


ICML 2024 | Signal representation is exponentially stronger, memory saving exceeds 35%, quantum implicit representation network is coming

The AIxiv column is a column where academic and technical content is published on this site. In the past few years, the AIxiv column of this site has received more than 2,000 reports, covering top laboratories from major universities and companies around the world, effectively promoting academic exchanges and dissemination. If you have excellent work that you want to share, please feel free to contribute or contact us for reporting. Submission email: liyazhou@jiqizhixin.com; zhaoyunfeng@jiqizhixin.com
Paper title: Quantum Implicit Neural Representations Paper authors: Jiaming Zhao, Wenbo Qiao, Peng Zhang*, Hui Gao Paper link: https://arxiv.org/abs /2406.03873
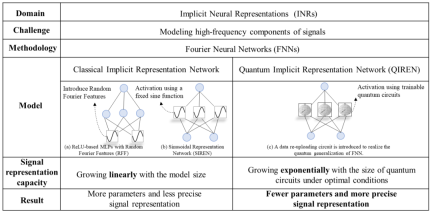
This work not only integrates quantum advantages into implicit neural representation, but also opens up a promising application direction for quantum neural networks - implicit neural representation. It is worth emphasizing that implicit neural representations have many other potential applications, such as representing scenes or 3D objects, time series prediction, and solving differential equations. For a large class of tasks that model continuous signals, we can consider introducing implicit representation networks as a basic component. Based on the theoretical and experimental foundations of this paper, we can extend QIREN to these applications in future work, and QIREN is expected to produce better results with fewer parameters in these fields. At the same time, we found a suitable application scenario for quantum machine learning. Thereby promoting further practical and innovative research within the quantum machine learning community.
The above is the detailed content of ICML 2024 | Signal representation is exponentially stronger, memory saving exceeds 35%, quantum implicit representation network is coming. For more information, please follow other related articles on the PHP Chinese website!
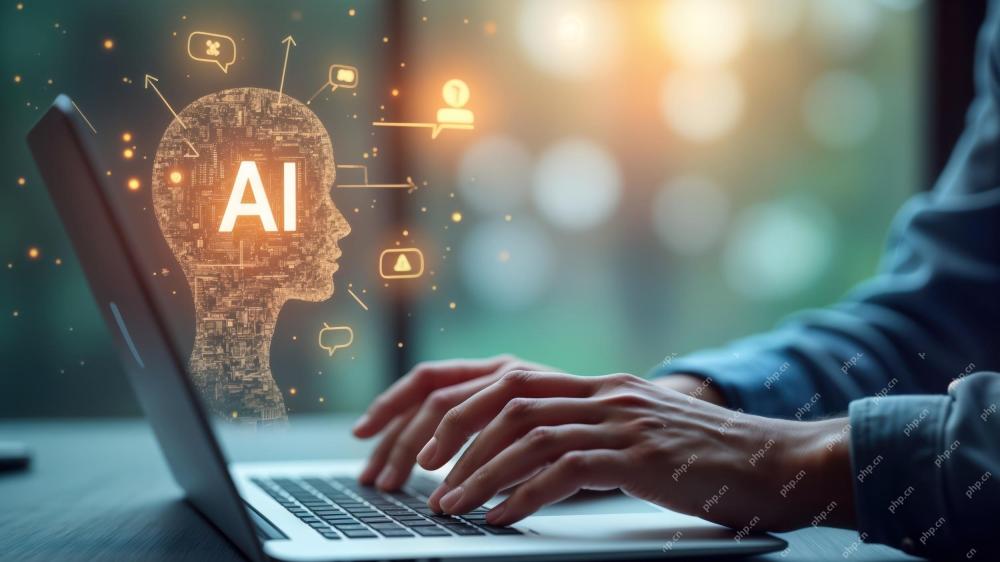
Generative AI, exemplified by chatbots like ChatGPT, offers project managers powerful tools to streamline workflows and ensure projects stay on schedule and within budget. However, effective use hinges on crafting the right prompts. Precise, detail
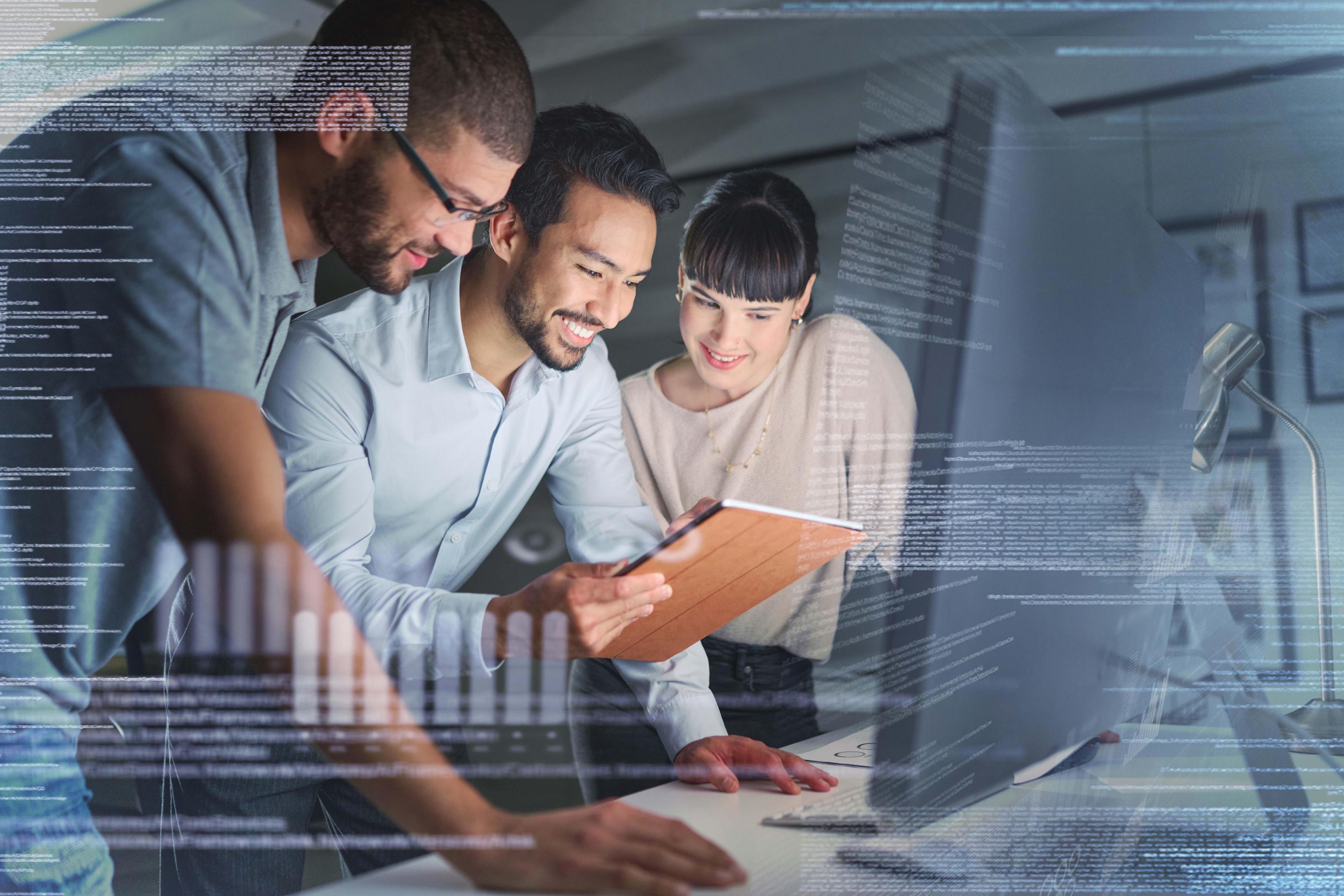
The challenge of defining Artificial General Intelligence (AGI) is significant. Claims of AGI progress often lack a clear benchmark, with definitions tailored to fit pre-determined research directions. This article explores a novel approach to defin
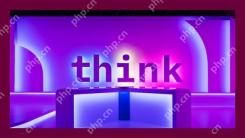
IBM Watsonx.data: Streamlining the Enterprise AI Data Stack IBM positions watsonx.data as a pivotal platform for enterprises aiming to accelerate the delivery of precise and scalable generative AI solutions. This is achieved by simplifying the compl
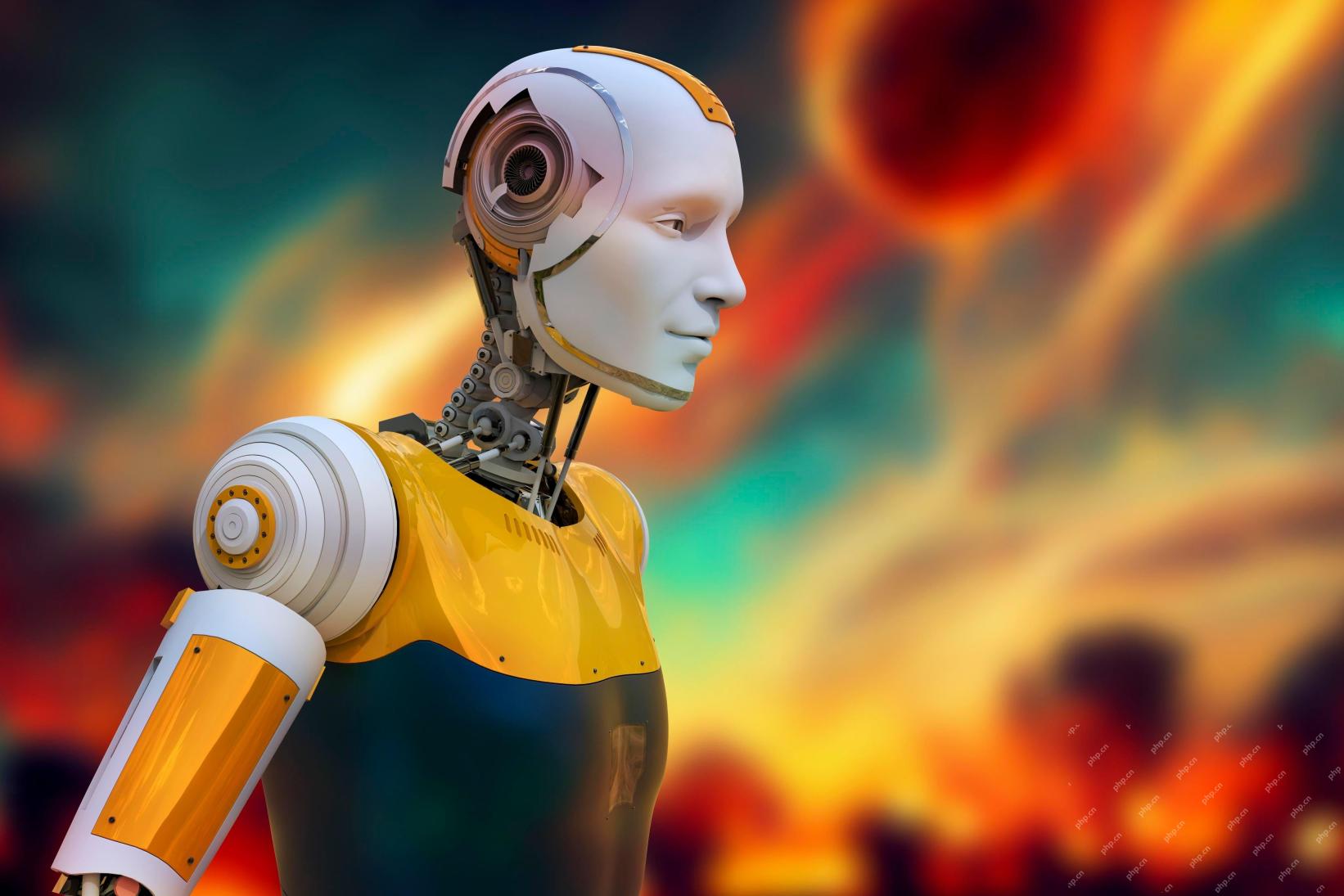
The rapid advancements in robotics, fueled by breakthroughs in AI and materials science, are poised to usher in a new era of humanoid robots. For years, industrial automation has been the primary focus, but the capabilities of robots are rapidly exp
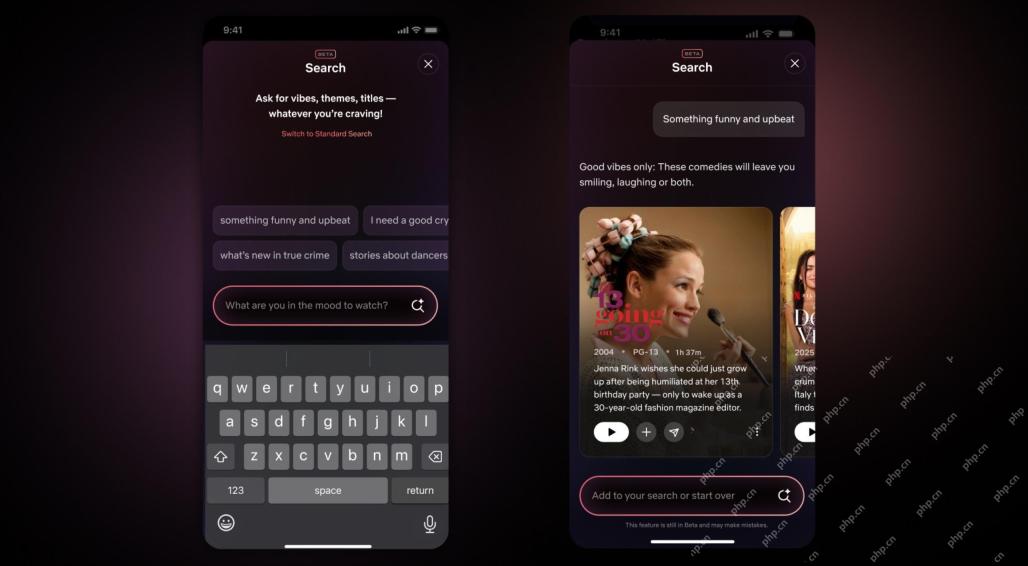
The biggest update of Netflix interface in a decade: smarter, more personalized, embracing diverse content Netflix announced its largest revamp of its user interface in a decade, not only a new look, but also adds more information about each show, and introduces smarter AI search tools that can understand vague concepts such as "ambient" and more flexible structures to better demonstrate the company's interest in emerging video games, live events, sports events and other new types of content. To keep up with the trend, the new vertical video component on mobile will make it easier for fans to scroll through trailers and clips, watch the full show or share content with others. This reminds you of the infinite scrolling and very successful short video website Ti
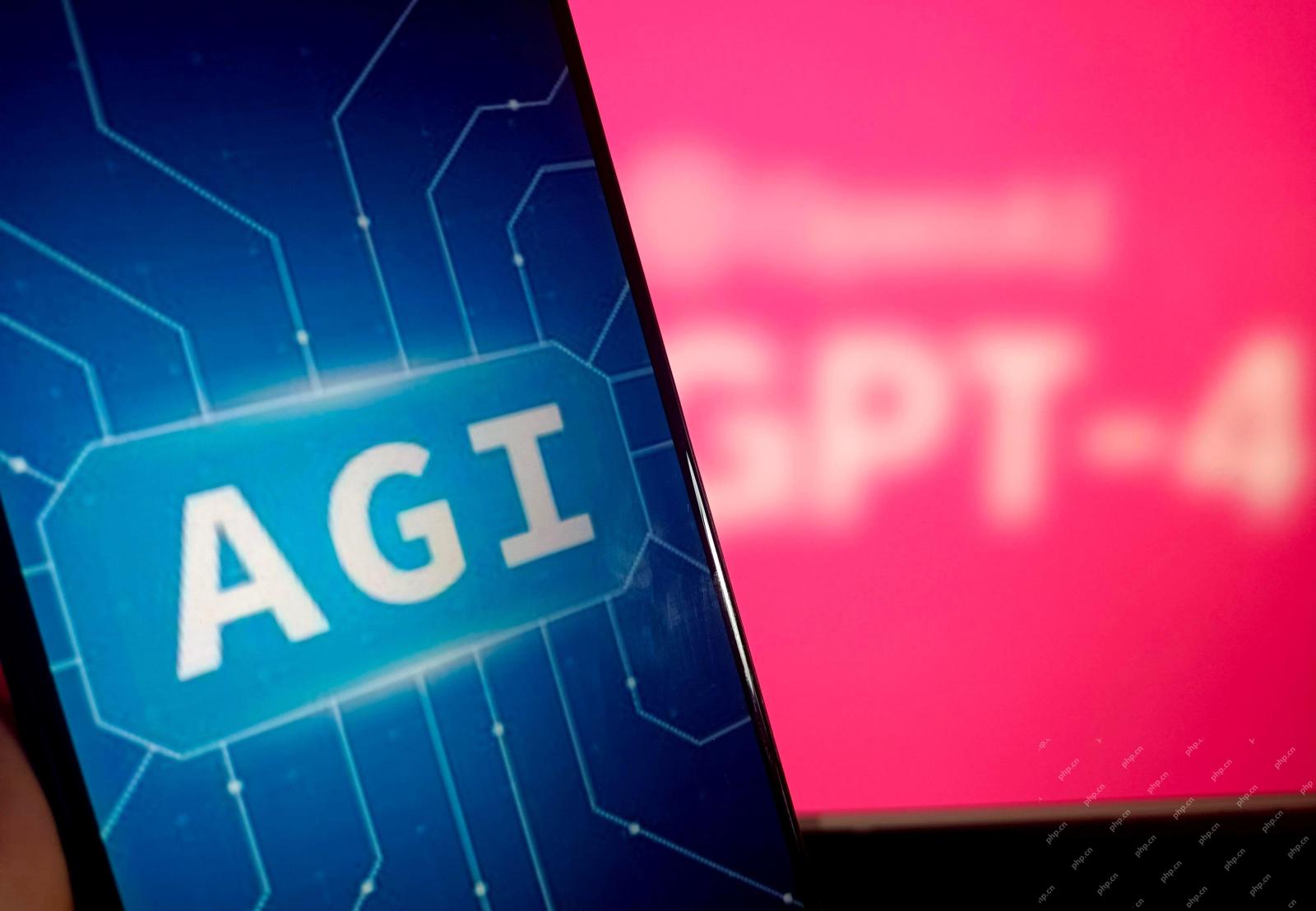
The growing discussion of general intelligence (AGI) in artificial intelligence has prompted many to think about what happens when artificial intelligence surpasses human intelligence. Whether this moment is close or far away depends on who you ask, but I don’t think it’s the most important milestone we should focus on. Which earlier AI milestones will affect everyone? What milestones have been achieved? Here are three things I think have happened. Artificial intelligence surpasses human weaknesses In the 2022 movie "Social Dilemma", Tristan Harris of the Center for Humane Technology pointed out that artificial intelligence has surpassed human weaknesses. What does this mean? This means that artificial intelligence has been able to use humans
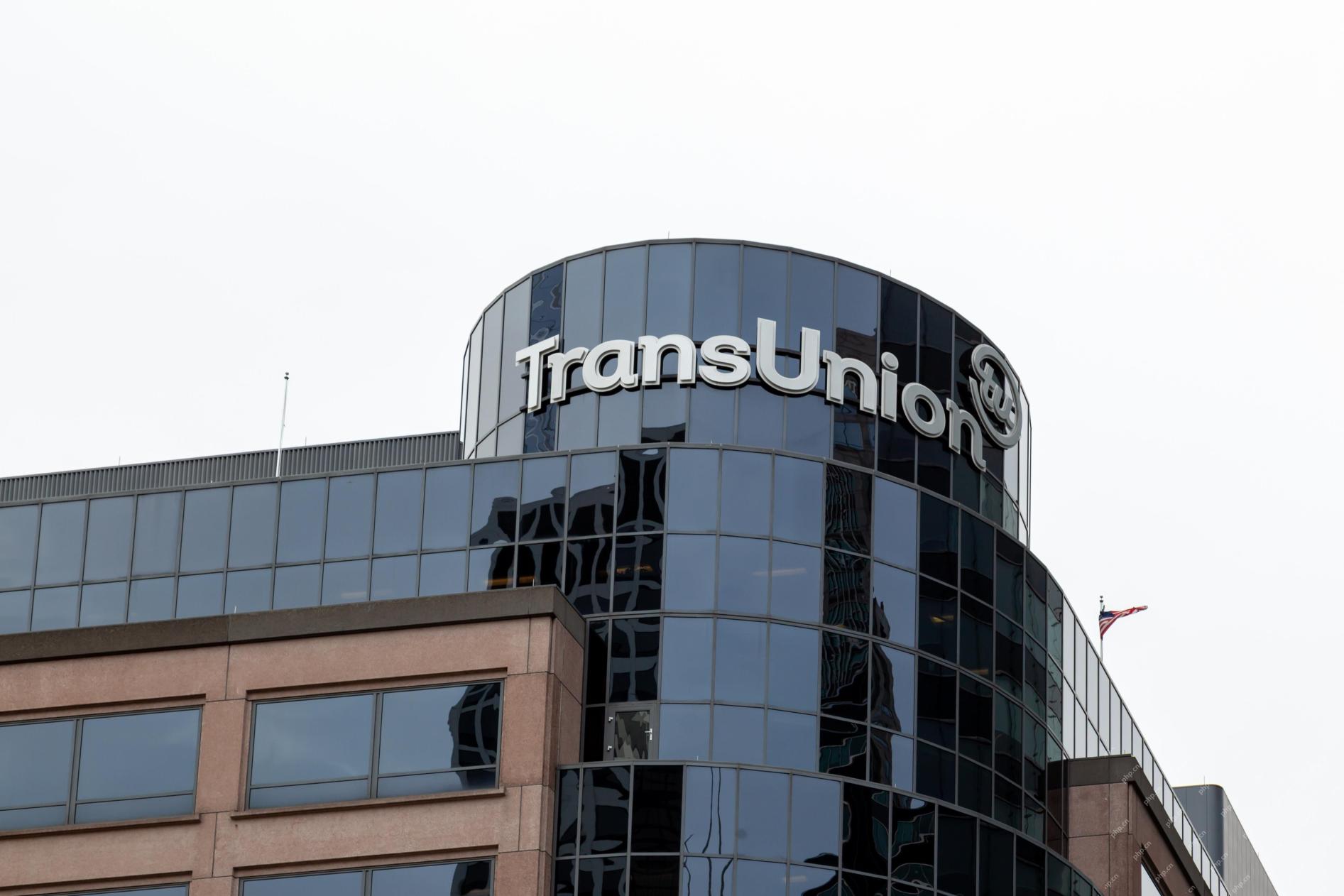
TransUnion's CTO, Ranganath Achanta, spearheaded a significant technological transformation since joining the company following its Neustar acquisition in late 2021. His leadership of over 7,000 associates across various departments has focused on u
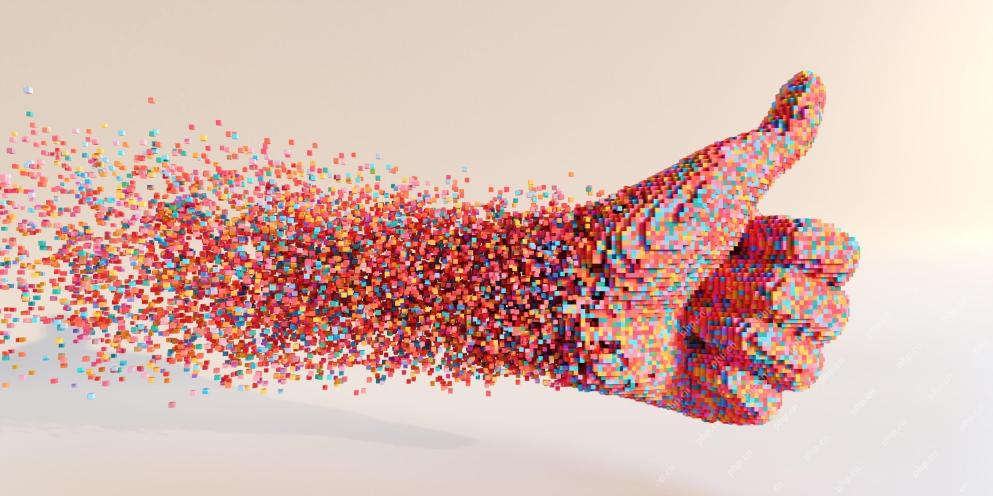
Building trust is paramount for successful AI adoption in business. This is especially true given the human element within business processes. Employees, like anyone else, harbor concerns about AI and its implementation. Deloitte researchers are sc


Hot AI Tools

Undresser.AI Undress
AI-powered app for creating realistic nude photos

AI Clothes Remover
Online AI tool for removing clothes from photos.

Undress AI Tool
Undress images for free

Clothoff.io
AI clothes remover

Video Face Swap
Swap faces in any video effortlessly with our completely free AI face swap tool!

Hot Article

Hot Tools

SAP NetWeaver Server Adapter for Eclipse
Integrate Eclipse with SAP NetWeaver application server.

mPDF
mPDF is a PHP library that can generate PDF files from UTF-8 encoded HTML. The original author, Ian Back, wrote mPDF to output PDF files "on the fly" from his website and handle different languages. It is slower than original scripts like HTML2FPDF and produces larger files when using Unicode fonts, but supports CSS styles etc. and has a lot of enhancements. Supports almost all languages, including RTL (Arabic and Hebrew) and CJK (Chinese, Japanese and Korean). Supports nested block-level elements (such as P, DIV),
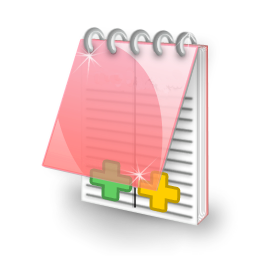
EditPlus Chinese cracked version
Small size, syntax highlighting, does not support code prompt function

SublimeText3 English version
Recommended: Win version, supports code prompts!

Zend Studio 13.0.1
Powerful PHP integrated development environment
