


Performance Measurement of Python Natural Language Processing: Assessing Model Accuracy and Efficiency
python Performance measurements of Natural Language Processing (NLP) models in
are useful for evaluating the effectiveness and Efficiency is crucial. The following are the main metrics used to evaluate the accuracy and efficiency of NLP models:
- Accuracy index:
- Precision: Measures the proportion of samples predicted as positive by the model that are actually positive.
- Recall (Recall): Measures the proportion of all actual positive samples predicted by the model that are predicted to be positive by the model.
- F1 score: The weighted average of precision and recall, providing a measure of the overall accuracy of the model.
- Accuracy: Measures the proportion of correct predictions among all samples predicted by the model.
Shows the actual and predicted values predicted by the model and is used to identify false positives and false negatives.
- Efficiency indicators:
- Training time: The time required to train the model.
- Prediction time: The time required to predict new data.
- Memory usage: The amount of memory required to train and predict the model. Complexity: Measures the computational complexity of the model
.
assessment method:
Performance evaluation of NLP models often involves the use of cross-validation to ensure the reliability of the results. Cross-validation divides the data set into multiple subsets, each subset in turn is used as atest set, while the remaining data is used as a training set. The model is trained and evaluated on each subset, and then the average performance metric is calculated across all subsets.
Optimize performance:
In order to- optimize the performance of the
- NLP model, the following aspects can be adjusted: Hyperparameters: Parameters of the model training algorithm, such as
- learning rate and regularization terms.
- Feature Engineering: Preprocess data to improve model performance.
- Model Architecture: Select the model type and configuration appropriate for the specific task.
Use techniques to increase the amount and diversity of training data.
Tools and Libraries: Python
There are many- tools
- and libraries available for performance measurement of NLP models, including: scikit-learn: A
- machine learning library that provides evaluation metrics and cross-validation functions. TensorFlow: A framework for training and evaluating
- deep learning models. Keras: Advanced Neural Networks api based on
- Tensorflow.
Provides pre-trained NLP models and metrics for their evaluation.
Factors affecting performance:
### ###Factors that affect NLP model performance include: ###- Data quality: The quality and size of the training and test data sets.
- Complexity of the model: The size and depth of the model architecture .
- Computing resources: Computing power used to train and predict models.
- Task type: The type and difficulty of the NLP task.
Best Practices:
Best practices when evaluating NLP models include:
- Use multiple accuracy metrics: Don’t rely on just one accuracy metric to evaluate your model’s performance.
- Consider efficiency indicators: Balance the accuracy and efficiency of the model.
- Report cross-validation results: Cross-validation results are provided to demonstrate the reliability of the performance.
- Compare model performance to baselines: Compare a model's performance to existing baselines to evaluate its effectiveness relative to other models.
The above is the detailed content of Performance Measurement of Python Natural Language Processing: Assessing Model Accuracy and Efficiency. For more information, please follow other related articles on the PHP Chinese website!
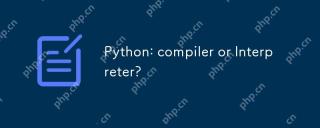
Python is an interpreted language, but it also includes the compilation process. 1) Python code is first compiled into bytecode. 2) Bytecode is interpreted and executed by Python virtual machine. 3) This hybrid mechanism makes Python both flexible and efficient, but not as fast as a fully compiled language.
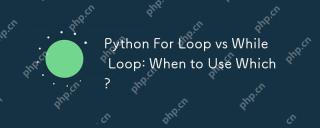
Useaforloopwheniteratingoverasequenceorforaspecificnumberoftimes;useawhileloopwhencontinuinguntilaconditionismet.Forloopsareidealforknownsequences,whilewhileloopssuitsituationswithundeterminediterations.
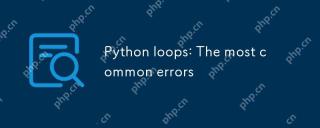
Pythonloopscanleadtoerrorslikeinfiniteloops,modifyinglistsduringiteration,off-by-oneerrors,zero-indexingissues,andnestedloopinefficiencies.Toavoidthese:1)Use'i
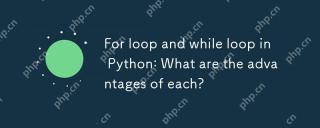
Forloopsareadvantageousforknowniterationsandsequences,offeringsimplicityandreadability;whileloopsareidealfordynamicconditionsandunknowniterations,providingcontrolovertermination.1)Forloopsareperfectforiteratingoverlists,tuples,orstrings,directlyacces
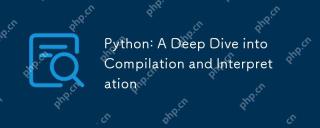
Pythonusesahybridmodelofcompilationandinterpretation:1)ThePythoninterpretercompilessourcecodeintoplatform-independentbytecode.2)ThePythonVirtualMachine(PVM)thenexecutesthisbytecode,balancingeaseofusewithperformance.
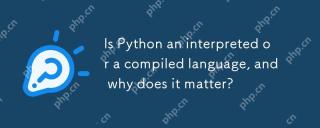
Pythonisbothinterpretedandcompiled.1)It'scompiledtobytecodeforportabilityacrossplatforms.2)Thebytecodeistheninterpreted,allowingfordynamictypingandrapiddevelopment,thoughitmaybeslowerthanfullycompiledlanguages.
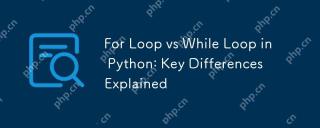
Forloopsareidealwhenyouknowthenumberofiterationsinadvance,whilewhileloopsarebetterforsituationswhereyouneedtoloopuntilaconditionismet.Forloopsaremoreefficientandreadable,suitableforiteratingoversequences,whereaswhileloopsoffermorecontrolandareusefulf
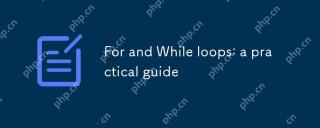
Forloopsareusedwhenthenumberofiterationsisknowninadvance,whilewhileloopsareusedwhentheiterationsdependonacondition.1)Forloopsareidealforiteratingoversequenceslikelistsorarrays.2)Whileloopsaresuitableforscenarioswheretheloopcontinuesuntilaspecificcond


Hot AI Tools

Undresser.AI Undress
AI-powered app for creating realistic nude photos

AI Clothes Remover
Online AI tool for removing clothes from photos.

Undress AI Tool
Undress images for free

Clothoff.io
AI clothes remover

Video Face Swap
Swap faces in any video effortlessly with our completely free AI face swap tool!

Hot Article

Hot Tools

PhpStorm Mac version
The latest (2018.2.1) professional PHP integrated development tool

DVWA
Damn Vulnerable Web App (DVWA) is a PHP/MySQL web application that is very vulnerable. Its main goals are to be an aid for security professionals to test their skills and tools in a legal environment, to help web developers better understand the process of securing web applications, and to help teachers/students teach/learn in a classroom environment Web application security. The goal of DVWA is to practice some of the most common web vulnerabilities through a simple and straightforward interface, with varying degrees of difficulty. Please note that this software

SublimeText3 Chinese version
Chinese version, very easy to use

SecLists
SecLists is the ultimate security tester's companion. It is a collection of various types of lists that are frequently used during security assessments, all in one place. SecLists helps make security testing more efficient and productive by conveniently providing all the lists a security tester might need. List types include usernames, passwords, URLs, fuzzing payloads, sensitive data patterns, web shells, and more. The tester can simply pull this repository onto a new test machine and he will have access to every type of list he needs.
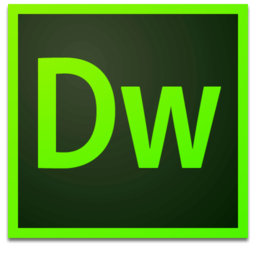
Dreamweaver Mac version
Visual web development tools
