When we write multi-threaded programs, we often encounter two types of variables.
One is a global variable, shared by multiple threads. In order to avoid changing the behavior, we have mentioned before that locking is required.
One is local variables. Only used by one thread, and threads do not affect each other.
For example, the count
variable defined in the task()
function in the following program is a local variable. Even if we create two threads, the increments of both count
will not affect each other, because count
is defined in task
.
import threading def task(): count = 0 for i in range(1000): count += 1 print count if __name__ == '__main__': t1 = threading.Thread(target=task) t1.start() t2 = threading.Thread(target=task) t2.start()
So, is it perfect to handle it this way? Not yet.
The above example is a very simple one, but when we encounter a more complex business logic, such as multiple local variables, multiple function calls, etc., defining local variables in this way will become unsuccinct. ,trouble.
Multiple calls of functions refer to, for example:
We have defined a function, methodA(), this method body calls methodB(), methodB() method body calls methodC()...
If we call methodA() in a thread and use a variable attr, then we need to pass attr to subsequent functions layer by layer.
Is there a way that allows us to define a variable in a thread, and then all functions in the thread can be called? This is simple and clear?
Python does it for us, that is ThreadLocal.
The usage of ThreadLocal only requires three steps:
Define an object threading.local
Bind parameters to the object within the thread. All bound parameters are thread-isolated.
Call within the thread.
The code is shown below:
# coding=utf-8 import threading local = threading.local() # 创建一个全局的对象 def task(): local.count = 0 # 初始化一个线程内变量,该变量线程间互不影响。 for i in range(1000): count_plus() def count_plus(): local.count += 1 print threading.current_thread().name, local.count if __name__ == '__main__': t1 = threading.Thread(target=task) t1.start() t2 = threading.Thread(target=task) t2.start()
For more python study notes - ThreadLocal related articles, please pay attention to the PHP Chinese website!
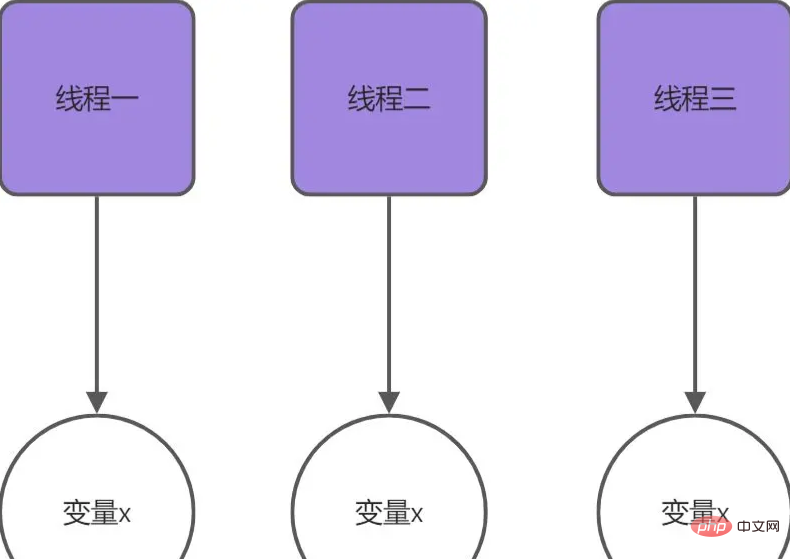
如图所示:快速开始接下来我们就先用一个简单的样例给大家展示一下ThreadLocal的基本用法packagecuit.pymjl.thradlocal;/***@authorPymjl*@version1.0*@date2022/7/110:56**/publicclassMainTest{staticThreadLocalthreadLocal=newThreadLocal();staticvoidprint(Stringstr){//打印当前线程中本地内存中本地变量的值System.out.
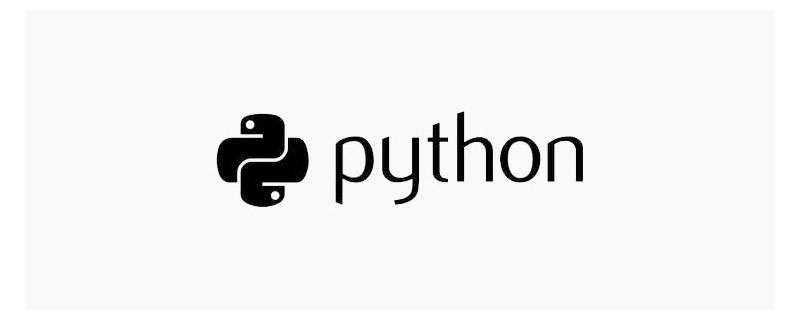
本篇文章给大家带来了关于Python的相关知识,其中主要介绍了关于Seaborn的相关问题,包括了数据可视化处理的散点图、折线图、条形图等等内容,下面一起来看一下,希望对大家有帮助。
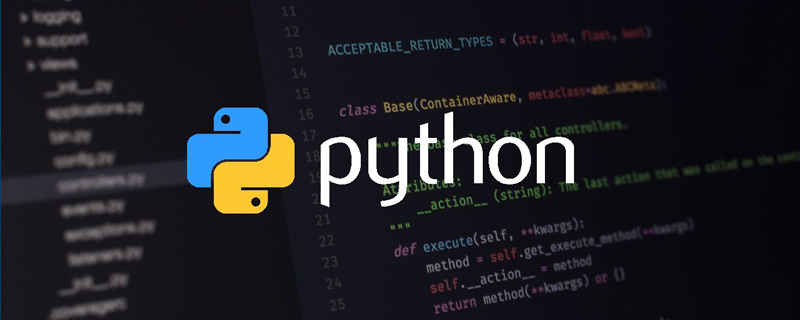
本篇文章给大家带来了关于Python的相关知识,其中主要介绍了关于进程池与进程锁的相关问题,包括进程池的创建模块,进程池函数等等内容,下面一起来看一下,希望对大家有帮助。

线程封闭线程封闭一般通过以下三个方法:Ad-hoc线程封闭:程序控制实现,最糟糕,忽略堆栈封闭:局部变量,无并发问题ThreadLocal线程封闭:特别好的封闭方法方法2是最常用的,变量定义在接口内,本文主要讲解方法三,SpringBoot项目通过自定义过滤器和拦截器实现ThreadLocal线程封闭。实现Filter接口自定义过滤器和继承HandlerInterceptorAdapter自定义拦截器。ThreadLocal线程封闭实现步骤封装ThredLocal的方法/***自定义Reques
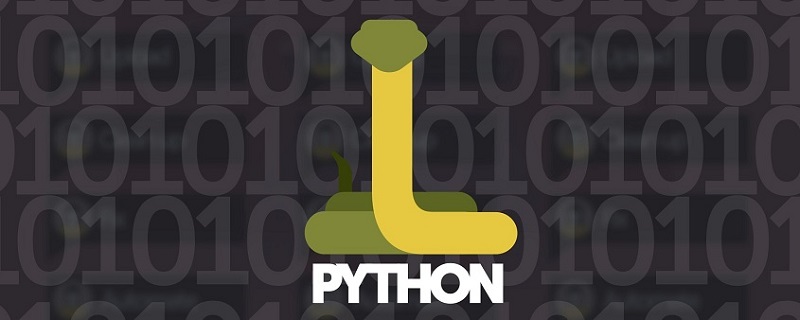
本篇文章给大家带来了关于Python的相关知识,其中主要介绍了关于数据类型之字符串、数字的相关问题,下面一起来看一下,希望对大家有帮助。
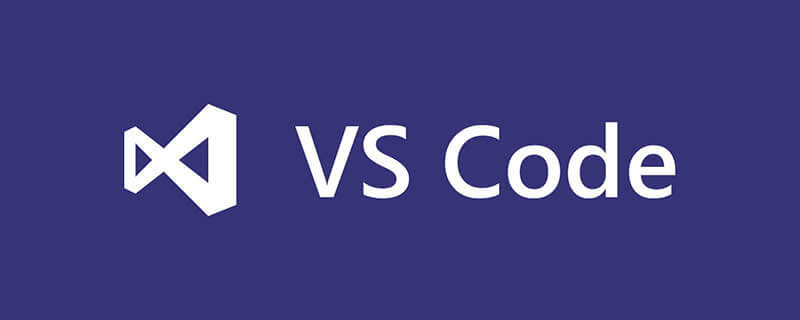
VS Code的确是一款非常热门、有强大用户基础的一款开发工具。本文给大家介绍一下10款高效、好用的插件,能够让原本单薄的VS Code如虎添翼,开发效率顿时提升到一个新的阶段。
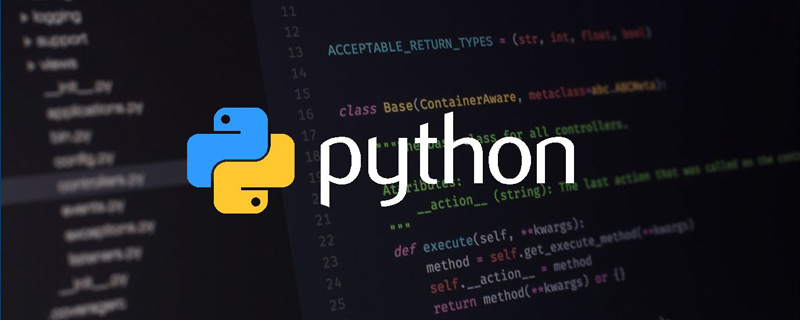
本篇文章给大家带来了关于Python的相关知识,其中主要介绍了关于numpy模块的相关问题,Numpy是Numerical Python extensions的缩写,字面意思是Python数值计算扩展,下面一起来看一下,希望对大家有帮助。


Hot AI Tools

Undresser.AI Undress
AI-powered app for creating realistic nude photos

AI Clothes Remover
Online AI tool for removing clothes from photos.

Undress AI Tool
Undress images for free

Clothoff.io
AI clothes remover

AI Hentai Generator
Generate AI Hentai for free.

Hot Article

Hot Tools

SAP NetWeaver Server Adapter for Eclipse
Integrate Eclipse with SAP NetWeaver application server.

SublimeText3 Linux new version
SublimeText3 Linux latest version
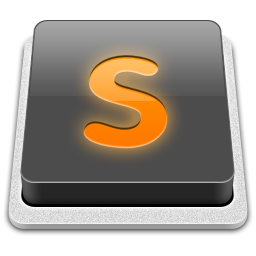
SublimeText3 Mac version
God-level code editing software (SublimeText3)

Zend Studio 13.0.1
Powerful PHP integrated development environment

SublimeText3 Chinese version
Chinese version, very easy to use
