作者:perrygeo
译者:赖勇浩(http://laiyonghao.com)
原文:http://www.perrygeo.net/wordpress/?p=116
我最喜欢的是Python,它的代码优雅而实用,可惜纯粹从速度上来看它比大多数语言都要慢。大多数人也认为的速度和易于使用是两极对立的——编写C代码的确非常痛苦。而 Cython 试图消除这种两重性,并让你同时拥有 Python 的语法和 C 数据类型和函数——它们两个都是世界上最好的。请记住,我绝不是我在这方面的专家,这是我的第一次Cython真实体验的笔记:
编辑:根据一些我收到的反馈,大家似乎有点混淆——Cython是用来生成 C 扩展到而不是独立的程序的。所有的加速都是针对一个已经存在的 Python 应用的一个函数进行的。没有使用 C 或 Lisp 重写整个应用程序,也没有手写C扩展 。只是用一个简单的方法来整合C的速度和C数据类型到 Python 函数中去。
现在可以说,我们能使下文的 great_circle 函数更快。所谓 great_circle 是计算沿地球表面两点之间的距离的问题:
p1.py
import math
def great_circle(lon1,lat1,lon2,lat2):
radius = 3956 #miles
x = math.pi/180.0
a = (90.0-lat1)*(x)
b = (90.0-lat2)*(x)
theta = (lon2-lon1)*(x)
c = math.acos((math.cos(a)*math.cos(b)) +
(math.sin(a)*math.sin(b)*math.cos(theta)))
return radius*c
让我们调用它 50 万次并测定它的时间 :
import timeit
lon1, lat1, lon2, lat2 = -72.345, 34.323, -61.823, 54.826
num = 500000
t = timeit.Timer("p1.great_circle(%f,%f,%f,%f)" % (lon1,lat1,lon2,lat2),
"import p1")
print "Pure python function", t.timeit(num), "sec"
约2.2秒 。它太慢了!
让我们试着快速地用Cython改写它,然后看看是否有差别:
c1.pyx
import math
def great_circle(float lon1,float lat1,float lon2,float lat2):
cdef float radius = 3956.0
cdef float pi = 3.14159265
cdef float x = pi/180.0
cdef float a,b,theta,c
a = (90.0-lat1)*(x)
b = (90.0-lat2)*(x)
theta = (lon2-lon1)*(x)
c = math.acos((math.cos(a)*math.cos(b)) + (math.sin(a)*math.sin(b)*math.cos(theta)))
return radius*c
请注意,我们仍然import math——cython让您在一定程度上混搭Python和C数据类型在。转换是自动的,但并非没有代价。在这个例子中我们所做的就是定义一个Python函数,声明它的输入参数是浮点数类型,并为所有变量声明类型为C浮点数据类型。计算部分它仍然使用了Python的 math 模块。
现在我们需要将其转换为C代码再编译为Python扩展。完成这一部的最好的办法是编写一个名为setup.py发布脚本。但是,现在我们用手工方式 ,以了解其中的巫术:
# this will create a c1.c file - the C source code to build a python extension
cython c1.pyx
# Compile the object file
gcc -c -fPIC -I/usr/include/python2.5/ c1.c
# Link it into a shared library
gcc -shared c1.o -o c1.so
现在你应该有一个c1.so(或.dll)文件,它可以被Python import。现在运行一下:
t = timeit.Timer("c1.great_circle(%f,%f,%f,%f)" % (lon1,lat1,lon2,lat2),
"import c1")
print "Cython function (still using python math)", t.timeit(num), "s
约1.8秒 。并没有我们一开始期望的那种大大的性能提升。使用 python 的 match 模块应该是瓶颈。现在让我们使用C标准库替代之:
c2.pyx
cdef extern from "math.h":
float cosf(float theta)
float sinf(float theta)
float acosf(float theta)
def great_circle(float lon1,float lat1,float lon2,float lat2):
cdef float radius = 3956.0
cdef float pi = 3.14159265
cdef float x = pi/180.0
cdef float a,b,theta,c
a = (90.0-lat1)*(x)
b = (90.0-lat2)*(x)
theta = (lon2-lon1)*(x)
c = acosf((cosf(a)*cosf(b)) + (sinf(a)*sinf(b)*cosf(theta)))
return radius*cec"
与 import math 相应,我们使用cdef extern 的方式使用从指定头文件声明函数(在此就是使用C标准库的math.h)。我们替代了代价高昂的的Python函数,然后建立新的共享库,并重新测试:
t = timeit.Timer("c2.great_circle(%f,%f,%f,%f)" % (lon1,lat1,lon2,lat2),
"import c2")
print "Cython function (using trig function from math.h)", t.timeit(num), "sec"
现在有点喜欢它了吧?0.4秒 -比纯Python函数有5倍的速度增长。我们还有什么方法可以再提高速度?c2.great_circle()仍是一个Python函数调用,这意味着它产生Python的API的开销(构建参数元组等),如果我们可以写一个纯粹的C函数的话,我们也许能够加快速度。
c3.pyx
cdef extern from "math.h":
float cosf(float theta)
float sinf(float theta)
float acosf(float theta)
cdef float _great_circle(float lon1,float lat1,float lon2,float lat2):
cdef float radius = 3956.0
cdef float pi = 3.14159265
cdef float x = pi/180.0
cdef float a,b,theta,c
a = (90.0-lat1)*(x)
b = (90.0-lat2)*(x)
theta = (lon2-lon1)*(x)
c = acosf((cosf(a)*cosf(b)) + (sinf(a)*sinf(b)*cosf(theta)))
return radius*c
def great_circle(float lon1,float lat1,float lon2,float lat2,int num):
cdef int i
cdef float x
for i from 0
x = _great_circle(lon1,lat1,lon2,lat2)
return x
请注意,我们仍然有一个Python函数( def ),它接受一个额外的参数 num。这个函数里的循环使用for i from 0
为了证明我们所做的已经足够优化,可以用纯C写一个小应用,然后测定时间:
#include
#include
#define NUM 500000
float great_circle(float lon1, float lat1, float lon2, float lat2){
float radius = 3956.0;
float pi = 3.14159265;
float x = pi/180.0;
float a,b,theta,c;
a = (90.0-lat1)*(x);
b = (90.0-lat2)*(x);
theta = (lon2-lon1)*(x);
c = acos((cos(a)*cos(b)) + (sin(a)*sin(b)*cos(theta)));
return radius*c;
}
int main() {
int i;
float x;
for (i=0; i
x = great_circle(-72.345, 34.323, -61.823, 54.826);
printf("%f", x);
}
用gcc -lm -o ctest ctest.c编译它,测试用time ./ctest ...大约0.2秒 。这使我有信心,我Cython扩展相对于我的C代码也极有效率(这并不是说我的C编程能力很弱)。
能够用 cython 优化多少性能通常取决于有多少循环,数字运算和Python函数调用,这些都会让程序变慢。已经有一些人报告说在某些案例上 100 至 1000 倍的速度提升。至于其他的任务,可能不会那么有用。在疯狂地用 Cython 重写 Python 代码之前,记住这一点:
"我们应该忘记小的效率,过早的优化是一切罪恶的根源,有 97% 的案例如此。"——Donald Knuth
换句话说,先用 Python 编写程序,然后看它是否能够满足需要。大多数情况下,它的性能已经足够好了……但有时候真的觉得慢了,那就使用分析器找到瓶颈函数,然后用cython重写,很快就能够得到更高的性能。
外部链接
WorldMill(http://trac.gispython.org/projects/PCL/wiki/WorldMill)——由Sean Gillies 用 Cython 编写的一个快速的,提供简洁的 python 接口的模块,封装了用以处理矢量地理空间数据的 libgdal 库。
编写更快的 Pyrex 代码(http://www.sagemath.org:9001/WritingFastPyrexCode)——Pyrex,是 Cython 的前身,它们有类似的目标和语法。
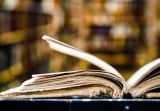
This tutorial demonstrates how to use Python to process the statistical concept of Zipf's law and demonstrates the efficiency of Python's reading and sorting large text files when processing the law. You may be wondering what the term Zipf distribution means. To understand this term, we first need to define Zipf's law. Don't worry, I'll try to simplify the instructions. Zipf's Law Zipf's law simply means: in a large natural language corpus, the most frequently occurring words appear about twice as frequently as the second frequent words, three times as the third frequent words, four times as the fourth frequent words, and so on. Let's look at an example. If you look at the Brown corpus in American English, you will notice that the most frequent word is "th
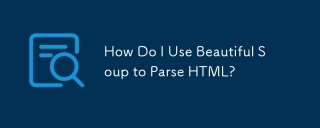
This article explains how to use Beautiful Soup, a Python library, to parse HTML. It details common methods like find(), find_all(), select(), and get_text() for data extraction, handling of diverse HTML structures and errors, and alternatives (Sel
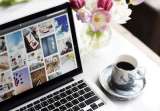
Dealing with noisy images is a common problem, especially with mobile phone or low-resolution camera photos. This tutorial explores image filtering techniques in Python using OpenCV to tackle this issue. Image Filtering: A Powerful Tool Image filter
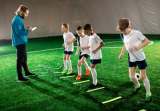
Python, a favorite for data science and processing, offers a rich ecosystem for high-performance computing. However, parallel programming in Python presents unique challenges. This tutorial explores these challenges, focusing on the Global Interprete
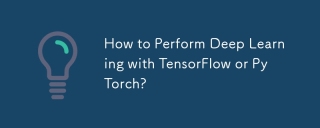
This article compares TensorFlow and PyTorch for deep learning. It details the steps involved: data preparation, model building, training, evaluation, and deployment. Key differences between the frameworks, particularly regarding computational grap
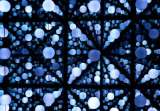
This tutorial demonstrates creating a custom pipeline data structure in Python 3, leveraging classes and operator overloading for enhanced functionality. The pipeline's flexibility lies in its ability to apply a series of functions to a data set, ge
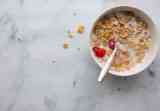
Serialization and deserialization of Python objects are key aspects of any non-trivial program. If you save something to a Python file, you do object serialization and deserialization if you read the configuration file, or if you respond to an HTTP request. In a sense, serialization and deserialization are the most boring things in the world. Who cares about all these formats and protocols? You want to persist or stream some Python objects and retrieve them in full at a later time. This is a great way to see the world on a conceptual level. However, on a practical level, the serialization scheme, format or protocol you choose may determine the speed, security, freedom of maintenance status, and other aspects of the program
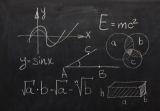
Python's statistics module provides powerful data statistical analysis capabilities to help us quickly understand the overall characteristics of data, such as biostatistics and business analysis. Instead of looking at data points one by one, just look at statistics such as mean or variance to discover trends and features in the original data that may be ignored, and compare large datasets more easily and effectively. This tutorial will explain how to calculate the mean and measure the degree of dispersion of the dataset. Unless otherwise stated, all functions in this module support the calculation of the mean() function instead of simply summing the average. Floating point numbers can also be used. import random import statistics from fracti


Hot AI Tools

Undresser.AI Undress
AI-powered app for creating realistic nude photos

AI Clothes Remover
Online AI tool for removing clothes from photos.

Undress AI Tool
Undress images for free

Clothoff.io
AI clothes remover

AI Hentai Generator
Generate AI Hentai for free.

Hot Article

Hot Tools

mPDF
mPDF is a PHP library that can generate PDF files from UTF-8 encoded HTML. The original author, Ian Back, wrote mPDF to output PDF files "on the fly" from his website and handle different languages. It is slower than original scripts like HTML2FPDF and produces larger files when using Unicode fonts, but supports CSS styles etc. and has a lot of enhancements. Supports almost all languages, including RTL (Arabic and Hebrew) and CJK (Chinese, Japanese and Korean). Supports nested block-level elements (such as P, DIV),

Notepad++7.3.1
Easy-to-use and free code editor

MinGW - Minimalist GNU for Windows
This project is in the process of being migrated to osdn.net/projects/mingw, you can continue to follow us there. MinGW: A native Windows port of the GNU Compiler Collection (GCC), freely distributable import libraries and header files for building native Windows applications; includes extensions to the MSVC runtime to support C99 functionality. All MinGW software can run on 64-bit Windows platforms.

Atom editor mac version download
The most popular open source editor

SublimeText3 Linux new version
SublimeText3 Linux latest version
