Introduction
Managing JDBC connections and JVM settings can be tedious for Python applications, especially when using databases such as Informix. This is where wbjdbc comes into play, a Python library designed to simplify these tasks. It automatically configures the environment so you can focus on the most important part: interacting with the data.
This article will guide you through the key features of wbjdbc, including how to automate connection setup, and provide practical examples for automating routine tasks.
What is wbjdbc?
wbjdbc is a Python library that simplifies JDBC and JVM setup, making it easy to connect to databases such as Informix. Its main functions include:
- Simplified JVM initialization: Automate JVM setup, including finding and loading jvm.dll.
-
Built-in JDBC driver support:
- Informix JDBC Driver (jdbc-4.50.10.1.jar)
- MongoDB BSON driver (bson-3.8.0.jar)
- Precompiled dependencies: Ensure compatibility and avoid common pitfalls.
- Lightweight and easy to install.
Install
To get started, install wbjdbc via pip:
pip install wbjdbc
Automated Informix database connection
This is a simple automation example using wbjdbc to connect to an Informix database and execute queries.
Example: Automated data retrieval tasks
from wbjdbc import start_jvm import jaydebeapi # 初始化JVM def initialize_environment(): start_jvm() print("JVM已初始化,驱动程序已加载。") # 连接到数据库 def connect_to_informix(): jdbc_url = "jdbc:informix-sqli://<host>:<port>/<database>:INFORMIXSERVER=<server_name>" user = "your_username" password = "your_password" print("正在建立数据库连接...") conn = jaydebeapi.connect("com.informix.jdbc.IfxDriver", jdbc_url, [user, password]) print("连接成功。") return conn # 自动化查询任务 def automate_query(): conn = connect_to_informix() cursor = conn.cursor() try: query = "SELECT * FROM customer WHERE active = 1" print("正在执行查询:", query) cursor.execute(query) results = cursor.fetchall() print("结果:") for row in results: print(row) finally: cursor.close() conn.close() print("数据库连接已关闭。") # 主要自动化工作流程 def main(): initialize_environment() automate_query() if __name__ == "__main__": main() </server_name></database></port></host>
Key points
-
JVM Initialization:
start_jvm()
Make sure the JVM and driver are set up correctly. - Connection Automation: Hides the complexity of configuring JDBC URLs and credentials.
- Query Execution: Automate routine queries so you can easily retrieve and process data programmatically.
Why choose wbjdbc?
- Save time: Eliminates manual setup and reduces boilerplate code.
- Reduce errors: Precompiled drivers minimize compatibility issues.
- Focus on automation: Ideal for tasks that require frequent database interaction.
Conclusion
wbjdbc changes the way Python developers interact with Informix databases, automating the tedious setup process and enabling efficient data operations. Whether you are running simple queries or building complex workflows, wbjdbc can meet your needs.
Ready to streamline your Informix database workflow? Install wbjdbc today and start automating your database tasks!
Resources
- wbjdbc on PyPI
- wbjdbc on GitHub
Feedback or questions? Share your thoughts in the comments below!
The above is the detailed content of Simplify Python-Informix Connections with wbjdbc. For more information, please follow other related articles on the PHP Chinese website!
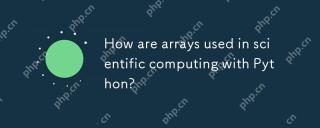
ArraysinPython,especiallyviaNumPy,arecrucialinscientificcomputingfortheirefficiencyandversatility.1)Theyareusedfornumericaloperations,dataanalysis,andmachinelearning.2)NumPy'simplementationinCensuresfasteroperationsthanPythonlists.3)Arraysenablequick
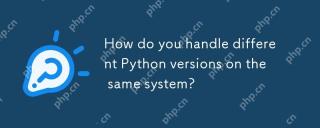
You can manage different Python versions by using pyenv, venv and Anaconda. 1) Use pyenv to manage multiple Python versions: install pyenv, set global and local versions. 2) Use venv to create a virtual environment to isolate project dependencies. 3) Use Anaconda to manage Python versions in your data science project. 4) Keep the system Python for system-level tasks. Through these tools and strategies, you can effectively manage different versions of Python to ensure the smooth running of the project.
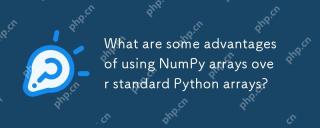
NumPyarrayshaveseveraladvantagesoverstandardPythonarrays:1)TheyaremuchfasterduetoC-basedimplementation,2)Theyaremorememory-efficient,especiallywithlargedatasets,and3)Theyofferoptimized,vectorizedfunctionsformathematicalandstatisticaloperations,making
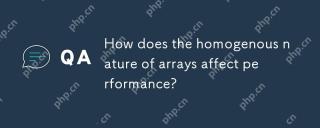
The impact of homogeneity of arrays on performance is dual: 1) Homogeneity allows the compiler to optimize memory access and improve performance; 2) but limits type diversity, which may lead to inefficiency. In short, choosing the right data structure is crucial.
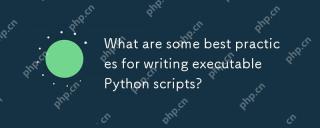
TocraftexecutablePythonscripts,followthesebestpractices:1)Addashebangline(#!/usr/bin/envpython3)tomakethescriptexecutable.2)Setpermissionswithchmod xyour_script.py.3)Organizewithacleardocstringanduseifname=="__main__":formainfunctionality.4
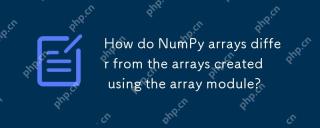
NumPyarraysarebetterfornumericaloperationsandmulti-dimensionaldata,whilethearraymoduleissuitableforbasic,memory-efficientarrays.1)NumPyexcelsinperformanceandfunctionalityforlargedatasetsandcomplexoperations.2)Thearraymoduleismorememory-efficientandfa
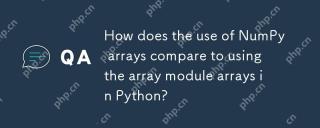
NumPyarraysarebetterforheavynumericalcomputing,whilethearraymoduleismoresuitableformemory-constrainedprojectswithsimpledatatypes.1)NumPyarraysofferversatilityandperformanceforlargedatasetsandcomplexoperations.2)Thearraymoduleislightweightandmemory-ef
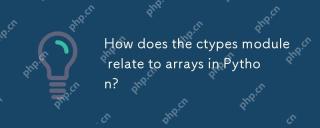
ctypesallowscreatingandmanipulatingC-stylearraysinPython.1)UsectypestointerfacewithClibrariesforperformance.2)CreateC-stylearraysfornumericalcomputations.3)PassarraystoCfunctionsforefficientoperations.However,becautiousofmemorymanagement,performanceo


Hot AI Tools

Undresser.AI Undress
AI-powered app for creating realistic nude photos

AI Clothes Remover
Online AI tool for removing clothes from photos.

Undress AI Tool
Undress images for free

Clothoff.io
AI clothes remover

Video Face Swap
Swap faces in any video effortlessly with our completely free AI face swap tool!

Hot Article

Hot Tools

PhpStorm Mac version
The latest (2018.2.1) professional PHP integrated development tool

Atom editor mac version download
The most popular open source editor
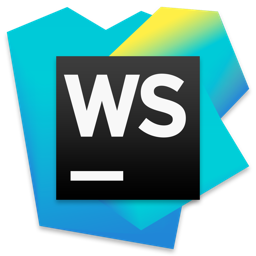
WebStorm Mac version
Useful JavaScript development tools

SecLists
SecLists is the ultimate security tester's companion. It is a collection of various types of lists that are frequently used during security assessments, all in one place. SecLists helps make security testing more efficient and productive by conveniently providing all the lists a security tester might need. List types include usernames, passwords, URLs, fuzzing payloads, sensitive data patterns, web shells, and more. The tester can simply pull this repository onto a new test machine and he will have access to every type of list he needs.
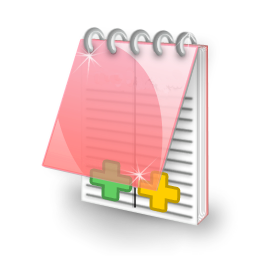
EditPlus Chinese cracked version
Small size, syntax highlighting, does not support code prompt function
