


How can you measure code coverage? What are some tools for code coverage analysis?
How can you measure code coverage? What are some tools for code coverage analysis?
Code coverage is a measure used to determine the extent to which the source code of a program is tested by a particular test suite. It is expressed as a percentage and calculated by determining the number of lines, statements, branches, or conditions that are executed during the test runs relative to the total number of such elements in the source code. Here are the main types of code coverage:
- Line Coverage: Measures whether each line of the source code has been executed.
- Statement Coverage: Measures whether each statement in the code has been executed. This is similar to line coverage but more precise in languages where a single line might contain multiple statements.
- Branch Coverage: Measures whether each branch (e.g., in if-else statements or switch cases) in the control flow has been executed.
- Path Coverage: Measures whether every possible path through a given part of the code has been executed.
- Condition Coverage: Measures whether each of the boolean sub-expressions evaluated to both true and false during testing.
Several tools are available for code coverage analysis, each designed to work with specific programming languages and development environments. Here are some notable tools:
- JaCoCo (Java): A popular code coverage tool for Java that can be used standalone or integrated into various build tools like Maven or Gradle.
- Istanbul (JavaScript): A JavaScript code coverage tool that can be used for both browser and Node.js applications.
- Coverage.py (Python): A tool for measuring code coverage of Python programs.
- gcov (C/C ): A test coverage program used in conjunction with GCC to analyze programs written in C and C .
- Visual Studio Code Coverage (C#, VB.NET): Integrated into Visual Studio, this tool helps in analyzing code coverage for .NET applications.
What are the benefits of using code coverage metrics in software development?
Using code coverage metrics in software development offers several benefits:
- Improved Test Quality: Code coverage metrics help in identifying areas of the code that are not adequately tested. This enables developers to write more comprehensive test cases, leading to better test quality and more robust software.
- Detection of Dead Code: By analyzing which parts of the code are never executed during testing, developers can identify and remove dead code, making the codebase cleaner and easier to maintain.
- Better Resource Allocation: Code coverage data helps in directing testing efforts more efficiently. Developers and testers can focus their resources on areas of the code that are critical or under-tested, ensuring that time and effort are used effectively.
- Compliance and Standards: In many industries, especially those that are heavily regulated such as finance and healthcare, meeting certain code coverage thresholds is a requirement. Code coverage metrics help in ensuring compliance with these standards.
- Confidence in Releases: Higher code coverage can increase confidence in the software's reliability and stability. When a high percentage of the code is tested, it is more likely that fewer bugs will be encountered in production.
How does code coverage impact the overall quality of a software project?
Code coverage has a significant impact on the overall quality of a software project in several ways:
- Reduction in Bugs: Higher code coverage means more of the code is tested, which can lead to the early detection and fixing of bugs. This results in a more stable and reliable software product.
- Enhanced Maintainability: When code coverage is high, it is easier to maintain the software. Developers can make changes with more confidence, knowing that the existing tests will catch any regressions introduced by their modifications.
- Improved Code Quality: The process of achieving high code coverage often leads to better code design. Developers may refactor code to make it more testable, which can result in cleaner, more modular, and more maintainable code.
- Better Risk Management: By knowing which parts of the code are well-tested and which are not, project managers can better assess and manage the risks associated with releasing the software. Areas with low coverage can be prioritized for additional testing or refactoring.
- Customer Satisfaction: Ultimately, higher code coverage can lead to fewer defects in the released software, which translates to higher customer satisfaction and a better reputation for the development team or company.
Can you recommend any open-source tools specifically designed for code coverage analysis?
Here are some open-source tools specifically designed for code coverage analysis:
- JaCoCo (Java): JaCoCo is an open-source code coverage library for Java. It is widely used and can be integrated with various build tools like Maven and Gradle. It provides detailed reports on line, branch, and method coverage.
- Istanbul (JavaScript): Istanbul is an open-source JavaScript code coverage tool that works with both browser and Node.js applications. It can be used standalone or integrated with testing frameworks like Mocha or Jest.
- Coverage.py (Python): Coverage.py is a popular open-source tool for measuring code coverage in Python. It supports various output formats and can be integrated with testing frameworks like pytest and unittest.
- gcov (C/C ): gcov is an open-source test coverage program that comes with the GNU Compiler Collection (GCC). It is used to analyze programs written in C and C and provides detailed coverage information.
- OpenCppCoverage (C ): OpenCppCoverage is an open-source code coverage tool for C that works on Windows. It can be integrated with Visual Studio and provides detailed reports on line and branch coverage.
These tools are widely used in the software development community and can help developers achieve high code coverage and improve the quality of their software projects.
The above is the detailed content of How can you measure code coverage? What are some tools for code coverage analysis?. For more information, please follow other related articles on the PHP Chinese website!
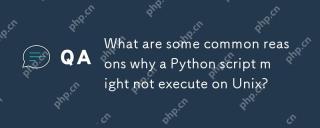
The reasons why Python scripts cannot run on Unix systems include: 1) Insufficient permissions, using chmod xyour_script.py to grant execution permissions; 2) Shebang line is incorrect or missing, you should use #!/usr/bin/envpython; 3) The environment variables are not set properly, and you can print os.environ debugging; 4) Using the wrong Python version, you can specify the version on the Shebang line or the command line; 5) Dependency problems, using virtual environment to isolate dependencies; 6) Syntax errors, using python-mpy_compileyour_script.py to detect.
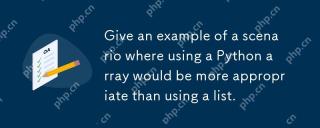
Using Python arrays is more suitable for processing large amounts of numerical data than lists. 1) Arrays save more memory, 2) Arrays are faster to operate by numerical values, 3) Arrays force type consistency, 4) Arrays are compatible with C arrays, but are not as flexible and convenient as lists.
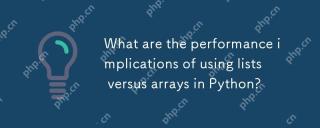
Listsare Better ForeflexibilityandMixdatatatypes, Whilearraysares Superior Sumerical Computation Sand Larged Datasets.1) Unselable List Xibility, MixedDatatypes, andfrequent elementchanges.2) Usarray's sensory -sensical operations, Largedatasets, AndwhenMemoryEfficiency
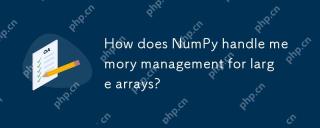
NumPymanagesmemoryforlargearraysefficientlyusingviews,copies,andmemory-mappedfiles.1)Viewsallowslicingwithoutcopying,directlymodifyingtheoriginalarray.2)Copiescanbecreatedwiththecopy()methodforpreservingdata.3)Memory-mappedfileshandlemassivedatasetsb
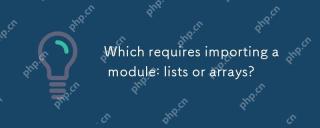
ListsinPythondonotrequireimportingamodule,whilearraysfromthearraymoduledoneedanimport.1)Listsarebuilt-in,versatile,andcanholdmixeddatatypes.2)Arraysaremorememory-efficientfornumericdatabutlessflexible,requiringallelementstobeofthesametype.
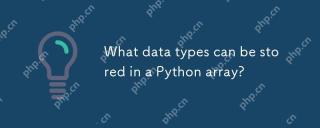
Pythonlistscanstoreanydatatype,arraymodulearraysstoreonetype,andNumPyarraysarefornumericalcomputations.1)Listsareversatilebutlessmemory-efficient.2)Arraymodulearraysarememory-efficientforhomogeneousdata.3)NumPyarraysareoptimizedforperformanceinscient
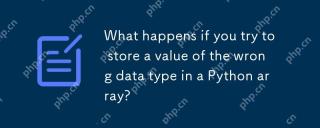
WhenyouattempttostoreavalueofthewrongdatatypeinaPythonarray,you'llencounteraTypeError.Thisisduetothearraymodule'sstricttypeenforcement,whichrequiresallelementstobeofthesametypeasspecifiedbythetypecode.Forperformancereasons,arraysaremoreefficientthanl
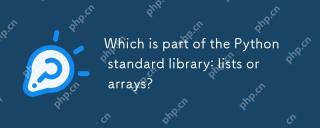
Pythonlistsarepartofthestandardlibrary,whilearraysarenot.Listsarebuilt-in,versatile,andusedforstoringcollections,whereasarraysareprovidedbythearraymoduleandlesscommonlyusedduetolimitedfunctionality.


Hot AI Tools

Undresser.AI Undress
AI-powered app for creating realistic nude photos

AI Clothes Remover
Online AI tool for removing clothes from photos.

Undress AI Tool
Undress images for free

Clothoff.io
AI clothes remover

Video Face Swap
Swap faces in any video effortlessly with our completely free AI face swap tool!

Hot Article

Hot Tools

Notepad++7.3.1
Easy-to-use and free code editor
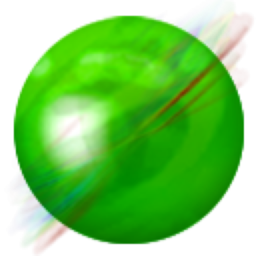
ZendStudio 13.5.1 Mac
Powerful PHP integrated development environment

SublimeText3 Chinese version
Chinese version, very easy to use
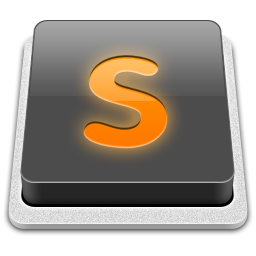
SublimeText3 Mac version
God-level code editing software (SublimeText3)

SublimeText3 Linux new version
SublimeText3 Linux latest version
