


What\'s the Most Efficient Way to Create and Populate a Pandas DataFrame Iteratively?
Creating an Empty Pandas DataFrame for Iterative Filling
Creating an empty Pandas DataFrame and iteratively filling it is a common task in data manipulation. However, the ideal approach may not be immediately apparent.
The Pitfalls of Row-wise DataFrame Growth
The code snippet you provided is one way to create an empty DataFrame and iteratively fill it. However, this method is inefficient and may lead to memory-related issues. The reason is that you are creating a new row for each iteration, which requires reallocating memory. This process becomes increasingly cumbersome as the DataFrame grows.
The Preferred Method: Accumulate Data in a List
The preferred approach is to accumulate data in a list and then create the DataFrame in one step using the pd.DataFrame() function. This method is significantly more efficient and memory-friendly. Here's how it works:
# Accumulate data in a list data = [] for row in some_function_that_yields_data(): data.append(row) # Create the DataFrame from the list df = pd.DataFrame(data)
Advantages of List Accumulation
- Computational Efficiency: Appending to a list is much faster than appending to a DataFrame, especially for large data sets.
- Memory Efficiency: Lists occupy less memory compared to DataFrames.
- Automatic Data Type Inference: pd.DataFrame automatically infers data types for each column, saving you the hassle of manual type assignment.
- Automatic Index Creation: When creating a DataFrame from a list, pandas automatically assigns a RangeIndex as the row index without requiring manual index management.
Alternatives to Avoid
- Append or Concat Inside a Loop: This method is very inefficient due to the constant memory reallocation required with each iteration.
- loc Inside a Loop: Similar to append or concat inside a loop, using df.loc[len(df)] for each iteration leads to memory overhead.
- Empty DataFrame of NaNs: Creating an empty DataFrame filled with NaNs also results in object data types, which can hinder pandas operations.
Conclusion
When dealing with large data sets, accumulating data in a list and creating the DataFrame in one step is the recommended approach. It is computationally efficient, memory-friendly, and simplifies the data manipulation process.
The above is the detailed content of What\'s the Most Efficient Way to Create and Populate a Pandas DataFrame Iteratively?. For more information, please follow other related articles on the PHP Chinese website!
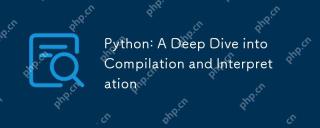
Pythonusesahybridmodelofcompilationandinterpretation:1)ThePythoninterpretercompilessourcecodeintoplatform-independentbytecode.2)ThePythonVirtualMachine(PVM)thenexecutesthisbytecode,balancingeaseofusewithperformance.
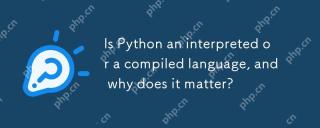
Pythonisbothinterpretedandcompiled.1)It'scompiledtobytecodeforportabilityacrossplatforms.2)Thebytecodeistheninterpreted,allowingfordynamictypingandrapiddevelopment,thoughitmaybeslowerthanfullycompiledlanguages.
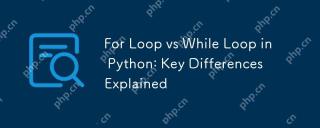
Forloopsareidealwhenyouknowthenumberofiterationsinadvance,whilewhileloopsarebetterforsituationswhereyouneedtoloopuntilaconditionismet.Forloopsaremoreefficientandreadable,suitableforiteratingoversequences,whereaswhileloopsoffermorecontrolandareusefulf
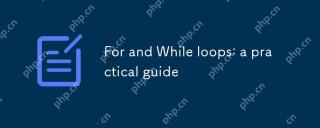
Forloopsareusedwhenthenumberofiterationsisknowninadvance,whilewhileloopsareusedwhentheiterationsdependonacondition.1)Forloopsareidealforiteratingoversequenceslikelistsorarrays.2)Whileloopsaresuitableforscenarioswheretheloopcontinuesuntilaspecificcond
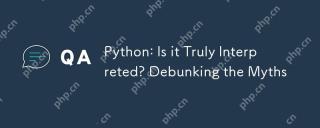
Pythonisnotpurelyinterpreted;itusesahybridapproachofbytecodecompilationandruntimeinterpretation.1)Pythoncompilessourcecodeintobytecode,whichisthenexecutedbythePythonVirtualMachine(PVM).2)Thisprocessallowsforrapiddevelopmentbutcanimpactperformance,req
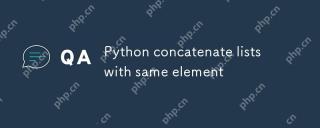
ToconcatenatelistsinPythonwiththesameelements,use:1)the operatortokeepduplicates,2)asettoremoveduplicates,or3)listcomprehensionforcontroloverduplicates,eachmethodhasdifferentperformanceandorderimplications.
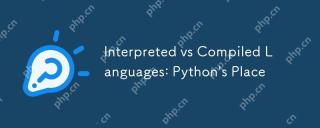
Pythonisaninterpretedlanguage,offeringeaseofuseandflexibilitybutfacingperformancelimitationsincriticalapplications.1)InterpretedlanguageslikePythonexecuteline-by-line,allowingimmediatefeedbackandrapidprototyping.2)CompiledlanguageslikeC/C transformt
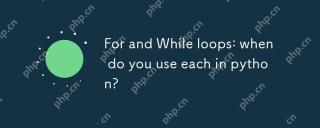
Useforloopswhenthenumberofiterationsisknowninadvance,andwhileloopswheniterationsdependonacondition.1)Forloopsareidealforsequenceslikelistsorranges.2)Whileloopssuitscenarioswheretheloopcontinuesuntilaspecificconditionismet,usefulforuserinputsoralgorit


Hot AI Tools

Undresser.AI Undress
AI-powered app for creating realistic nude photos

AI Clothes Remover
Online AI tool for removing clothes from photos.

Undress AI Tool
Undress images for free

Clothoff.io
AI clothes remover

Video Face Swap
Swap faces in any video effortlessly with our completely free AI face swap tool!

Hot Article

Hot Tools

SublimeText3 Chinese version
Chinese version, very easy to use

mPDF
mPDF is a PHP library that can generate PDF files from UTF-8 encoded HTML. The original author, Ian Back, wrote mPDF to output PDF files "on the fly" from his website and handle different languages. It is slower than original scripts like HTML2FPDF and produces larger files when using Unicode fonts, but supports CSS styles etc. and has a lot of enhancements. Supports almost all languages, including RTL (Arabic and Hebrew) and CJK (Chinese, Japanese and Korean). Supports nested block-level elements (such as P, DIV),

SecLists
SecLists is the ultimate security tester's companion. It is a collection of various types of lists that are frequently used during security assessments, all in one place. SecLists helps make security testing more efficient and productive by conveniently providing all the lists a security tester might need. List types include usernames, passwords, URLs, fuzzing payloads, sensitive data patterns, web shells, and more. The tester can simply pull this repository onto a new test machine and he will have access to every type of list he needs.

MinGW - Minimalist GNU for Windows
This project is in the process of being migrated to osdn.net/projects/mingw, you can continue to follow us there. MinGW: A native Windows port of the GNU Compiler Collection (GCC), freely distributable import libraries and header files for building native Windows applications; includes extensions to the MSVC runtime to support C99 functionality. All MinGW software can run on 64-bit Windows platforms.

SAP NetWeaver Server Adapter for Eclipse
Integrate Eclipse with SAP NetWeaver application server.
