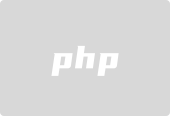
How to make artificial intelligence real? So how to make artificial intelligence real, from data to wisdom? Let’s delve deeper.
How to make artificial intelligence real
Making artificial intelligence real requires considering many aspects, such as data, models, algorithms, user experience and ethics . Here are some suggestions to help achieve realism:
-
Diverse and quality data: Use diverse and high-quality data sets to train the model. Ensure that the data set contains a variety of situations, contexts, and features to improve the model's generalization ability.
-
Transparency and interpretability: Design models with transparency and interpretability. Users need to understand the decision-making process of artificial intelligence systems, especially in key areas (such as medical, finance, etc.). Explainability helps build user trust in the system.
-
Fairness and unbiasedness: Ensure that AI systems are fair across different groups and avoid bias against certain groups. Monitoring and correcting potential bias in models is key to ensuring impartiality.
-
Human-machine co-design: Design artificial intelligence systems as tools to work with human users, rather than to replace humans. This kind of collaborative design helps to better integrate artificial intelligence technology and human intelligence, improving the practicality and acceptability of the system.
-
Personalization and adaptability: Build systems that can be personalized to user needs. By taking individual differences into account, systems can better meet user expectations and improve user experience.
-
User participation and feedback: Absorb user feedback and incorporate it into the process of model improvement. User participation can ensure that the system better meets user needs while improving users' trust in the system.
-
Real-time learning and updating: Real-time learning and updating of the system to adapt to changing environments and needs. This can be achieved through techniques such as online learning and incremental learning.
-
Ethical and regulatory compliance: Strictly abide by relevant ethics and regulations to ensure that the development and use of artificial intelligence systems comply with social and statutory ethical standards.
-
Security and Privacy: Emphasis on system security to prevent potential abuse and attacks. At the same time, the privacy rights of users are protected and compliance with handling of sensitive information is ensured.
-
Sustainable development: Incorporate the development and use of artificial intelligence systems into the scope of sustainable development and consider its long-term impact on the environment, society and economy.
By comprehensively considering these factors, artificial intelligence can be more realistic and develop in tandem with the complex and ever-changing real world.
How to make artificial intelligence real - from data to wisdom
To make artificial intelligence real, it needs to be upgraded from simple data processing to the level of deep intelligence . This involves data collection, processing, model training and intelligent system applications. Here are some recommended steps:
1. Data collection: Collect diverse, high-quality data, including structured and unstructured data.
2. Data processing: Use appropriate technologies and algorithms for data cleaning, integration and transformation to ensure data accuracy and consistency.
3. Model training: Select appropriate machine learning algorithms and models for training, and use large-scale data sets to optimize and adjust the model.
4. Practical application: Apply the trained model to actual scenarios and integrate it with existing systems to achieve intelligent
-
Data collection and cleaning: First, you need to ensure the quality and diversity of the data collected. This involves collecting large amounts of data from a variety of sources, including structured data (e.g. tabular data in databases), semi-structured data (e.g. log files) and unstructured data (e.g. text, images, audio). Data cleaning is a critical step in ensuring data quality, including handling missing values, outliers, and erroneous data.
-
Feature engineering: Feature engineering refers to converting raw data into features that can be used in machine learning models. This may involve transforming, scaling, combining, etc. the data to extract features that are meaningful to the problem. Good feature engineering can improve model performance.
-
Choose an appropriate model: Choose an appropriate machine learning or deep learning model based on the nature of the problem. This may include traditional supervised learning models (such as decision trees, support vector machines), deep learning models (such as neural networks), or some other domain-specific model.
-
Model training: Use a large amount of labeled data to train the selected model. This includes adjusting parameters in the model to allow it to better fit the data and improve its ability to generalize to new data.
-
Continuous learning: Realize continuous learning of the model so that the model can adapt to new data and changes in a timely manner. This can be achieved through online learning techniques, incremental learning, or regular model updates.
-
Interpretability and transparency: Considering the needs of some application scenarios, ensure that the model has a certain degree of interpretability and transparency so that users and stakeholders can understand the decision-making process of the model.
-
Practical application: Deploy the model to the actual application environment and monitor its performance. This includes ensuring that the model can effectively handle new data in a production environment and updating it when necessary.
-
Ethics and regulations: Considering that artificial intelligence applications may involve sensitive information, ensure that relevant ethics and regulations are followed during model development and application, and privacy and fairness are guaranteed.
-
User feedback and improvements: Collect user feedback and use this feedback to continuously improve the model. This helps ensure that AI systems are aligned with user needs and expectations.
Through these steps, artificial intelligence can gradually achieve deeper intelligence, developing from simple data processing to applications with realism and intelligence.
The above is the detailed content of Making artificial intelligence real: Strategies from data to intelligence. For more information, please follow other related articles on the PHP Chinese website!