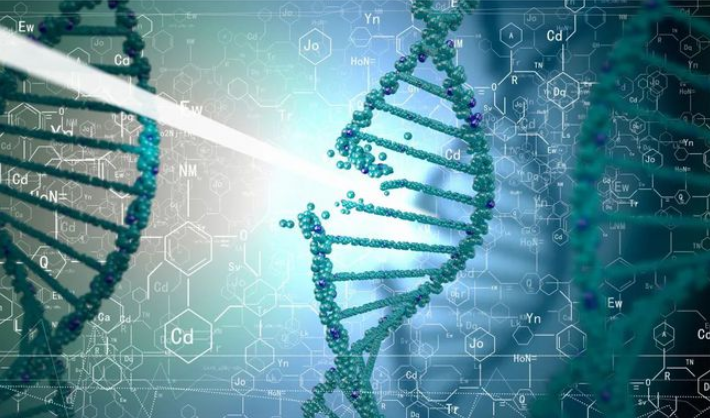
Editor: KXSpatial transcriptomics and multi-omics data integration
Spatial transcriptomics is a major development after single-cell transcriptomics, making the integration of multi-omics data crucial .
SpatialGlue: Graph Neural Network Model with Dual Attention Mechanism
A research team from the Singapore Agency for Science, Technology and Research (A*STAR), BGI and Renji Hospital Affiliated to Shanghai Jiao Tong University School of Medicine proposed a method called It is SpatialGlue's graph neural network model, which integrates multi-omics data through a dual attention mechanism to reveal the histologically relevant structure of tissue samples in a spatially aware manner.
Advantages of SpatialGlue
SpatialGlue is able to combine multiple data modalities with their respective spatial contexts. Compared with other methods, SpatialGlue has the following advantages:
- Capture more anatomical details.
- Resolve spatial domains (such as cerebral cortex) more accurately.
- Identify cell types located in different regions (such as spleen macrophage subpopulations).
A powerful tool for spatial omics analysis
This study highlights the powerful ability of multimodal spatial omics in analyzing biological complexity.
Related research
Related research was titled "Deciphering spatial domains from spatial multi-omics with SpatialGlue" and was published in "Nature Methods" on June 21.
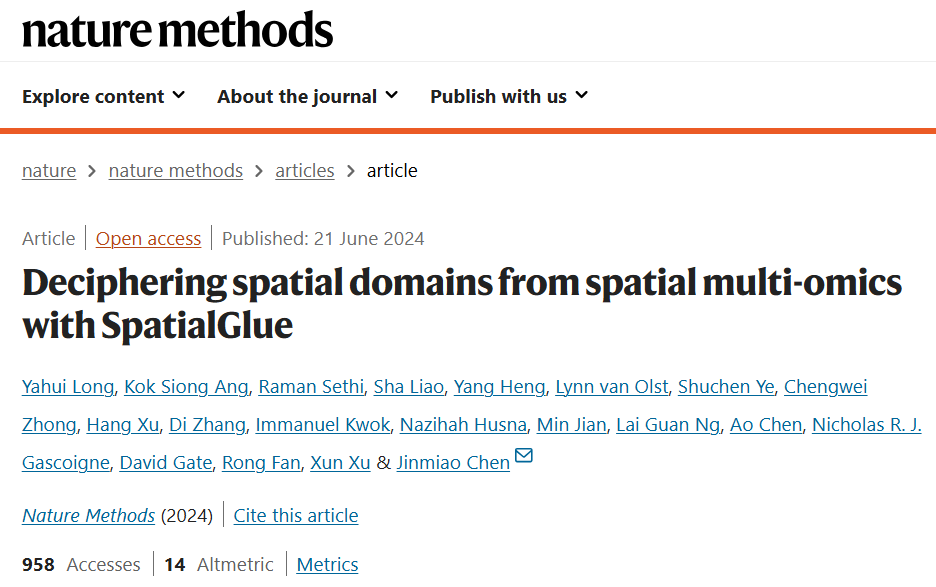
Spatial multi-omics data integration faces challenges. Currently, spatial technology is being extended to spatial multi-omics, that is, different omics are analyzed simultaneously on a single tissue section. These technologies can be broadly divided into two categories: sequencing-based and imaging-based. It provides the possibility to deeply understand the properties of cells and emerging tissues.
To fully exploit spatial multi-omics data to build a coherent picture of the tissue under study, spatially aware integration of heterogeneous data modalities is required. Multi-omics data integration faces significant challenges as feature counts of different modalities may vary greatly and have different statistical distributions. This challenge is exacerbated when combining spatial information with feature counts within each data modality.
Currently, there are no tools specifically designed for spatial multi-omics acquired from the same tissue section. Therefore, tools specifically tailored for spatial multi-omics data are needed to address the challenge of integrating spatial multi-omics data for downstream analysis. In particular, new methods enabling cross-omics integration of spatial perception are needed.
SpatialGlue model structure SpatialGlue interprets the spatial domain of tissue samples with higher resolution by effectively combining multi-omics modal data with spatial information. SpatialGlue is a deep learning model based on graph neural networks (GNN).
SpatialGlue first uses the k-nearest neighbor (KNN) algorithm to build a spatial neighbor graph using spatial coordinates and a feature neighbor graph using normalized expression data for each omics modality.
Then, for each modality, the GNN encoder takes the normalized representation and the neighbor graph to learn two graph-specific representations by iteratively aggregating the representations of neighbors. To capture the importance of different graphs, an intra-modal attention aggregation layer is designed to adaptively integrate graph-specific representations and obtain modality-specific representations.
Finally, to preserve the importance of different modalities, SpatialGlue uses an inter-modal attention aggregation layer to adaptively integrate modality-specific representations and output a final blob-integrated representation.
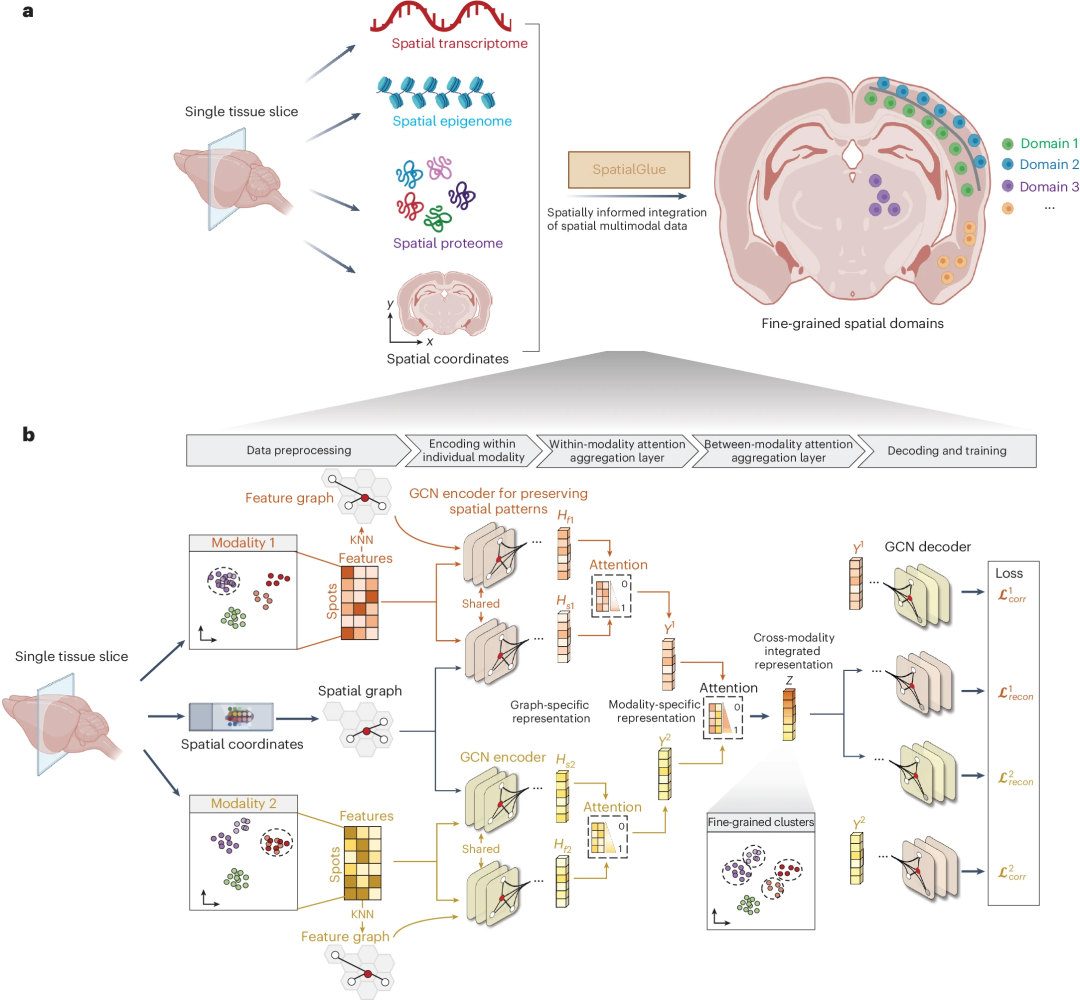
Illustration: Interpretable deep dual attention model for spatial multi-omics data analysis. (Source: paper) To evaluate the effectiveness of the proposed SpatialGlue model, the researchers first verified the importance of attention and other components through a series of ablation studies using simulated data. Subsequently, the sensitivity of SpatialGlue to the number of neighbors of the input, the principal component analysis (PCA) dimension, and the number of GNN layers are characterized.
Higher resolution captures more anatomical detail The researchers first tested SpatialGlue on simulated and experimentally acquired human lymph node data and conducted benchmarks. SpatialGlue's quantitative performance outperforms other methods and captures more anatomical detail.
Quantitative benchmarking demonstrates that SpatialGlue outperforms 10 state-of-the-art single-modality and non-spatial methods on 5 simulated and 12 real-world datasets, highlighting the importance of spatial information and cross-omics integration. importance.
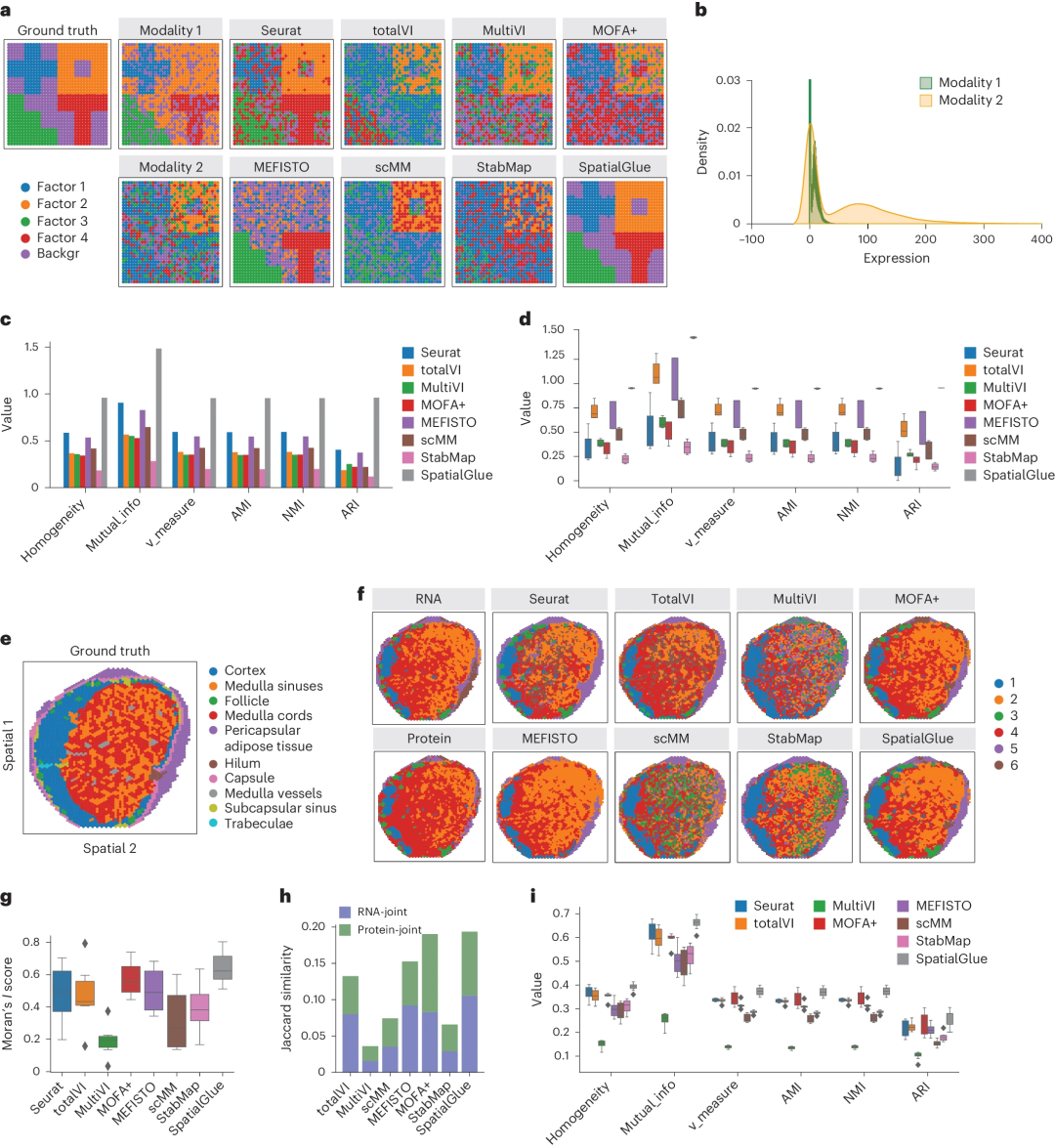
1. Illustration: SpatialGlue accurately identifies spatial domains in simulated and real data. (Source: paper)
- Next, SpatialGlue was applied to a mouse brain epigenome-transcriptome dataset, revealing finer cortical layers compared to the original study, which allows for further investigation of gene regulation at higher spatial resolution.

Illustration: SpatialGlue dissects the spatial epigenomic transcriptome of mouse brain samples at higher resolution. (Source: paper)
Finally, SpatialGlue was further applied to data acquired by Stereo-CITE-seq and SPOTS, demonstrating its broad applicability to various technology platforms. The researchers tested eight methods. Overall, SpatialGlue scores highest in Jaccard similarity and ranks second in Moran's I score. This excellent performance was further replicated using three additional mouse thymus sections.
The researchers said: "We believe that SpatialGlue will become a valuable analysis tool for spatial multi-omics data now and in the future. There are several possible ways to expand SpatialGlue. One of them is to use images as modalities. We plan to extend SpatialGlue, Merge image data with attentional aggregation layers within or between modalities. We also plan to extend the capabilities of SpatialGlue by integrating multi-omics data acquired from serial tissue sections.
Note: Cover comes from the Internet
.
The above is the detailed content of Integrating multi-omics data, BGI team’s graph neural network model SpatialGlue was published in Nature sub-journal. For more information, please follow other related articles on the PHP Chinese website!