


For what types of operations are arrays significantly faster than lists?
Arrays are significantly faster than lists for operations benefiting from direct memory access and fixed-size structures. 1) Accessing elements: Arrays provide constant-time access due to contiguous memory storage. 2) Iteration: Arrays leverage cache locality for faster iteration. 3) Memory allocation: Arrays allocate memory once, avoiding costly resizing. 4) Numerical computations: Arrays are optimized for large dataset operations, as seen in libraries like NumPy.
Arrays are significantly faster than lists for certain types of operations due to their fixed-size nature and direct memory access. Let's dive into the details and explore when arrays outperform lists, why that happens, and how we can leverage this knowledge in our programming.
When I first started coding, I was fascinated by the simplicity and efficiency of arrays. I remember working on a project where speed was critical, and using arrays instead of lists made a noticeable difference. Let's unpack this and see why arrays can be your secret weapon in certain scenarios.
Arrays shine in operations that involve direct memory access and fixed-size data structures. Here's how they outperform lists:
Accessing Elements: Arrays allow for constant-time access to elements using an index. This is because arrays store elements in contiguous memory locations, so accessing an element is simply a matter of calculating the memory address. In contrast, lists often use a more complex structure, like a linked list or a dynamic array, which can lead to slower access times, especially for large datasets.
Iteration: When iterating over an array, the CPU can take advantage of cache locality, which means that once a portion of the array is loaded into the cache, subsequent elements are quickly accessible. This leads to faster iteration compared to lists, which might not have the same level of cache efficiency due to their dynamic nature.
Memory Allocation: Arrays have a fixed size, so memory allocation happens only once when the array is created. Lists, on the other hand, may need to resize themselves, which involves copying elements to a new memory location. This resizing can be costly, especially if it happens frequently.
Numerical Computations: In scenarios where you're performing numerical computations on large datasets, arrays are often the better choice. Libraries like NumPy in Python are built around arrays for this reason, providing optimized operations that take advantage of the array's structure.
Let's look at some code to illustrate these points. Here's an example in C that demonstrates the difference in accessing elements between an array and a vector (which is similar to a list in other languages):
#include <iostream> #include <vector> #include <chrono> int main() { const int size = 1000000; int arr[size]; std::vector<int> vec(size); // Initialize array and vector for (int i = 0; i < size; i) { arr[i] = i; vec[i] = i; } // Measure time for array access auto start = std::chrono::high_resolution_clock::now(); int sumArr = 0; for (int i = 0; i < size; i) { sumArr = arr[i]; } auto end = std::chrono::high_resolution_clock::now(); auto durationArr = std::chrono::duration_cast<std::chrono::microseconds>(end - start); // Measure time for vector access start = std::chrono::high_resolution_clock::now(); int sumVec = 0; for (int i = 0; i < size; i) { sumVec = vec[i]; } end = std::chrono::high_resolution_clock::now(); auto durationVec = std::chrono::duration_cast<std::chrono::microseconds>(end - start); std::cout << "Array access time: " << durationArr.count() << " microseconds" << std::endl; std::cout << "Vector access time: " << durationVec.count() << " microseconds" << std::endl; return 0; }
This code measures the time taken to sum up all elements in an array and a vector. You'll likely find that the array access is faster due to its direct memory access and cache efficiency.
Now, let's talk about some of the trade-offs and potential pitfalls:
Fixed Size: Arrays have a fixed size, which can be a limitation. If you need to add or remove elements frequently, a list might be more suitable despite its slower access times.
Memory Management: While arrays are faster for certain operations, they require manual memory management in languages like C or C . This can lead to memory leaks or buffer overflows if not handled correctly.
Flexibility: Lists offer more flexibility in terms of operations like insertion and deletion at arbitrary positions. Arrays are less flexible in this regard, which can be a disadvantage in certain scenarios.
In my experience, choosing between arrays and lists often comes down to understanding the specific requirements of your project. If you're working on a performance-critical application where you know the size of your data in advance and need fast access, arrays are a great choice. However, if your data is dynamic and you need to frequently modify the structure, lists might be more appropriate despite their slower access times.
To wrap up, arrays are significantly faster than lists for operations that benefit from direct memory access and fixed-size structures. By understanding these differences, you can make informed decisions in your coding projects, optimizing for both performance and flexibility as needed. Remember, the best tool is the one that fits your specific use case, and sometimes, that tool is an array.
The above is the detailed content of For what types of operations are arrays significantly faster than lists?. For more information, please follow other related articles on the PHP Chinese website!
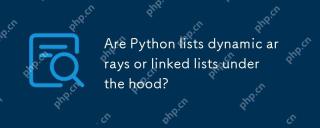
Pythonlistsareimplementedasdynamicarrays,notlinkedlists.1)Theyarestoredincontiguousmemoryblocks,whichmayrequirereallocationwhenappendingitems,impactingperformance.2)Linkedlistswouldofferefficientinsertions/deletionsbutslowerindexedaccess,leadingPytho
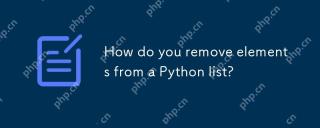
Pythonoffersfourmainmethodstoremoveelementsfromalist:1)remove(value)removesthefirstoccurrenceofavalue,2)pop(index)removesandreturnsanelementataspecifiedindex,3)delstatementremoveselementsbyindexorslice,and4)clear()removesallitemsfromthelist.Eachmetho
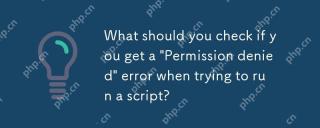
Toresolvea"Permissiondenied"errorwhenrunningascript,followthesesteps:1)Checkandadjustthescript'spermissionsusingchmod xmyscript.shtomakeitexecutable.2)Ensurethescriptislocatedinadirectorywhereyouhavewritepermissions,suchasyourhomedirectory.
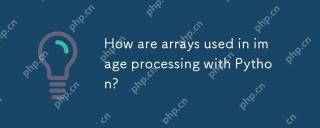
ArraysarecrucialinPythonimageprocessingastheyenableefficientmanipulationandanalysisofimagedata.1)ImagesareconvertedtoNumPyarrays,withgrayscaleimagesas2Darraysandcolorimagesas3Darrays.2)Arraysallowforvectorizedoperations,enablingfastadjustmentslikebri
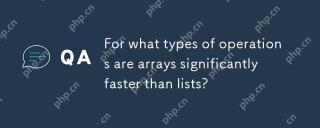
Arraysaresignificantlyfasterthanlistsforoperationsbenefitingfromdirectmemoryaccessandfixed-sizestructures.1)Accessingelements:Arraysprovideconstant-timeaccessduetocontiguousmemorystorage.2)Iteration:Arraysleveragecachelocalityforfasteriteration.3)Mem
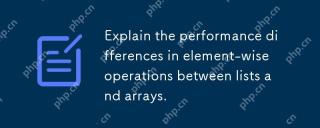
Arraysarebetterforelement-wiseoperationsduetofasteraccessandoptimizedimplementations.1)Arrayshavecontiguousmemoryfordirectaccess,enhancingperformance.2)Listsareflexiblebutslowerduetopotentialdynamicresizing.3)Forlargedatasets,arrays,especiallywithlib
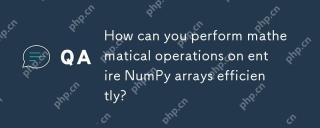
Mathematical operations of the entire array in NumPy can be efficiently implemented through vectorized operations. 1) Use simple operators such as addition (arr 2) to perform operations on arrays. 2) NumPy uses the underlying C language library, which improves the computing speed. 3) You can perform complex operations such as multiplication, division, and exponents. 4) Pay attention to broadcast operations to ensure that the array shape is compatible. 5) Using NumPy functions such as np.sum() can significantly improve performance.
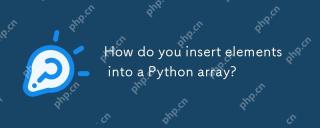
In Python, there are two main methods for inserting elements into a list: 1) Using the insert(index, value) method, you can insert elements at the specified index, but inserting at the beginning of a large list is inefficient; 2) Using the append(value) method, add elements at the end of the list, which is highly efficient. For large lists, it is recommended to use append() or consider using deque or NumPy arrays to optimize performance.


Hot AI Tools

Undresser.AI Undress
AI-powered app for creating realistic nude photos

AI Clothes Remover
Online AI tool for removing clothes from photos.

Undress AI Tool
Undress images for free

Clothoff.io
AI clothes remover

Video Face Swap
Swap faces in any video effortlessly with our completely free AI face swap tool!

Hot Article

Hot Tools

SublimeText3 English version
Recommended: Win version, supports code prompts!
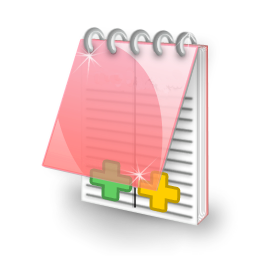
EditPlus Chinese cracked version
Small size, syntax highlighting, does not support code prompt function

Safe Exam Browser
Safe Exam Browser is a secure browser environment for taking online exams securely. This software turns any computer into a secure workstation. It controls access to any utility and prevents students from using unauthorized resources.

MantisBT
Mantis is an easy-to-deploy web-based defect tracking tool designed to aid in product defect tracking. It requires PHP, MySQL and a web server. Check out our demo and hosting services.

Zend Studio 13.0.1
Powerful PHP integrated development environment
