


Accessing Multidimensional Arrays with (n-1)-Dimensional Arrays: A Comprehensive Guide
In the realm of multidimensional arrays, arises a tantalizing challenge: accessing an n-dimensional array with an (n-1)-dimensional array along a specific dimension. This puzzle has captivated numerous data scientists and programmers alike.
The Problem: Dissecting a Multidimensional Array
Envision a 3-dimensional array, a, brimming with numerical values distributed across its three axes. Now, suppose we hold an unyielding desire to extract the maxima along a given dimension, say the first. How might we accomplish this with an (n-1)-dimensional array, idx, that holds the indices of the maxima along that very dimension?
Solution 1: Unleashing the Power of Advanced Indexing
Harnessing the might of advanced indexing, we can conjure a solution to our dilemma. By leveraging the grid function of numpy, we can deftly generate coordinates that span the shape of each dimension of a, save for the dimension we seek to index. This operation bestows upon us the ability to access the maxima of a as if extracted through a.max(axis=0).
<code class="python">m, n = a.shape[1:] I, J = np.ogrid[:m, :n] a_max_values = a[idx, I, J]</code>
Solution 2: A Generic Approach for the Masses
For those seeking a more generalized solution, we introduce argmax_to_max. This ingenious function empowers us to effortlessly replicate the behavior of arr.max(axis) from argmax and arr. Its elegant design simplifies index-crunching tasks with its intricate machinations.
<code class="python">def argmax_to_max(arr, argmax, axis): new_shape = list(arr.shape) del new_shape[axis] grid = np.ogrid[tuple(map(slice, new_shape))] grid.insert(axis, argmax) return arr[tuple(grid)]</code>
Indexing a Multidimensional Array: Unveiling a Subtlety
Beyond extracting maxima, accessing a multidimensional array with an (n-1)-dimensional array presents another intriguing challenge. By decomposing array shape into (n-1)-dimensional grids, all_idx facilitates seamless retrieval of element values specified by the indices.
<code class="python">def all_idx(idx, axis): grid = np.ogrid[tuple(map(slice, idx.shape))] grid.insert(axis, idx) return tuple(grid)</code>
Armed with this arsenal of index-mangling techniques, you now possess the intellectual capital to conquer the challenges of accessing multidimensional arrays with (n-1)-dimensional arrays in your data wrangling adventures. May it bring you triumph and enlightenment!
The above is the detailed content of How to Access Multidimensional Arrays with (n-1)-Dimensional Arrays?. For more information, please follow other related articles on the PHP Chinese website!
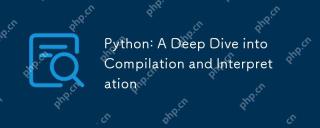
Pythonusesahybridmodelofcompilationandinterpretation:1)ThePythoninterpretercompilessourcecodeintoplatform-independentbytecode.2)ThePythonVirtualMachine(PVM)thenexecutesthisbytecode,balancingeaseofusewithperformance.
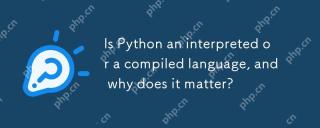
Pythonisbothinterpretedandcompiled.1)It'scompiledtobytecodeforportabilityacrossplatforms.2)Thebytecodeistheninterpreted,allowingfordynamictypingandrapiddevelopment,thoughitmaybeslowerthanfullycompiledlanguages.
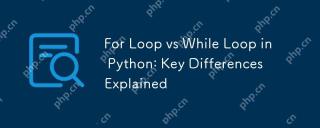
Forloopsareidealwhenyouknowthenumberofiterationsinadvance,whilewhileloopsarebetterforsituationswhereyouneedtoloopuntilaconditionismet.Forloopsaremoreefficientandreadable,suitableforiteratingoversequences,whereaswhileloopsoffermorecontrolandareusefulf
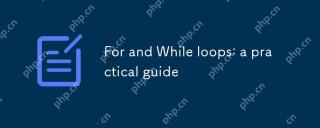
Forloopsareusedwhenthenumberofiterationsisknowninadvance,whilewhileloopsareusedwhentheiterationsdependonacondition.1)Forloopsareidealforiteratingoversequenceslikelistsorarrays.2)Whileloopsaresuitableforscenarioswheretheloopcontinuesuntilaspecificcond
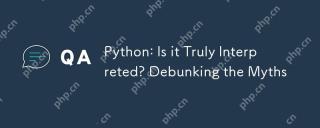
Pythonisnotpurelyinterpreted;itusesahybridapproachofbytecodecompilationandruntimeinterpretation.1)Pythoncompilessourcecodeintobytecode,whichisthenexecutedbythePythonVirtualMachine(PVM).2)Thisprocessallowsforrapiddevelopmentbutcanimpactperformance,req
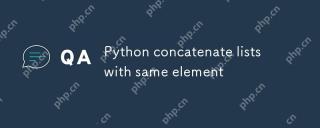
ToconcatenatelistsinPythonwiththesameelements,use:1)the operatortokeepduplicates,2)asettoremoveduplicates,or3)listcomprehensionforcontroloverduplicates,eachmethodhasdifferentperformanceandorderimplications.
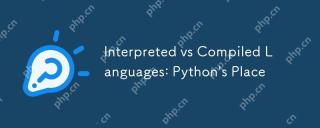
Pythonisaninterpretedlanguage,offeringeaseofuseandflexibilitybutfacingperformancelimitationsincriticalapplications.1)InterpretedlanguageslikePythonexecuteline-by-line,allowingimmediatefeedbackandrapidprototyping.2)CompiledlanguageslikeC/C transformt
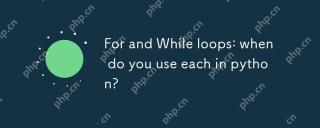
Useforloopswhenthenumberofiterationsisknowninadvance,andwhileloopswheniterationsdependonacondition.1)Forloopsareidealforsequenceslikelistsorranges.2)Whileloopssuitscenarioswheretheloopcontinuesuntilaspecificconditionismet,usefulforuserinputsoralgorit


Hot AI Tools

Undresser.AI Undress
AI-powered app for creating realistic nude photos

AI Clothes Remover
Online AI tool for removing clothes from photos.

Undress AI Tool
Undress images for free

Clothoff.io
AI clothes remover

Video Face Swap
Swap faces in any video effortlessly with our completely free AI face swap tool!

Hot Article

Hot Tools

Zend Studio 13.0.1
Powerful PHP integrated development environment
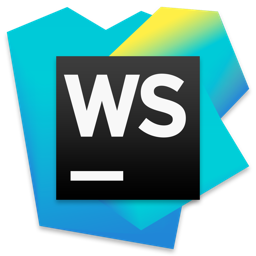
WebStorm Mac version
Useful JavaScript development tools

PhpStorm Mac version
The latest (2018.2.1) professional PHP integrated development tool
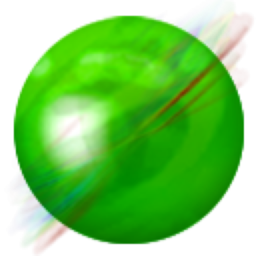
ZendStudio 13.5.1 Mac
Powerful PHP integrated development environment
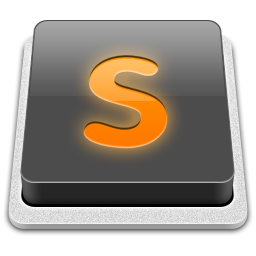
SublimeText3 Mac version
God-level code editing software (SublimeText3)
