本文介紹了實現一個稀疏混合專家語言模型(MoE)的方法,詳細解釋了模型的實施過程,包括採用稀疏混合專家取代傳統的前饋神經網路,實現top-k 門控和雜訊的top-k 門控,以及採用Kaiming He 初始化技術。作者也說明了從 makemore 架構保持不變的元素,例如資料集處理、分詞預處理和語言建模任務。最後還提供了一個 GitHub 倉庫鏈接,用於實現模型的整個過程,是一本不可多得的實戰教科書。
內容簡介
混合專家模型(MoE)在發布後開始受到廣泛關注,特別是在稀疏化的混合專家語言模型中。雖然大部分的組件與傳統transformers相似,但稀疏混合專家語言模型的訓練穩定性存在一些問題,儘管看起來相對簡單。
這種可設定的小規模稀疏 MoE 實作方法在Hugging Face的一篇部落格中被介紹了出來,這對於想要進行新方法快速試驗的研究者來說可能非常有幫助。部落格還提供了基於PyTorch的詳細程式碼,可以在此連結找到:https://github.com/AviSoori1x/makeMoE/tree/main。這樣的小規模實現有助於研究者們在這個領域進行快速試驗。
本站對此進行了整理,以颯讀者。
本文在makemore 架構的基礎上,進行了幾處變更:
使用稀疏混合專家取代單獨的前饋神經網路;
Top-k 閘控和雜訊的Top-k 閘控;
參數初始化使用了Kaiming He 初始化方法,但本文重點是可自訂初始化方法,包括選擇Xavier/Glorot 等初始化方法。
同時,以下模組與makemore 保持一致:
#資料集、預處理(分詞)部分以及Andrej 最初選擇的語言建模任務- 產生莎士比亞文風的文本內容
Casusal 自註意力機制
訓練循環
因果縮放點積注意力機制
#下面這段程式碼展示了自註意力機制的基本概念,並且著重於使用經典的縮放點積自註意力(scaled dot product self-attention.)實現。在這個自註意力變體機制中,查詢矩陣、鍵矩陣和值矩陣都來自相同的輸入序列。同時為了確保自回歸語言生成過程的完整性,特別是在純解碼器模型中,使用了一種遮罩機制。
這種遮罩機制非常關鍵,因為它可以掩蓋當前 token 所處位置之後的任何訊息,從而引導模型只專注於序列的前面部分。這種了遮擋 token 後面內容的注意力稱為因果自註意力。值得注意的是,稀疏混合專家模型並不局限於僅限解碼器的 Transformer 架構。事實上,這一領域的許多重要的成果都是圍繞著 T5 架構展開的,T5 架構也包含了 Transformer 模型中的編碼器和解碼器元件。
#This code is borrowed from Andrej Karpathy's makemore repository linked in the repo.The self attention layers in Sparse mixture of experts models are the same asin regular transformer modelstorch.manual_seed(1337)B,T,C = 4,8,32 # batch, time, channelsx = torch.randn(B,T,C)# let's see a single Head perform self-attentionhead_size = 16key = nn.Linear(C, head_size, bias=False)query = nn.Linear(C, head_size, bias=False)value = nn.Linear(C, head_size, bias=False)k = key(x) # (B, T, 16)q = query(x) # (B, T, 16)wei =q @ k.transpose(-2, -1) # (B, T, 16) @ (B, 16, T) ---> (B, T, T)tril = torch.tril(torch.ones(T, T))#wei = torch.zeros((T,T))wei = wei.masked_fill(tril == 0, float('-inf'))wei = F.softmax(wei, dim=-1) #B,T,Tv = value(x) #B,T,Hout = wei @ v # (B,T,T) @ (B,T,H) -> (B,T,H)out.shape###
torch.Size([4, 8, 16])######然後,因果自註意力和多頭因果自註意力的程式碼可整理如下。多頭自註意力並行應用多個注意力頭,每個注意力頭單獨關注通道的一個部分(嵌入維度)。多頭自註意力從本質上改善了學習過程,並由於其固有的並行能力提高了模型訓練的效率。下面這段程式碼使用了 dropout 來進行正規化,來防止過度擬合。 ######
#Causal scaled dot product self-Attention Headn_embd = 64n_head = 4n_layer = 4head_size = 16dropout = 0.1class Head(nn.Module):""" one head of self-attention """def __init__(self, head_size):super().__init__()self.key = nn.Linear(n_embd, head_size, bias=False)self.query = nn.Linear(n_embd, head_size, bias=False)self.value = nn.Linear(n_embd, head_size, bias=False)self.register_buffer('tril', torch.tril(torch.ones(block_size, block_size)))self.dropout = nn.Dropout(dropout)def forward(self, x):B,T,C = x.shapek = self.key(x) # (B,T,C)q = self.query(x) # (B,T,C)# compute attention scores ("affinities")wei = q @ k.transpose(-2,-1) * C**-0.5 # (B, T, C) @ (B, C, T) -> (B, T, T)wei = wei.masked_fill(self.tril[:T, :T] == 0, float('-inf')) # (B, T, T)wei = F.softmax(wei, dim=-1) # (B, T, T)wei = self.dropout(wei)# perform the weighted aggregation of the valuesv = self.value(x) # (B,T,C)out = wei @ v # (B, T, T) @ (B, T, C) -> (B, T, C) return out######多頭自註意力的實作方式如下:######
#Multi-Headed Self Attentionclass MultiHeadAttention(nn.Module):""" multiple heads of self-attention in parallel """def __init__(self, num_heads, head_size):super().__init__()self.heads = nn.ModuleList([Head(head_size) for _ in range(num_heads)])self.proj = nn.Linear(n_embd, n_embd)self.dropout = nn.Dropout(dropout)def forward(self, x):out = torch.cat([h(x) for h in self.heads], dim=-1)out = self.dropout(self.proj(out)) return out#########建立一個專家模組######## ####即一個簡單的多層感知器#########在稀疏混合專家架構中,每個transformer 區塊內的自註意力機制保持不變。不過,每個區塊的結構發生了巨大的變化:標準的前饋###神經網路###被多個稀疏活化的前饋網路(即專家網路)所取代。所謂“稀疏激活”,是指序列中的每個 token 只被分配給有限數量的專家(通常是一個或兩個)。 ###
这有助于提高训练和推理速度,因为每次前向传递都会激活少数专家。不过,所有专家都必须存在 GPU 内存中,因此当参数总数达到数千亿甚至数万亿时,就会产生部署方面的问题。
#Expert moduleclass Expert(nn.Module):""" An MLP is a simple linear layer followed by a non-linearity i.e. each Expert """def __init__(self, n_embd):super().__init__()self.net = nn.Sequential(nn.Linear(n_embd, 4 * n_embd),nn.ReLU(),nn.Linear(4 * n_embd, n_embd),nn.Dropout(dropout),)def forward(self, x): return self.net(x)
Top-k 门控的一个例子
门控网络,也称为路由,确定哪个专家网络接收来自多头注意力的 token 的输出。举个例子解释路由的机制,假设有 4 个专家,token 需要被路由到前 2 个专家中。首先需要通过线性层将 token 输入到门控网络中。该层将对应于(Batch size,Tokens,n_embed)的输入张量从(2,4,32)维度,投影到对应于(Batch size、Tokens,num_expert)的新形状:(2、4,4)。其中 n_embed 是输入的通道维度,num_experts 是专家网络的计数。
接下来,沿最后一个维度,找出最大的前两个值及其相应的索引。
#Understanding how gating worksnum_experts = 4top_k=2n_embed=32#Example multi-head attention output for a simple illustrative example, consider n_embed=32, context_length=4 and batch_size=2mh_output = torch.randn(2, 4, n_embed)topkgate_linear = nn.Linear(n_embed, num_experts) # nn.Linear(32, 4)logits = topkgate_linear(mh_output)top_k_logits, top_k_indices = logits.topk(top_k, dim=-1)# Get top-k expertstop_k_logits, top_k_indices
#output:(tensor([[[ 0.0246, -0.0190],[ 0.1991,0.1513],[ 0.9749,0.7185],[ 0.4406, -0.8357]], [[ 0.6206, -0.0503],[ 0.8635,0.3784],[ 0.6828,0.5972],[ 0.4743,0.3420]]], grad_fn=<TopkBackward0>), tensor([[[2, 3],[2, 1],[3, 1],[2, 1]], [[0, 2], [0, 3], [3, 2], [3, 0]]]))
通过仅保留沿最后一个维度进行比较的前 k 大的值,来获得稀疏门控的输出。用负无穷值填充其余部分,在使用 softmax 激活函数。负无穷会被映射至零,而最大的前两个值会更加突出,且和为 1。要求和为 1 是为了对专家输出的内容进行加权。
zeros = torch.full_like(logits, float('-inf')) #full_like clones a tensor and fills it with a specified value (like infinity) for masking or calculations.sparse_logits = zeros.scatter(-1, top_k_indices, top_k_logits)sparse_logits
#outputtensor([[[ -inf,-inf,0.0246, -0.0190], [ -inf,0.1513,0.1991,-inf], [ -inf,0.7185,-inf,0.9749], [ -inf, -0.8357,0.4406,-inf]],[[ 0.6206,-inf, -0.0503,-inf], [ 0.8635,-inf,-inf,0.3784], [ -inf,-inf,0.5972,0.6828], [ 0.3420,-inf,-inf,0.4743]]], grad_fn=<ScatterBackward0>)
gating_output= F.softmax(sparse_logits, dim=-1)gating_output
#ouputtensor([[[0.0000, 0.0000, 0.5109, 0.4891], [0.0000, 0.4881, 0.5119, 0.0000], [0.0000, 0.4362, 0.0000, 0.5638], [0.0000, 0.2182, 0.7818, 0.0000]],[[0.6617, 0.0000, 0.3383, 0.0000], [0.6190, 0.0000, 0.0000, 0.3810], [0.0000, 0.0000, 0.4786, 0.5214], [0.4670, 0.0000, 0.0000, 0.5330]]], grad_fn=<SoftmaxBackward0>)
使用有噪声的 top-k 门控以实现负载平衡
# First define the top k router moduleclass TopkRouter(nn.Module):def __init__(self, n_embed, num_experts, top_k):super(TopkRouter, self).__init__()self.top_k = top_kself.linear =nn.Linear(n_embed, num_experts) def forward(self, mh_ouput):# mh_ouput is the output tensor from multihead self attention blocklogits = self.linear(mh_output)top_k_logits, indices = logits.topk(self.top_k, dim=-1)zeros = torch.full_like(logits, float('-inf'))sparse_logits = zeros.scatter(-1, indices, top_k_logits)router_output = F.softmax(sparse_logits, dim=-1) return router_output, indices
接下来使用下面这段代码来测试程序:
#Testing this out:num_experts = 4top_k = 2n_embd = 32mh_output = torch.randn(2, 4, n_embd)# Example inputtop_k_gate = TopkRouter(n_embd, num_experts, top_k)gating_output, indices = top_k_gate(mh_output)gating_output.shape, gating_output, indices#And it works!!
#output(torch.Size([2, 4, 4]), tensor([[[0.5284, 0.0000, 0.4716, 0.0000],[0.0000, 0.4592, 0.0000, 0.5408],[0.0000, 0.3529, 0.0000, 0.6471],[0.3948, 0.0000, 0.0000, 0.6052]], [[0.0000, 0.5950, 0.4050, 0.0000], [0.4456, 0.0000, 0.5544, 0.0000], [0.7208, 0.0000, 0.0000, 0.2792], [0.0000, 0.0000, 0.5659, 0.4341]]], grad_fn=<SoftmaxBackward0>), tensor([[[0, 2],[3, 1],[3, 1],[3, 0]], [[1, 2], [2, 0], [0, 3], [2, 3]]]))
尽管最近发布的 mixtral 的论文没有提到这一点,但本文的作者相信有噪声的 Top-k 门控机制是训练 MoE 模型的一个重要工具。从本质上讲,不会希望所有的 token 都发送给同一组「受欢迎」的专家网络。人们需要的是能在开发和探索之间取得良好平衡。为此,为了负载平衡,从门控的线性层向 logits 激活函数添加标准正态噪声是有帮助的,这使训练更有效率。
#Changing the above to accomodate noisy top-k gatingclass NoisyTopkRouter(nn.Module):def __init__(self, n_embed, num_experts, top_k):super(NoisyTopkRouter, self).__init__()self.top_k = top_k#layer for router logitsself.topkroute_linear = nn.Linear(n_embed, num_experts)self.noise_linear =nn.Linear(n_embed, num_experts)def forward(self, mh_output):# mh_ouput is the output tensor from multihead self attention blocklogits = self.topkroute_linear(mh_output)#Noise logitsnoise_logits = self.noise_linear(mh_output)#Adding scaled unit gaussian noise to the logitsnoise = torch.randn_like(logits)*F.softplus(noise_logits)noisy_logits = logits + noisetop_k_logits, indices = noisy_logits.topk(self.top_k, dim=-1)zeros = torch.full_like(noisy_logits, float('-inf'))sparse_logits = zeros.scatter(-1, indices, top_k_logits)router_output = F.softmax(sparse_logits, dim=-1) return router_output, indices
再次尝试代码:
#Testing this out, again:num_experts = 8top_k = 2n_embd = 16mh_output = torch.randn(2, 4, n_embd)# Example inputnoisy_top_k_gate = NoisyTopkRouter(n_embd, num_experts, top_k)gating_output, indices = noisy_top_k_gate(mh_output)gating_output.shape, gating_output, indices#It works!!
#output(torch.Size([2, 4, 8]), tensor([[[0.4181, 0.0000, 0.5819, 0.0000, 0.0000, 0.0000, 0.0000, 0.0000],[0.4693, 0.5307, 0.0000, 0.0000, 0.0000, 0.0000, 0.0000, 0.0000],[0.0000, 0.4985, 0.5015, 0.0000, 0.0000, 0.0000, 0.0000, 0.0000],[0.0000, 0.0000, 0.0000, 0.2641, 0.0000, 0.7359, 0.0000, 0.0000]], [[0.0000, 0.0000, 0.0000, 0.6301, 0.0000, 0.3699, 0.0000, 0.0000], [0.0000, 0.0000, 0.0000, 0.4766, 0.0000, 0.0000, 0.0000, 0.5234], [0.0000, 0.0000, 0.0000, 0.6815, 0.0000, 0.0000, 0.3185, 0.0000], [0.4482, 0.5518, 0.0000, 0.0000, 0.0000, 0.0000, 0.0000, 0.0000]]], grad_fn=<SoftmaxBackward0>), tensor([[[2, 0],[1, 0],[2, 1],[5, 3]], [[3, 5], [7, 3], [3, 6], [1, 0]]]))
创建稀疏化的混合专家模块
在获得门控网络的输出结果之后,对于给定的 token,将前 k 个值选择性地与来自相应的前 k 个专家的输出相乘。这种选择性乘法的结果是一个加权和,该加权和构成 SparseMoe 模块的输出。这个过程的关键和难点是避免不必要的乘法运算,只为前 k 名专家进行正向转播。为每个专家执行前向传播将破坏使用稀疏 MoE 的目的,因为这个过程将不再是稀疏的。
class SparseMoE(nn.Module):def __init__(self, n_embed, num_experts, top_k):super(SparseMoE, self).__init__()self.router = NoisyTopkRouter(n_embed, num_experts, top_k)self.experts = nn.ModuleList([Expert(n_embed) for _ in range(num_experts)])self.top_k = top_kdef forward(self, x):gating_output, indices = self.router(x)final_output = torch.zeros_like(x)# Reshape inputs for batch processingflat_x = x.view(-1, x.size(-1))flat_gating_output = gating_output.view(-1, gating_output.size(-1))# Process each expert in parallelfor i, expert in enumerate(self.experts):# Create a mask for the inputs where the current expert is in top-kexpert_mask = (indices == i).any(dim=-1)flat_mask = expert_mask.view(-1)if flat_mask.any():expert_input = flat_x[flat_mask]expert_output = expert(expert_input)# Extract and apply gating scoresgating_scores = flat_gating_output[flat_mask, i].unsqueeze(1)weighted_output = expert_output * gating_scores# Update final output additively by indexing and addingfinal_output[expert_mask] += weighted_output.squeeze(1) return final_output
运行以下代码来用样本测试上述实现,可以看到确实如此!
import torchimport torch.nn as nn#Let's test this outnum_experts = 8top_k = 2n_embd = 16dropout=0.1mh_output = torch.randn(4, 8, n_embd)# Example multi-head attention outputsparse_moe = SparseMoE(n_embd, num_experts, top_k)final_output = sparse_moe(mh_output)print("Shape of the final output:", final_output.shape)
Shape of the final output: torch.Size([4, 8, 16])
需要强调的是,如上代码所示,从路由 / 门控网络输出的 top_k 本身也很重要。索引确定了被激活的专家是哪些, 对应的值又决定了权重大小。下图进一步解释了加权求和的概念。
模块整合
将多头自注意力和稀疏混合专家相结合,形成稀疏混合专家 transformer 块。就像在 vanilla transformer 块中一样,也要使用残差以确保训练稳定,并避免梯度消失等问题。此外,要采用层归一化来进一步稳定学习过程。
#Create a self attention + mixture of experts block, that may be repeated several number of timesclass Block(nn.Module):""" Mixture of Experts Transformer block: communication followed by computation (multi-head self attention + SparseMoE) """def __init__(self, n_embed, n_head, num_experts, top_k):# n_embed: embedding dimension, n_head: the number of heads we'd likesuper().__init__()head_size = n_embed // n_headself.sa = MultiHeadAttention(n_head, head_size)self.smoe = SparseMoE(n_embed, num_experts, top_k)self.ln1 = nn.LayerNorm(n_embed)self.ln2 = nn.LayerNorm(n_embed)def forward(self, x):x = x + self.sa(self.ln1(x))x = x + self.smoe(self.ln2(x)) return x
最后,将所有内容整合在一起,形成稀疏混合专家语言模型。
class SparseMoELanguageModel(nn.Module):def __init__(self):super().__init__()# each token directly reads off the logits for the next token from a lookup table self.token_embedding_table = nn.Embedding(vocab_size, n_embed) self.position_embedding_table = nn.Embedding(block_size, n_embed)self.blocks = nn.Sequential(*[Block(n_embed, n_head=n_head, num_experts=num_experts,top_k=top_k) for _ in range(n_layer)])self.ln_f = nn.LayerNorm(n_embed) # final layer normself.lm_head = nn.Linear(n_embed, vocab_size)def forward(self, idx, targets=None):B, T = idx.shape# idx and targets are both (B,T) tensor of integerstok_emb = self.token_embedding_table(idx) # (B,T,C)pos_emb = self.position_embedding_table(torch.arange(T, device=device)) # (T,C)x = tok_emb + pos_emb # (B,T,C)x = self.blocks(x) # (B,T,C)x = self.ln_f(x) # (B,T,C)logits = self.lm_head(x) # (B,T,vocab_size)if targets is None:loss = Noneelse:B, T, C = logits.shapelogits = logits.view(B*T, C)targets = targets.view(B*T)loss = F.cross_entropy(logits, targets)return logits, lossdef generate(self, idx, max_new_tokens):# idx is (B, T) array of indices in the current contextfor _ in range(max_new_tokens):# crop idx to the last block_size tokensidx_cond = idx[:, -block_size:]# get the predictionslogits, loss = self(idx_cond)# focus only on the last time steplogits = logits[:, -1, :] # becomes (B, C)# apply softmax to get probabilitiesprobs = F.softmax(logits, dim=-1) # (B, C)# sample from the distributionidx_next = torch.multinomial(probs, num_samples=1) # (B, 1)# append sampled index to the running sequenceidx = torch.cat((idx, idx_next), dim=1) # (B, T+1) return idx
参数初始化对于深度神经网络的高效训练非常重要。由于专家中存在 ReLU 激活,因此这里使用了 Kaiming He 初始化。也可以尝试在 transformer 中更常用的 Glorot 初始化。杰里米 - 霍华德(Jeremy Howard)的《Fastai》第 2 部分有一个从头开始实现这些功能的精彩讲座:https://course.fast.ai/Lessons/lesson17.html
Glorot 参数初始化通常被用于 transformer 模型,因此这是一个可能提高模型性能的方法。
def kaiming_init_weights(m):if isinstance (m, (nn.Linear)): init.kaiming_normal_(m.weight)model = SparseMoELanguageModel()model.apply(kaiming_init_weights)
本文作者使用 mlflow 跟踪并记录重要指标和训练超参数。
#Using MLFlowm = model.to(device)# print the number of parameters in the modelprint(sum(p.numel() for p in m.parameters())/1e6, 'M parameters')# create a PyTorch optimizeroptimizer = torch.optim.AdamW(model.parameters(), lr=learning_rate)#mlflow.set_experiment("makeMoE")with mlflow.start_run():#If you use mlflow.autolog() this will be automatically logged. I chose to explicitly log here for completenessparams = {"batch_size": batch_size , "block_size" : block_size, "max_iters": max_iters, "eval_interval": eval_interval,"learning_rate": learning_rate, "device": device, "eval_iters": eval_iters, "dropout" : dropout, "num_experts": num_experts, "top_k": top_k }mlflow.log_params(params)for iter in range(max_iters):# every once in a while evaluate the loss on train and val setsif iter % eval_interval == 0 or iter == max_iters - 1:losses = estimate_loss()print(f"step {iter}: train loss {losses['train']:.4f}, val loss {losses['val']:.4f}")metrics = {"train_loss": losses['train'], "val_loss": losses['val']}mlflow.log_metrics(metrics, step=iter)# sample a batch of dataxb, yb = get_batch('train')# evaluate the losslogits, loss = model(xb, yb)optimizer.zero_grad(set_to_none=True)loss.backward()optimizer.step()
8.996545 M parametersstep 0: train loss 5.3223, val loss 5.3166step 100: train loss 2.7351, val loss 2.7429step 200: train loss 2.5125, val loss 2.5233...step 4999: train loss 1.5712, val loss 1.7508
记录训练和验证损失可以很好地指示训练的进展情况。该图显示,可能应该在 4500 次时停止(当验证损失稍微增加时)
接下来可以使用这个模型逐字符自回归地生成文本。
# generate from the model. Not great. Not too bad eithercontext = torch.zeros((1, 1), dtype=torch.long, device=device)print(decode(m.generate(context, max_new_tokens=2000)[0].tolist()))
DUKE VINCENVENTIO:If it ever fecond he town sue kigh now,That thou wold'st is steen 't.SIMNA:Angent her; no, my a born Yorthort,Romeoos soun and lawf to your sawe with ch a woft ttastly defy,To declay the soul art; and meart smad.CORPIOLLANUS:Which I cannot shall do from by born und ot cold warrike,What king we best anone wrave's going of heard and goodThus playvage; you have wold the grace....
本文参考内容:
在实施过程中,作者大量参考了以下出版物:
混合专家模型:https://arxiv.org/pdf/2401.04088.pdf
超大型神经网络:稀疏门控混合专家层:https://arxiv.org/pdf/1701.06538.pdf
来自 Andrej Karpathy 的原始 makemore 实现:https://github.com/karpathy/makemore
还可以尝试以下几种方法,来提高模型性能:
提高混合专家模块的效率;
尝试不同的神经网络初始化策略;
从字符级到子词级的分词;
对专家数量和 k 的取值(每个 token 激活的专家数量)进行贝叶斯超参数搜索。这可以归类为神经架构搜索。
优化专家能力。
以上是手把手教你,從零開始實作一個稀疏混合專家架構語言模型(MoE)的詳細內容。更多資訊請關注PHP中文網其他相關文章!
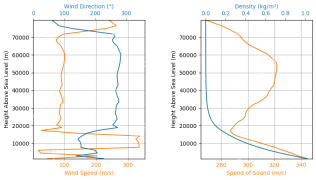
模擬火箭發射的火箭發射:綜合指南 本文指導您使用強大的Python庫Rocketpy模擬高功率火箭發射。 我們將介紹從定義火箭組件到分析模擬的所有內容
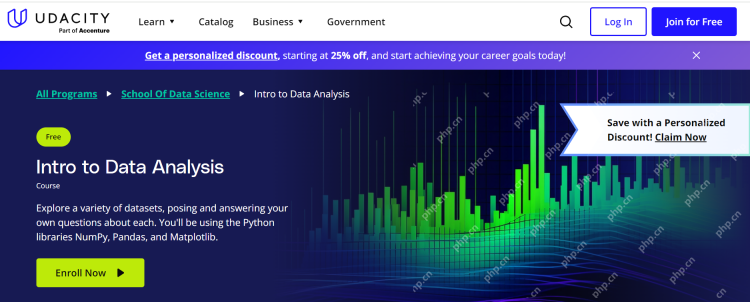
踏上數據驅動的職業旅程而不會破壞銀行! 本文重點介紹了五個非凡的免費數據分析課程,非常適合兩位經驗豐富的專業人士,他們尋求擴大技能和好奇的新手渴望探索T
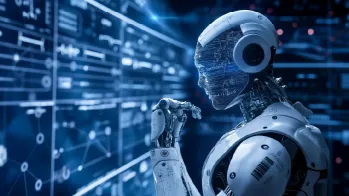
利用AI代理商的力量與OpenAgi:綜合指南 想像一下不懈的助手,總是可以簡化您的任務並提供有見地的建議。這就是AI代理商的承諾,Openagi賦予您建造它們
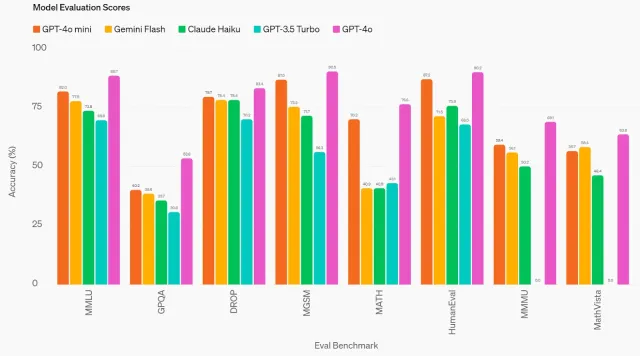
Openai的最新產品GPT-4O Mini標誌著朝著負擔得起且可訪問的高級AI邁出的重要一步。 這種小型語言模型(SLM)直接挑戰諸如Llama 3和Gemma 2之類的競爭對手,具有低潛伏期,成本效益和A
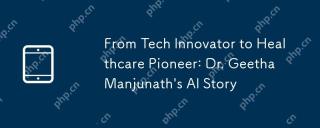
Niramai Analytix的創始人兼首席執行官Geetha Manjunath博士的這一集由“領導數據”的劇集。 Manjunath博士擁有AI和Healthcare的25年以上的經驗,並獲得了印度科學學院的博士學位和MBA來回。
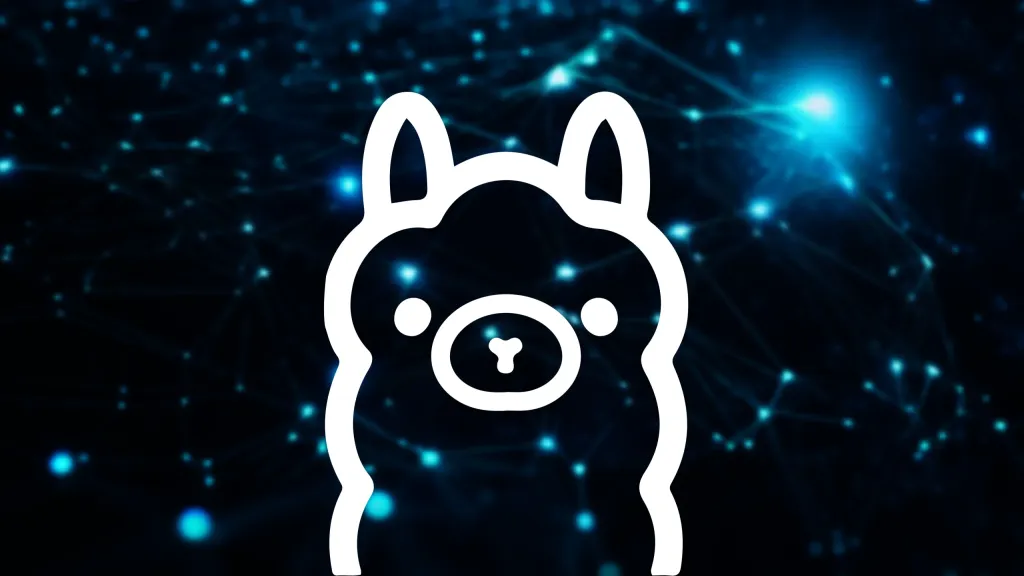
利用Ollama本地開源LLMS的力量:綜合指南 運行大型語言模型(LLMS)本地提供無與倫比的控制和透明度,但是設置環境可能令人生畏。 Ollama簡化了這個過程
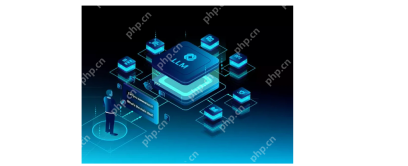
利用微調LLM的功能與Monsterapi:綜合指南 想像一個虛擬助手完美理解並預測您的需求。 由於大型語言模型(LLMS)的進步,這已成為現實。 但是,
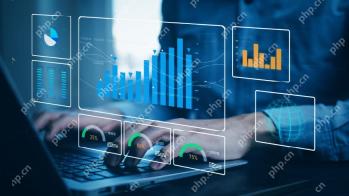
數據科學的基本統計測試:綜合指南 從數據中解鎖有價值的見解至關重要。 掌握統計測試對於實現這一目標至關重要。這些測試使數據科學家能夠嚴格瓦爾


熱AI工具

Undresser.AI Undress
人工智慧驅動的應用程序,用於創建逼真的裸體照片

AI Clothes Remover
用於從照片中去除衣服的線上人工智慧工具。

Undress AI Tool
免費脫衣圖片

Clothoff.io
AI脫衣器

AI Hentai Generator
免費產生 AI 無盡。

熱門文章

熱工具

MinGW - Minimalist GNU for Windows
這個專案正在遷移到osdn.net/projects/mingw的過程中,你可以繼續在那裡關注我們。 MinGW:GNU編譯器集合(GCC)的本機Windows移植版本,可自由分發的導入函式庫和用於建置本機Windows應用程式的頭檔;包括對MSVC執行時間的擴展,以支援C99功能。 MinGW的所有軟體都可以在64位元Windows平台上運作。

SublimeText3漢化版
中文版,非常好用
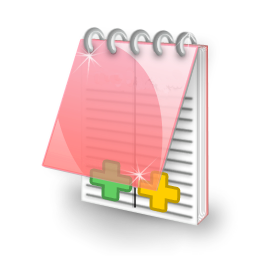
EditPlus 中文破解版
體積小,語法高亮,不支援程式碼提示功能

Atom編輯器mac版下載
最受歡迎的的開源編輯器

禪工作室 13.0.1
強大的PHP整合開發環境