What is multithreading in Python?
Multithreading in Python refers to the concurrent execution of multiple threads within the same Python program. A thread is a lightweight process that can execute independently while sharing the same resources as the main program, such as memory space. In Python, multithreading is implemented using the threading
module, which allows developers to create and manage threads easily.
The primary advantage of multithreading is that it allows a program to perform multiple tasks simultaneously, which can lead to improved performance, particularly in applications that involve I/O operations or other tasks where waiting time can be utilized by other threads. Python's Global Interpreter Lock (GIL) does affect true parallelism when using multiple threads for CPU-bound tasks, but it remains beneficial for I/O-bound operations.
How can multithreading improve the performance of Python applications?
Multithreading can significantly enhance the performance of Python applications, particularly in the following ways:
- I/O-bound Operations: Multithreading is especially useful for applications that perform a lot of I/O operations, such as reading/writing files, network communications, or database queries. While one thread is waiting for an I/O operation to complete, other threads can continue executing, thereby making better use of the CPU.
- Concurrent Processing: Applications that need to perform multiple independent tasks can benefit from multithreading by allowing these tasks to run concurrently. This is particularly useful in applications like web servers where handling multiple client requests simultaneously is crucial.
- Responsiveness: Multithreading can improve the responsiveness of applications by allowing background tasks to run without blocking the main thread. For example, a GUI application can remain responsive to user inputs while performing background operations like data processing.
- Resource Sharing: Threads within the same process can easily share data and resources, which can lead to more efficient use of memory and other system resources.
While multithreading can improve performance in many scenarios, it's important to understand that due to Python's GIL, true parallelism in CPU-bound tasks is limited. For CPU-bound operations, other techniques like multiprocessing might be more effective.
What are the key challenges when implementing multithreading in Python?
Implementing multithreading in Python comes with several challenges:
- Global Interpreter Lock (GIL): The GIL is a mutex that protects access to Python objects, preventing multiple threads from executing Python bytecodes at once. This limits the effectiveness of multithreading for CPU-bound tasks, as only one thread can execute at a time.
- Race Conditions: When multiple threads access shared resources simultaneously, they can lead to race conditions, where the outcome depends on the relative timing of the threads. This can result in unpredictable behavior and bugs that are difficult to reproduce and debug.
- Deadlocks: A deadlock occurs when two or more threads are unable to proceed because each is waiting for the other to release a resource. Deadlocks can be challenging to identify and resolve.
- Complexity in Debugging: Multithreaded programs can be much harder to debug than single-threaded programs. Issues like race conditions and deadlocks may only appear intermittently, making them difficult to trace.
- Thread Safety: Ensuring that data and functions are thread-safe can be challenging. Improperly synchronized access to shared resources can lead to data corruption and other concurrency issues.
- Overhead: Creating and managing threads incurs overhead, and too many threads can lead to context switching and performance degradation.
What are some best practices for using multithreading effectively in Python?
To use multithreading effectively in Python, consider the following best practices:
- Use Threading for I/O-bound Tasks: Given the limitations of the GIL, use threading for tasks that involve waiting on I/O operations. This can significantly improve the responsiveness and efficiency of your application.
- Avoid Sharing Mutable State: To minimize the risk of race conditions, avoid sharing mutable state between threads whenever possible. If sharing is necessary, use thread-safe data structures and synchronization primitives like locks or semaphores.
-
Use Thread Pools: Instead of creating a new thread for each task, use a thread pool to manage a fixed number of threads. This can help reduce the overhead associated with thread creation and management. Python's
concurrent.futures
module provides a high-level interface for working with thread pools. -
Implement Proper Synchronization: Use synchronization primitives such as
Lock
,RLock
,Semaphore
, andCondition
to manage access to shared resources and prevent race conditions and deadlocks. - Avoid Deep Nesting: Deeply nested thread hierarchies can be difficult to manage and debug. Try to keep your thread structures as flat as possible.
- Test Thoroughly: Multithreaded applications can exhibit unpredictable behavior, so thorough testing is crucial. Use testing frameworks and consider stress testing to identify concurrency issues.
-
Consider Alternatives for CPU-bound Tasks: For CPU-bound tasks, consider using multiprocessing instead of multithreading. Python's
multiprocessing
module allows you to bypass the GIL and achieve true parallelism.
By following these best practices, you can maximize the benefits of multithreading in your Python applications while minimizing the associated challenges.
The above is the detailed content of What is multithreading in Python?. For more information, please follow other related articles on the PHP Chinese website!
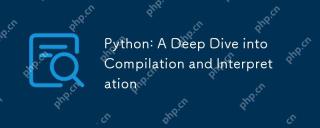
Pythonusesahybridmodelofcompilationandinterpretation:1)ThePythoninterpretercompilessourcecodeintoplatform-independentbytecode.2)ThePythonVirtualMachine(PVM)thenexecutesthisbytecode,balancingeaseofusewithperformance.
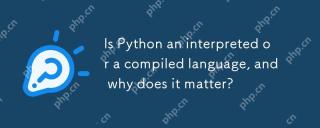
Pythonisbothinterpretedandcompiled.1)It'scompiledtobytecodeforportabilityacrossplatforms.2)Thebytecodeistheninterpreted,allowingfordynamictypingandrapiddevelopment,thoughitmaybeslowerthanfullycompiledlanguages.
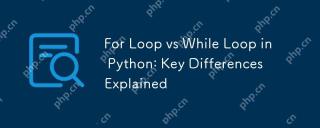
Forloopsareidealwhenyouknowthenumberofiterationsinadvance,whilewhileloopsarebetterforsituationswhereyouneedtoloopuntilaconditionismet.Forloopsaremoreefficientandreadable,suitableforiteratingoversequences,whereaswhileloopsoffermorecontrolandareusefulf
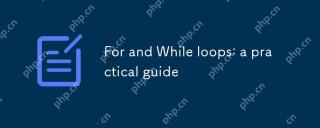
Forloopsareusedwhenthenumberofiterationsisknowninadvance,whilewhileloopsareusedwhentheiterationsdependonacondition.1)Forloopsareidealforiteratingoversequenceslikelistsorarrays.2)Whileloopsaresuitableforscenarioswheretheloopcontinuesuntilaspecificcond
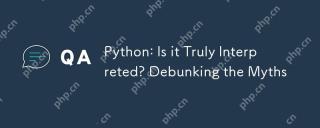
Pythonisnotpurelyinterpreted;itusesahybridapproachofbytecodecompilationandruntimeinterpretation.1)Pythoncompilessourcecodeintobytecode,whichisthenexecutedbythePythonVirtualMachine(PVM).2)Thisprocessallowsforrapiddevelopmentbutcanimpactperformance,req
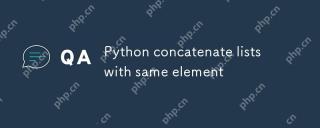
ToconcatenatelistsinPythonwiththesameelements,use:1)the operatortokeepduplicates,2)asettoremoveduplicates,or3)listcomprehensionforcontroloverduplicates,eachmethodhasdifferentperformanceandorderimplications.
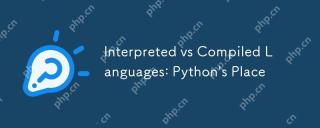
Pythonisaninterpretedlanguage,offeringeaseofuseandflexibilitybutfacingperformancelimitationsincriticalapplications.1)InterpretedlanguageslikePythonexecuteline-by-line,allowingimmediatefeedbackandrapidprototyping.2)CompiledlanguageslikeC/C transformt
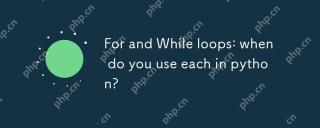
Useforloopswhenthenumberofiterationsisknowninadvance,andwhileloopswheniterationsdependonacondition.1)Forloopsareidealforsequenceslikelistsorranges.2)Whileloopssuitscenarioswheretheloopcontinuesuntilaspecificconditionismet,usefulforuserinputsoralgorit


Hot AI Tools

Undresser.AI Undress
AI-powered app for creating realistic nude photos

AI Clothes Remover
Online AI tool for removing clothes from photos.

Undress AI Tool
Undress images for free

Clothoff.io
AI clothes remover

Video Face Swap
Swap faces in any video effortlessly with our completely free AI face swap tool!

Hot Article

Hot Tools

SublimeText3 English version
Recommended: Win version, supports code prompts!

Zend Studio 13.0.1
Powerful PHP integrated development environment

SecLists
SecLists is the ultimate security tester's companion. It is a collection of various types of lists that are frequently used during security assessments, all in one place. SecLists helps make security testing more efficient and productive by conveniently providing all the lists a security tester might need. List types include usernames, passwords, URLs, fuzzing payloads, sensitive data patterns, web shells, and more. The tester can simply pull this repository onto a new test machine and he will have access to every type of list he needs.

MantisBT
Mantis is an easy-to-deploy web-based defect tracking tool designed to aid in product defect tracking. It requires PHP, MySQL and a web server. Check out our demo and hosting services.
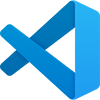
VSCode Windows 64-bit Download
A free and powerful IDE editor launched by Microsoft
