NumPy manages memory for large arrays efficiently using views, copies, and memory-mapped files. 1) Views allow slicing without copying, directly modifying the original array. 2) Copies can be created with the copy() method for preserving data. 3) Memory-mapped files handle massive datasets by treating disk files as in-memory arrays.
When it comes to handling large arrays, NumPy's memory management is a fascinating topic that can make or break your data processing tasks. Let's dive into how NumPy manages memory for those hefty arrays and explore some practical insights and examples.
NumPy's approach to memory management is both efficient and user-friendly, especially when you're dealing with arrays that could make your system sweat. At its core, NumPy uses a concept called "views" rather than copies, which is a game-changer for performance. Imagine you've got a giant dataset; NumPy lets you slice and dice it without creating new copies, saving both memory and time.
Let's look at how this works in practice:
import numpy as np # Create a large array big_array = np.arange(1000000) # Create a view of the array view = big_array[::2] # Modify the view view[0] = 999 # Check the original array print(big_array[0]) # Output: 999
In this example, view
is not a new array but a view into big_array
. When we modify view
, we're directly altering big_array
. This is a powerful feature, but it's also a double-edged sword. If you're not careful, you might end up modifying data you didn't intend to change.
Now, let's talk about when NumPy does create copies. If you want to ensure you're working with a separate copy, you can use the copy()
method:
# Create a copy of the array copy = big_array.copy() # Modify the copy copy[0] = 888 # Check the original array print(big_array[0]) # Output: 999
This is crucial when you need to preserve the original data. However, creating copies can be memory-intensive, so use it wisely.
Another aspect of NumPy's memory management is its use of contiguous memory blocks. This is particularly important for large arrays because it allows for faster access and manipulation. When you create an array, NumPy tries to allocate memory in a single, contiguous block, which can significantly improve performance.
But what happens when you're dealing with truly massive datasets that don't fit into memory? NumPy has you covered with memory-mapped files. These allow you to work with files on disk as if they were in memory, which is a lifesaver for big data:
# Create a memory-mapped file fp = np.memmap('memmapped.dat', dtype='float32', mode='w ', shape=(1000000,)) # Use it like a regular array fp[:] = np.arange(1000000, dtype='float32') # Flush changes to disk fp.flush() # Later, you can reopen it fp = np.memmap('memmapped.dat', dtype='float32', mode='r ', shape=(1000000,)) print(fp[:10]) # Output: [0. 1. 2. 3. 4. 5. 6. 7. 8. 9.]
This approach is fantastic for handling datasets that are too large for your RAM, but it comes with its own set of challenges. For instance, operations on memory-mapped files can be slower than in-memory operations, and you need to be mindful of file locking and concurrent access.
In terms of performance optimization, one of the key strategies is to minimize the creation of intermediate arrays. NumPy's vectorized operations are designed to work directly on the data without creating unnecessary copies. Here's an example of how to optimize a simple operation:
# Inefficient way result = np.zeros_like(big_array) for i in range(len(big_array)): result[i] = big_array[i] * 2 # Efficient way result = big_array * 2
The second approach is not only more concise but also much faster because it avoids creating an intermediate array.
When it comes to best practices, always consider the size of your arrays and the operations you're performing. If you're working with large datasets, think about whether you can use views instead of copies, and consider using memory-mapped files for truly massive datasets. Also, keep an eye on your system's memory usage; NumPy can be a memory hog if you're not careful.
In my experience, one of the common pitfalls is underestimating the memory requirements of your operations. I once worked on a project where we were processing satellite imagery, and our initial approach led to out-of-memory errors. By switching to memory-mapped files and optimizing our operations, we were able to handle the data without crashing the system.
To wrap up, NumPy's memory management for large arrays is a powerful tool that, when used correctly, can handle even the most demanding data processing tasks. Just remember to use views wisely, create copies only when necessary, and leverage memory-mapped files for those truly massive datasets. With these strategies in mind, you'll be well-equipped to tackle any data challenge that comes your way.
The above is the detailed content of How does NumPy handle memory management for large arrays?. For more information, please follow other related articles on the PHP Chinese website!
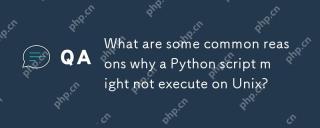
The reasons why Python scripts cannot run on Unix systems include: 1) Insufficient permissions, using chmod xyour_script.py to grant execution permissions; 2) Shebang line is incorrect or missing, you should use #!/usr/bin/envpython; 3) The environment variables are not set properly, and you can print os.environ debugging; 4) Using the wrong Python version, you can specify the version on the Shebang line or the command line; 5) Dependency problems, using virtual environment to isolate dependencies; 6) Syntax errors, using python-mpy_compileyour_script.py to detect.
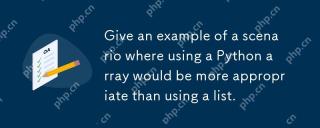
Using Python arrays is more suitable for processing large amounts of numerical data than lists. 1) Arrays save more memory, 2) Arrays are faster to operate by numerical values, 3) Arrays force type consistency, 4) Arrays are compatible with C arrays, but are not as flexible and convenient as lists.
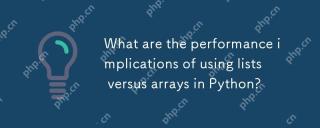
Listsare Better ForeflexibilityandMixdatatatypes, Whilearraysares Superior Sumerical Computation Sand Larged Datasets.1) Unselable List Xibility, MixedDatatypes, andfrequent elementchanges.2) Usarray's sensory -sensical operations, Largedatasets, AndwhenMemoryEfficiency
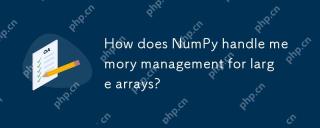
NumPymanagesmemoryforlargearraysefficientlyusingviews,copies,andmemory-mappedfiles.1)Viewsallowslicingwithoutcopying,directlymodifyingtheoriginalarray.2)Copiescanbecreatedwiththecopy()methodforpreservingdata.3)Memory-mappedfileshandlemassivedatasetsb
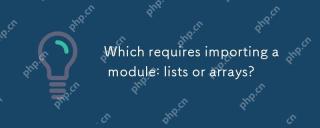
ListsinPythondonotrequireimportingamodule,whilearraysfromthearraymoduledoneedanimport.1)Listsarebuilt-in,versatile,andcanholdmixeddatatypes.2)Arraysaremorememory-efficientfornumericdatabutlessflexible,requiringallelementstobeofthesametype.
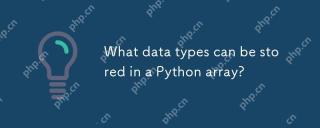
Pythonlistscanstoreanydatatype,arraymodulearraysstoreonetype,andNumPyarraysarefornumericalcomputations.1)Listsareversatilebutlessmemory-efficient.2)Arraymodulearraysarememory-efficientforhomogeneousdata.3)NumPyarraysareoptimizedforperformanceinscient
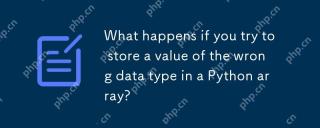
WhenyouattempttostoreavalueofthewrongdatatypeinaPythonarray,you'llencounteraTypeError.Thisisduetothearraymodule'sstricttypeenforcement,whichrequiresallelementstobeofthesametypeasspecifiedbythetypecode.Forperformancereasons,arraysaremoreefficientthanl
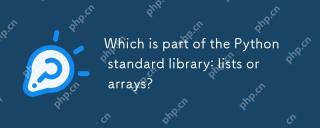
Pythonlistsarepartofthestandardlibrary,whilearraysarenot.Listsarebuilt-in,versatile,andusedforstoringcollections,whereasarraysareprovidedbythearraymoduleandlesscommonlyusedduetolimitedfunctionality.


Hot AI Tools

Undresser.AI Undress
AI-powered app for creating realistic nude photos

AI Clothes Remover
Online AI tool for removing clothes from photos.

Undress AI Tool
Undress images for free

Clothoff.io
AI clothes remover

Video Face Swap
Swap faces in any video effortlessly with our completely free AI face swap tool!

Hot Article

Hot Tools

mPDF
mPDF is a PHP library that can generate PDF files from UTF-8 encoded HTML. The original author, Ian Back, wrote mPDF to output PDF files "on the fly" from his website and handle different languages. It is slower than original scripts like HTML2FPDF and produces larger files when using Unicode fonts, but supports CSS styles etc. and has a lot of enhancements. Supports almost all languages, including RTL (Arabic and Hebrew) and CJK (Chinese, Japanese and Korean). Supports nested block-level elements (such as P, DIV),
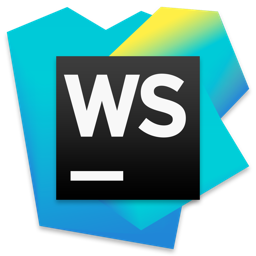
WebStorm Mac version
Useful JavaScript development tools

SAP NetWeaver Server Adapter for Eclipse
Integrate Eclipse with SAP NetWeaver application server.

Notepad++7.3.1
Easy-to-use and free code editor
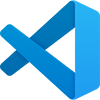
VSCode Windows 64-bit Download
A free and powerful IDE editor launched by Microsoft
