


Golang Machine Learning Applications: Building Intelligent Algorithms and Data-Driven Solutions
Use machine learning in Golang to develop intelligent algorithms and data-driven solutions: Install the Gonum library for machine learning algorithms and utilities. Linear regression using Gonum's LinearRegression model, a supervised learning algorithm. Train the model using training data, which contains input variables and target variables. Predict house prices based on new features, from which the model will extract a linear relationship.
Golang Machine Learning Applications: Building Intelligent Algorithms and Data-Driven Solutions
Introduction
In today’s data-driven era, machine learning (ML) has become an indispensable technology that allows us to extract insights from data and build intelligent algorithms. Using Golang for machine learning enables high-performance and scalable ML applications. In this tutorial, we’ll take a deep dive into how to use popular machine learning libraries in Golang to build intelligent algorithms and data-driven solutions.
Installation Library
First, we need to install Golang’s machine learning library. We recommend using the [Gonum library](https://pkg.go.dev/gonum.org/v1/gonum), which provides a wide range of ML algorithms and utilities. Run the following command to install:
go get gonum.org/v1/gonum
Practical Case: Linear Regression
As a practical case, we will build an application that uses the linear regression algorithm to predict housing prices. Linear regression is a supervised learning algorithm that learns a linear relationship between input variables and a target variable.
Define model
First, we need to define a LinearRegression
model, you can use in the
gonum library regression
Package:
import ( "gonum.org/v1/gonum/mat" "gonum.org/v1/gonum/stat/regression" ) type LinearRegression struct { model *regression.LinearRegression }
Training model
Next, we train the model using the training data. The training data contains house characteristics (such as square footage, number of bedrooms) and house prices.
func (r *LinearRegression) Train(data [][]float64, labels []float64) error { if len(data) == 0 || len(labels) == 0 { return errors.New("invalid data or labels") } x := mat.NewDense(len(data), len(data[0])) y := mat.NewVecDense(len(labels), labels) for i, row := range data { for j, value := range row { x.Set(i, j, value) } } r.model = regression.LinearRegression{} if err := r.model.Fit(x, y); err != nil { return err } return nil }
Predicting house prices
Once the model is trained, we can use new features to predict house prices:
func (r *LinearRegression) Predict(input []float64) (float64, error) { if len(input) != len(r.model.Predictors()) { return 0, errors.New("invalid input size") } x := mat.NewVecDense(len(input), input) return r.model.Predict(x), nil }
Conclusion
In this tutorial, we learned how to use machine learning libraries in Golang to build intelligent algorithms. We illustrate the process of model training and prediction by creating a practical case of a linear regression model. Golang, with its high performance and scalability, is ideal for building ML applications to solve complex real-world problems.
The above is the detailed content of Golang Machine Learning Applications: Building Intelligent Algorithms and Data-Driven Solutions. For more information, please follow other related articles on the PHP Chinese website!
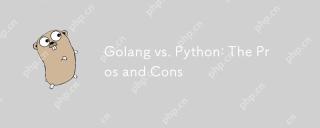
Golangisidealforbuildingscalablesystemsduetoitsefficiencyandconcurrency,whilePythonexcelsinquickscriptinganddataanalysisduetoitssimplicityandvastecosystem.Golang'sdesignencouragesclean,readablecodeanditsgoroutinesenableefficientconcurrentoperations,t
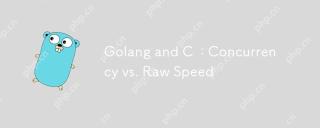
Golang is better than C in concurrency, while C is better than Golang in raw speed. 1) Golang achieves efficient concurrency through goroutine and channel, which is suitable for handling a large number of concurrent tasks. 2)C Through compiler optimization and standard library, it provides high performance close to hardware, suitable for applications that require extreme optimization.
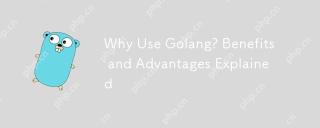
Reasons for choosing Golang include: 1) high concurrency performance, 2) static type system, 3) garbage collection mechanism, 4) rich standard libraries and ecosystems, which make it an ideal choice for developing efficient and reliable software.
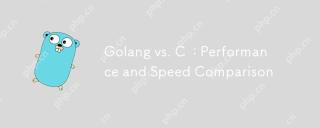
Golang is suitable for rapid development and concurrent scenarios, and C is suitable for scenarios where extreme performance and low-level control are required. 1) Golang improves performance through garbage collection and concurrency mechanisms, and is suitable for high-concurrency Web service development. 2) C achieves the ultimate performance through manual memory management and compiler optimization, and is suitable for embedded system development.
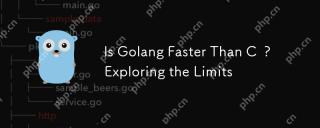
Golang performs better in compilation time and concurrent processing, while C has more advantages in running speed and memory management. 1.Golang has fast compilation speed and is suitable for rapid development. 2.C runs fast and is suitable for performance-critical applications. 3. Golang is simple and efficient in concurrent processing, suitable for concurrent programming. 4.C Manual memory management provides higher performance, but increases development complexity.
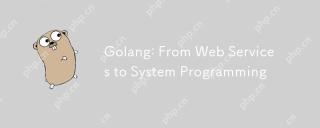
Golang's application in web services and system programming is mainly reflected in its simplicity, efficiency and concurrency. 1) In web services, Golang supports the creation of high-performance web applications and APIs through powerful HTTP libraries and concurrent processing capabilities. 2) In system programming, Golang uses features close to hardware and compatibility with C language to be suitable for operating system development and embedded systems.
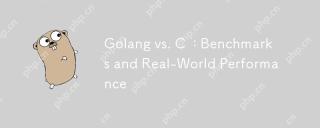
Golang and C have their own advantages and disadvantages in performance comparison: 1. Golang is suitable for high concurrency and rapid development, but garbage collection may affect performance; 2.C provides higher performance and hardware control, but has high development complexity. When making a choice, you need to consider project requirements and team skills in a comprehensive way.
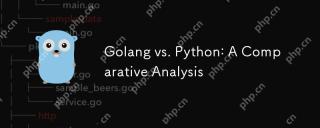
Golang is suitable for high-performance and concurrent programming scenarios, while Python is suitable for rapid development and data processing. 1.Golang emphasizes simplicity and efficiency, and is suitable for back-end services and microservices. 2. Python is known for its concise syntax and rich libraries, suitable for data science and machine learning.


Hot AI Tools

Undresser.AI Undress
AI-powered app for creating realistic nude photos

AI Clothes Remover
Online AI tool for removing clothes from photos.

Undress AI Tool
Undress images for free

Clothoff.io
AI clothes remover

Video Face Swap
Swap faces in any video effortlessly with our completely free AI face swap tool!

Hot Article

Hot Tools

Notepad++7.3.1
Easy-to-use and free code editor

DVWA
Damn Vulnerable Web App (DVWA) is a PHP/MySQL web application that is very vulnerable. Its main goals are to be an aid for security professionals to test their skills and tools in a legal environment, to help web developers better understand the process of securing web applications, and to help teachers/students teach/learn in a classroom environment Web application security. The goal of DVWA is to practice some of the most common web vulnerabilities through a simple and straightforward interface, with varying degrees of difficulty. Please note that this software

MantisBT
Mantis is an easy-to-deploy web-based defect tracking tool designed to aid in product defect tracking. It requires PHP, MySQL and a web server. Check out our demo and hosting services.

SublimeText3 Chinese version
Chinese version, very easy to use

mPDF
mPDF is a PHP library that can generate PDF files from UTF-8 encoded HTML. The original author, Ian Back, wrote mPDF to output PDF files "on the fly" from his website and handle different languages. It is slower than original scripts like HTML2FPDF and produces larger files when using Unicode fonts, but supports CSS styles etc. and has a lot of enhancements. Supports almost all languages, including RTL (Arabic and Hebrew) and CJK (Chinese, Japanese and Korean). Supports nested block-level elements (such as P, DIV),