Author: Trix Cyrus
Waymap Pentesting tool: Click Here
TrixSec Github: Click Here
TrixSec Telegram: Click Here
Getting started with AI and Machine Learning requires a well-prepared development environment. This article will guide you through setting up the tools and libraries needed for your AI/ML journey, ensuring a smooth start for beginners. We’ll also discuss online platforms like Google Colab for those who want to avoid complex local setups.
System Requirements for AI/ML Development
Before diving into AI and Machine Learning projects, it’s essential to ensure your system can handle the computational demands. While most basic tasks can run on standard machines, more advanced projects (like deep learning) may require better hardware. Here’s a breakdown of system requirements based on project complexity:
1. For Beginners: Small Projects and Learning
-
Operating System: Windows 10/11, macOS, or any modern Linux distribution.
-
Processor: Dual-core CPU (Intel i5 or AMD equivalent).
-
RAM: 8 GB (minimum); 16 GB recommended for smoother multitasking.
-
Storage:
- 20 GB free space for Python, libraries, and small datasets.
- An SSD is highly recommended for faster performance.
-
GPU (Graphics Card): Not necessary; CPU will suffice for basic ML tasks.
-
Internet Connection: Required for downloading libraries, datasets, and using cloud platforms.
2. For Intermediate Projects: Larger Datasets
-
Processor: Quad-core CPU (Intel i7 or AMD Ryzen 5 equivalent).
-
RAM: 16 GB minimum; 32 GB recommended for large datasets.
-
Storage:
- 50–100 GB free space for datasets and experiments.
- SSD for quick data loading and operations.
-
GPU:
- Dedicated GPU with at least 4 GB VRAM (e.g., NVIDIA GTX 1650 or AMD Radeon RX 550).
- Useful for training larger models or experimenting with neural networks.
-
Display: Dual monitors can improve productivity during model debugging and visualization.
3. For Advanced Projects: Deep Learning and Large Models
-
Processor: High-performance CPU (Intel i9 or AMD Ryzen 7/9).
-
RAM: 32–64 GB to handle memory-intensive operations and large datasets.
-
Storage:
- 1 TB or more (SSD strongly recommended).
- External storage may be needed for datasets.
-
GPU:
- NVIDIA GPUs are preferred for deep learning due to CUDA support.
- Recommended: NVIDIA RTX 3060 (12 GB VRAM) or higher (e.g., RTX 3090, RTX 4090).
- For budget options: NVIDIA RTX 2060 or RTX 2070.
-
Cooling and Power Supply:
- Ensure proper cooling for GPUs, especially during long training sessions.
- Reliable power supply to support hardware.
4. Cloud Platforms: If Your System Falls Short
If your system doesn’t meet the above specs or you need more computational power, consider using cloud platforms:
-
Google Colab: Free with access to GPUs (upgradable to Colab Pro for longer runtime and better GPUs).
-
AWS EC2 or SageMaker: High-performance instances for large-scale ML projects.
-
Azure ML or GCP AI Platform: Suitable for enterprise-level projects.
-
Kaggle Kernels: Free for experiments with smaller datasets.
Recommended Setup Based on Use Case
Use Case |
CPU |
RAM |
GPU |
Storage |
Learning Basics |
Dual-Core i5 |
8–16 GB |
None/Integrated |
20–50 GB |
Intermediate ML Projects |
Quad-Core i7 |
16–32 GB |
GTX 1650 (4 GB) |
50–100 GB |
Deep Learning (Large Models) |
High-End i9/Ryzen 9 |
32–64 GB |
RTX 3060 (12 GB) |
1 TB SSD |
Cloud Platforms |
Not Required Locally |
N/A |
Cloud GPUs (e.g., T4, V100) |
N/A |
Use Case |
CPU |
RAM |
GPU |
Storage |
Learning Basics |
Dual-Core i5 |
8–16 GB |
None/Integrated |
20–50 GB |
Intermediate ML Projects |
Quad-Core i7 |
16–32 GB |
GTX 1650 (4 GB) |
50–100 GB |
Deep Learning (Large Models) |
High-End i9/Ryzen 9 |
32–64 GB |
RTX 3060 (12 GB) |
1 TB SSD |
Cloud Platforms |
Not Required Locally |
N/A |
Cloud GPUs (e.g., T4, V100) |
N/A |
Step 1: Installing Python
Python is the go-to language for AI/ML due to its simplicity and a vast ecosystem of libraries. Here’s how you can install it:
-
Download Python:
- Visit python.org and download the latest stable version (preferably Python 3.9 or later).
-
Install Python:
- Follow the installation steps for your operating system (Windows, macOS, or Linux).
- Make sure to check the option to add Python to PATH during installation.
-
Verify Installation:
- Open a terminal and type:
python --version
You should see the installed version of Python.
Step 2: Setting Up a Virtual Environment
To keep your projects organized and avoid dependency conflicts, it’s a good idea to use a virtual environment.
-
Create a Virtual Environment:
python -m venv env
-
Activate the Virtual Environment:
.\env\Scripts\activate
-
On macOS/Linux:
source env/bin/activate
-
Install Libraries Within the Environment:
After activation, any library installed will be isolated to this environment.
Step 3: Installing Essential Libraries
Once Python is ready, install the following libraries, which are essential for AI/ML:
-
NumPy: For numerical computations.
pip install numpy
-
pandas: For data manipulation and analysis.
pip install pandas
-
Matplotlib and Seaborn: For data visualization.
pip install matplotlib seaborn
-
scikit-learn: For basic ML algorithms and tools.
pip install scikit-learn
-
TensorFlow/PyTorch: For deep learning.
pip install tensorflow
or
pip install torch torchvision
-
Jupyter Notebook: An interactive environment for coding and visualizations.
pip install notebook
Step 4: Exploring Jupyter Notebooks
Jupyter Notebooks provide an interactive way to write and test code, making them perfect for learning AI/ML.
-
Launch Jupyter Notebook:
jupyter notebook
This will open a web interface in your browser.
-
Create a New Notebook:
- Click New > Python 3 Notebook and start coding!
Step 5: Setting Up Google Colab (Optional)
For those who don’t want to set up a local environment, Google Colab is a great alternative. It’s free and provides powerful GPUs for training AI models.
-
Visit Google Colab:
- Go to colab.research.google.com.
-
Create a New Notebook:
- Click New Notebook to start.
Install Libraries (if needed):
Libraries like NumPy and pandas are pre-installed, but you can install others using:
python --version
Step 6: Testing the Setup
To ensure everything is working, run this simple test in your Jupyter Notebook or Colab:
python -m venv env
Output Should Be
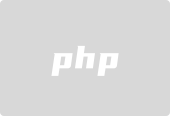
Common Errors and Solutions
-
Library Not Found:
- Ensure you’ve installed the library in the active virtual environment.
-
Python Not Recognized:
- Verify Python is added to your system PATH.
-
Jupyter Notebook Issues:
- Ensure you’ve installed Jupyter in the correct environment.
~Trixsec
The above is the detailed content of Part Building Your Own AI - Setting Up the Environment for AI/ML Development. For more information, please follow other related articles on the PHP Chinese website!