Introduction
Mean squared error (MSE), a fundamental concept in statistics and machine learning, is a key metric for assessing model accuracy. It quantifies the discrepancy between a model's predictions and the actual values. MSE's simplicity and effectiveness make it a popular choice for evaluating model performance. This article explores mean squared error through practical examples.
Overview
This article covers:
- The mathematical definition and representation of mean squared error.
- Calculating MSE for a given set of actual and predicted values.
- Understanding MSE's sensitivity to outliers and its implications for model evaluation.
- Comparing MSE with other error metrics, such as Root Mean Squared Error and Mean Absolute Error.
- Applying MSE in real-world scenarios, including forecasting, hyperparameter tuning, and model assessment.
Table of contents
- What is Mean Squared Error?
- Key Concepts
- Examples
- Practical Applications
- Limitations
- Frequently Asked Questions
What is Mean Squared Error?
Mean squared error represents the average of the squared differences between predicted and observed values. Mathematically:
Squaring the errors prevents positive and negative differences from canceling each other out. Furthermore, squaring amplifies the impact of larger errors, making MSE particularly sensitive to outliers.
Key Concepts
Understanding these concepts is crucial for working with MSE:
Error Calculation
The error for each prediction is the difference between the predicted and actual value. This indicates the prediction's accuracy, with positive or negative values representing over- or under-estimation.
Averaging Squared Errors
The mean squared error is calculated by summing the squared errors and dividing by the number of observations. This averaging provides a scaled measure of the average prediction error.
Outlier Sensitivity
MSE's sensitivity to outliers stems from the squaring operation. Large errors are disproportionately weighted, significantly influencing the overall MSE value.
Comparison with Other Metrics
- Mean Absolute Error (MAE): MAE averages the absolute differences, unlike MSE. Less sensitive to outliers, MAE doesn't penalize large errors as severely.
- Root Mean Squared Error (RMSE): RMSE is the square root of MSE. Expressed in the original data's units, RMSE offers better interpretability.
Examples
Let's illustrate MSE calculation with examples:
Example 1: Simple Linear Regression
Consider a simple linear regression model predicting house prices based on size:
Actual Price ($) | Predicted Price ($) |
---|---|
200,000 | 195,000 |
250,000 | 260,000 |
300,000 | 310,000 |
350,000 | 345,000 |
400,000 | 390,000 |
The MSE calculation involves these steps:
1. Calculate errors:
2. Square the errors:
3. Sum the squared errors:
4. Divide by the number of observations:
The resulting MSE is 70,000,000.
Example 2: Model Comparison
Suppose two models predict the same data, with Model A having an MSE of 10,000 and Model B having an MSE of 5,000. Model B is preferred due to its lower MSE, indicating better predictive accuracy.
Practical Applications
MSE finds application in various areas:
Model Evaluation
MSE is frequently used to compare the performance of different regression models. The model with the lowest MSE is generally considered the most accurate.
Hyperparameter Tuning
During model training, MSE can serve as a loss function, guiding the optimization process. Minimizing MSE helps find optimal model parameters.
Forecasting
In time series analysis, MSE assesses the accuracy of forecasts. Lower MSE values signify more precise predictions, crucial for informed decision-making.
Limitations
Despite its usefulness, MSE has limitations:
- Outlier Sensitivity: Large errors disproportionately influence MSE.
- Interpretability: MSE's units are the square of the original data's units, potentially reducing interpretability.
Conclusion
Mean Squared Error is a vital metric for evaluating the accuracy of predictive models. Its simplicity and efficiency make it a popular choice for model comparison and evaluation. However, for a comprehensive analysis, consider using additional metrics like MAE and RMSE, and be mindful of its sensitivity to outliers. Understanding MSE's strengths and limitations leads to better model development and more accurate predictions.
Frequently Asked Questions
Q1. What is MSE? A. Mean Squared Error (MSE) measures the average squared difference between predicted and actual values, assessing a model's predictive accuracy.
Q2. Why is MSE sensitive to outliers? A. The squaring of errors in MSE amplifies the impact of large errors, making it sensitive to outliers.
Q3. When should I use MSE over other error metrics? A. MSE is often used alongside MAE and RMSE. It's particularly useful when large errors are highly undesirable.
The above is the detailed content of Mean Squared Error: Definition and Formula. For more information, please follow other related articles on the PHP Chinese website!
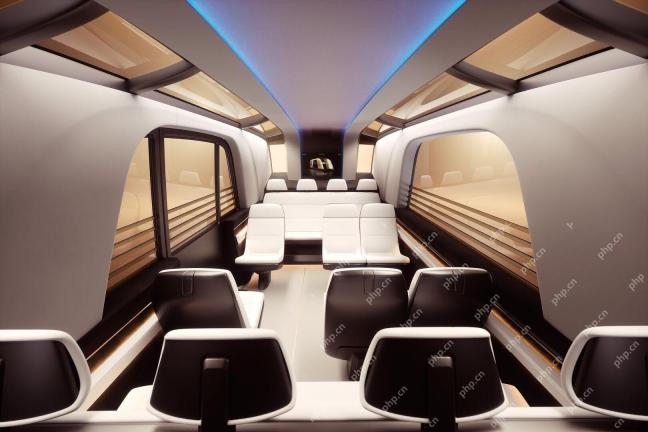
Since 2008, I've championed the shared-ride van—initially dubbed the "robotjitney," later the "vansit"—as the future of urban transportation. I foresee these vehicles as the 21st century's next-generation transit solution, surpas
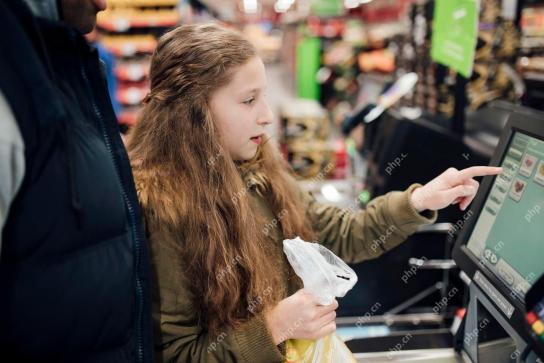
Revolutionizing the Checkout Experience Sam's Club's innovative "Just Go" system builds on its existing AI-powered "Scan & Go" technology, allowing members to scan purchases via the Sam's Club app during their shopping trip.
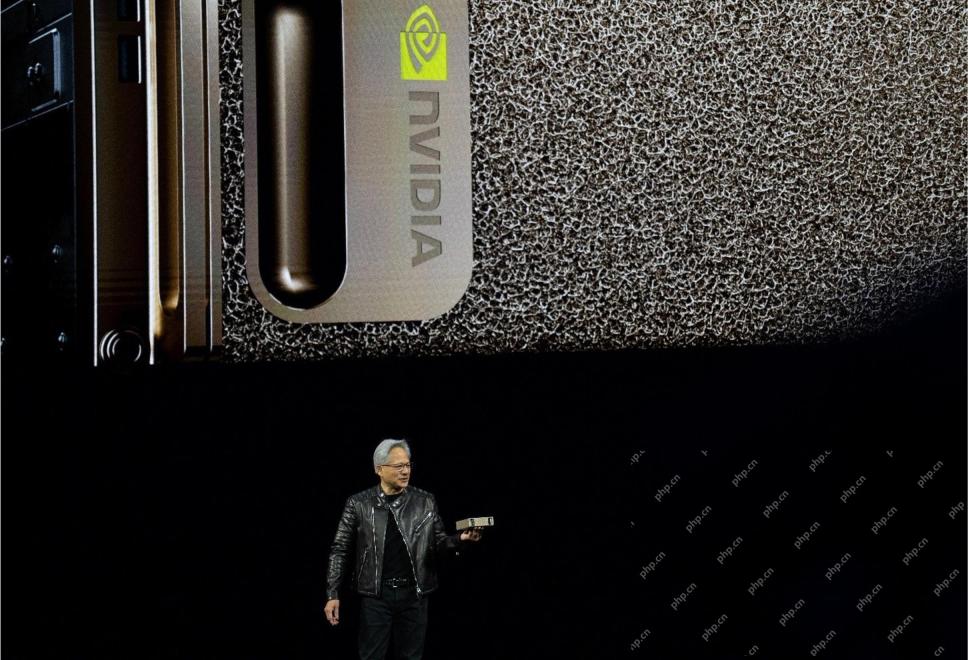
Nvidia's Enhanced Predictability and New Product Lineup at GTC 2025 Nvidia, a key player in AI infrastructure, is focusing on increased predictability for its clients. This involves consistent product delivery, meeting performance expectations, and
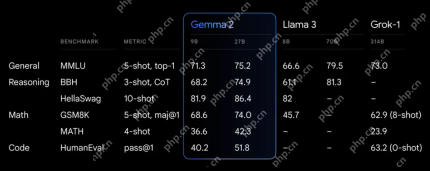
Google's Gemma 2: A Powerful, Efficient Language Model Google's Gemma family of language models, celebrated for efficiency and performance, has expanded with the arrival of Gemma 2. This latest release comprises two models: a 27-billion parameter ver
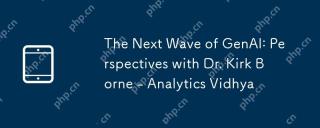
This Leading with Data episode features Dr. Kirk Borne, a leading data scientist, astrophysicist, and TEDx speaker. A renowned expert in big data, AI, and machine learning, Dr. Borne offers invaluable insights into the current state and future traje
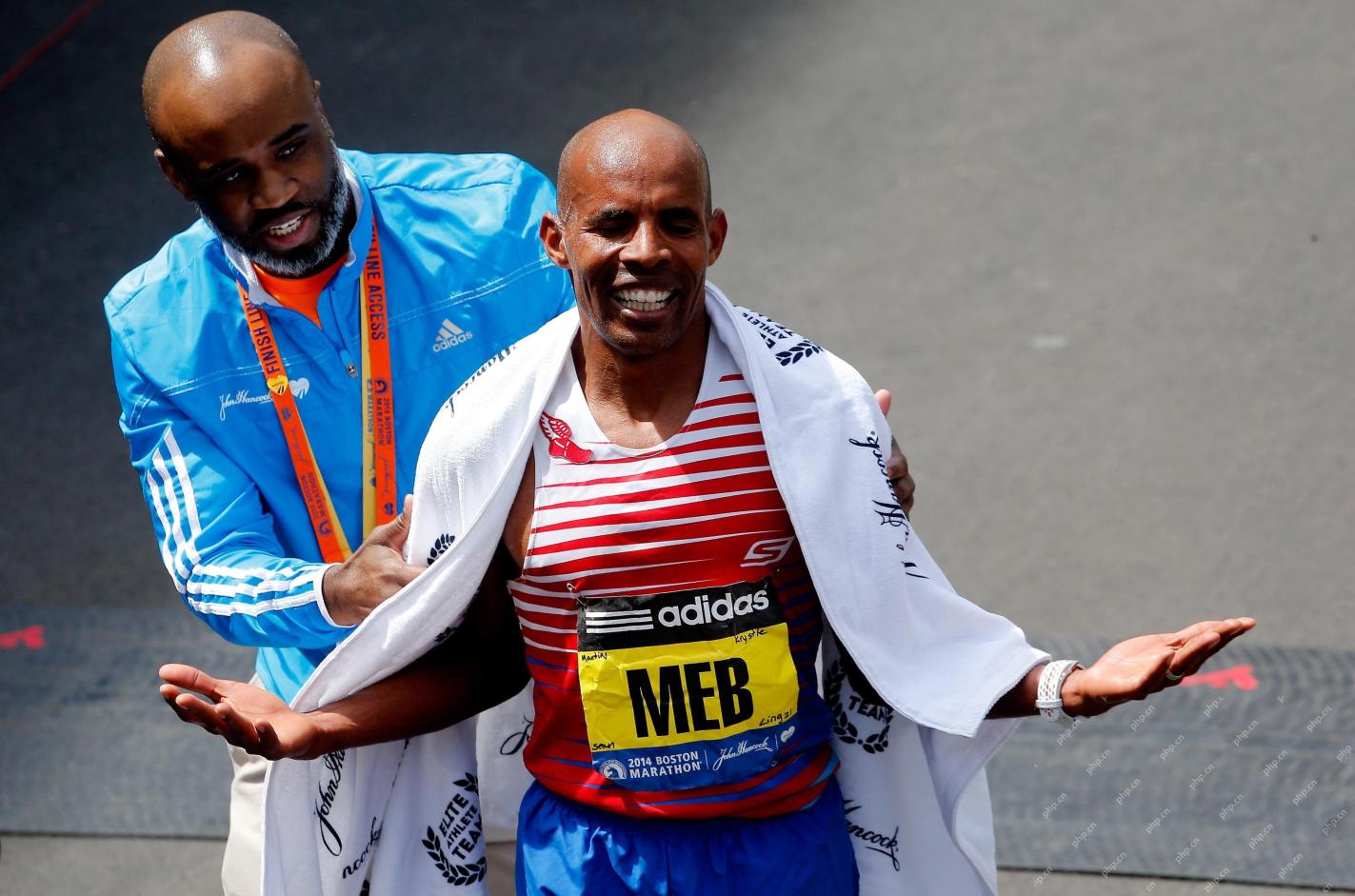
There were some very insightful perspectives in this speech—background information about engineering that showed us why artificial intelligence is so good at supporting people’s physical exercise. I will outline a core idea from each contributor’s perspective to demonstrate three design aspects that are an important part of our exploration of the application of artificial intelligence in sports. Edge devices and raw personal data This idea about artificial intelligence actually contains two components—one related to where we place large language models and the other is related to the differences between our human language and the language that our vital signs “express” when measured in real time. Alexander Amini knows a lot about running and tennis, but he still
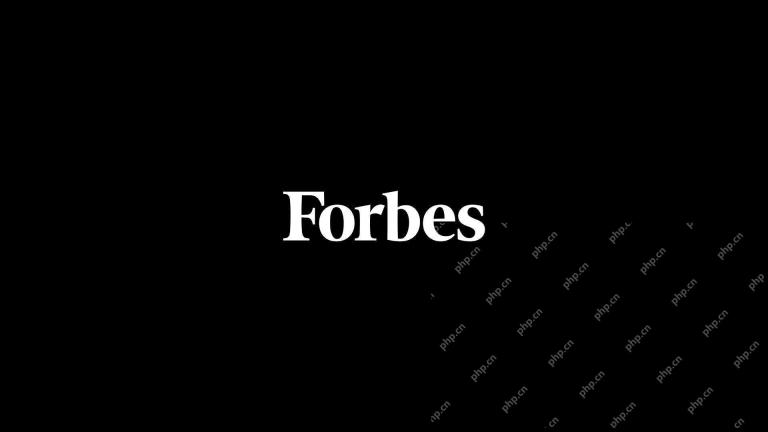
Caterpillar's Chief Information Officer and Senior Vice President of IT, Jamie Engstrom, leads a global team of over 2,200 IT professionals across 28 countries. With 26 years at Caterpillar, including four and a half years in her current role, Engst
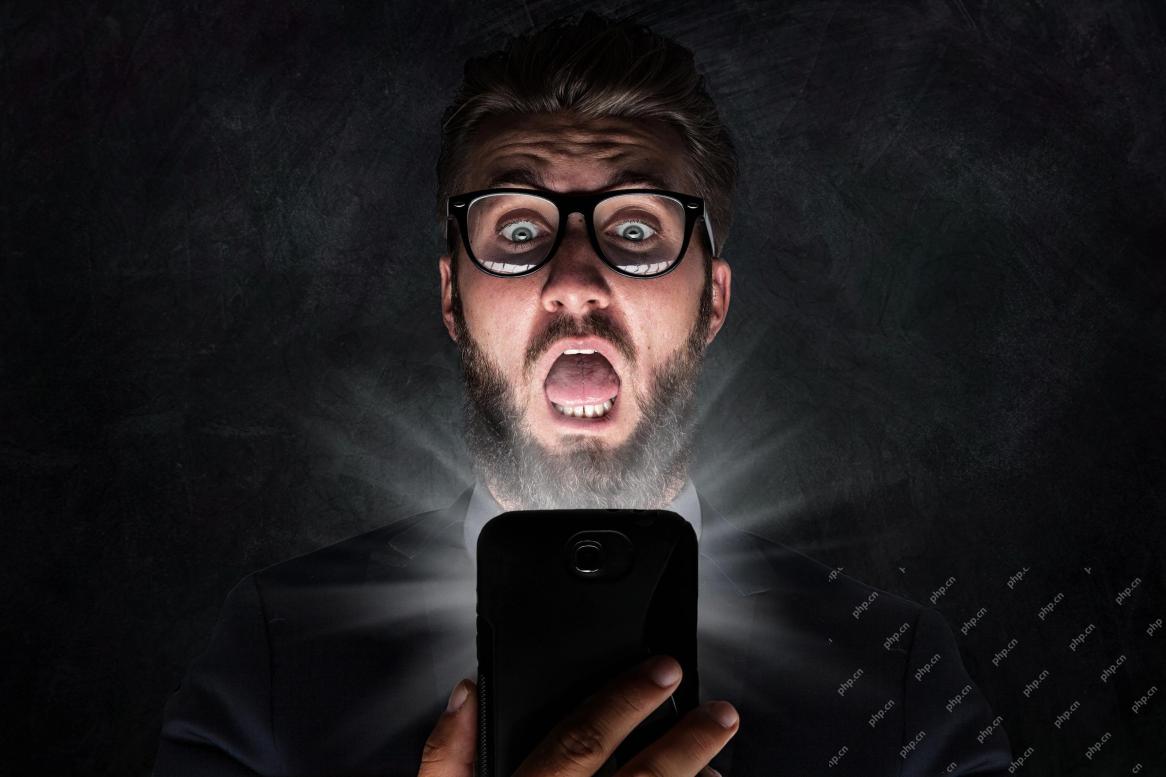
Google Photos' New Ultra HDR Tool: A Quick Guide Enhance your photos with Google Photos' new Ultra HDR tool, transforming standard images into vibrant, high-dynamic-range masterpieces. Ideal for social media, this tool boosts the impact of any photo,


Hot AI Tools

Undresser.AI Undress
AI-powered app for creating realistic nude photos

AI Clothes Remover
Online AI tool for removing clothes from photos.

Undress AI Tool
Undress images for free

Clothoff.io
AI clothes remover

Video Face Swap
Swap faces in any video effortlessly with our completely free AI face swap tool!

Hot Article

Hot Tools

Atom editor mac version download
The most popular open source editor

SublimeText3 English version
Recommended: Win version, supports code prompts!

mPDF
mPDF is a PHP library that can generate PDF files from UTF-8 encoded HTML. The original author, Ian Back, wrote mPDF to output PDF files "on the fly" from his website and handle different languages. It is slower than original scripts like HTML2FPDF and produces larger files when using Unicode fonts, but supports CSS styles etc. and has a lot of enhancements. Supports almost all languages, including RTL (Arabic and Hebrew) and CJK (Chinese, Japanese and Korean). Supports nested block-level elements (such as P, DIV),

DVWA
Damn Vulnerable Web App (DVWA) is a PHP/MySQL web application that is very vulnerable. Its main goals are to be an aid for security professionals to test their skills and tools in a legal environment, to help web developers better understand the process of securing web applications, and to help teachers/students teach/learn in a classroom environment Web application security. The goal of DVWA is to practice some of the most common web vulnerabilities through a simple and straightforward interface, with varying degrees of difficulty. Please note that this software

MinGW - Minimalist GNU for Windows
This project is in the process of being migrated to osdn.net/projects/mingw, you can continue to follow us there. MinGW: A native Windows port of the GNU Compiler Collection (GCC), freely distributable import libraries and header files for building native Windows applications; includes extensions to the MSVC runtime to support C99 functionality. All MinGW software can run on 64-bit Windows platforms.