


Without dividing it into tokens, learn directly from bytes efficiently. Mamba can also be used in this way.
When defining a language model, basic word segmentation methods are often used to divide sentences into words, subwords or characters. Subword segmentation has long been the most popular choice because it strikes a balance between training efficiency and the ability to handle words outside the vocabulary. However, some studies have pointed out problems with subword segmentation, such as lack of robustness to the handling of typos, spelling and case changes, and morphological changes. Therefore, these issues need to be carefully considered in the design of language models to improve the accuracy and robustness of the model.
Therefore, some researchers have chosen an approach that uses byte sequences, that is, through end-to-end mapping of raw data to prediction results, without any word segmentation. Compared with sub-word models, byte-level language models are easier to generalize to different writing forms and morphological changes. However, modeling text as bytes means that the generated sequences are longer than the corresponding subwords. In order to improve efficiency, it needs to be achieved by improving the architecture.
Autoregressive Transformer occupies a dominant position in language modeling, but its efficiency problem is particularly prominent. Its computational cost increases quadratically as the sequence length increases, resulting in poor scalability for long sequences. To solve this problem, the researchers compressed the Transformer’s internal representation in order to handle long sequences. One such approach is the development of a length-aware modeling approach that merges groups of tokens within an intermediate layer, thereby reducing computational cost. Recently, Yu et al. proposed a method called MegaByte Transformer. It uses fixed-size byte fragments to simulate compressed forms as subwords, thus reducing computational cost. However, this may not be the best solution at present and requires further research and improvement.
In a latest study, scholars at Cornell University introduced an efficient and simple byte-level language model called MambaByte. This model is derived from a direct improvement of the recently introduced Mamba architecture. The Mamba architecture is built on the state space model (SSM) method, while MambaByte introduces a more efficient selection mechanism, making it perform better when processing discrete data such as text, and also provides an efficient GPU implementation. The researchers briefly observed using unmodified Mamba and found that it was able to alleviate a major computational bottleneck in language modeling, thereby eliminating the need for patches and making full use of available computational resources.
- ##Paper title: MambaByte: Token-free Selective State Space Model
- Paper link: https://arxiv.org/pdf/2401.13660.pdf
In the experiment , they compared MambaByte with Transformers, SSM, and MegaByte (patching) architectures. These architectures are evaluated under fixed parameter and computational settings and on multiple long-form text datasets. Figure 1 summarizes their main findings.
Compared with byte-level Transformers, MambaByte provides a faster and high-performance solution, while computing efficiency has also been significantly improved. . The researchers also compared token-free language models with current state-of-the-art subword models and found that MambaByte is competitive in this regard and can handle longer sequences. The results of this study show that MambaByte can be a powerful alternative to existing tokenizers that rely on them, and is expected to promote the further development of end-to-end learning.
Background: Selective State Space Sequence Model
SSM models the temporal evolution of hidden states using first-order differential equations. Linear time-invariant SSM has shown good results in a variety of deep learning tasks. However, recently Mamba authors Gu and Dao argued that the constant dynamics of these methods lack input-dependent context selection in hidden states, which may be necessary for tasks such as language modeling. Therefore, they proposed the Mamba method, which is dynamically defined by taking a given input x(t) ∈ R, hidden state h(t) ∈ R^n, and output y(t) ∈ R as a time-varying continuous state at time t is:
Its parameters are the diagonal time-invariant system matrix A∈R^(n×n), and Time-varying input and output matrices B (t)∈R^(n×1) and C (t)∈R^(1×n).
To model discrete time series such as bytes, the continuous time dynamics in (1) must be approximated through discretization. This produces a discrete-time latent recurrence, with new matrices A, B and C at each time step, i.e.
Please note that ( 2) Similar to a linear version of a recurrent neural network, it can be applied in this recurrent form during language model generation. The discretization requires that each input position has a time step, that is, Δ[k], corresponding to x [k] = x (t_k) of . The discrete-time matrices A, B and C can then be calculated from Δ[k]. Figure 2 shows how Mamba models discrete sequences.
In Mamba, the SSM term is input selective, that is, B, C and Δ are defined as the input x [k]∈R^ Function of d:
where W_B ∈ R^(n×d) (the definition of C is similar), W_Δ ∈ R^(d×r) and W_R ∈ R^(r×d) (for some r ≪d) are learnable weights, while softplus ensures positivity. Note that for each input dimension d, the SSM parameters A, B, and C are the same, but the number of time steps Δ is different; this results in a hidden state size of n × d for each time step k.
Mamba embeds this SSM layer into a complete neural network language model. Specifically, the model employs a series of gating layers inspired by previous gated SSMs. Figure 3 shows the Mamba architecture that combines SSM layers with gated neural networks.
Parallel scan of linear recurrence. At training time, the authors have access to the entire sequence x, allowing more efficient calculation of linear recurrence. Research by Smith et al. [2023] demonstrates that sequential recurrence in linear SSM can be efficiently calculated using efficient parallel scans. For Mamba, the author first maps recurrence to L tuple sequences, where e_k =, and then defines an association operator
such that
. Finally, they applied a parallel scan to compute the sequence
. In general, this takes
time, using L/2 processors, where
is the cost of the matrix multiplication. Note that A is a diagonal matrix and linear recurrence can be computed in parallel in
time and O (nL) space. Parallel scans using diagonal matrices also run very efficiently, requiring only O (nL) FLOPs.
Experimental results
Table 2 shows the bits per byte (BPB) for each dataset. In this experiment, the MegaByte758M 262M and MambaByte models used the same number of FLOPs per byte (see Table 1). The authors found that MambaByte consistently outperformed MegaByte on all datasets. Additionally, the authors note that due to funding constraints they were unable to train MambaByte on the full 80B bytes, but MambaByte still outperformed MegaByte with 63% less computation and 63% less training data. Additionally, MambaByte-353M outperforms byte-scale Transformer and PerceiverAR.
##In so few training steps, why is MambaByte better than Does a much larger model perform better? Figure 1 further explores this relationship by looking at models with the same number of parameters. The figure shows that for MegaByte models of the same parameter size, the model with less input patching performs better, but after calculating normalization, they perform similarly. In fact, the full-length Transformer, while slower in absolute terms, also performs similarly to MegaByte after computational normalization. In contrast, switching to the Mamba architecture can significantly improve computational usage and model performance.
Based on these findings, Table 3 compares larger versions of these models on the PG19 dataset. In this experiment, the authors compared MambaByte-972M with MegaByte-1.3B 350M and other byte-level models as well as several SOTA subword models. They found that MambaByte-972M outperformed all byte-level models and was competitive with subword models even when trained on only 150B bytes.
Text generation. Autoregressive inference in Transformer models requires caching the entire context, which significantly affects generation speed. MambaByte does not have this bottleneck because it retains only one time-varying hidden state per layer, so the time per generation step is constant. Table 4 compares text generation speeds of MambaByte-972M and MambaByte-1.6B with MegaByte-1.3B 350M on an A100 80GB PCIe GPU. Although MegaByte greatly reduces the generation cost through patching, they observed that MambaByte is 2.6 times faster under similar parameter settings due to the use of loop generation.
The above is the detailed content of Without dividing it into tokens, learn directly from bytes efficiently. Mamba can also be used in this way.. For more information, please follow other related articles on the PHP Chinese website!
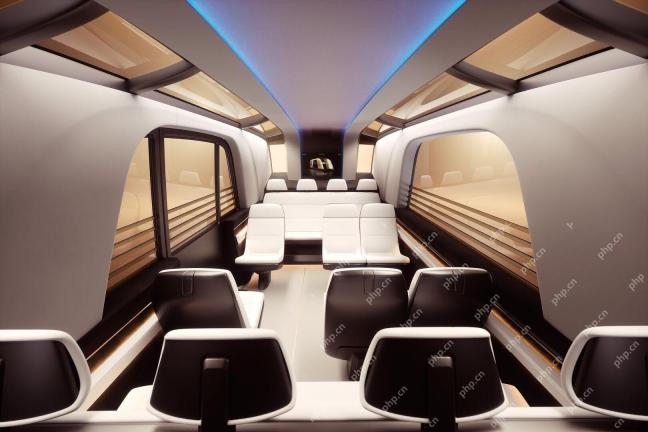
Since 2008, I've championed the shared-ride van—initially dubbed the "robotjitney," later the "vansit"—as the future of urban transportation. I foresee these vehicles as the 21st century's next-generation transit solution, surpas
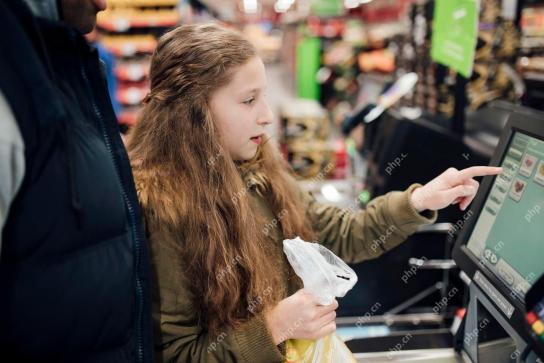
Revolutionizing the Checkout Experience Sam's Club's innovative "Just Go" system builds on its existing AI-powered "Scan & Go" technology, allowing members to scan purchases via the Sam's Club app during their shopping trip.
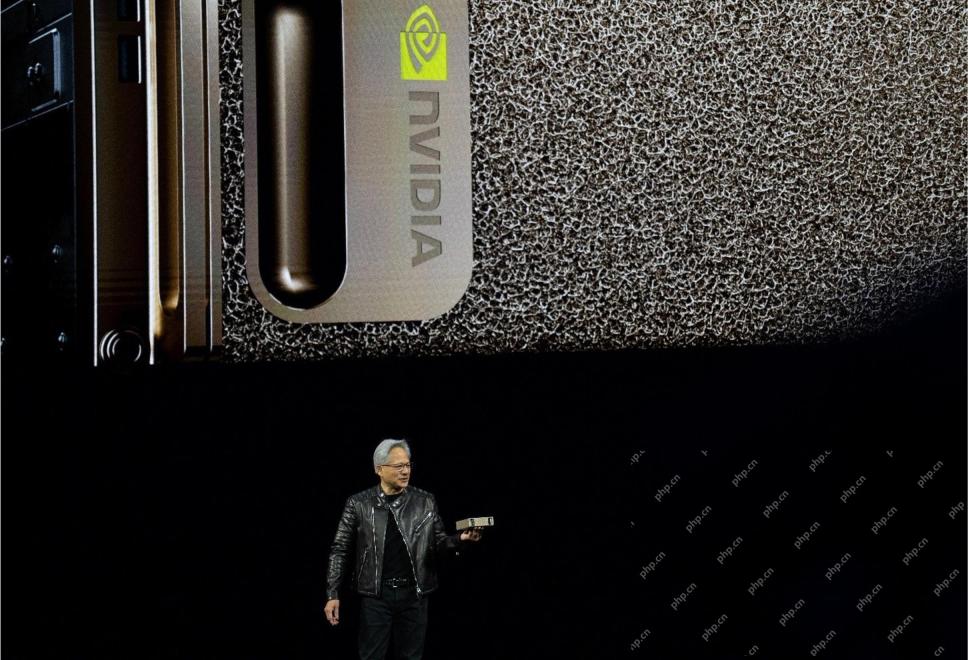
Nvidia's Enhanced Predictability and New Product Lineup at GTC 2025 Nvidia, a key player in AI infrastructure, is focusing on increased predictability for its clients. This involves consistent product delivery, meeting performance expectations, and
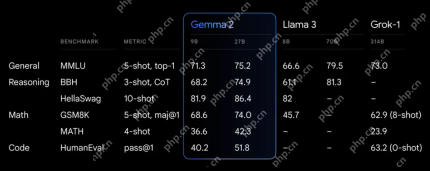
Google's Gemma 2: A Powerful, Efficient Language Model Google's Gemma family of language models, celebrated for efficiency and performance, has expanded with the arrival of Gemma 2. This latest release comprises two models: a 27-billion parameter ver
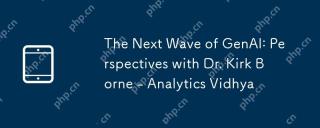
This Leading with Data episode features Dr. Kirk Borne, a leading data scientist, astrophysicist, and TEDx speaker. A renowned expert in big data, AI, and machine learning, Dr. Borne offers invaluable insights into the current state and future traje
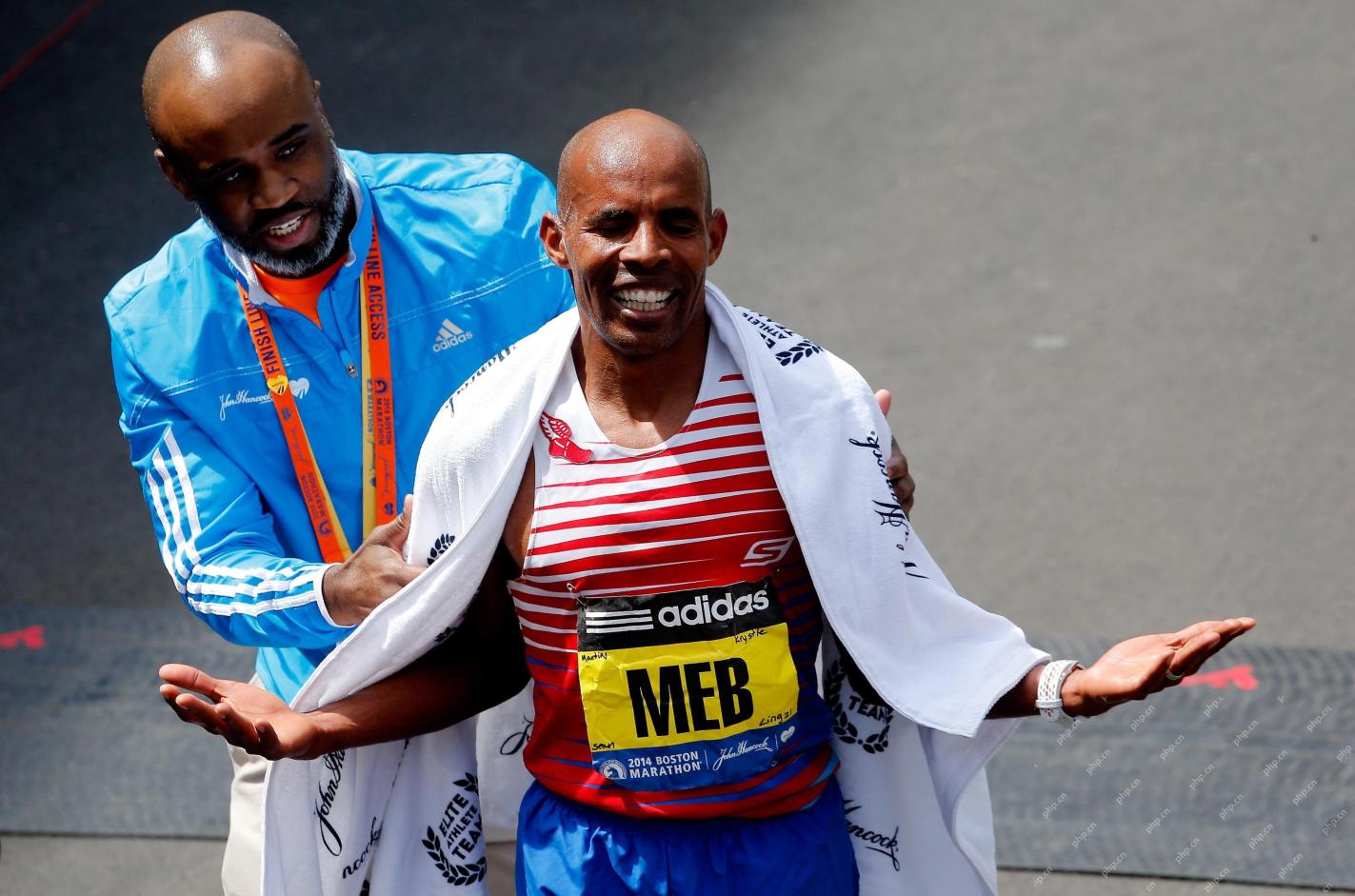
There were some very insightful perspectives in this speech—background information about engineering that showed us why artificial intelligence is so good at supporting people’s physical exercise. I will outline a core idea from each contributor’s perspective to demonstrate three design aspects that are an important part of our exploration of the application of artificial intelligence in sports. Edge devices and raw personal data This idea about artificial intelligence actually contains two components—one related to where we place large language models and the other is related to the differences between our human language and the language that our vital signs “express” when measured in real time. Alexander Amini knows a lot about running and tennis, but he still
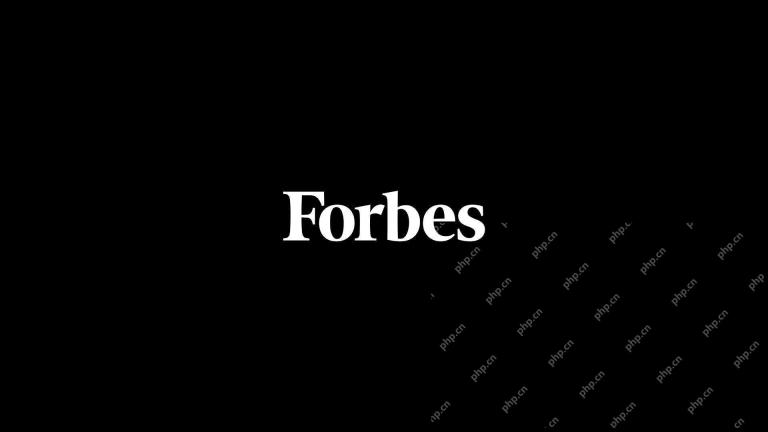
Caterpillar's Chief Information Officer and Senior Vice President of IT, Jamie Engstrom, leads a global team of over 2,200 IT professionals across 28 countries. With 26 years at Caterpillar, including four and a half years in her current role, Engst
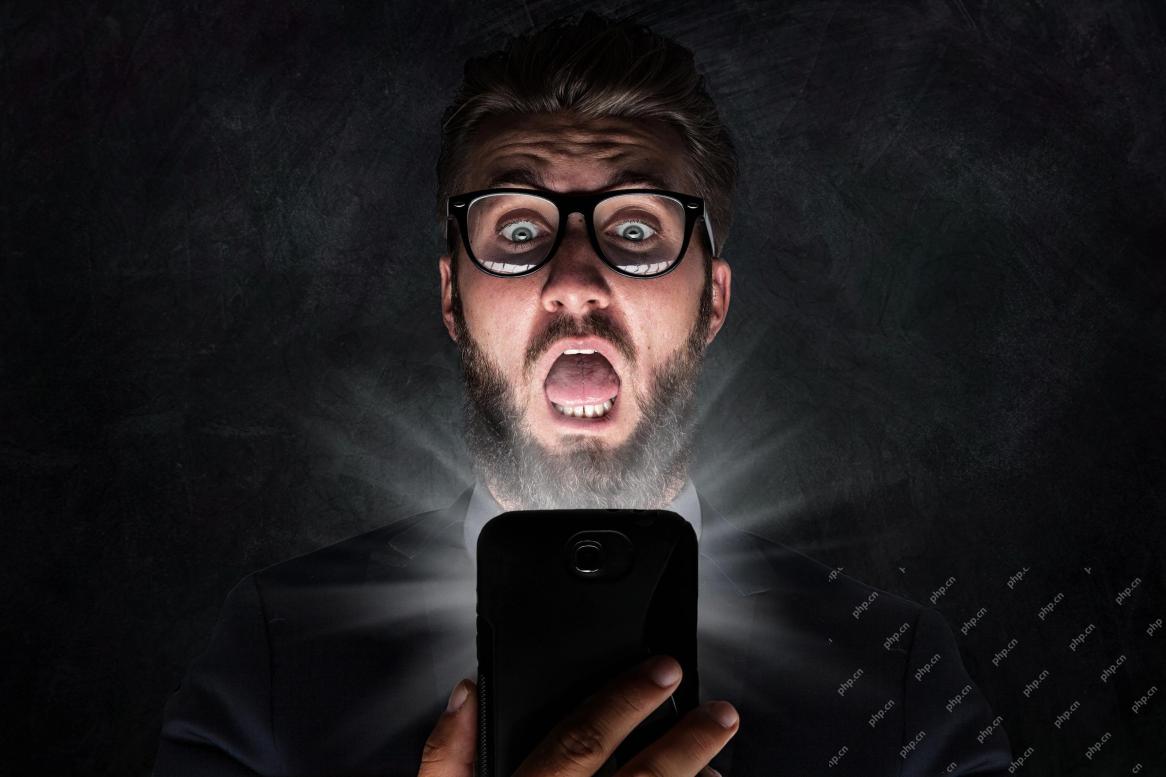
Google Photos' New Ultra HDR Tool: A Quick Guide Enhance your photos with Google Photos' new Ultra HDR tool, transforming standard images into vibrant, high-dynamic-range masterpieces. Ideal for social media, this tool boosts the impact of any photo,


Hot AI Tools

Undresser.AI Undress
AI-powered app for creating realistic nude photos

AI Clothes Remover
Online AI tool for removing clothes from photos.

Undress AI Tool
Undress images for free

Clothoff.io
AI clothes remover

Video Face Swap
Swap faces in any video effortlessly with our completely free AI face swap tool!

Hot Article

Hot Tools
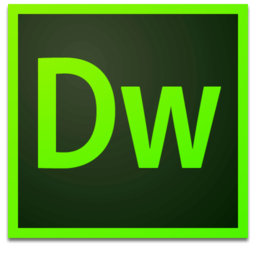
Dreamweaver Mac version
Visual web development tools

SublimeText3 Linux new version
SublimeText3 Linux latest version

SecLists
SecLists is the ultimate security tester's companion. It is a collection of various types of lists that are frequently used during security assessments, all in one place. SecLists helps make security testing more efficient and productive by conveniently providing all the lists a security tester might need. List types include usernames, passwords, URLs, fuzzing payloads, sensitive data patterns, web shells, and more. The tester can simply pull this repository onto a new test machine and he will have access to every type of list he needs.
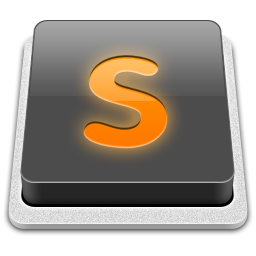
SublimeText3 Mac version
God-level code editing software (SublimeText3)

SublimeText3 Chinese version
Chinese version, very easy to use