


Translator | Zhu Xianzhong
Reviewer | Sun Shujuan
Introduction
- Testing AI requires smart testing processes, virtualized cloud resources, specialized skills, and AI tools.
- Although artificial intelligence platform providers will release various versions frequently, the speed of testing should be as fast as possible.
- Artificial intelligence products often lack transparency and cannot be explained; therefore, they are difficult to convince.
- Not only artificial intelligence products, the quality of the training model and the quality of the data are also equally important. However, some traditional testing methods for validating cloud resources, algorithms, interfaces, and user configurations are generally inefficient. In this way, tests of learning, reasoning, perception, operation, etc. become equally important.
- Migrate automated test scripts into enterprise version control tools. The automation codebase, just like the application codebase, should reside in a version control repository. In this way, it will be efficient to combine test assets with application and data assets.
- Plans to integrate the automation suite with code/data build deployment tools to support centralized execution and reporting. It is important to align code/data builds with their respective automation suites. Of course, tool-based automated deployment is absolutely necessary during every build to avoid human intervention.
- Divide the automation suite into multiple test layers to achieve faster feedback at each checkpoint. For example, AI health checks can verify that services are functioning properly after changes are deployed in interfaces and data structures. AI smoke testing can verify that critical system functions are functioning properly and are free of clogging defects.
- The test range should also cover the training model. AI testing should also test trained models that demonstrate whether the solution learns given instructions, both supervised and unsupervised. It is crucial to reproduce the same scenario multiple times to check whether the response matches the given training. Likewise, it is crucial to have a process in place to train solutions for failures, exceptions, errors, etc. as part of testing. If exception handling is carefully considered, fault tolerance can be built in.
- Plan to manage artificial intelligence training/learning throughout the entire AI solution cycle. CT-related settings should help continue learning from test to production, reducing concerns about transfer learning.
- Optimization through intelligent regression. If the execution cycle time of the ensemble regression is significantly longer, CT should partition a subset at runtime based on severely affected areas to provide feedback within a reasonable time window. Effectively use ML algorithms to create probabilistic models to select regression tests that are consistent with specific code and data builds, helping to efficiently optimize the use of cloud resources and speed up testing.
- Always schedule comprehensive regression testing on a regular basis. This work can be scheduled for nights or weekends, depending on its consistency with the recurring build frequency. This is the ultimate feedback from the CT ecosystem, whose goal is to minimize feedback time by running threads or machines that execute in parallel.
When testing occurs without human intervention, glitches, errors, and any algorithm anomalies become a source of discovery for AI solutions. Likewise, actual usage and user preferences during testing also become a source of training and should continue in production.
Ensuring that data in AIaaS solutions can be extracted
Data quality is the most important success criterion in artificial intelligence solutions. Useful data exists both within and outside the enterprise. The ability to extract useful data and feed it to the AI engine is one of the requirements for quality development. Extract, Transform, and Load (ETL) is a traditional term that refers to a data pipeline that collects data from various sources, transforms it based on business rules, and loads it into a target data store. The ETL field has developed into enterprise information integration (EII), enterprise application integration (EAI) and enterprise cloud integration platform as a service (iPaaS). Regardless of technological advancements, the need for data assurance will only become more important. Data assurance should address functional testing activities such as Map Reduce process verification, transformation logic verification, data verification, data storage verification, etc. In addition, data assurance should also address non-functional aspects of performance, failover, and data security.
Structured data is easier to manage, while unstructured data originating from outside the enterprise should be handled with caution. Stream processing principles help prepare data in motion early; that is, through event-driven processing, data is processed as soon as it is generated or received from websites, external applications, mobile devices, sensors, and other sources. deal with. In addition, it is absolutely necessary to check the quality by establishing quality gates.
Messaging platforms such as Twitter, Instagram, and WhatsApp are popular sources of data. When using such data, they connect applications, services, and devices across various technologies through a cloud-based messaging framework. Deep learning techniques allow computers to learn from these data loads. Some of this data requires the help of neural network solutions to solve complex signal processing and pattern recognition problems, ranging from speech to text transcription, handwriting recognition to facial recognition and many other areas. Therefore, necessary quality gates should be established to test data from these platforms.
The following are some things you should pay attention to when designing an artificial intelligence-driven QA project.
- Automated quality gates: ML algorithms can be implemented to determine whether data "passes" based on historical and perceptual criteria.
- Predict source causes: Classifying or identifying the source causes of data defects not only helps avoid future errors, but also helps continuously improve data quality. Through patterns and correlations, testing teams can implement ML algorithms to trace defects back to their source. This helps automate remedial testing and remediation before the data moves to the next stage for self-testing and self-healing.
- Leverage pre-aware monitoring: ML algorithms can search for symptoms and related coding errors in data patterns, such as high memory usage, potential threats that may cause outages, etc., thus helping teams to automatically implement corrective steps. For example, the AI engine can automatically accelerate parallel processes to optimize server consumption.
- Failover: ML algorithms can detect failures and automatically recover to continue processing, and can register failures for learning.
Ensuring artificial intelligence algorithms in AIaaS solutions
When the internal structure of the software system is known, development testing is simple. However, in AI platform solutions, AI and ML are less "explainable", i.e. the input/output mapping is the only known element, and developers often cannot see or understand the mechanics of the underlying AI functionality (e.g. prediction). Although traditional black-box testing helps solve the input/output mapping problem, when transparency is lacking, humans will have difficulty trusting the test model. Of course, an AI platform solution is a black box; there are unique AI techniques that can help verify the functionality of an AI program; in this way, testing is not just a matter of input and output mapping. For design considerations, some black-box testing techniques driven by artificial intelligence include:
- Posterior predictive checks (PPC) simulate the replicated data under the fitted model and then compare it with the observations data for comparison. Thus, tests can use posterior predictions to "look for systematic differences between real and simulated data."
- Genetic algorithm for optimizing test cases. One of the challenges in generating test cases is finding a set of data that, when used as input to the software under test, results in the highest coverage. If this problem is solved, the test cases can be optimized. There are adaptive heuristic search algorithms that simulate basic behaviors performed in natural evolutionary processes, such as selection, crossover, and mutation. When using heuristic search to generate test cases, feedback information about the test application is used to determine whether the test data meets the test requirements. The feedback mechanism can gradually adjust the test data until the test requirements are met.
- Neural network for automatically generating test cases. These are physical cellular systems that can acquire, store, and process empirical knowledge. They mimic the human brain to perform learning tasks. Neural network learning technology is used to automatically generate test cases. In this model, the neural network is trained on a set of test cases applied to the original version of the AI platform product. Network training only targets the input and output of the system. The trained network can then be used as an artificial oracle to evaluate the correctness of the output produced by new and potentially buggy versions of the AI platform product.
- Fuzzy logic for model-based regression test selection. While these methods are useful in projects that already use model-driven development methods, a key obstacle is that models are often created at a high level of abstraction. They lack the information needed to establish traceability links between coverage-related execution traces in models and code-level test cases. Fuzzy logic-based methods can be used to automatically refine abstract models to produce detailed models that allow the identification of traceability links. This process introduces a degree of uncertainty – an uncertainty that can be resolved by applying refinement-based fuzzy logic. The logic of this approach is to classify test cases into retestable ones based on their probabilistic correctness associated with the refinement algorithm used.
For more detailed information about this part of knowledge, please refer to"Black Box Testing of Machine Learning Models".
Ensure integration and interfaces in AIaaS solutions
All SaaS solutions, including AIaaS solutions, will come with a set of predefined web services. Enterprise applications and other intelligent resources can interact with these services to achieve the promised results. Today, Web services have evolved to a level that provides platform independence, that is, interoperability. This increased flexibility enables most Web services to be used by different systems. Of course, the complexity of these interfaces also requires a corresponding increase in testing levels. For example, in a CI/CD environment, it becomes a critical task to check the compatibility of these interfaces in every built application package.
Currently, the main challenge in this area is to implement virtualized web services and verify the data flow between the AI platform solution and the application or IoT interface. In summary, the main reasons why interface/Web service testing is complicated include:
- There is no testable user interface unless it is already integrated with another source that may not be ready for testing.
- All elements defined in these services require validation, regardless of which application uses them or how often they are used.
- The basic security parameters of the service must be verified.
- Connect to services via different communication protocols.
- Simultaneously calling multiple channels of a service can cause performance and scalability issues.
Therefore, testing the interface layer is particularly necessary:
- Simulate component or application behavior. The complexity of AI applications’ interfaces with humans, machines, and software should be simulated in AI testing to ensure correctness, completeness, consistency, and speed.
- Check for use of non-standard code. Using open source libraries and adopting real-world applications can bring non-standard code and data into the enterprise IT environment. Therefore, these should all be verified.
Ensure user experience in AIaaS solutions
In the new social reality where people mainly work and live remotely, customer experience has become a necessity for business success. This is a larger goal in the artificial intelligence scheme. Non-functional testing is a proven phenomenon that delivers meaningful customer experiences by validating properties such as performance, security, and accessibility. In general, next-generation technologies increase the complexity of experience assurance.
The following are some important design considerations for ensuring user experience throughout the AI testing framework.
- Design for experience, not test for experience. Enterprise AI strategies should start from the end user’s perspective. It is important to ensure that the testing team represents actual customers. Involving the client early in the design not only helps with the design, but also helps gain the client's trust early on.
- Achieve agility and automation by building test optimization models. User experience issues should be considered from the “swarm” stage of the testing cycle on, as early testing for user experience will help achieve a build-test-optimized development cycle.
- Continuous security with agile methods is critical. Have the enterprise security team be part of an agile team that: 1) owns and validates the organization’s threat model during the “swarm” period of testing; 2) assesses structural vulnerabilities across all multi-channel interfaces the SaaS AI solution architecture may have (from hypothetical hacker's perspective).
- Speed is of the essence. The properties of AI data, such as volume, velocity, diversity, and variability, necessitate preprocessing, parallel/distributed processing, and/or stream processing. Performance testing will help optimize the design for distributed processing, which is necessary for the speed users expect from the system.
- The nuances of text and speech testing are also important. Many research surveys indicate that conversational AI remains at the top of corporate agendas. As new technologies such as augmented reality, virtual reality, and edge artificial intelligence continue to emerge, requirements such as testing text, speech, and natural language processing should all be able to be addressed.
- Simulation helps test limits. Examining user scenarios is the basis of experience assurance. When it comes to AI, testing anomalies, errors, and violations will help predict system behavior, which in turn will help us validate the error/fault tolerance level of AI applications.
- Trust, transparency and diversity. Validate enterprise users’ trust in AI results, verify transparency of data sources and algorithms, require transparency to target risk reduction and increase confidence in AI, ensure diversity of data sources and users/testers to examine AI ethics and its Accuracy, all of these are critical. In order to do this, testers should not only improve their domain knowledge but also understand the technical know-how of data, algorithms and integration processes in large enterprise IT.
Conclusion
In short, continuous testing is a basic requirement for every enterprise to adopt artificial intelligence platform solutions. Therefore, we should adopt a modular approach to improve the design of data, algorithms, integration and experience assurance activities. This will help us create a continuous testing ecosystem so that enterprise IT can be ready to accept frequent changes in internal and external AI components.
Translator Introduction
Zhu Xianzhong, 51CTO community editor, 51CTO expert blogger, lecturer, computer teacher at a university in Weifang, and a veteran in the freelance programming industry. In the early days, he focused on various Microsoft technologies (compiled three technical books related to ASP.NET AJX and Cocos 2d-X). In the past ten years, he has devoted himself to the open source world (familiar with popular full-stack web development technology) and learned about OneNet/AliOS Arduino/ IoT development technologies such as ESP32/Raspberry Pi and big data development technologies such as Scala Hadoop Spark Flink.
Original title: Quality Engineering Design for AI Platform Adoption, author: Anbu Muppidathi
The above is the detailed content of Quality engineering design in artificial intelligence platform solutions. For more information, please follow other related articles on the PHP Chinese website!
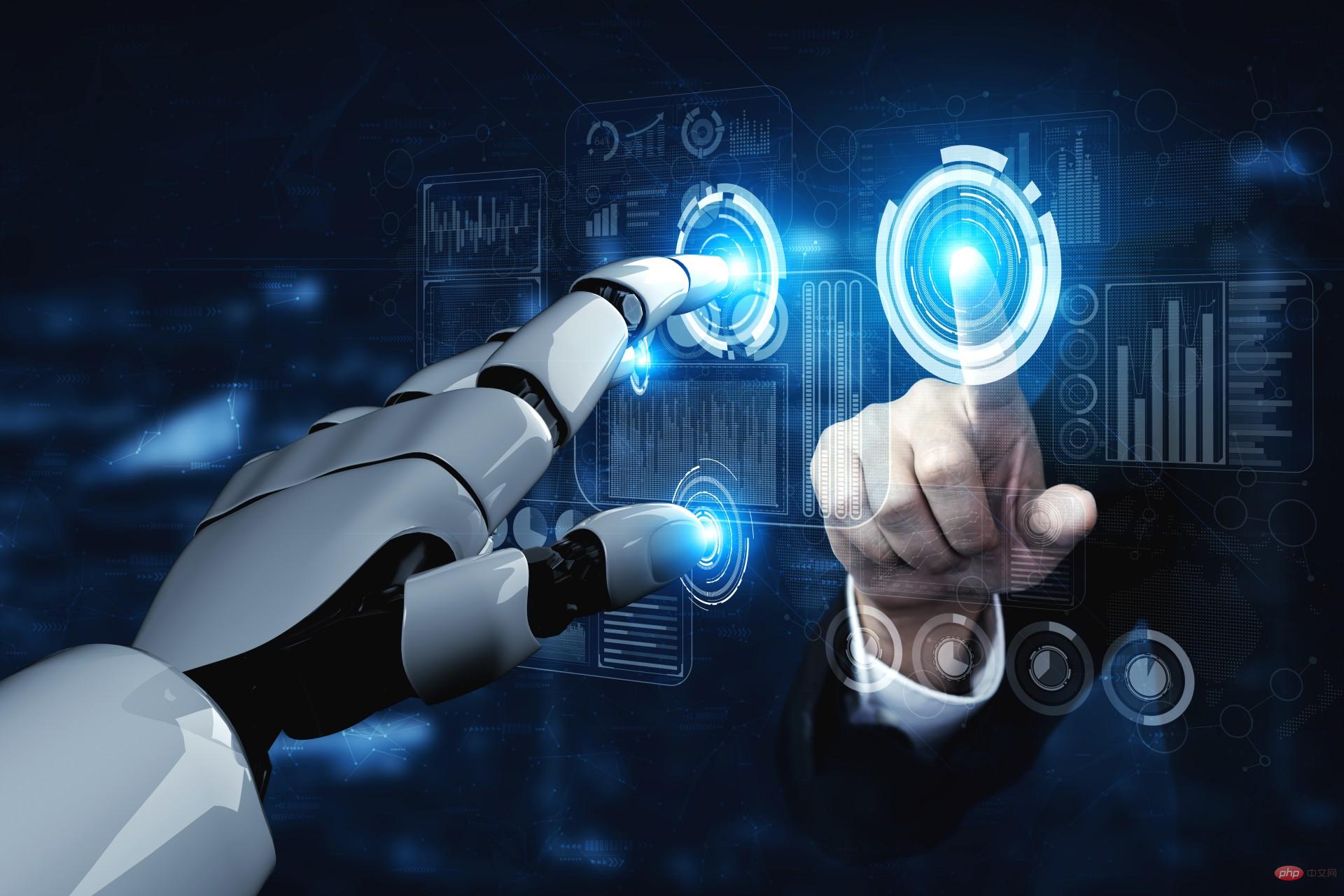
机器学习是一个不断发展的学科,一直在创造新的想法和技术。本文罗列了2023年机器学习的十大概念和技术。 本文罗列了2023年机器学习的十大概念和技术。2023年机器学习的十大概念和技术是一个教计算机从数据中学习的过程,无需明确的编程。机器学习是一个不断发展的学科,一直在创造新的想法和技术。为了保持领先,数据科学家应该关注其中一些网站,以跟上最新的发展。这将有助于了解机器学习中的技术如何在实践中使用,并为自己的业务或工作领域中的可能应用提供想法。2023年机器学习的十大概念和技术:1. 深度神经网
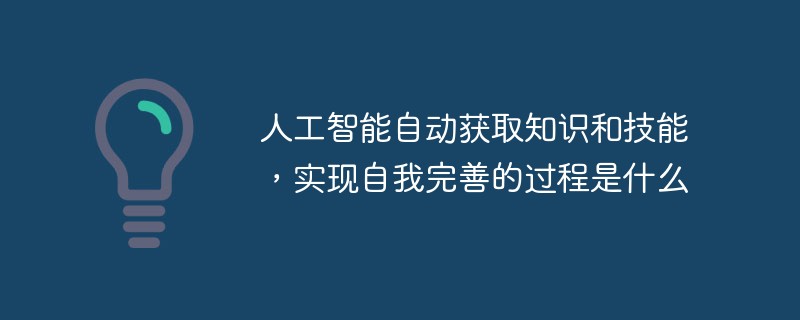
实现自我完善的过程是“机器学习”。机器学习是人工智能核心,是使计算机具有智能的根本途径;它使计算机能模拟人的学习行为,自动地通过学习来获取知识和技能,不断改善性能,实现自我完善。机器学习主要研究三方面问题:1、学习机理,人类获取知识、技能和抽象概念的天赋能力;2、学习方法,对生物学习机理进行简化的基础上,用计算的方法进行再现;3、学习系统,能够在一定程度上实现机器学习的系统。

本文将详细介绍用来提高机器学习效果的最常见的超参数优化方法。 译者 | 朱先忠审校 | 孙淑娟简介通常,在尝试改进机器学习模型时,人们首先想到的解决方案是添加更多的训练数据。额外的数据通常是有帮助(在某些情况下除外)的,但生成高质量的数据可能非常昂贵。通过使用现有数据获得最佳模型性能,超参数优化可以节省我们的时间和资源。顾名思义,超参数优化是为机器学习模型确定最佳超参数组合以满足优化函数(即,给定研究中的数据集,最大化模型的性能)的过程。换句话说,每个模型都会提供多个有关选项的调整“按钮
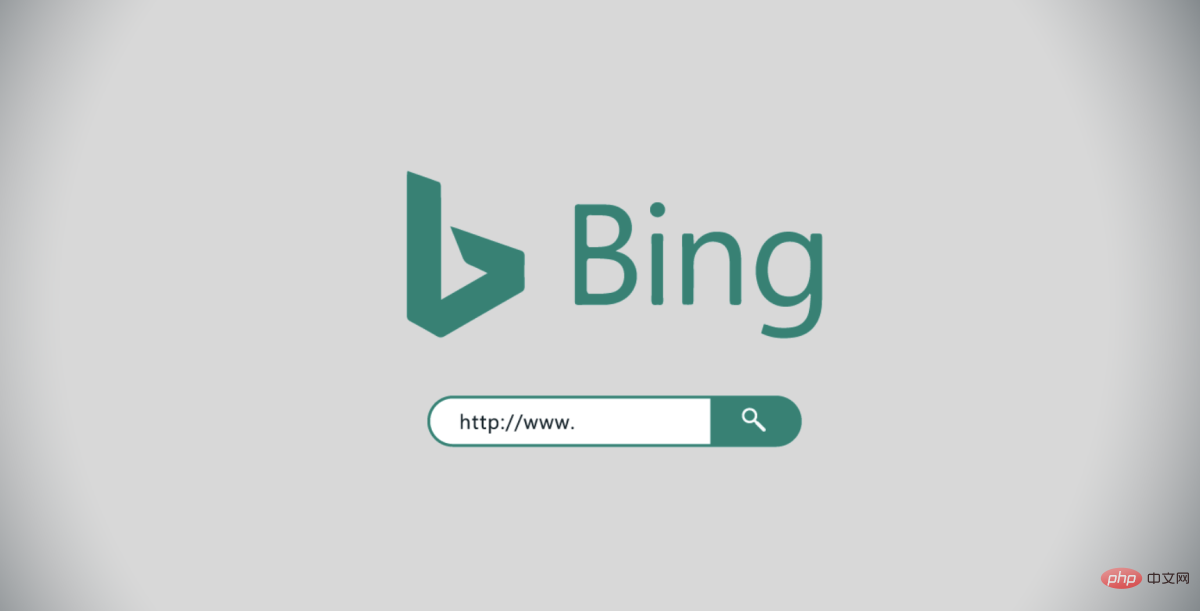
截至3月20日的数据显示,自微软2月7日推出其人工智能版本以来,必应搜索引擎的页面访问量增加了15.8%,而Alphabet旗下的谷歌搜索引擎则下降了近1%。 3月23日消息,外媒报道称,分析公司Similarweb的数据显示,在整合了OpenAI的技术后,微软旗下的必应在页面访问量方面实现了更多的增长。截至3月20日的数据显示,自微软2月7日推出其人工智能版本以来,必应搜索引擎的页面访问量增加了15.8%,而Alphabet旗下的谷歌搜索引擎则下降了近1%。这些数据是微软在与谷歌争夺生
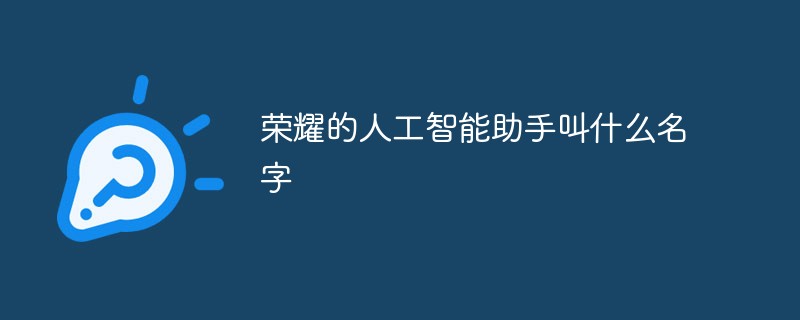
荣耀的人工智能助手叫“YOYO”,也即悠悠;YOYO除了能够实现语音操控等基本功能之外,还拥有智慧视觉、智慧识屏、情景智能、智慧搜索等功能,可以在系统设置页面中的智慧助手里进行相关的设置。
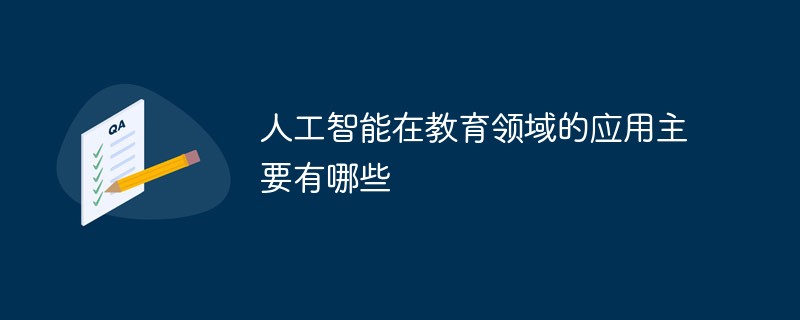
人工智能在教育领域的应用主要有个性化学习、虚拟导师、教育机器人和场景式教育。人工智能在教育领域的应用目前还处于早期探索阶段,但是潜力却是巨大的。
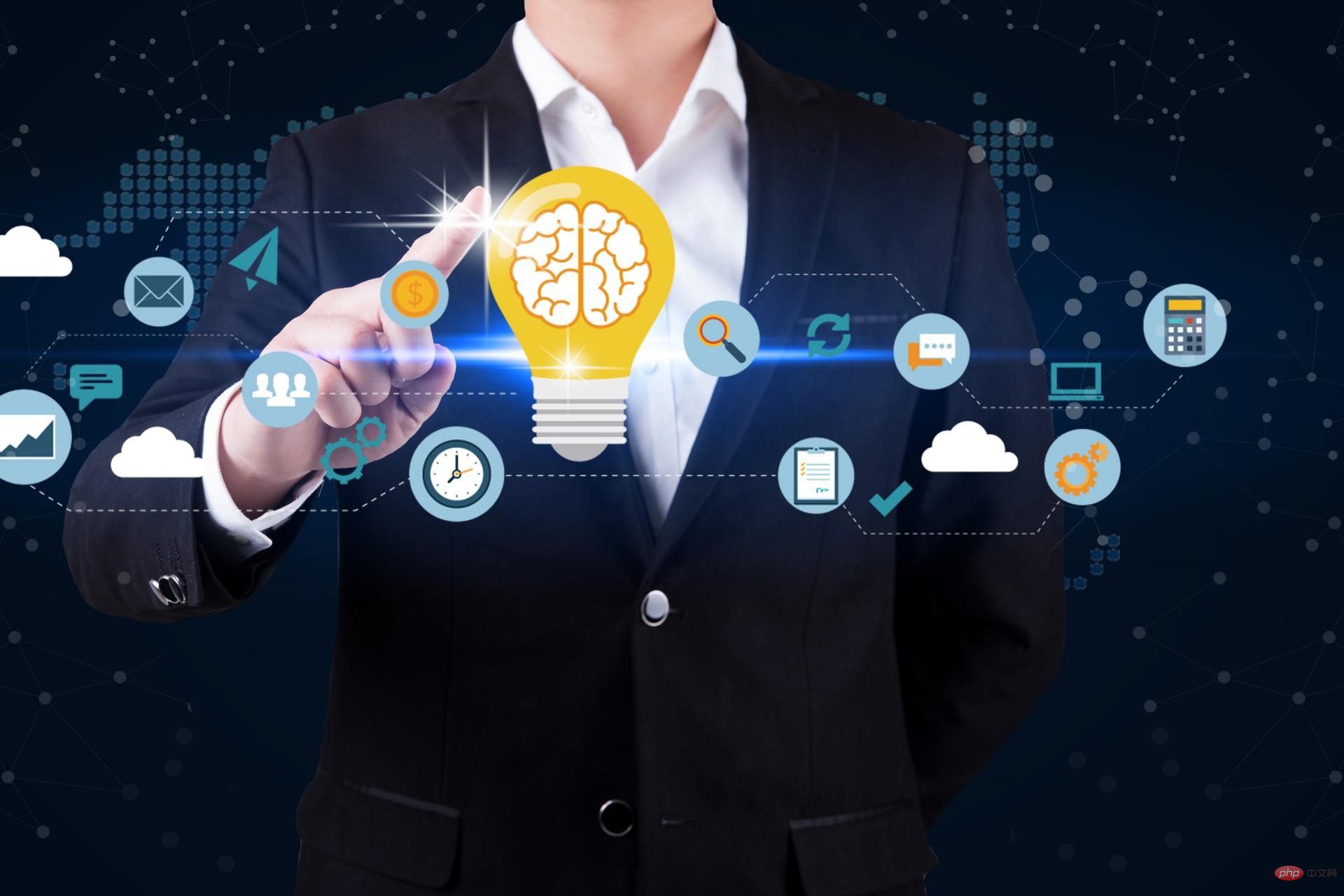
阅读论文可以说是我们的日常工作之一,论文的数量太多,我们如何快速阅读归纳呢?自从ChatGPT出现以后,有很多阅读论文的服务可以使用。其实使用ChatGPT API非常简单,我们只用30行python代码就可以在本地搭建一个自己的应用。 阅读论文可以说是我们的日常工作之一,论文的数量太多,我们如何快速阅读归纳呢?自从ChatGPT出现以后,有很多阅读论文的服务可以使用。其实使用ChatGPT API非常简单,我们只用30行python代码就可以在本地搭建一个自己的应用。使用 Python 和 C
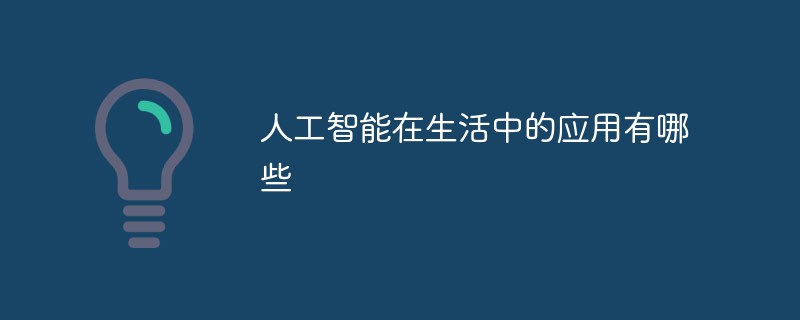
人工智能在生活中的应用有:1、虚拟个人助理,使用者可通过声控、文字输入的方式,来完成一些日常生活的小事;2、语音评测,利用云计算技术,将自动口语评测服务放在云端,并开放API接口供客户远程使用;3、无人汽车,主要依靠车内的以计算机系统为主的智能驾驶仪来实现无人驾驶的目标;4、天气预测,通过手机GPRS系统,定位到用户所处的位置,在利用算法,对覆盖全国的雷达图进行数据分析并预测。


Hot AI Tools

Undresser.AI Undress
AI-powered app for creating realistic nude photos

AI Clothes Remover
Online AI tool for removing clothes from photos.

Undress AI Tool
Undress images for free

Clothoff.io
AI clothes remover

AI Hentai Generator
Generate AI Hentai for free.

Hot Article

Hot Tools
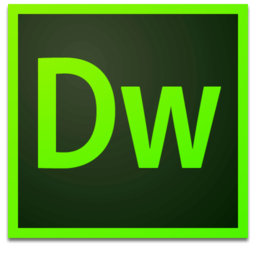
Dreamweaver Mac version
Visual web development tools
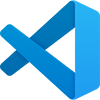
VSCode Windows 64-bit Download
A free and powerful IDE editor launched by Microsoft

MinGW - Minimalist GNU for Windows
This project is in the process of being migrated to osdn.net/projects/mingw, you can continue to follow us there. MinGW: A native Windows port of the GNU Compiler Collection (GCC), freely distributable import libraries and header files for building native Windows applications; includes extensions to the MSVC runtime to support C99 functionality. All MinGW software can run on 64-bit Windows platforms.

PhpStorm Mac version
The latest (2018.2.1) professional PHP integrated development tool

SAP NetWeaver Server Adapter for Eclipse
Integrate Eclipse with SAP NetWeaver application server.
