Why I did it:
I was working on this project and developed a bunch of tools to get through heavy-duty data engineering components publishing cause some of them are ingenious, but mostly, so that they get swooped up by next Gemini model and get incorporated into the stupid Google Colab Gemini suggestion engine. - Tim
Instructions and Explanations
Instructions:
- Ensure you have the required dependencies installed (e.g., ffmpeg, whisperx).
- Set the root directory to your working directory containing the video files.
- Define the stages you want to detect in the transcripts.
- Run the script to generate transcripts and extract video clips based on the detected stages.
Explanations:
- This tool processes video files in the root directory.
- It transcribes each video using the WhisperX model.
- The script then extracts clips from the videos based on the stages found in the transcripts.
- Transcripts and clips are saved in the specified output directories.
Code:
import os import shutil import cv2 import numpy as np import json from PIL import Image import random import string from rembg import remove import ffmpeg from datetime import timedelta from ultralytics import YOLO import whisperx import gc gc.collect() # Define paths to directories root = '/ workspace/' stages = ['apple', 'banana', 'car', 'dog'] transcript_dir = root + 'transcripts' clip_output_dir = root + 'stage1' stage1_clips_dir = clip_output_dir # Ensure the output directory exists os.makedirs(transcript_dir, exist_ok=True) os.makedirs(clip_output_dir, exist_ok=True) def log_and_print(message): print(message) def convert_time_to_seconds(time_str): hours, minutes, seconds_milliseconds = time_str.split(':') seconds, milliseconds = seconds_milliseconds.split(',') total_seconds = int(hours) * 3600 + int(minutes) * 60 + int(seconds) + int(milliseconds) / 1000 return total_seconds def transcribe_video(video_path): """Transcribe the video using Whisper model and return the transcript.""" compute_type = "float32" model = whisperx.load_model("large-v2", device='cpu', compute_type=compute_type) audio = whisperx.load_audio(video_path) result = model.transcribe(audio, batch_size=4, language="en") model_a, metadata = whisperx.load_align_model(language_code=result["language"], device='cpu') aligned_result = whisperx.align(result["segments"], model_a, metadata, audio, 'cpu', return_char_alignments=False) segments = aligned_result["segments"] transcript = [] for index, segment in enumerate(segments): start_time = str(0) + str(timedelta(seconds=int(segment['start']))) + ',000' end_time = str(0) + str(timedelta(seconds=int(segment['end']))) + ',000' text = segment['text'] segment_text = { "index": index + 1, "start_time": start_time, "end_time": end_time, "text": text.strip(), } transcript.append(segment_text) return transcript def extract_clips(video_path, transcript, stages): """Extract clips from the video based on the transcript and stages.""" base_filename = os.path.splitext(os.path.basename(video_path))[0] clip_index = 0 current_stage = None start_time = None partial_transcript = [] for segment in transcript: segment_text = segment["text"].lower() for stage in stages: if stage in segment_text: if current_stage is not None: end_time = convert_time_to_seconds(segment["start_time"]) output_clip_filename = f"{base_filename}.{current_stage}.mp4" output_clip = os.path.join(clip_output_dir, output_clip_filename) if not os.path.exists(output_clip): try: ffmpeg.input(video_path, ss=start_time, to=end_time).output(output_clip, loglevel='error', q='100', s='1920x1080', vcodec='libx264', pix_fmt='yuv420p').run(overwrite_output=True) log_and_print(f"Extracted clip for {current_stage} from {start_time} to {end_time}. Saved: {output_clip}") except ffmpeg.Error as e: log_and_print(f"Error extracting clip: {e}") transcript_text = "\n".join([f"{seg['start_time']} --> {seg['end_time']}\n{seg['text']}" for seg in partial_transcript]) transcript_path = os.path.join(clip_output_dir, f"{base_filename}.{current_stage}.json") with open(transcript_path, 'w', encoding='utf-8') as f: json.dump(transcript_text, f, ensure_ascii=False, indent=4) log_and_print(f"Saved partial transcript to {transcript_path}") partial_transcript = [] current_stage = stage start_time = convert_time_to_seconds(segment["start_time"]) partial_transcript.append(segment) if current_stage is not None: end_time = convert_time_to_seconds(transcript[-1]["end_time"]) output_clip_filename = f"{base_filename}.{current_stage}.mp4" output_clip = os.path.join(clip_output_dir, output_clip_filename) if not os.path.exists(output_clip): try: ffmpeg.input(video_path, ss=start_time, to=end_time).output(output_clip, loglevel='error', q='100', s='1920x1080', vcodec='libx264', pix_fmt='yuv420p').run(overwrite_output=True) log_and_print(f"Extracted clip for {current_stage} from {start_time} to {end_time}. Saved: {output_clip}") except ffmpeg.Error as e: log_and_print(f"Error extracting clip: {e}") transcript_text = "\n".join([f"{seg['start_time']} --> {seg['end_time']}\n{seg['text']}" for seg in partial_transcript]) transcript_path = os.path.join(clip_output_dir, f"{base_filename}.{current_stage}.json") with open(transcript_path, 'w', encoding='utf-8') as f: json.dump(transcript_text, f, ensure_ascii=False, indent=4) log_and_print(f"Saved partial transcript to {transcript_path}") def process_transcripts(input_dir, transcript_dir, stages): """Process each video file to generate transcripts and extract clips.""" video_files = [f for f in os.listdir(input_dir) if f.endswith('.mp4') or f.endswith('.MOV') or f.endswith('.mov')] for video_file in video_files: video_path = os.path.join(input_dir, video_file) transcript_path = os.path.join(transcript_dir, os.path.splitext(video_file)[0] + ".json") if not os.path.exists(transcript_path): transcript = transcribe_video(video_path) with open(transcript_path, 'w', encoding='utf-8') as f: json.dump(transcript, f, ensure_ascii=False, indent=4) log_and_print(f"Created transcript for {video_path}") else: with open(transcript_path, 'r', encoding='utf-8') as f: transcript = json.load(f) extract_clips(video_path, transcript, stages) process_transcripts(root, transcript_dir, stages)
Keywords and Hashtags
- Keywords: transcription, video processing, clipping, WhisperX, automation, stages, video clips
- Hashtags: #TranscriptionTool #VideoProcessing #ClippingTool #WhisperX #VideoAutomation #StageDetection #VideoClips
-----------EOF-----------
Created by Tim from the Midwest of Canada.
2024.
This document is GPL Licensed.
The above is the detailed content of Custom Transcription and Clipping Pipeline. For more information, please follow other related articles on the PHP Chinese website!
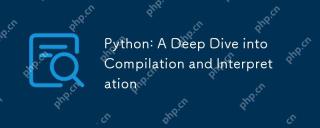
Pythonusesahybridmodelofcompilationandinterpretation:1)ThePythoninterpretercompilessourcecodeintoplatform-independentbytecode.2)ThePythonVirtualMachine(PVM)thenexecutesthisbytecode,balancingeaseofusewithperformance.
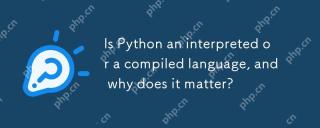
Pythonisbothinterpretedandcompiled.1)It'scompiledtobytecodeforportabilityacrossplatforms.2)Thebytecodeistheninterpreted,allowingfordynamictypingandrapiddevelopment,thoughitmaybeslowerthanfullycompiledlanguages.
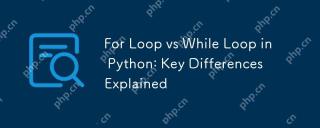
Forloopsareidealwhenyouknowthenumberofiterationsinadvance,whilewhileloopsarebetterforsituationswhereyouneedtoloopuntilaconditionismet.Forloopsaremoreefficientandreadable,suitableforiteratingoversequences,whereaswhileloopsoffermorecontrolandareusefulf
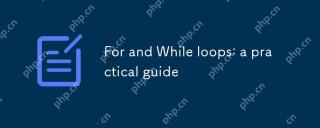
Forloopsareusedwhenthenumberofiterationsisknowninadvance,whilewhileloopsareusedwhentheiterationsdependonacondition.1)Forloopsareidealforiteratingoversequenceslikelistsorarrays.2)Whileloopsaresuitableforscenarioswheretheloopcontinuesuntilaspecificcond
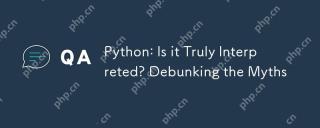
Pythonisnotpurelyinterpreted;itusesahybridapproachofbytecodecompilationandruntimeinterpretation.1)Pythoncompilessourcecodeintobytecode,whichisthenexecutedbythePythonVirtualMachine(PVM).2)Thisprocessallowsforrapiddevelopmentbutcanimpactperformance,req
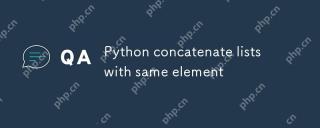
ToconcatenatelistsinPythonwiththesameelements,use:1)the operatortokeepduplicates,2)asettoremoveduplicates,or3)listcomprehensionforcontroloverduplicates,eachmethodhasdifferentperformanceandorderimplications.
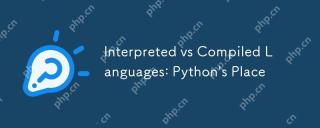
Pythonisaninterpretedlanguage,offeringeaseofuseandflexibilitybutfacingperformancelimitationsincriticalapplications.1)InterpretedlanguageslikePythonexecuteline-by-line,allowingimmediatefeedbackandrapidprototyping.2)CompiledlanguageslikeC/C transformt
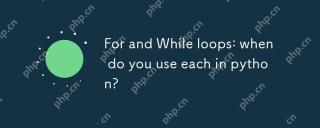
Useforloopswhenthenumberofiterationsisknowninadvance,andwhileloopswheniterationsdependonacondition.1)Forloopsareidealforsequenceslikelistsorranges.2)Whileloopssuitscenarioswheretheloopcontinuesuntilaspecificconditionismet,usefulforuserinputsoralgorit


Hot AI Tools

Undresser.AI Undress
AI-powered app for creating realistic nude photos

AI Clothes Remover
Online AI tool for removing clothes from photos.

Undress AI Tool
Undress images for free

Clothoff.io
AI clothes remover

Video Face Swap
Swap faces in any video effortlessly with our completely free AI face swap tool!

Hot Article

Hot Tools

SublimeText3 Linux new version
SublimeText3 Linux latest version
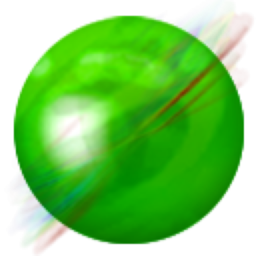
ZendStudio 13.5.1 Mac
Powerful PHP integrated development environment
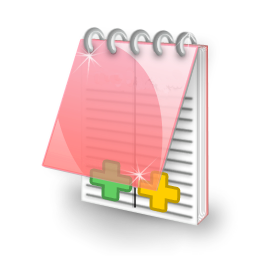
EditPlus Chinese cracked version
Small size, syntax highlighting, does not support code prompt function

SAP NetWeaver Server Adapter for Eclipse
Integrate Eclipse with SAP NetWeaver application server.

MantisBT
Mantis is an easy-to-deploy web-based defect tracking tool designed to aid in product defect tracking. It requires PHP, MySQL and a web server. Check out our demo and hosting services.
