Learning method for zero-based image recognition
Image recognition based on zero-shot learning is an emerging technology, which is different from traditional image recognition methods. Traditional image recognition requires learning features and classification rules through training data, while zero-shot learning does not require pre-training the model. It performs real-time classification based on the characteristics of the image to be recognized, thereby achieving fast and accurate recognition. Image recognition with zero-shot learning has been widely used in smart home, face recognition, smart security and other fields. It can help smart home devices quickly identify user needs and respond accordingly. In face recognition, zero-shot learning can accurately identify faces based on their features and improve recognition accuracy. In the field of intelligent security, zero-shot learning can help identify dangerous objects and provide a safer and more reliable monitoring system. In short, image recognition technology based on zero-shot learning is fast and accurate, providing more intelligent solutions for various fields.
Zero-shot image recognition is mainly divided into two stages: feature extraction and classification.
In the feature extraction stage, the zero-shot learning image recognition algorithm will automatically analyze various features in the image to be recognized, such as color, shape, texture, etc., and represent them as vectors . These vectors can be regarded as the "fingerprints" of the image to be recognized and used for subsequent classification.
In the classification stage, the zero-shot learning image recognition algorithm uses feature vectors to compare with previously learned category feature vectors to find the category closest to the image to be recognized. These category feature vectors are extracted from other images, and they represent features of each category. When recognizing a new image, the zero-shot learning image recognition algorithm assigns the image to be recognized to the closest category based on how similar it is to the feature vectors of each category.
In order to better understand zero-shot learning, we can illustrate it through an example. We adopt the Animals with Attributes 2 (AWA2) dataset, which contains 50 different animal categories, each category is described by 85 attributes. We randomly selected 10 categories as the training set and the remaining 40 categories as the testing set. We used an attribute-based approach for model training.
First, we need to import the necessary libraries and datasets:
import numpy as np import pandas as pd import scipy.io as sio from sklearn.preprocessing import StandardScaler from sklearn.linear_model import LogisticRegression # 导入数据集 data = sio.loadmat('data/awa2.mat') train_labels = data['train_labels'].astype(int).squeeze() test_labels = data['test_labels'].astype(int).squeeze() train_attributes = StandardScaler().fit_transform(data['train_attributes']) test_attributes = StandardScaler().fit_transform(data['test_attributes'])
Then, we need to convert the attribute descriptions into vectors in the embedding space. We use principal component analysis (PCA) to convert attribute descriptions into vectors in embedding space. We select the first 10 principal components as embedding vectors.
from sklearn.decomposition import PCA # 将属性描述转换为嵌入空间中的向量 pca = PCA(n_components=10) train_embed = pca.fit_transform(train_attributes) test_embed = pca.transform(test_attributes)
Next, we need to train a classifier to predict the categories in the test set. We use logistic regression as classifier.
# 训练分类器 clf = LogisticRegression(random_state=0, max_iter=1000) clf.fit(train_embed, train_labels) # 在测试集上进行预测 predicted_labels = clf.predict(test_embed)
Finally, we can calculate the accuracy to evaluate the performance of the model.
# 计算准确率 accuracy = np.mean(predicted_labels == test_labels) print('Accuracy:', accuracy)
In this example, we used an attribute-based approach to train the model and selected the first 10 principal components as embedding vectors. Finally, we got a model with an accuracy of 0.55 on the test set.
The above is the detailed content of Learning method for zero-based image recognition. For more information, please follow other related articles on the PHP Chinese website!
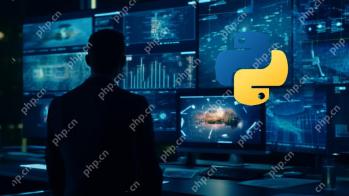
Python GUI Development Simplified with PySimpleGUI Developing user-friendly graphical interfaces (GUIs) in Python can be challenging. However, PySimpleGUI offers a streamlined and accessible solution. This article explores PySimpleGUI's core functio
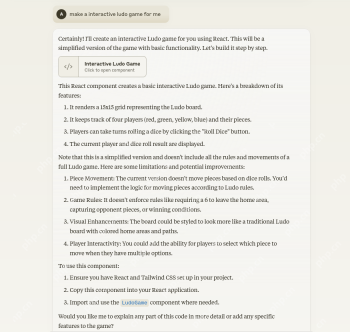
Introduction Large language models (LLMs) rapidly transform how we interact with information and complete tasks. Among these, Claude 3.5 Sonnet, developed by Anthropic AI, stands out for its exceptional capabilities. Experts o
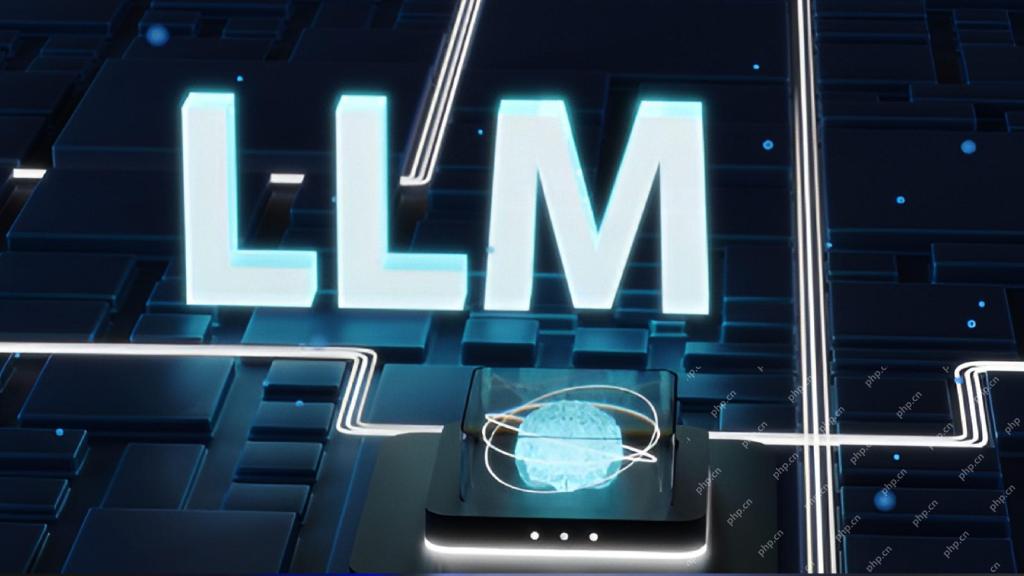
Introduction Large Language Models (LLMs) have made significant strides in natural language processing and generation. However, the typical zero-shot approach, producing output in a single pass without refinement, has limitations. A key challenge i
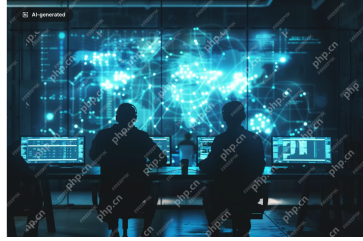
Functional vs. Object-Oriented Programming: A Detailed Comparison Object-oriented programming (OOP) and functional programming (FP) are the most prevalent programming paradigms, offering diverse approaches to software development. Understanding thei
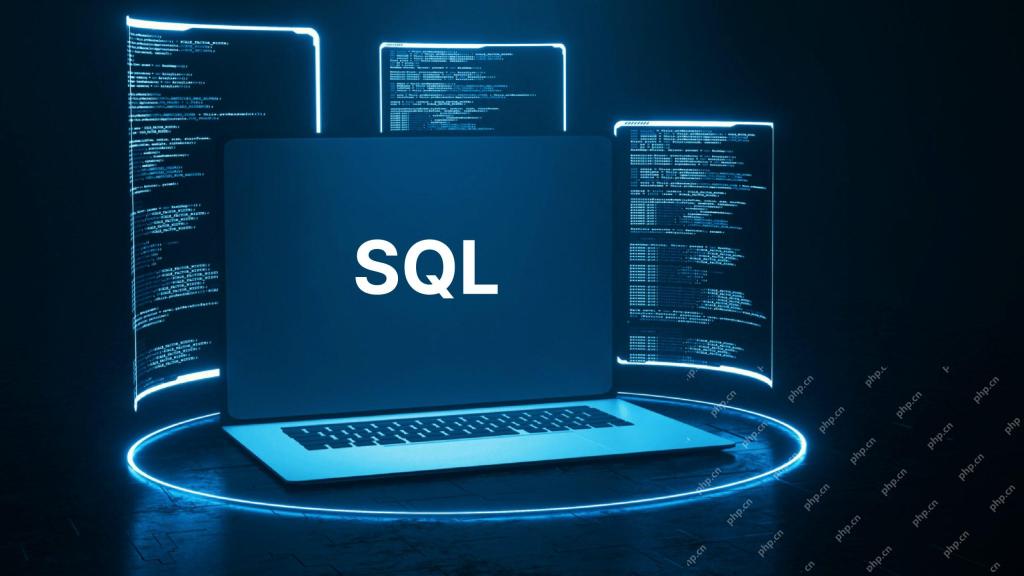
Introduction SQL keys are fundamental, with primary, foreign, and candidate keys holding significant importance. Often overlooked, however, are alternate keys, which play a crucial role in database design, data integrity, and efficient record retrie
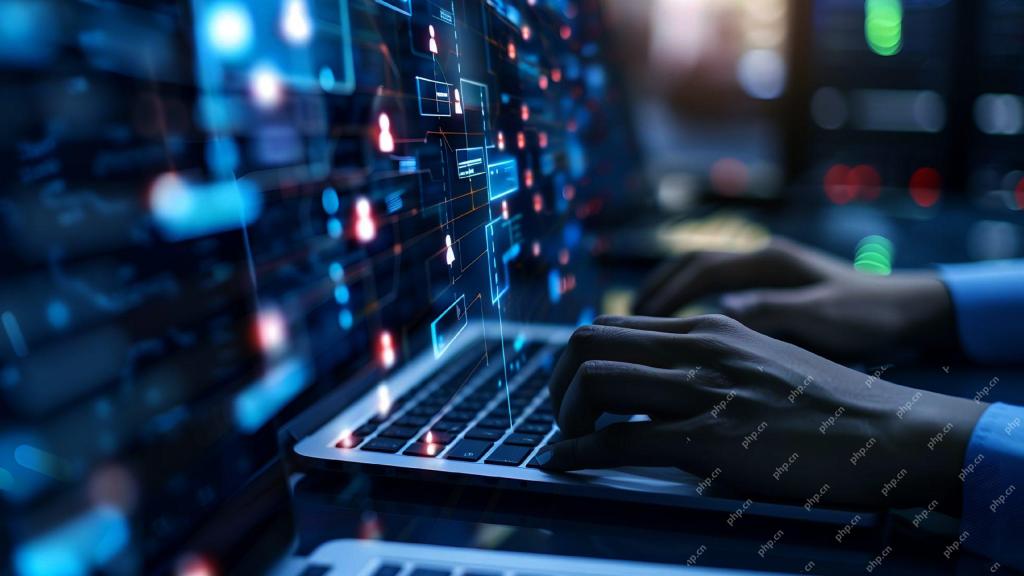
Introduction SQL indexes are essential for optimizing database performance. They act as lookup tables, significantly speeding up data retrieval. Think of them as a book's index – they help you find specific information quickly without reading the en
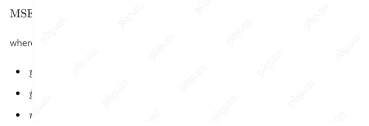
Introduction Mean squared error (MSE), a fundamental concept in statistics and machine learning, is a key metric for assessing model accuracy. It quantifies the discrepancy between a model's predictions and the actual values. MSE's simplicity and e
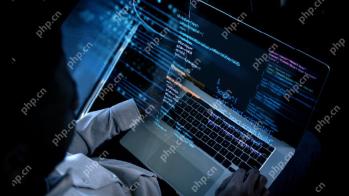
Introduction Mastering data formatting is essential for any data scientist or analyst. Well-formatted data enhances readability and user-friendliness, ensuring stakeholders can easily grasp insights. SQL Server's FORMAT() function offers powerful ca


Hot AI Tools

Undresser.AI Undress
AI-powered app for creating realistic nude photos

AI Clothes Remover
Online AI tool for removing clothes from photos.

Undress AI Tool
Undress images for free

Clothoff.io
AI clothes remover

Video Face Swap
Swap faces in any video effortlessly with our completely free AI face swap tool!

Hot Article

Hot Tools

MantisBT
Mantis is an easy-to-deploy web-based defect tracking tool designed to aid in product defect tracking. It requires PHP, MySQL and a web server. Check out our demo and hosting services.

mPDF
mPDF is a PHP library that can generate PDF files from UTF-8 encoded HTML. The original author, Ian Back, wrote mPDF to output PDF files "on the fly" from his website and handle different languages. It is slower than original scripts like HTML2FPDF and produces larger files when using Unicode fonts, but supports CSS styles etc. and has a lot of enhancements. Supports almost all languages, including RTL (Arabic and Hebrew) and CJK (Chinese, Japanese and Korean). Supports nested block-level elements (such as P, DIV),
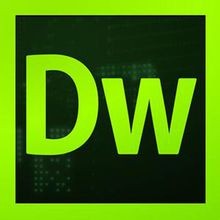
Dreamweaver CS6
Visual web development tools

DVWA
Damn Vulnerable Web App (DVWA) is a PHP/MySQL web application that is very vulnerable. Its main goals are to be an aid for security professionals to test their skills and tools in a legal environment, to help web developers better understand the process of securing web applications, and to help teachers/students teach/learn in a classroom environment Web application security. The goal of DVWA is to practice some of the most common web vulnerabilities through a simple and straightforward interface, with varying degrees of difficulty. Please note that this software
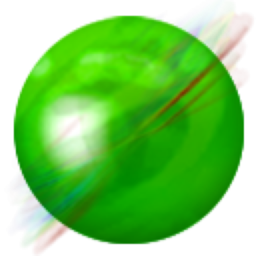
ZendStudio 13.5.1 Mac
Powerful PHP integrated development environment