


Anyone who works with Python knows that it can be a bit annoying to deal with managing virtual environments and dependencies. This is essential for maintaining control over projects, especially those that require specific libraries or different versions of Python. In these scenarios, the UV tool appears as a very interesting solution, combining environment and version management functionalities in an efficient and simplified flow. It was made by the same developers as Ruff, a formatter and linter that, like UV, was also written in Rust. In other words, performance is not a problem. In this post, we're going to talk a little about how UV can help developers maintain organization, productivity and flexibility in the development of their projects.
What is UV?
UV is a tool that integrates virtual environment management, dependency control and Python version management functionalities. Inspired by popular tools like pyenv and pipenv, UV brings together the best of both worlds, allowing you to easily create and manage virtual environments at the same time in which it maintains robust control over Python dependencies and versions.
With UV, you can:
- Switch between Python versions in a simple way (similar to Node's nvm)
- Create and manage specific virtual environments for each project
- Manage dependencies with version control and ease of installation
- Maintain a history of project dependencies to ensure portability
1. Virtual Environment Management
Virtual environments allow you to isolate a project's dependencies to avoid conflicts between packages and versions. However, manually managing these environments can be laborious and sometimes confusing. With UV, this task becomes much more fluid.
Creating a virtual environment is simple and quick with the uv venv command. After that, you can activate the environment with source .venv/Scripts/activate or even configure UV to activate the environment automatically when entering the project folder, facilitating the process and avoiding compatibility problems between different projects.
In addition, UV offers practical functionality to view existing environments and delete them when they are no longer needed, freeing up space and keeping your system organized.
2. Python Version Management
Many developers face the challenge of managing different versions of Python on their systems. Certain projects may require version 3.7, while others depend on functionality from version 3.9 or 3.11. With UV, you can easily install and switch between multiple versions of Python, all intuitively.
To install a new version of Python, just run uv python install
3. Dependency Management and requirements.txt File
Just like pipenv, UV helps you manage dependencies in an organized way. With simple commands like uv add
UV also allows you to install specific package versions, which helps you avoid compatibility issues when working in a team or sharing the project with other developers. And if you need a clean installation of dependencies, the uv sync command reconfigures the environment based on the requirements.txt file, ensuring everything is in order.
4. Productivity and Ease of Use
The simplicity of UV is a big differentiator, as it allows developers to focus on what really matters: the code. With easy-to-remember commands and an intuitive structure, UV saves time and reduces the complexity of working with virtual environments and dependencies.
For example, when using UV, you no longer need to remember extensive commands to create or activate environments, or worry about Python version conflicts between projects. With a single tool, you have everything you need for complete management of environments and dependencies, resulting in significantly greater productivity.
It is still possible to integrate UV with other development tools, such as Ruff, already mentioned, and even Docker. This way, you can adapt the UV settings to the specific needs of your project. Anyway, there are many possibilities. The tool's documentation is very complete and is worth checking out.
The above is the detailed content of UV - The Tool that Simplifies Environment and Dependency Management in Python. For more information, please follow other related articles on the PHP Chinese website!
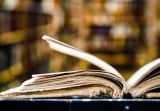
This tutorial demonstrates how to use Python to process the statistical concept of Zipf's law and demonstrates the efficiency of Python's reading and sorting large text files when processing the law. You may be wondering what the term Zipf distribution means. To understand this term, we first need to define Zipf's law. Don't worry, I'll try to simplify the instructions. Zipf's Law Zipf's law simply means: in a large natural language corpus, the most frequently occurring words appear about twice as frequently as the second frequent words, three times as the third frequent words, four times as the fourth frequent words, and so on. Let's look at an example. If you look at the Brown corpus in American English, you will notice that the most frequent word is "th
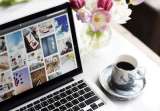
Dealing with noisy images is a common problem, especially with mobile phone or low-resolution camera photos. This tutorial explores image filtering techniques in Python using OpenCV to tackle this issue. Image Filtering: A Powerful Tool Image filter
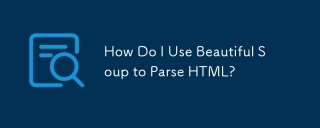
This article explains how to use Beautiful Soup, a Python library, to parse HTML. It details common methods like find(), find_all(), select(), and get_text() for data extraction, handling of diverse HTML structures and errors, and alternatives (Sel
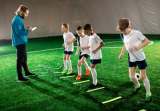
Python, a favorite for data science and processing, offers a rich ecosystem for high-performance computing. However, parallel programming in Python presents unique challenges. This tutorial explores these challenges, focusing on the Global Interprete
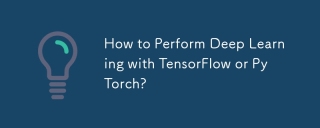
This article compares TensorFlow and PyTorch for deep learning. It details the steps involved: data preparation, model building, training, evaluation, and deployment. Key differences between the frameworks, particularly regarding computational grap
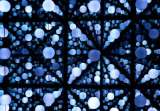
This tutorial demonstrates creating a custom pipeline data structure in Python 3, leveraging classes and operator overloading for enhanced functionality. The pipeline's flexibility lies in its ability to apply a series of functions to a data set, ge
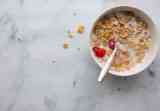
Serialization and deserialization of Python objects are key aspects of any non-trivial program. If you save something to a Python file, you do object serialization and deserialization if you read the configuration file, or if you respond to an HTTP request. In a sense, serialization and deserialization are the most boring things in the world. Who cares about all these formats and protocols? You want to persist or stream some Python objects and retrieve them in full at a later time. This is a great way to see the world on a conceptual level. However, on a practical level, the serialization scheme, format or protocol you choose may determine the speed, security, freedom of maintenance status, and other aspects of the program
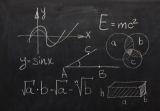
Python's statistics module provides powerful data statistical analysis capabilities to help us quickly understand the overall characteristics of data, such as biostatistics and business analysis. Instead of looking at data points one by one, just look at statistics such as mean or variance to discover trends and features in the original data that may be ignored, and compare large datasets more easily and effectively. This tutorial will explain how to calculate the mean and measure the degree of dispersion of the dataset. Unless otherwise stated, all functions in this module support the calculation of the mean() function instead of simply summing the average. Floating point numbers can also be used. import random import statistics from fracti


Hot AI Tools

Undresser.AI Undress
AI-powered app for creating realistic nude photos

AI Clothes Remover
Online AI tool for removing clothes from photos.

Undress AI Tool
Undress images for free

Clothoff.io
AI clothes remover

AI Hentai Generator
Generate AI Hentai for free.

Hot Article

Hot Tools

Safe Exam Browser
Safe Exam Browser is a secure browser environment for taking online exams securely. This software turns any computer into a secure workstation. It controls access to any utility and prevents students from using unauthorized resources.

SublimeText3 Linux new version
SublimeText3 Linux latest version
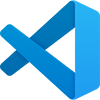
VSCode Windows 64-bit Download
A free and powerful IDE editor launched by Microsoft

Atom editor mac version download
The most popular open source editor
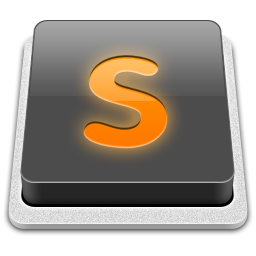
SublimeText3 Mac version
God-level code editing software (SublimeText3)
