


My neural network is trained (from scratch) to get further away from the goal
This is my first time creating a neural network and I decided to create it in golang which is usually not the language used for this purpose , but I want to get a good understanding of how they work from scratch with only the basic libraries.
The goal of this program is to train a neural network to be able to add two numbers (1-10). To do this, I created a neural network class called rawai (the best name I could think of) and gave it a 1 input layer (array of size 2), 1 hidden layer (array of size 2 array) and 1 output layer (array of size 1).
The weight has two 2d arrays, one is ih (hidden input) [2,2], and the other is ho, [2,1].
The following is the code to start ai, train and test ai. You will see several debugging statements that I have used and any other functions that are not golang or its packages will be shown in the following code of my rawai class. This is called by my main function:
func additionneuralnetworktest() { nn := newrawai(2, 2, 1, 1/math.pow(10, 15)) fmt.printf("weights ih before: %v\n\nweights ho after: %v\n", nn.weightsih, nn.weightsho) //train neural network // for epoch := 0; epoch < 10000000; epoch++ { for i := 0; i <= 10; i++ { for j := 0; j <= 10; j++ { inputs := make([]float64, 2) targets := make([]float64, 1) inputs[0] = float64(i) inputs[1] = float64(j) targets[0] = float64(i) + float64(j) nn.train(inputs, targets) if epoch%20000 == 0 && i == 5 && j == 5 { fmt.printf("[training] [epoch %d] %f + %f = %f targets[%f]\n", epoch, inputs[0], inputs[1], nn.outputlayer[0], targets[0]) } } } } // test neural network a := rand.intn(10) + 1 b := rand.intn(10) + 1 inputs := make([]float64, 2) inputs[0] = float64(a) inputs[1] = float64(b) prediction := nn.feedforward(inputs)[0] fmt.printf("%d + %d = %f\n", a, b, prediction) fmt.printf("weights ih: %v\n\nweights ho: %v\n", nn.weightsih, nn.weightsho) }
The following is all the code in the rawai file:
type RawAI struct { InputLayer []float64 `json:"input_layer"` HiddenLayer []float64 `json:"hidden_layer"` OutputLayer []float64 `json:"output_layer"` WeightsIH [][]float64 `json:"weights_ih"` WeightsHO [][]float64 `json:"weights_ho"` LearningRate float64 `json:"learning_rate"` } func NewRawAI(inputSize, hiddenSize, outputSize int, learningRate float64) *RawAI { nn := RawAI{ InputLayer: make([]float64, inputSize), HiddenLayer: make([]float64, hiddenSize), OutputLayer: make([]float64, outputSize), WeightsIH: randomMatrix(inputSize, hiddenSize), WeightsHO: randomMatrix(hiddenSize, outputSize), LearningRate: learningRate, } return &nn } func (nn *RawAI) FeedForward(inputs []float64) []float64 { // Set input layer for i := 0; i < len(inputs); i++ { nn.InputLayer[i] = inputs[i] } // Compute hidden layer for i := 0; i < len(nn.HiddenLayer); i++ { sum := 0.0 for j := 0; j < len(nn.InputLayer); j++ { sum += nn.InputLayer[j] * nn.WeightsIH[j][i] } nn.HiddenLayer[i] = sum if math.IsNaN(sum) { panic(fmt.Sprintf("Sum is NaN on Hidden Layer:\nInput Layer: %v\nHidden Layer: %v\nWeights IH: %v\n", nn.InputLayer, nn.HiddenLayer, nn.WeightsIH)) } } // Compute output layer for k := 0; k < len(nn.OutputLayer); k++ { sum := 0.0 for j := 0; j < len(nn.HiddenLayer); j++ { sum += nn.HiddenLayer[j] * nn.WeightsHO[j][k] } nn.OutputLayer[k] = sum if math.IsNaN(sum) { panic(fmt.Sprintf("Sum is NaN on Output Layer:\n Model: %v\n", nn)) } } return nn.OutputLayer } func (nn *RawAI) Train(inputs []float64, targets []float64) { nn.FeedForward(inputs) // Compute output layer error outputErrors := make([]float64, len(targets)) for k := 0; k < len(targets); k++ { outputErrors[k] = targets[k] - nn.OutputLayer[k] } // Compute hidden layer error hiddenErrors := make([]float64, len(nn.HiddenLayer)) for j := 0; j < len(nn.HiddenLayer); j++ { errorSum := 0.0 for k := 0; k < len(nn.OutputLayer); k++ { errorSum += outputErrors[k] * nn.WeightsHO[j][k] } hiddenErrors[j] = errorSum * sigmoidDerivative(nn.HiddenLayer[j]) if math.IsInf(math.Abs(hiddenErrors[j]), 1) { //Find out why fmt.Printf("Hidden Error is Infinite:\nTargets:%v\nOutputLayer:%v\n\n", targets, nn.OutputLayer) } } // Update weights for j := 0; j < len(nn.HiddenLayer); j++ { for k := 0; k < len(nn.OutputLayer); k++ { delta := nn.LearningRate * outputErrors[k] * nn.HiddenLayer[j] nn.WeightsHO[j][k] += delta } } for i := 0; i < len(nn.InputLayer); i++ { for j := 0; j < len(nn.HiddenLayer); j++ { delta := nn.LearningRate * hiddenErrors[j] * nn.InputLayer[i] nn.WeightsIH[i][j] += delta if math.IsNaN(delta) { fmt.Print(fmt.Sprintf("Delta is NaN.\n Learning Rate: %f\nHidden Errors: %f\nInput: %f\n", nn.LearningRate, hiddenErrors[j], nn.InputLayer[i])) } if math.IsNaN(nn.WeightsIH[i][j]) { fmt.Print(fmt.Sprintf("Delta is NaN.\n Learning Rate: %f\nHidden Errors: %f\nInput: %f\n", nn.LearningRate, hiddenErrors[j], nn.InputLayer[i])) } } } } func (nn *RawAI) ExportWeights(filename string) error { weightsJson, err := json.Marshal(nn) if err != nil { return err } err = ioutil.WriteFile(filename, weightsJson, 0644) if err != nil { return err } return nil } func (nn *RawAI) ImportWeights(filename string) error { weightsJson, err := ioutil.ReadFile(filename) if err != nil { return err } err = json.Unmarshal(weightsJson, nn) if err != nil { return err } return nil } //RawAI Tools: func randomMatrix(rows, cols int) [][]float64 { matrix := make([][]float64, rows) for i := 0; i < rows; i++ { matrix[i] = make([]float64, cols) for j := 0; j < cols; j++ { matrix[i][j] = 1.0 } } return matrix } func sigmoid(x float64) float64 { return 1.0 / (1.0 + exp(-x)) } func sigmoidDerivative(x float64) float64 { return x * (1.0 - x) } func exp(x float64) float64 { return 1.0 + x + (x*x)/2.0 + (x*x*x)/6.0 + (x*x*x*x)/24.0 }
The output example is as follows: As you can see, it slowly moves away from the target and continues to do so. After asking, googling and searching this site I couldn't find where my error was so I decided to ask this question.
Correct answer
I think you are using the mean squared error
and forgetting the -
after differentiating.
So change:
outputerrors[k] = (targets[k] - nn.outputlayer[k])
To:
outputErrors[k] = -(targets[k] - nn.OutputLayer[k])
The above is the detailed content of My neural network is trained (from scratch) to get further away from the goal. For more information, please follow other related articles on the PHP Chinese website!
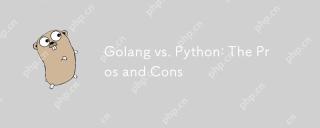
Golangisidealforbuildingscalablesystemsduetoitsefficiencyandconcurrency,whilePythonexcelsinquickscriptinganddataanalysisduetoitssimplicityandvastecosystem.Golang'sdesignencouragesclean,readablecodeanditsgoroutinesenableefficientconcurrentoperations,t
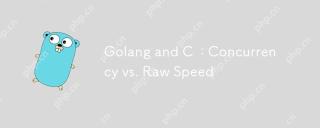
Golang is better than C in concurrency, while C is better than Golang in raw speed. 1) Golang achieves efficient concurrency through goroutine and channel, which is suitable for handling a large number of concurrent tasks. 2)C Through compiler optimization and standard library, it provides high performance close to hardware, suitable for applications that require extreme optimization.
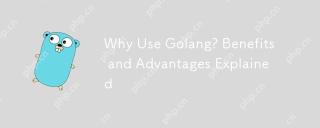
Reasons for choosing Golang include: 1) high concurrency performance, 2) static type system, 3) garbage collection mechanism, 4) rich standard libraries and ecosystems, which make it an ideal choice for developing efficient and reliable software.
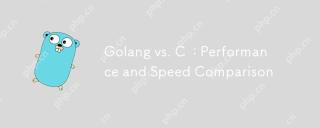
Golang is suitable for rapid development and concurrent scenarios, and C is suitable for scenarios where extreme performance and low-level control are required. 1) Golang improves performance through garbage collection and concurrency mechanisms, and is suitable for high-concurrency Web service development. 2) C achieves the ultimate performance through manual memory management and compiler optimization, and is suitable for embedded system development.
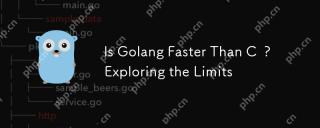
Golang performs better in compilation time and concurrent processing, while C has more advantages in running speed and memory management. 1.Golang has fast compilation speed and is suitable for rapid development. 2.C runs fast and is suitable for performance-critical applications. 3. Golang is simple and efficient in concurrent processing, suitable for concurrent programming. 4.C Manual memory management provides higher performance, but increases development complexity.
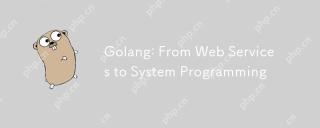
Golang's application in web services and system programming is mainly reflected in its simplicity, efficiency and concurrency. 1) In web services, Golang supports the creation of high-performance web applications and APIs through powerful HTTP libraries and concurrent processing capabilities. 2) In system programming, Golang uses features close to hardware and compatibility with C language to be suitable for operating system development and embedded systems.
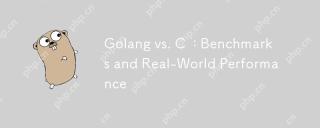
Golang and C have their own advantages and disadvantages in performance comparison: 1. Golang is suitable for high concurrency and rapid development, but garbage collection may affect performance; 2.C provides higher performance and hardware control, but has high development complexity. When making a choice, you need to consider project requirements and team skills in a comprehensive way.
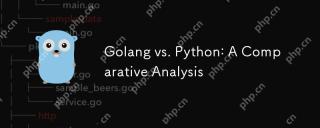
Golang is suitable for high-performance and concurrent programming scenarios, while Python is suitable for rapid development and data processing. 1.Golang emphasizes simplicity and efficiency, and is suitable for back-end services and microservices. 2. Python is known for its concise syntax and rich libraries, suitable for data science and machine learning.


Hot AI Tools

Undresser.AI Undress
AI-powered app for creating realistic nude photos

AI Clothes Remover
Online AI tool for removing clothes from photos.

Undress AI Tool
Undress images for free

Clothoff.io
AI clothes remover

Video Face Swap
Swap faces in any video effortlessly with our completely free AI face swap tool!

Hot Article

Hot Tools

PhpStorm Mac version
The latest (2018.2.1) professional PHP integrated development tool
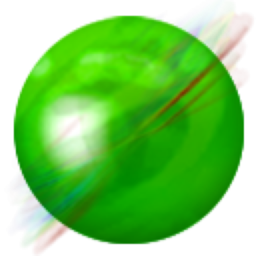
ZendStudio 13.5.1 Mac
Powerful PHP integrated development environment
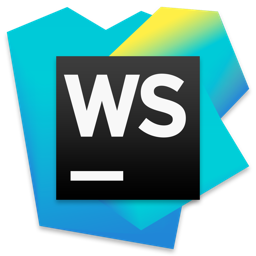
WebStorm Mac version
Useful JavaScript development tools

Safe Exam Browser
Safe Exam Browser is a secure browser environment for taking online exams securely. This software turns any computer into a secure workstation. It controls access to any utility and prevents students from using unauthorized resources.

Notepad++7.3.1
Easy-to-use and free code editor