Lists in Python do not require importing a module, while arrays from the array module do need an import. 1) Lists are built-in, versatile, and can hold mixed data types. 2) Arrays are more memory-efficient for numeric data but less flexible, requiring all elements to be of the same type.
In Python, neither lists nor arrays inherently require importing a module to use them, but arrays do need an import if you're referring to the array
module. Let's dive into this and explore more about lists and arrays in Python.
Lists in Python are built-in data structures, meaning you don't need to import any module to use them. They're versatile, can hold elements of different types, and are dynamically sized. Here's a quick example of using a list:
# Creating a list my_list = [1, 2, 3, 'four', 5.0] # Accessing elements print(my_list[0]) # Output: 1 print(my_list[3]) # Output: four
On the other hand, if you're talking about arrays in Python, you might be referring to the array
module. This module provides an array
type that is more memory-efficient than lists for storing large amounts of numeric data. To use this, you need to import the array
module:
import array # Creating an array of integers my_array = array.array('i', [1, 2, 3, 4, 5]) # Accessing elements print(my_array[0]) # Output: 1 print(my_array[3]) # Output: 4
The array
module is particularly useful when you're dealing with large datasets of a single type, as it can be more efficient in terms of memory usage. However, it's less flexible than lists because all elements must be of the same type.
Now, let's dive deeper into the nuances of using lists and arrays in Python, sharing some insights and experiences along the way.
When it comes to choosing between lists and arrays, it's often about balancing flexibility and performance. Lists are great for general-purpose programming because of their flexibility. You can mix and match different types of elements, and they're easy to work with. I've used lists extensively in projects where data types were unpredictable or mixed, and they've never let me down.
However, when I've worked on projects requiring large datasets of a single type, like scientific computing or data processing, arrays have been a game-changer. The memory efficiency of arrays can make a significant difference in performance. I once worked on a project analyzing large financial datasets, and switching from lists to arrays shaved off considerable processing time.
One thing to watch out for with arrays is their type constraint. If you try to add an element of a different type, you'll get a TypeError
. This can be both a blessing and a curse. It's great for maintaining data integrity, but it can be a pain if you're not careful. Here's an example of what can go wrong:
import array my_array = array.array('i', [1, 2, 3]) try: my_array.append('four') # This will raise a TypeError except TypeError as e: print(f"Error: {e}") # Output: Error: an integer is required (got type str)
In terms of performance, lists are generally slower than arrays for numeric data because of their flexibility. Lists need to store type information for each element, which can lead to higher memory usage and slower access times. Arrays, on the other hand, have a fixed type, which allows for more efficient memory usage and faster operations.
When optimizing code, it's worth considering whether you can use arrays instead of lists. Here's a quick comparison of performance:
import timeit import array # List performance list_time = timeit.timeit(''' my_list = [1, 2, 3, 4, 5] sum(my_list) ''', number=1000000) # Array performance array_time = timeit.timeit(''' import array my_array = array.array('i', [1, 2, 3, 4, 5]) sum(my_array) ''', number=1000000) print(f"List time: {list_time:.6f} seconds") print(f"Array time: {array_time:.6f} seconds")
You'll likely see that arrays are faster for this simple operation. However, the difference might be negligible for small datasets, and lists might be a better choice for their ease of use and flexibility.
In conclusion, while lists don't require importing a module and are highly flexible, arrays do need the array
module but offer better performance for numeric data. The choice between them depends on your specific needs, and understanding their strengths and weaknesses can help you write more efficient and effective Python code.
The above is the detailed content of Which requires importing a module: lists or arrays?. For more information, please follow other related articles on the PHP Chinese website!
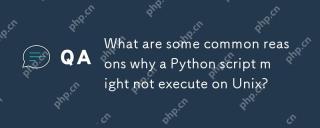
The reasons why Python scripts cannot run on Unix systems include: 1) Insufficient permissions, using chmod xyour_script.py to grant execution permissions; 2) Shebang line is incorrect or missing, you should use #!/usr/bin/envpython; 3) The environment variables are not set properly, and you can print os.environ debugging; 4) Using the wrong Python version, you can specify the version on the Shebang line or the command line; 5) Dependency problems, using virtual environment to isolate dependencies; 6) Syntax errors, using python-mpy_compileyour_script.py to detect.
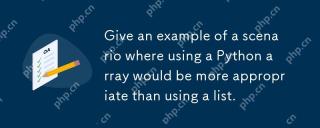
Using Python arrays is more suitable for processing large amounts of numerical data than lists. 1) Arrays save more memory, 2) Arrays are faster to operate by numerical values, 3) Arrays force type consistency, 4) Arrays are compatible with C arrays, but are not as flexible and convenient as lists.
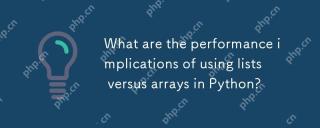
Listsare Better ForeflexibilityandMixdatatatypes, Whilearraysares Superior Sumerical Computation Sand Larged Datasets.1) Unselable List Xibility, MixedDatatypes, andfrequent elementchanges.2) Usarray's sensory -sensical operations, Largedatasets, AndwhenMemoryEfficiency
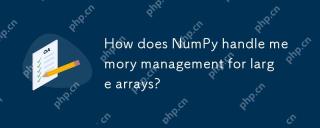
NumPymanagesmemoryforlargearraysefficientlyusingviews,copies,andmemory-mappedfiles.1)Viewsallowslicingwithoutcopying,directlymodifyingtheoriginalarray.2)Copiescanbecreatedwiththecopy()methodforpreservingdata.3)Memory-mappedfileshandlemassivedatasetsb
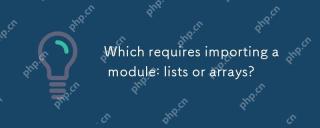
ListsinPythondonotrequireimportingamodule,whilearraysfromthearraymoduledoneedanimport.1)Listsarebuilt-in,versatile,andcanholdmixeddatatypes.2)Arraysaremorememory-efficientfornumericdatabutlessflexible,requiringallelementstobeofthesametype.
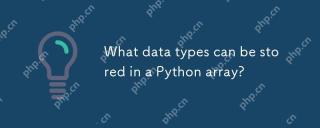
Pythonlistscanstoreanydatatype,arraymodulearraysstoreonetype,andNumPyarraysarefornumericalcomputations.1)Listsareversatilebutlessmemory-efficient.2)Arraymodulearraysarememory-efficientforhomogeneousdata.3)NumPyarraysareoptimizedforperformanceinscient
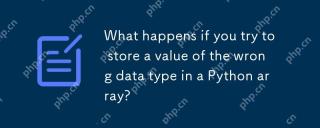
WhenyouattempttostoreavalueofthewrongdatatypeinaPythonarray,you'llencounteraTypeError.Thisisduetothearraymodule'sstricttypeenforcement,whichrequiresallelementstobeofthesametypeasspecifiedbythetypecode.Forperformancereasons,arraysaremoreefficientthanl
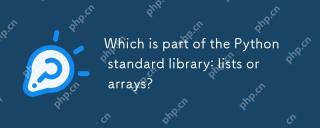
Pythonlistsarepartofthestandardlibrary,whilearraysarenot.Listsarebuilt-in,versatile,andusedforstoringcollections,whereasarraysareprovidedbythearraymoduleandlesscommonlyusedduetolimitedfunctionality.


Hot AI Tools

Undresser.AI Undress
AI-powered app for creating realistic nude photos

AI Clothes Remover
Online AI tool for removing clothes from photos.

Undress AI Tool
Undress images for free

Clothoff.io
AI clothes remover

Video Face Swap
Swap faces in any video effortlessly with our completely free AI face swap tool!

Hot Article

Hot Tools

mPDF
mPDF is a PHP library that can generate PDF files from UTF-8 encoded HTML. The original author, Ian Back, wrote mPDF to output PDF files "on the fly" from his website and handle different languages. It is slower than original scripts like HTML2FPDF and produces larger files when using Unicode fonts, but supports CSS styles etc. and has a lot of enhancements. Supports almost all languages, including RTL (Arabic and Hebrew) and CJK (Chinese, Japanese and Korean). Supports nested block-level elements (such as P, DIV),
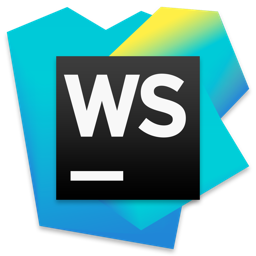
WebStorm Mac version
Useful JavaScript development tools

SAP NetWeaver Server Adapter for Eclipse
Integrate Eclipse with SAP NetWeaver application server.

Notepad++7.3.1
Easy-to-use and free code editor
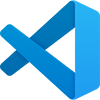
VSCode Windows 64-bit Download
A free and powerful IDE editor launched by Microsoft
