


Mastering Pythons Async: Boost Your App Performance with Coroutines and Event Loops
Python's async programming is a game-changer for building high-performance applications. I've been using it for years, and it never ceases to amaze me how powerful it can be when used correctly.
At the heart of Python's async model are coroutines and event loops. Coroutines are special functions that can pause and resume their execution, allowing for efficient multitasking without the overhead of threads. Event loops, on the other hand, are the engines that drive these coroutines, managing their execution and handling I/O operations.
Let's start with coroutines. In Python, we define them using the async def syntax. Here's a simple example:
async def greet(name): print(f"Hello, {name}!") await asyncio.sleep(1) print(f"Goodbye, {name}!")
This coroutine greets a person, waits for a second, and then says goodbye. The await keyword is crucial here - it allows the coroutine to pause its execution and give control back to the event loop.
But how do coroutines work under the hood? They're actually built on top of Python's generator functionality. When you call a coroutine, it doesn't run immediately. Instead, it returns a coroutine object. This object can be sent values and can yield values, just like a generator.
The event loop is responsible for actually running these coroutines. It maintains a queue of tasks (which are wrappers around coroutines) and executes them one by one. When a coroutine hits an await statement, the event loop suspends it and moves on to the next task. This is the essence of cooperative multitasking - tasks voluntarily give up control, allowing others to run.
Here's a simplified version of how an event loop might work:
class EventLoop: def __init__(self): self.ready = deque() self.sleeping = [] def call_soon(self, callback): self.ready.append(callback) def call_later(self, delay, callback): deadline = time.time() + delay heapq.heappush(self.sleeping, (deadline, callback)) def run_forever(self): while True: self.run_once() def run_once(self): now = time.time() while self.sleeping and self.sleeping[0][0] <p>This event loop manages two types of tasks: those that are ready to run (in the ready deque) and those that are sleeping (in the sleeping list). The run_forever method keeps running tasks until there are no more left.</p> <p>Now, let's talk about task scheduling. The asyncio module in Python provides a more sophisticated event loop with advanced scheduling capabilities. It can handle I/O operations, run subprocesses, and even integrate with other event loops.</p> <p>Here's how you might use asyncio to run multiple coroutines concurrently:<br> </p> <pre class="brush:php;toolbar:false">import asyncio async def task1(): print("Task 1 starting") await asyncio.sleep(2) print("Task 1 finished") async def task2(): print("Task 2 starting") await asyncio.sleep(1) print("Task 2 finished") async def main(): await asyncio.gather(task1(), task2()) asyncio.run(main())
This script will start both tasks, but task2 will finish before task1 because it sleeps for a shorter time.
One of the most powerful applications of async programming is in network operations. Let's look at a simple asynchronous web server:
import asyncio async def handle_client(reader, writer): data = await reader.read(100) message = data.decode() addr = writer.get_extra_info('peername') print(f"Received {message!r} from {addr!r}") response = f"Echo: {message}\n" writer.write(response.encode()) await writer.drain() print("Close the connection") writer.close() async def main(): server = await asyncio.start_server( handle_client, '127.0.0.1', 8888) addr = server.sockets[0].getsockname() print(f'Serving on {addr}') async with server: await server.serve_forever() asyncio.run(main())
This server can handle multiple clients concurrently without using threads, making it highly efficient.
But async programming isn't just for servers. It's also great for clients, especially when you need to make multiple network requests. Here's a simple web scraper that can fetch multiple pages concurrently:
async def greet(name): print(f"Hello, {name}!") await asyncio.sleep(1) print(f"Goodbye, {name}!")
This scraper can fetch multiple pages simultaneously, significantly speeding up the process compared to a synchronous approach.
Now, let's dive into some more advanced concepts. One interesting feature of Python's async model is that you can create your own event loops. This can be useful if you need to integrate async code with other frameworks or if you want to optimize for specific use cases.
Here's a simple custom event loop that can run both synchronous and asynchronous callbacks:
class EventLoop: def __init__(self): self.ready = deque() self.sleeping = [] def call_soon(self, callback): self.ready.append(callback) def call_later(self, delay, callback): deadline = time.time() + delay heapq.heappush(self.sleeping, (deadline, callback)) def run_forever(self): while True: self.run_once() def run_once(self): now = time.time() while self.sleeping and self.sleeping[0][0] <p>This custom loop is very basic, but it demonstrates the core principles. You could extend this to handle more complex scenarios, like I/O operations or timers.</p> <p>Debugging async code can be challenging, especially when you're dealing with complex applications. One technique I find helpful is to use asyncio's debug mode. You can enable it like this:<br> </p> <pre class="brush:php;toolbar:false">import asyncio async def task1(): print("Task 1 starting") await asyncio.sleep(2) print("Task 1 finished") async def task2(): print("Task 2 starting") await asyncio.sleep(1) print("Task 2 finished") async def main(): await asyncio.gather(task1(), task2()) asyncio.run(main())
This will provide more detailed error messages and warnings about things like coroutines that never complete or callbacks that take too long to run.
Another useful debugging technique is to use asyncio's task introspection features. For example, you can get a list of all running tasks:
import asyncio async def handle_client(reader, writer): data = await reader.read(100) message = data.decode() addr = writer.get_extra_info('peername') print(f"Received {message!r} from {addr!r}") response = f"Echo: {message}\n" writer.write(response.encode()) await writer.drain() print("Close the connection") writer.close() async def main(): server = await asyncio.start_server( handle_client, '127.0.0.1', 8888) addr = server.sockets[0].getsockname() print(f'Serving on {addr}') async with server: await server.serve_forever() asyncio.run(main())
This can help you understand what your program is doing at any given moment.
When it comes to optimizing async code, one key principle is to minimize the time spent in synchronous operations. Any long-running synchronous code will block the event loop, preventing other coroutines from running. If you have CPU-intensive tasks, consider running them in a separate thread or process.
Another optimization technique is to use asyncio.gather when you have multiple coroutines that can run concurrently. This is more efficient than awaiting them one by one:
import asyncio import aiohttp async def fetch_page(session, url): async with session.get(url) as response: return await response.text() async def main(): urls = [ 'http://example.com', 'http://example.org', 'http://example.net' ] async with aiohttp.ClientSession() as session: tasks = [fetch_page(session, url) for url in urls] pages = await asyncio.gather(*tasks) for url, page in zip(urls, pages): print(f"Page from {url}: {len(page)} bytes") asyncio.run(main())
Lastly, remember that async programming isn't always the best solution. For I/O-bound tasks with lots of waiting, it can provide significant performance improvements. But for CPU-bound tasks, traditional multithreading or multiprocessing might be more appropriate.
In conclusion, Python's async programming model, built on coroutines and event loops, offers a powerful way to write efficient, scalable applications. Whether you're building web servers, network clients, or data processing pipelines, understanding these concepts can help you take full advantage of Python's async capabilities. As with any powerful tool, it requires practice and careful thought to use effectively, but the results can be truly impressive.
Our Creations
Be sure to check out our creations:
Investor Central | Smart Living | Epochs & Echoes | Puzzling Mysteries | Hindutva | Elite Dev | JS Schools
We are on Medium
Tech Koala Insights | Epochs & Echoes World | Investor Central Medium | Puzzling Mysteries Medium | Science & Epochs Medium | Modern Hindutva
The above is the detailed content of Mastering Pythons Async: Boost Your App Performance with Coroutines and Event Loops. For more information, please follow other related articles on the PHP Chinese website!
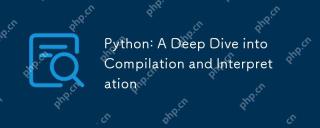
Pythonusesahybridmodelofcompilationandinterpretation:1)ThePythoninterpretercompilessourcecodeintoplatform-independentbytecode.2)ThePythonVirtualMachine(PVM)thenexecutesthisbytecode,balancingeaseofusewithperformance.
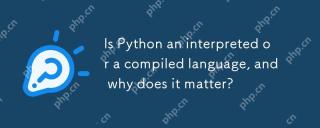
Pythonisbothinterpretedandcompiled.1)It'scompiledtobytecodeforportabilityacrossplatforms.2)Thebytecodeistheninterpreted,allowingfordynamictypingandrapiddevelopment,thoughitmaybeslowerthanfullycompiledlanguages.
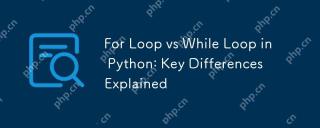
Forloopsareidealwhenyouknowthenumberofiterationsinadvance,whilewhileloopsarebetterforsituationswhereyouneedtoloopuntilaconditionismet.Forloopsaremoreefficientandreadable,suitableforiteratingoversequences,whereaswhileloopsoffermorecontrolandareusefulf
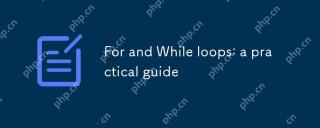
Forloopsareusedwhenthenumberofiterationsisknowninadvance,whilewhileloopsareusedwhentheiterationsdependonacondition.1)Forloopsareidealforiteratingoversequenceslikelistsorarrays.2)Whileloopsaresuitableforscenarioswheretheloopcontinuesuntilaspecificcond
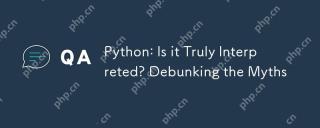
Pythonisnotpurelyinterpreted;itusesahybridapproachofbytecodecompilationandruntimeinterpretation.1)Pythoncompilessourcecodeintobytecode,whichisthenexecutedbythePythonVirtualMachine(PVM).2)Thisprocessallowsforrapiddevelopmentbutcanimpactperformance,req
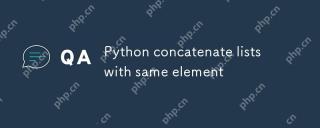
ToconcatenatelistsinPythonwiththesameelements,use:1)the operatortokeepduplicates,2)asettoremoveduplicates,or3)listcomprehensionforcontroloverduplicates,eachmethodhasdifferentperformanceandorderimplications.
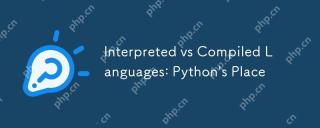
Pythonisaninterpretedlanguage,offeringeaseofuseandflexibilitybutfacingperformancelimitationsincriticalapplications.1)InterpretedlanguageslikePythonexecuteline-by-line,allowingimmediatefeedbackandrapidprototyping.2)CompiledlanguageslikeC/C transformt
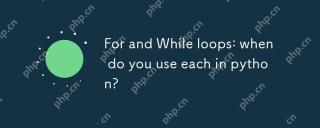
Useforloopswhenthenumberofiterationsisknowninadvance,andwhileloopswheniterationsdependonacondition.1)Forloopsareidealforsequenceslikelistsorranges.2)Whileloopssuitscenarioswheretheloopcontinuesuntilaspecificconditionismet,usefulforuserinputsoralgorit


Hot AI Tools

Undresser.AI Undress
AI-powered app for creating realistic nude photos

AI Clothes Remover
Online AI tool for removing clothes from photos.

Undress AI Tool
Undress images for free

Clothoff.io
AI clothes remover

Video Face Swap
Swap faces in any video effortlessly with our completely free AI face swap tool!

Hot Article

Hot Tools

SublimeText3 English version
Recommended: Win version, supports code prompts!

Zend Studio 13.0.1
Powerful PHP integrated development environment

SecLists
SecLists is the ultimate security tester's companion. It is a collection of various types of lists that are frequently used during security assessments, all in one place. SecLists helps make security testing more efficient and productive by conveniently providing all the lists a security tester might need. List types include usernames, passwords, URLs, fuzzing payloads, sensitive data patterns, web shells, and more. The tester can simply pull this repository onto a new test machine and he will have access to every type of list he needs.

MantisBT
Mantis is an easy-to-deploy web-based defect tracking tool designed to aid in product defect tracking. It requires PHP, MySQL and a web server. Check out our demo and hosting services.
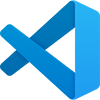
VSCode Windows 64-bit Download
A free and powerful IDE editor launched by Microsoft
