How are arrays used in image processing with Python?
Arrays are crucial in Python image processing as they enable efficient manipulation and analysis of image data. 1) Images are converted to NumPy arrays, with grayscale images as 2D arrays and color images as 3D arrays. 2) Arrays allow for vectorized operations, enabling fast adjustments like brightness changes. 3) They are essential for complex tasks like filtering and edge detection using convolution. 4) Challenges include handling different image formats and managing memory for large images. 5) Performance can be optimized using NumPy's operations, parallel processing, or GPU acceleration. 6) Best practices involve validating input data and writing modular code for flexibility.
Arrays are fundamental in image processing with Python, serving as the backbone for manipulating and analyzing image data. When you dive into image processing, you'll find that images are essentially arrays of pixel values. In Python, libraries like NumPy and PIL (Python Imaging Library) leverage arrays to handle these pixels efficiently. Let's explore how arrays are used in this fascinating field.
When you open an image in Python, it's typically converted into a NumPy array. Each pixel in the image corresponds to an element in this array. For grayscale images, you're dealing with a 2D array where each element represents the intensity of the pixel. For color images, you'll encounter a 3D array, with each pixel broken down into its RGB components.
Here's a quick example to illustrate:
from PIL import Image import numpy as np # Open an image file image = Image.open('example.jpg') # Convert image to NumPy array image_array = np.array(image) print(image_array.shape) # Output: (height, width, 3) for RGB images
Now, why are arrays so crucial? They allow for vectorized operations, which means you can perform operations on entire images or regions at once, rather than looping through each pixel. This is where the real magic happens in image processing.
For instance, if you want to adjust the brightness of an image, you can simply add a value to the entire array:
# Increase brightness by 50 brighter_image = image_array 50 # Clip values to ensure they stay within 0-255 range brighter_image = np.clip(brighter_image, 0, 255).astype(np.uint8) # Convert back to image result = Image.fromarray(brighter_image) result.save('brighter_example.jpg')
This approach is not only elegant but also incredibly fast, thanks to NumPy's optimized operations.
Arrays also play a key role in more complex image processing tasks like filtering, edge detection, and image segmentation. For example, to apply a blur filter, you can use convolution, which is essentially multiplying the image array with a kernel array:
from scipy import ndimage # Define a 5x5 Gaussian blur kernel kernel = np.array([ [1, 4, 6, 4, 1], [4, 16, 24, 16, 4], [6, 24, 36, 24, 6], [4, 16, 24, 16, 4], [1, 4, 6, 4, 1] ]) / 256 # Apply the blur filter blurred_image = ndimage.convolve(image_array, kernel) # Convert back to image blurred_result = Image.fromarray(blurred_image.astype(np.uint8)) blurred_result.save('blurred_example.jpg')
When working with arrays in image processing, you'll encounter a few challenges. One common pitfall is dealing with different image formats and color spaces. For example, some images might be in CMYK rather than RGB, which requires converting the array before processing. Another challenge is memory management, especially with large images or when processing multiple images in a loop. You might need to consider using memory-mapped arrays or processing images in chunks.
In terms of performance optimization, leveraging NumPy's vectorized operations is key. However, for very large images or intensive operations, you might want to explore parallel processing with libraries like Dask or even GPU acceleration with CUDA or OpenCL.
From my experience, one of the best practices in image processing with arrays is to always validate your input data. Ensure that the image array has the expected shape and data type before processing. Also, consider writing modular code where you can easily swap out different filters or operations without rewriting your entire pipeline.
To wrap up, arrays are the lifeblood of image processing in Python. They enable efficient, vectorized operations that make complex image manipulations possible. Whether you're adjusting brightness, applying filters, or performing advanced segmentation, understanding how to work with arrays will unlock a world of possibilities in image processing.
The above is the detailed content of How are arrays used in image processing with Python?. For more information, please follow other related articles on the PHP Chinese website!
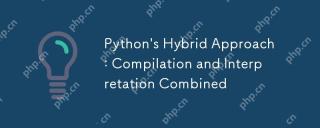
Pythonusesahybridapproach,combiningcompilationtobytecodeandinterpretation.1)Codeiscompiledtoplatform-independentbytecode.2)BytecodeisinterpretedbythePythonVirtualMachine,enhancingefficiencyandportability.
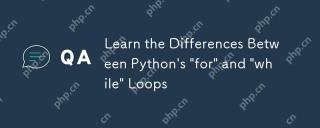
ThekeydifferencesbetweenPython's"for"and"while"loopsare:1)"For"loopsareidealforiteratingoversequencesorknowniterations,while2)"while"loopsarebetterforcontinuinguntilaconditionismetwithoutpredefinediterations.Un
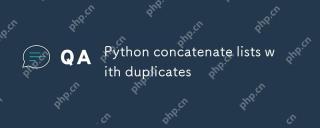
In Python, you can connect lists and manage duplicate elements through a variety of methods: 1) Use operators or extend() to retain all duplicate elements; 2) Convert to sets and then return to lists to remove all duplicate elements, but the original order will be lost; 3) Use loops or list comprehensions to combine sets to remove duplicate elements and maintain the original order.
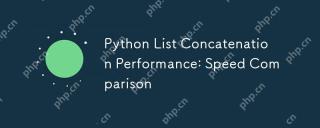
ThefastestmethodforlistconcatenationinPythondependsonlistsize:1)Forsmalllists,the operatorisefficient.2)Forlargerlists,list.extend()orlistcomprehensionisfaster,withextend()beingmorememory-efficientbymodifyinglistsin-place.
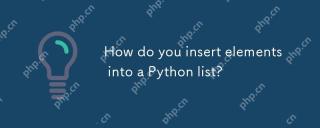
ToinsertelementsintoaPythonlist,useappend()toaddtotheend,insert()foraspecificposition,andextend()formultipleelements.1)Useappend()foraddingsingleitemstotheend.2)Useinsert()toaddataspecificindex,thoughit'sslowerforlargelists.3)Useextend()toaddmultiple
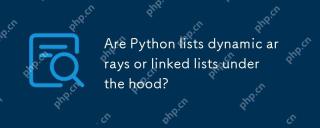
Pythonlistsareimplementedasdynamicarrays,notlinkedlists.1)Theyarestoredincontiguousmemoryblocks,whichmayrequirereallocationwhenappendingitems,impactingperformance.2)Linkedlistswouldofferefficientinsertions/deletionsbutslowerindexedaccess,leadingPytho
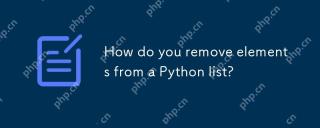
Pythonoffersfourmainmethodstoremoveelementsfromalist:1)remove(value)removesthefirstoccurrenceofavalue,2)pop(index)removesandreturnsanelementataspecifiedindex,3)delstatementremoveselementsbyindexorslice,and4)clear()removesallitemsfromthelist.Eachmetho
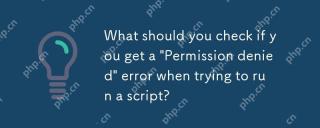
Toresolvea"Permissiondenied"errorwhenrunningascript,followthesesteps:1)Checkandadjustthescript'spermissionsusingchmod xmyscript.shtomakeitexecutable.2)Ensurethescriptislocatedinadirectorywhereyouhavewritepermissions,suchasyourhomedirectory.


Hot AI Tools

Undresser.AI Undress
AI-powered app for creating realistic nude photos

AI Clothes Remover
Online AI tool for removing clothes from photos.

Undress AI Tool
Undress images for free

Clothoff.io
AI clothes remover

Video Face Swap
Swap faces in any video effortlessly with our completely free AI face swap tool!

Hot Article

Hot Tools

MinGW - Minimalist GNU for Windows
This project is in the process of being migrated to osdn.net/projects/mingw, you can continue to follow us there. MinGW: A native Windows port of the GNU Compiler Collection (GCC), freely distributable import libraries and header files for building native Windows applications; includes extensions to the MSVC runtime to support C99 functionality. All MinGW software can run on 64-bit Windows platforms.

Zend Studio 13.0.1
Powerful PHP integrated development environment

Safe Exam Browser
Safe Exam Browser is a secure browser environment for taking online exams securely. This software turns any computer into a secure workstation. It controls access to any utility and prevents students from using unauthorized resources.

Notepad++7.3.1
Easy-to-use and free code editor
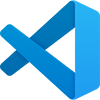
VSCode Windows 64-bit Download
A free and powerful IDE editor launched by Microsoft
