


python As a powerful programming language, it provides a rich for data visualization Tool box. These tools enable data scientists and analysts to transform complex data into intuitive and understandable visualizations that reveal patterns, trends and insights.
1. Matplotlib: basic and flexibleMatplotlib is one of the most popular
Python visualization libraries. It provides a range of plotting functions, including line graphs, bar graphs, scatter plots, and histograms. It allows for a high degree of customization, allowing you to create professional-grade visualizations.
import matplotlib.pyplot as plt
plt.plot(x, y)
plt.xlabel("x-axis")
plt.ylabel("y-axis")
plt.title("My Plot")
plt.show()
Seaborn is built on Matplotlib and provides a more advanced interface that focuses more on statistical data visualization. It offers pre-made themes and color schemes that simplify creating beautiful and informative visualizations.
import seaborn as sns sns.scatterplot(x, y) sns.set_theme() plt.show()3. Pandas Profiling: Quick Insights
pandas
Profiling is an automateddata analysis and exploration tool. It generates an interactive html report with detailed statistics and visualizations about the columns and rows in the dataframe, which helps quickly identify patterns and outliers.
4. Plotly: interactive and dynamicPlotly is a popular interactive visualization library. It allows the creation of 2D and
3D interactive charts that can be viewed in a web browser. Plotly is especially useful for exploring complex data sets.
import plotly.express as px
fig = px.scatter_3d(df, x="x", y="y", z="z")
fig.show()
Bokeh is a visualization library focusing on
performance optimization. It uses just-in-time compiler technology to generate visualizations on the client side, enabling high frame rates and fast response times for interactive visualizations.
from bokeh.models import ColumnDataSource
from bokeh.plotting import figure, output_notebook
output_notebook()
source = ColumnDataSource(data=df)
p = figure(x_axis_label="x", y_axis_label="y")
p.circle(source=source, x="x", y="y")
When choosing a Python visualization tool, it is important to consider the type of data, the level of interaction required, and the complexity of the visualization. By leveraging the rich toolbox provided by Python, you can unleash the power of data visualization to gain clear insights and effectively communicate your findings.
The above is the detailed content of Python's Visualization Toolbox: Exploring Unlimited Possibilities of Data. For more information, please follow other related articles on the PHP Chinese website!
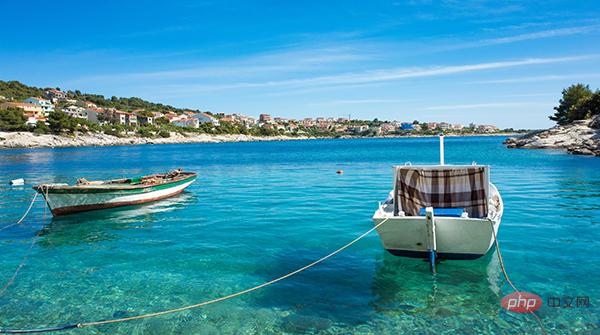
大家好,我是啃书君!正所谓:有朋自远方来,不亦乐乎?有朋友来找我们玩,是一件很快乐的事情,那么我们要尽地主之谊,好好带朋友去玩耍!那么问题来了,什么时候去哪里玩最好呢,哪里玩的地方最多呢?今天将手把手教你使用线程池爬取同程旅行的景点信息及评论数据并做词云、数据可视化!!!带你了解各个城市的游玩景点信息。在开始爬取数据之前,我们首先来了解一下线程。线程进程:进程是代码在数据集合上的一次运行活动,是系统进行资源分配和调度的基本单位。线程:是轻量级的进程,是程序执行的最小单元,是进程的一个执行路径。一
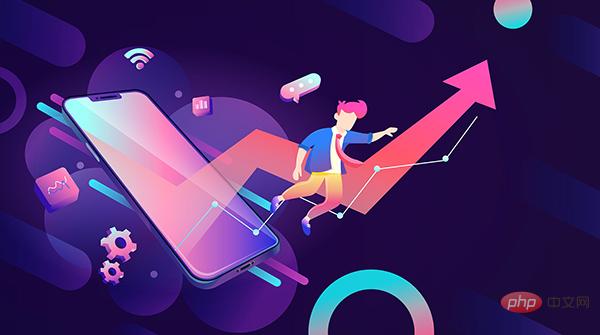
1、TransBigData简介TransBigData为处理常见的交通时空大数据(如出租车GPS数据、共享单车数据和公交车GPS数据等)提供了快速而简洁的方法。TransBigData为交通时空大数据分析的各个阶段提供了多种处理方法,代码简洁、高效、灵活、易用,可以用简洁的代码实现复杂的数据任务。目前,TransBigData主要提供以下方法: 数据预处理:对数据集提供快速计算数据量、时间段、采样间隔等基本信息的方法,也针对多种数据噪声提供了相应的清洗方法。 数据栅格化:提供在研究区域内生成、
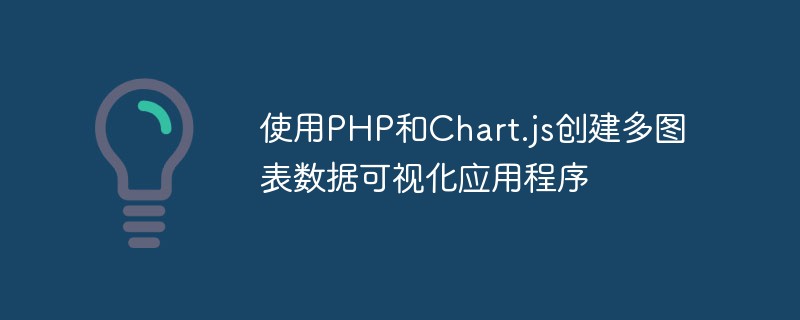
随着互联网的不断发展,大量的数据被生成并存储在各种网络应用程序和系统中,尤其是像电子商务、社交网络、金融和制造业等领域。为了从这些数据中汲取更多的信息,数据可视化已成为一种流行的方法。通过将数据转换为图形形式,用户可以更加容易地理解和分析数据。在本文中,我们将介绍如何使用PHP和Chart.js来创建多图表数据可视化应用程序。什么是Chart.js?Char
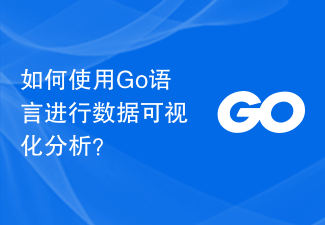
随着大数据时代的到来,数据可视化分析在各行各业中扮演着至关重要的角色。而Go语言作为一种快速、高效、安全的编程语言,也逐渐在数据可视化分析领域占据一席之地。本文将探讨如何使用Go语言进行数据可视化分析。一、Go语言常用的数据可视化库Plotly:可用于在浏览器中创建交互式的图形,支持多种图形类型,如线图、条形图、散点图、热力图等。Gonum/plo
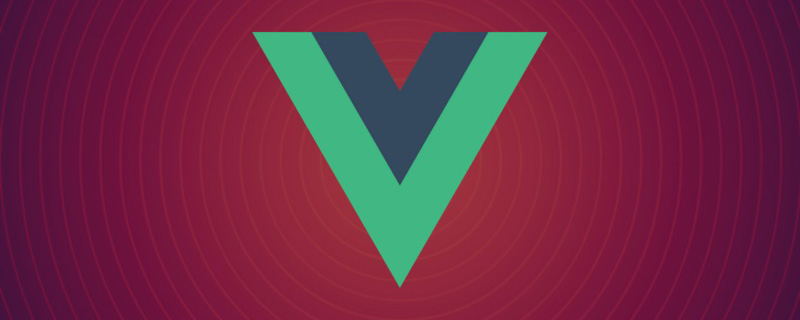
在我们使用高德地图的时候,官方给我们推荐了很多案例,demo,但是这些案例都是使用原生方法接入,并没有提供vue或者react 的demo,vue2的 接入网上也很多人都有写过,下面本篇文章就来看看 vue3怎么使用常用的高德地图api,希望对大家有所帮助!
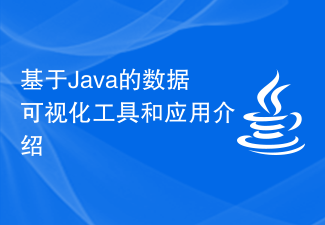
近年来,随着数据的爆炸性增长和互联网技术的不断发展,数据可视化成为越来越受关注的领域。数据可视化是将数据转化为易于理解和分析的图形化形式,帮助人们更快速和准确地理解数据。而基于Java的数据可视化工具和应用则成为了当前比较热门的技术。Java的数据可视化工具和应用优势应用范围广Java语言有很强的跨平台性,可以在不同操作系统上安装和运行,而且可以通过Java
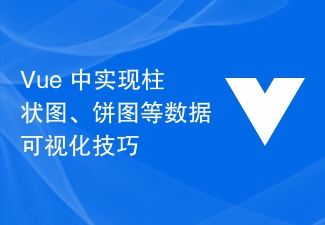
近年来,数据可视化相关技术的快速发展,使得复杂数据更易于被理解和分析。Vue作为一种流行的前端框架,具有良好的可扩展性和易用性,被广泛应用于数据可视化领域。本文将介绍Vue中实现柱状图、饼图等数据可视化的技巧。一、使用ECharts实现柱状图ECharts是一款基于JavaScript的开源可视化库,提供了多种图表类型,其中包括柱状图。下面以
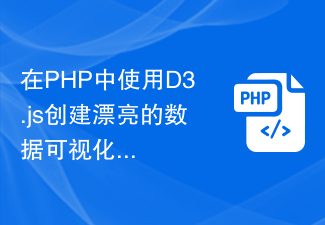
随着互联网时代的到来,数据已经成为了我们生活和工作中必不可少的一部分。在处理和分析数据的过程中,数据可视化已经成为了一种非常流行的技术。数据可视化可以让我们更好地理解数据,发现数据中的规律和趋势,同时更好地向别人展示数据分析结果。在本文中,我们将会介绍如何在PHP中使用D3.js创建漂亮的数据可视化。一、什么是D3.jsD3.js(Data-DrivenD


Hot AI Tools

Undresser.AI Undress
AI-powered app for creating realistic nude photos

AI Clothes Remover
Online AI tool for removing clothes from photos.

Undress AI Tool
Undress images for free

Clothoff.io
AI clothes remover

AI Hentai Generator
Generate AI Hentai for free.

Hot Article

Hot Tools

SublimeText3 English version
Recommended: Win version, supports code prompts!

mPDF
mPDF is a PHP library that can generate PDF files from UTF-8 encoded HTML. The original author, Ian Back, wrote mPDF to output PDF files "on the fly" from his website and handle different languages. It is slower than original scripts like HTML2FPDF and produces larger files when using Unicode fonts, but supports CSS styles etc. and has a lot of enhancements. Supports almost all languages, including RTL (Arabic and Hebrew) and CJK (Chinese, Japanese and Korean). Supports nested block-level elements (such as P, DIV),

SAP NetWeaver Server Adapter for Eclipse
Integrate Eclipse with SAP NetWeaver application server.
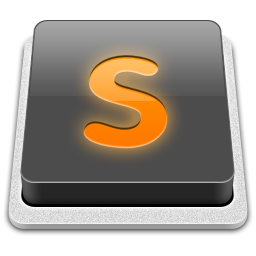
SublimeText3 Mac version
God-level code editing software (SublimeText3)

MantisBT
Mantis is an easy-to-deploy web-based defect tracking tool designed to aid in product defect tracking. It requires PHP, MySQL and a web server. Check out our demo and hosting services.