Python, a favorite for data science and processing, offers a rich ecosystem for high-performance computing. However, parallel programming in Python presents unique challenges. This tutorial explores these challenges, focusing on the Global Interpreter Lock (GIL), the differences between threads and processes, and the distinction between parallel and concurrent programming. We'll then build a practical example demonstrating these concepts.
The Global Interpreter Lock (GIL): A Python Parallelism Hurdle
The GIL, a mutex in CPython (the most common Python implementation), ensures thread safety. While beneficial for integrating with non-thread-safe libraries and speeding up non-parallel code, the GIL prevents true parallelism through multithreading. Only one native thread can execute Python bytecodes at a time.
However, operations outside the GIL's scope (like I/O-bound tasks) can run in parallel. This opens possibilities for parallel processing, especially when combined with libraries designed for computation-heavy tasks.
Threads vs. Processes: Choosing the Right Approach
Parallelism can be achieved using threads or processes. Threads are lightweight, sharing memory within a process, while processes are heavier, each with its own memory space.
-
Threads: Suitable for I/O-bound tasks where concurrency is sufficient. The GIL limits true parallelism, but threads can still improve performance by overlapping I/O operations.
-
Processes: Ideal for CPU-bound tasks requiring true parallelism. Multiple processes can utilize multiple CPU cores simultaneously, bypassing the GIL's limitations.
Parallel vs. Concurrent: Understanding the Nuances
Parallelism implies simultaneous execution of tasks, leveraging multiple cores. Concurrency, on the other hand, focuses on managing tasks to maximize efficiency, even without true simultaneous execution. Concurrency can improve performance by cleverly scheduling tasks, allowing I/O-bound operations to proceed while other tasks are performed.
A Practical Example: Comparing Techniques
The following code demonstrates serial, threaded, and process-based approaches to a computation-heavy task (crunch_numbers
), highlighting the performance differences:
import time import threading import multiprocessing NUM_WORKERS = 4 def crunch_numbers(): # Simulate a CPU-bound task for _ in range(10000000): pass # Replace with actual computation start_time = time.time() for _ in range(NUM_WORKERS): crunch_numbers() end_time = time.time() print("Serial time=", end_time - start_time) start_time = time.time() threads = [threading.Thread(target=crunch_numbers) for _ in range(NUM_WORKERS)] [thread.start() for thread in threads] [thread.join() for thread in threads] end_time = time.time() print("Threads time=", end_time - start_time) start_time = time.time() processes = [multiprocessing.Process(target=crunch_numbers) for _ in range(NUM_WORKERS)] [process.start() for process in processes] [process.join() for process in processes] end_time = time.time() print("Parallel time=", end_time - start_time)
The output will show a significant performance improvement with the multiprocessing approach due to true parallelism. The threaded approach might show little to no improvement because of the GIL.
Python's Parallel and Concurrent Programming Ecosystem
Python offers various libraries for parallel and concurrent programming:
-
_thread
: A low-level interface to OS threads. -
multiprocessing
: Provides a higher-level API for process management. -
concurrent.futures
: Offers a consistent interface for both threads and processes. -
gevent
: A coroutine-based library enabling efficient concurrency. -
Celery
: A distributed task queue ideal for complex, high-performance scenarios.
Remember: Processes offer true parallelism but are more resource-intensive. Threads are lighter but are limited by the GIL in Python. Choose the approach best suited to your task's nature (CPU-bound vs. I/O-bound) and performance requirements. Concurrency can often provide significant performance gains, even without true parallelism.
The above is the detailed content of Introduction to Parallel and Concurrent Programming in Python. For more information, please follow other related articles on the PHP Chinese website!
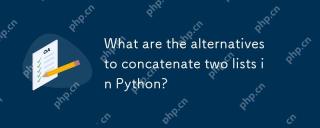
There are many methods to connect two lists in Python: 1. Use operators, which are simple but inefficient in large lists; 2. Use extend method, which is efficient but will modify the original list; 3. Use the = operator, which is both efficient and readable; 4. Use itertools.chain function, which is memory efficient but requires additional import; 5. Use list parsing, which is elegant but may be too complex. The selection method should be based on the code context and requirements.
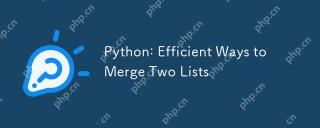
There are many ways to merge Python lists: 1. Use operators, which are simple but not memory efficient for large lists; 2. Use extend method, which is efficient but will modify the original list; 3. Use itertools.chain, which is suitable for large data sets; 4. Use * operator, merge small to medium-sized lists in one line of code; 5. Use numpy.concatenate, which is suitable for large data sets and scenarios with high performance requirements; 6. Use append method, which is suitable for small lists but is inefficient. When selecting a method, you need to consider the list size and application scenarios.
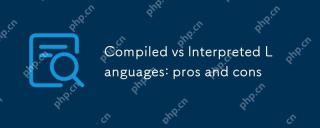
Compiledlanguagesofferspeedandsecurity,whileinterpretedlanguagesprovideeaseofuseandportability.1)CompiledlanguageslikeC arefasterandsecurebuthavelongerdevelopmentcyclesandplatformdependency.2)InterpretedlanguageslikePythonareeasiertouseandmoreportab
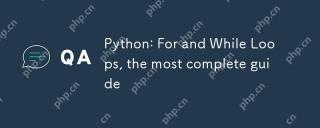
In Python, a for loop is used to traverse iterable objects, and a while loop is used to perform operations repeatedly when the condition is satisfied. 1) For loop example: traverse the list and print the elements. 2) While loop example: guess the number game until you guess it right. Mastering cycle principles and optimization techniques can improve code efficiency and reliability.
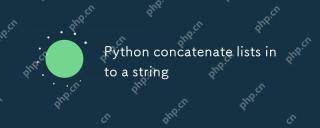
To concatenate a list into a string, using the join() method in Python is the best choice. 1) Use the join() method to concatenate the list elements into a string, such as ''.join(my_list). 2) For a list containing numbers, convert map(str, numbers) into a string before concatenating. 3) You can use generator expressions for complex formatting, such as ','.join(f'({fruit})'forfruitinfruits). 4) When processing mixed data types, use map(str, mixed_list) to ensure that all elements can be converted into strings. 5) For large lists, use ''.join(large_li
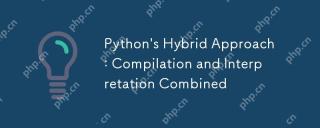
Pythonusesahybridapproach,combiningcompilationtobytecodeandinterpretation.1)Codeiscompiledtoplatform-independentbytecode.2)BytecodeisinterpretedbythePythonVirtualMachine,enhancingefficiencyandportability.
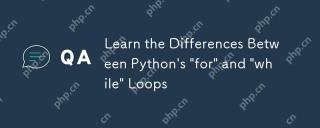
ThekeydifferencesbetweenPython's"for"and"while"loopsare:1)"For"loopsareidealforiteratingoversequencesorknowniterations,while2)"while"loopsarebetterforcontinuinguntilaconditionismetwithoutpredefinediterations.Un
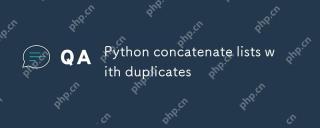
In Python, you can connect lists and manage duplicate elements through a variety of methods: 1) Use operators or extend() to retain all duplicate elements; 2) Convert to sets and then return to lists to remove all duplicate elements, but the original order will be lost; 3) Use loops or list comprehensions to combine sets to remove duplicate elements and maintain the original order.


Hot AI Tools

Undresser.AI Undress
AI-powered app for creating realistic nude photos

AI Clothes Remover
Online AI tool for removing clothes from photos.

Undress AI Tool
Undress images for free

Clothoff.io
AI clothes remover

Video Face Swap
Swap faces in any video effortlessly with our completely free AI face swap tool!

Hot Article

Hot Tools

Safe Exam Browser
Safe Exam Browser is a secure browser environment for taking online exams securely. This software turns any computer into a secure workstation. It controls access to any utility and prevents students from using unauthorized resources.
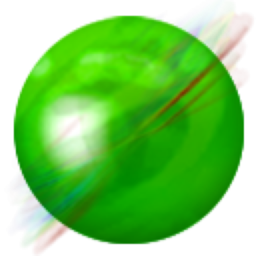
ZendStudio 13.5.1 Mac
Powerful PHP integrated development environment

SecLists
SecLists is the ultimate security tester's companion. It is a collection of various types of lists that are frequently used during security assessments, all in one place. SecLists helps make security testing more efficient and productive by conveniently providing all the lists a security tester might need. List types include usernames, passwords, URLs, fuzzing payloads, sensitive data patterns, web shells, and more. The tester can simply pull this repository onto a new test machine and he will have access to every type of list he needs.

PhpStorm Mac version
The latest (2018.2.1) professional PHP integrated development tool

MinGW - Minimalist GNU for Windows
This project is in the process of being migrated to osdn.net/projects/mingw, you can continue to follow us there. MinGW: A native Windows port of the GNU Compiler Collection (GCC), freely distributable import libraries and header files for building native Windows applications; includes extensions to the MSVC runtime to support C99 functionality. All MinGW software can run on 64-bit Windows platforms.
