Dropping Rows with Duplicate Values in Multiple Columns Using Python Pandas
The pandas drop_duplicates function is a powerful tool for removing duplicate rows from a DataFrame, but what if you only want to drop rows that are duplicates across a subset of columns?
Example
Consider the following DataFrame:
A | B | C |
---|---|---|
foo | 0 | A |
foo | 1 | A |
foo | 1 | B |
bar | 1 | A |
Suppose you want to drop rows that match on columns A and C. In this case, you would want to drop rows 0 and 1.
Using drop_duplicates with the keep Parameter
To achieve this, you can use the drop_duplicates function with the keep parameter set to False. This parameter specifies how to handle duplicate rows. By default, keep is set to first, which means that the first occurrence of a duplicate row will be kept. Setting keep to False will drop all duplicate rows.
The following code demonstrates how to drop rows with duplicate values in columns A and C:
import pandas as pd df = pd.DataFrame({"A": ["foo", "foo", "foo", "bar"], "B": [0, 1, 1, 1], "C": ["A", "A", "B", "A"]}) # Drop rows with duplicate values in columns 'A' and 'C' df = df.drop_duplicates(subset=['A', 'C'], keep=False) print(df)
Output:
A B C 2 foo 1 B 3 bar 1 A
As you can see, rows 0 and 1 have been dropped, as they are duplicates with respect to columns A and C.
The above is the detailed content of How to Drop Duplicate Rows Across Specific Columns in Pandas?. For more information, please follow other related articles on the PHP Chinese website!
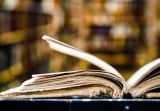
This tutorial demonstrates how to use Python to process the statistical concept of Zipf's law and demonstrates the efficiency of Python's reading and sorting large text files when processing the law. You may be wondering what the term Zipf distribution means. To understand this term, we first need to define Zipf's law. Don't worry, I'll try to simplify the instructions. Zipf's Law Zipf's law simply means: in a large natural language corpus, the most frequently occurring words appear about twice as frequently as the second frequent words, three times as the third frequent words, four times as the fourth frequent words, and so on. Let's look at an example. If you look at the Brown corpus in American English, you will notice that the most frequent word is "th
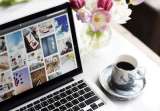
Dealing with noisy images is a common problem, especially with mobile phone or low-resolution camera photos. This tutorial explores image filtering techniques in Python using OpenCV to tackle this issue. Image Filtering: A Powerful Tool Image filter
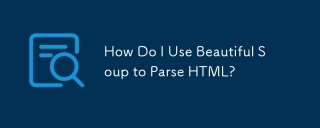
This article explains how to use Beautiful Soup, a Python library, to parse HTML. It details common methods like find(), find_all(), select(), and get_text() for data extraction, handling of diverse HTML structures and errors, and alternatives (Sel
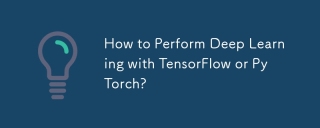
This article compares TensorFlow and PyTorch for deep learning. It details the steps involved: data preparation, model building, training, evaluation, and deployment. Key differences between the frameworks, particularly regarding computational grap
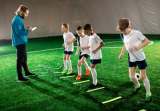
Python, a favorite for data science and processing, offers a rich ecosystem for high-performance computing. However, parallel programming in Python presents unique challenges. This tutorial explores these challenges, focusing on the Global Interprete
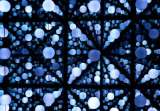
This tutorial demonstrates creating a custom pipeline data structure in Python 3, leveraging classes and operator overloading for enhanced functionality. The pipeline's flexibility lies in its ability to apply a series of functions to a data set, ge
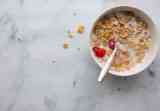
Serialization and deserialization of Python objects are key aspects of any non-trivial program. If you save something to a Python file, you do object serialization and deserialization if you read the configuration file, or if you respond to an HTTP request. In a sense, serialization and deserialization are the most boring things in the world. Who cares about all these formats and protocols? You want to persist or stream some Python objects and retrieve them in full at a later time. This is a great way to see the world on a conceptual level. However, on a practical level, the serialization scheme, format or protocol you choose may determine the speed, security, freedom of maintenance status, and other aspects of the program
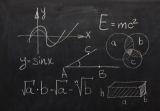
Python's statistics module provides powerful data statistical analysis capabilities to help us quickly understand the overall characteristics of data, such as biostatistics and business analysis. Instead of looking at data points one by one, just look at statistics such as mean or variance to discover trends and features in the original data that may be ignored, and compare large datasets more easily and effectively. This tutorial will explain how to calculate the mean and measure the degree of dispersion of the dataset. Unless otherwise stated, all functions in this module support the calculation of the mean() function instead of simply summing the average. Floating point numbers can also be used. import random import statistics from fracti


Hot AI Tools

Undresser.AI Undress
AI-powered app for creating realistic nude photos

AI Clothes Remover
Online AI tool for removing clothes from photos.

Undress AI Tool
Undress images for free

Clothoff.io
AI clothes remover

AI Hentai Generator
Generate AI Hentai for free.

Hot Article

Hot Tools

mPDF
mPDF is a PHP library that can generate PDF files from UTF-8 encoded HTML. The original author, Ian Back, wrote mPDF to output PDF files "on the fly" from his website and handle different languages. It is slower than original scripts like HTML2FPDF and produces larger files when using Unicode fonts, but supports CSS styles etc. and has a lot of enhancements. Supports almost all languages, including RTL (Arabic and Hebrew) and CJK (Chinese, Japanese and Korean). Supports nested block-level elements (such as P, DIV),

SublimeText3 Linux new version
SublimeText3 Linux latest version

Notepad++7.3.1
Easy-to-use and free code editor

PhpStorm Mac version
The latest (2018.2.1) professional PHP integrated development tool
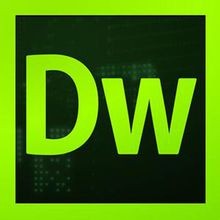
Dreamweaver CS6
Visual web development tools
