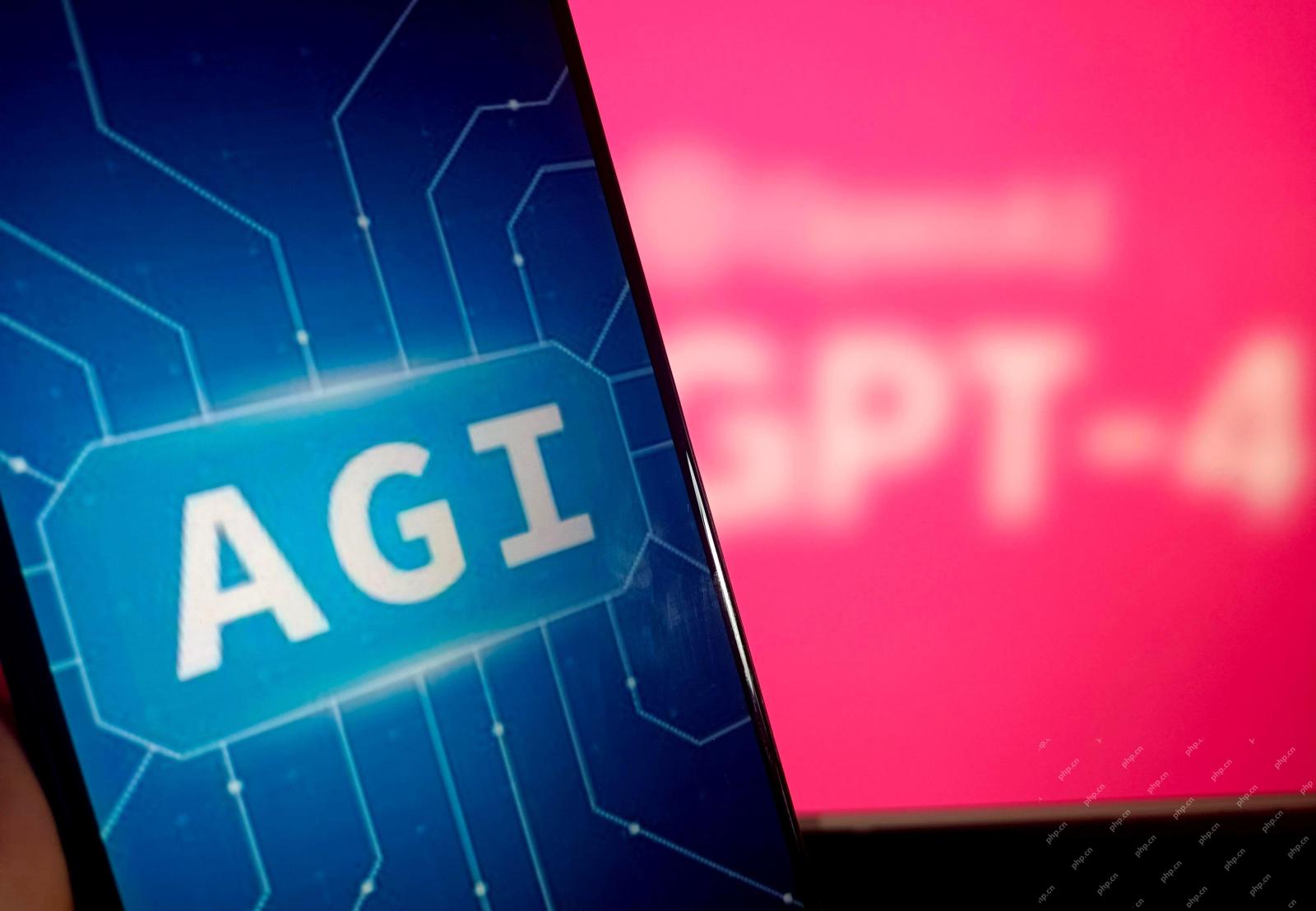
The growing discussion of general intelligence (AGI) in artificial intelligence has prompted many to think about what happens when artificial intelligence surpasses human intelligence. Whether this moment is close or far away depends on who you ask, but I don’t think it’s the most important milestone we should focus on. Which earlier AI milestones will affect everyone? What milestones have been achieved? Here are three things I think have happened. Artificial intelligence surpasses human weaknesses In the 2022 movie "Social Dilemma", Tristan Harris of the Center for Humane Technology pointed out that artificial intelligence has surpassed human weaknesses. What does this mean? This means that artificial intelligence has been able to use humans
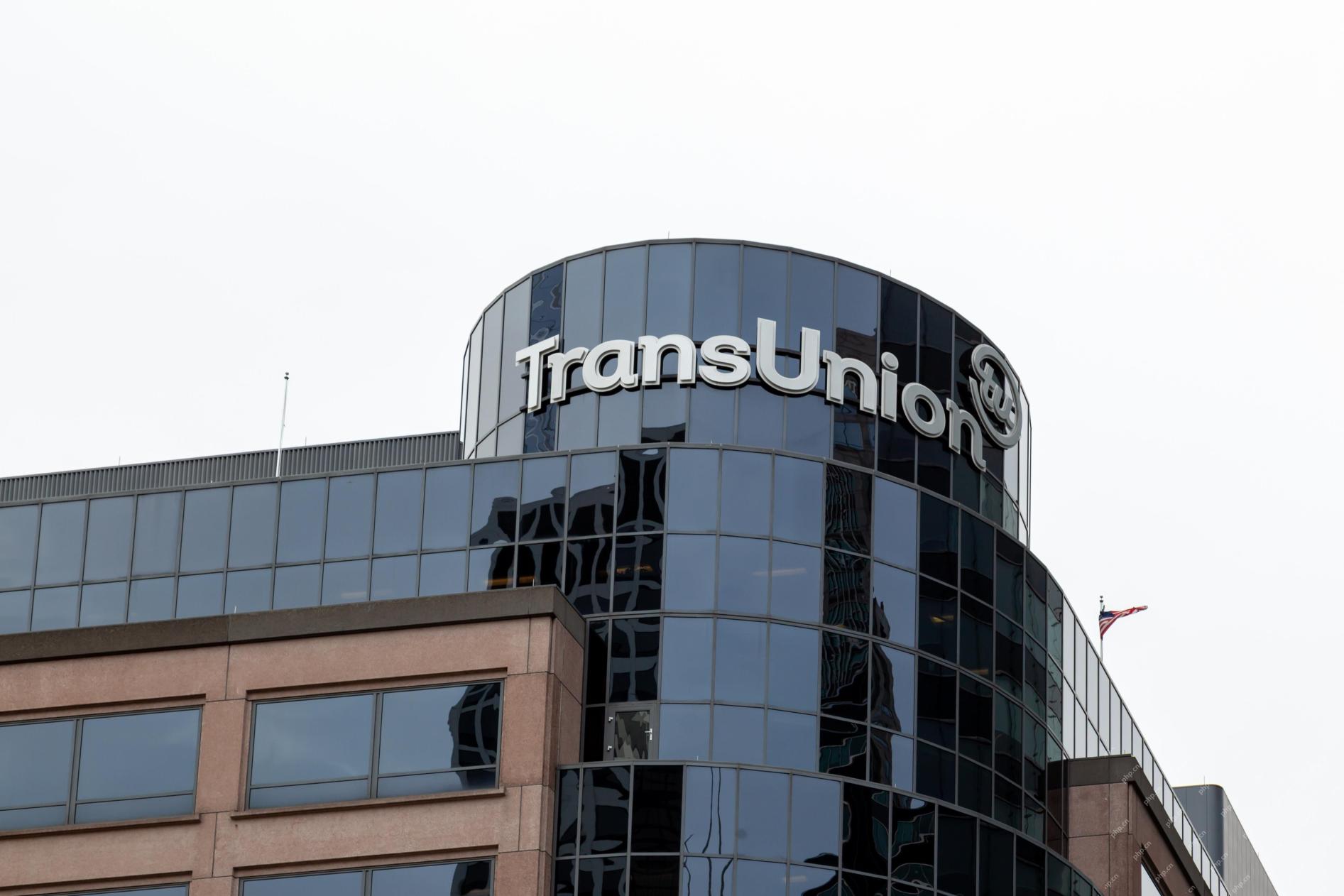
TransUnion's CTO, Ranganath Achanta, spearheaded a significant technological transformation since joining the company following its Neustar acquisition in late 2021. His leadership of over 7,000 associates across various departments has focused on u
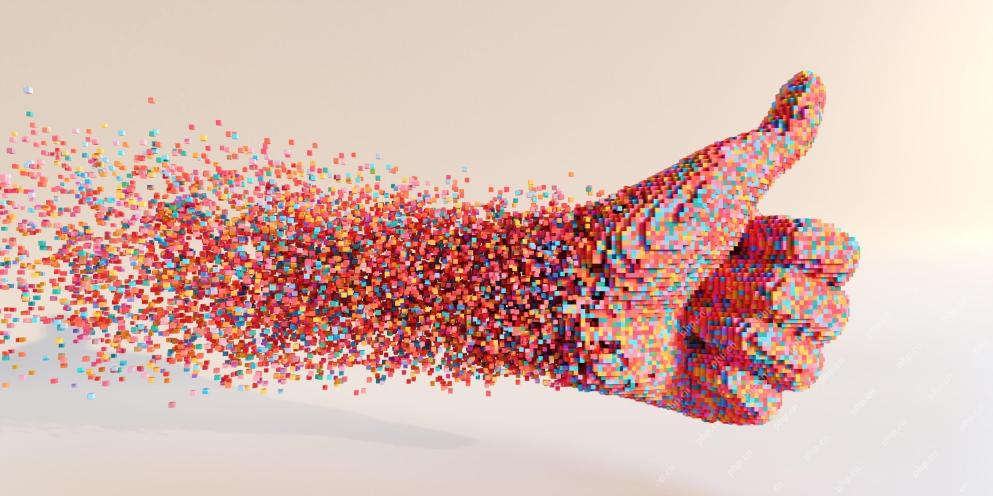
Building trust is paramount for successful AI adoption in business. This is especially true given the human element within business processes. Employees, like anyone else, harbor concerns about AI and its implementation. Deloitte researchers are sc


Hot AI Tools

Undresser.AI Undress
AI-powered app for creating realistic nude photos

AI Clothes Remover
Online AI tool for removing clothes from photos.

Undress AI Tool
Undress images for free

Clothoff.io
AI clothes remover

Video Face Swap
Swap faces in any video effortlessly with our completely free AI face swap tool!

Hot Article

Hot Tools
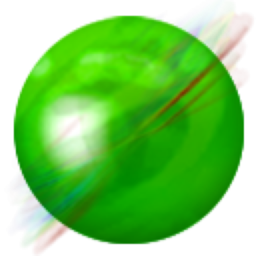
ZendStudio 13.5.1 Mac
Powerful PHP integrated development environment
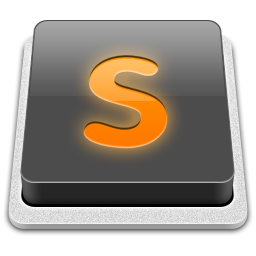
SublimeText3 Mac version
God-level code editing software (SublimeText3)
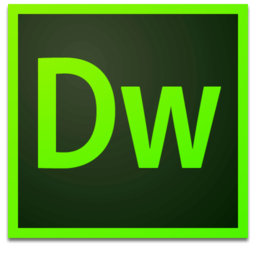
Dreamweaver Mac version
Visual web development tools
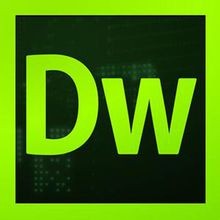
Dreamweaver CS6
Visual web development tools

Safe Exam Browser
Safe Exam Browser is a secure browser environment for taking online exams securely. This software turns any computer into a secure workstation. It controls access to any utility and prevents students from using unauthorized resources.
