


Summary of some tips for implementing iterators in Python programming
yield implements iterator
As described in the introduction, it is a little troublesome to implement iter, next manually every time to implement an iterable function, and the required code is also relatively objective. In python, an iterator can also be implemented by using yield. Yield has a key function. It can interrupt the current execution logic, maintain the scene (the status of various values, the execution position, etc.), return the corresponding value, and the next execution can seamlessly continue the last time. Continue execution at the place where the loop continues until the pre-set exit conditions are met or an error occurs and is forced to be interrupted.
Its specific function is that it can be used as return to return a value from the function. The difference is that after returning with yield, the function can continue execution from the point returned by yield. In other words, yield returns the function, hands a return value to the caller, and then "teleports" back, allowing the function to continue running until the yield statement returns a new value. After using yield to return, what the caller actually gets is an iterator object, and the value of the iterator is the return value. Calling the next() method of the iterator will cause the function to restore the execution environment of the yield statement and continue running until Until the next yield is encountered, if no yield is encountered, an exception will be thrown to indicate the end of the iteration.
Take a look at an example:
>>> def test_yield(): ... yield 1 ... yield 2 ... yield (1,2) ... >>> a = test_yield() >>> a.next() 1 >>> a.next() 2 >>> a.next() (1, 2) >>> a.next() Traceback (most recent call last): File "<stdin>", line 1, in ? StopIteration
Just listening to the description, I think it is very consistent with the way iterators work, right? Indeed, yield can turn the function it is located into an iterator. Let’s briefly describe the work using the most classic example of the Fibonacci sequence. Method:
def fab(max): n, a, b = 0, 0, 1 while n < max: print b, "is generated" yield b a, b = b, a + b n = n + 1 >>> for item in fab(5): ... print item ... 1 is generated 1 1 is generated 1 2 is generated 2 3 is generated 3 5 is generated 5
Let’s recall the syntactic sugar of the for keyword. When traversing the Fibonacci sequence values within 5, it is obvious that fab(5) generates an iterable object. When the traversal starts, its iter The method is called to return an actual working iterator object, and each call to its next method returns a Fibonacci sequence value which is then printed.
We can print out the properties of the object returned by calling the generator function to see what is going on:
>>> temp_gen = fab(5) >>> dir(temp_gen) ['__class__', '__delattr__', '__doc__', '__format__', '__getattribute__', '__hash__', '__init__', '__iter__', '__name__', '__new__', '__reduce__', '__reduce_ex__', '__repr__', '__setattr__', '__sizeof__', '__str__', '__subclasshook__', 'close', 'gi_code', 'gi_frame', 'gi_running', 'next', 'send', 'throw']
As described above, simply calling fab will not cause the function to start returning any value immediately, and it can be seen from the printed attribute list of fab(5) that the object returned by the generator function contains __iter__, next realization. Compared with our manual implementation, using yield is very convenient to achieve the functions we want, and the amount of code is reduced a lot.
Generator Expression
Another way to generate iterator objects more elegantly in python is to use generator expression. It has a very similar writing method to list parsing expression. It just changes the square brackets [] into () , but the actual effect of small changes is indeed very different:
>>> temp_gen = (x for x in range(5)) >>> temp_gen <generator object <genexpr> at 0x7192d8> >>> for item in temp_gen: ... print item ... 0 1 2 3 4 >>> dir(temp_gen) ['__class__', '__delattr__', '__doc__', '__format__', '__getattribute__', '__hash__', '__init__', '__iter__', '__name__', '__new__', '__reduce__', '__reduce_ex__', '__repr__', '__setattr__', '__sizeof__', '__str__', '__subclasshook__', 'close', 'gi_code', 'gi_frame', 'gi_running', 'next', 'send', 'throw']
After reading the description of yield above, this example and the corresponding output log are quite straightforward. Whether it is the print log description of temp_gen, the output result of the for statement traversal or the attribute list output by calling dir, they are all naked. Indicates that the generator expression does produce an object capable of supporting iteration. In addition, functions can also be called in expressions to add appropriate filtering conditions.
Built-in libraries itertools and iter
Python’s built-in library itertools provides a large number of tool methods that can help us create objects that can efficiently traverse and iterate. It contains many interesting and useful methods, such as chain, izip/izip_longest, combinations, ifilter and so on. There is also a built-in iter function in python that is very useful, it can return an iterator object, and then you can view the corresponding help document for a brief look:
>>> iter('abc') <iterator object at 0x718590> >>> str_iterator = iter('abc') >>> next(str_iterator) 'a' >>> next(str_iterator) 'b' >>> lst_gen = iter([1,2,3,4]) >>> lst_gen <listiterator object at 0x728e30> >>> dir(lst_gen) ['__class__', '__delattr__', '__doc__', '__format__', '__getattribute__', '__hash__', '__init__', '__iter__', '__length_hint__', '__new__', '__reduce__', '__reduce_ex__', '__repr__', '__setattr__', '__sizeof__', '__str__', '__subclasshook__', 'next'] >>> help(iter) Help on built-in function iter in module builtins: iter(...) iter(iterable) -> iterator iter(callable, sentinel) -> iterator Get an iterator from an object. In the first form, the argument must supply its own iterator, or be a sequence. In the second form, the callable is called until it returns the sentinel.
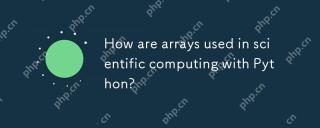
ArraysinPython,especiallyviaNumPy,arecrucialinscientificcomputingfortheirefficiencyandversatility.1)Theyareusedfornumericaloperations,dataanalysis,andmachinelearning.2)NumPy'simplementationinCensuresfasteroperationsthanPythonlists.3)Arraysenablequick
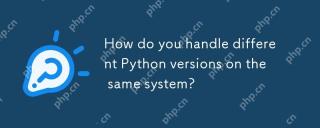
You can manage different Python versions by using pyenv, venv and Anaconda. 1) Use pyenv to manage multiple Python versions: install pyenv, set global and local versions. 2) Use venv to create a virtual environment to isolate project dependencies. 3) Use Anaconda to manage Python versions in your data science project. 4) Keep the system Python for system-level tasks. Through these tools and strategies, you can effectively manage different versions of Python to ensure the smooth running of the project.
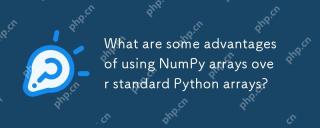
NumPyarrayshaveseveraladvantagesoverstandardPythonarrays:1)TheyaremuchfasterduetoC-basedimplementation,2)Theyaremorememory-efficient,especiallywithlargedatasets,and3)Theyofferoptimized,vectorizedfunctionsformathematicalandstatisticaloperations,making
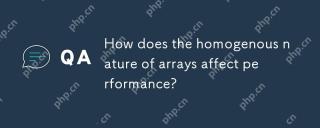
The impact of homogeneity of arrays on performance is dual: 1) Homogeneity allows the compiler to optimize memory access and improve performance; 2) but limits type diversity, which may lead to inefficiency. In short, choosing the right data structure is crucial.
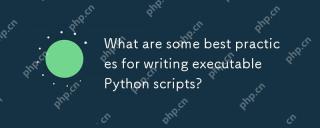
TocraftexecutablePythonscripts,followthesebestpractices:1)Addashebangline(#!/usr/bin/envpython3)tomakethescriptexecutable.2)Setpermissionswithchmod xyour_script.py.3)Organizewithacleardocstringanduseifname=="__main__":formainfunctionality.4
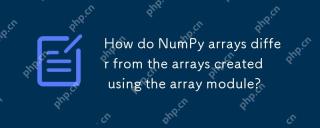
NumPyarraysarebetterfornumericaloperationsandmulti-dimensionaldata,whilethearraymoduleissuitableforbasic,memory-efficientarrays.1)NumPyexcelsinperformanceandfunctionalityforlargedatasetsandcomplexoperations.2)Thearraymoduleismorememory-efficientandfa
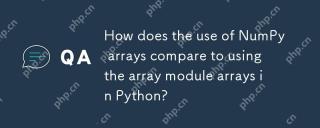
NumPyarraysarebetterforheavynumericalcomputing,whilethearraymoduleismoresuitableformemory-constrainedprojectswithsimpledatatypes.1)NumPyarraysofferversatilityandperformanceforlargedatasetsandcomplexoperations.2)Thearraymoduleislightweightandmemory-ef
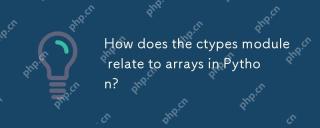
ctypesallowscreatingandmanipulatingC-stylearraysinPython.1)UsectypestointerfacewithClibrariesforperformance.2)CreateC-stylearraysfornumericalcomputations.3)PassarraystoCfunctionsforefficientoperations.However,becautiousofmemorymanagement,performanceo


Hot AI Tools

Undresser.AI Undress
AI-powered app for creating realistic nude photos

AI Clothes Remover
Online AI tool for removing clothes from photos.

Undress AI Tool
Undress images for free

Clothoff.io
AI clothes remover

Video Face Swap
Swap faces in any video effortlessly with our completely free AI face swap tool!

Hot Article

Hot Tools

PhpStorm Mac version
The latest (2018.2.1) professional PHP integrated development tool

Atom editor mac version download
The most popular open source editor
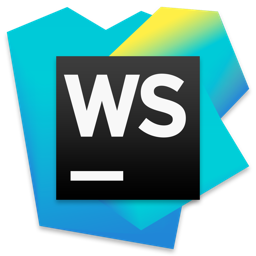
WebStorm Mac version
Useful JavaScript development tools

SecLists
SecLists is the ultimate security tester's companion. It is a collection of various types of lists that are frequently used during security assessments, all in one place. SecLists helps make security testing more efficient and productive by conveniently providing all the lists a security tester might need. List types include usernames, passwords, URLs, fuzzing payloads, sensitive data patterns, web shells, and more. The tester can simply pull this repository onto a new test machine and he will have access to every type of list he needs.
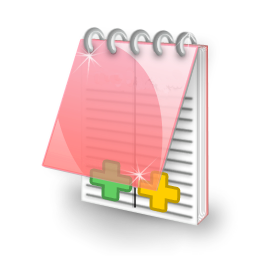
EditPlus Chinese cracked version
Small size, syntax highlighting, does not support code prompt function
