GPT-4, regarded as one of the most powerful language models in the world since its release, has unfortunately experienced a series of crises of trust.
If we connect the "intermittent intelligence" incident earlier this year with OpenAI's redesign of the GPT-4 architecture, then there are recent reports that GPT-4 has become "lazy" "The rumors are even more interesting. Someone tested and found that as long as you tell GPT-4 "it is winter vacation", it will become lazy, as if it has entered a hibernation state.
To solve the problem of poor zero-sample performance of the model on new tasks, we can take the following methods: 1. Data enhancement: Increase the generalization ability of the model by expanding and transforming existing data. For example, image data can be altered by rotation, scaling, translation, etc., or by synthesizing new data samples. 2. Transfer learning: Use models that have been trained on other tasks to transfer their parameters and knowledge to new tasks. This can leverage existing knowledge and experience to improve
Recently, researchers from the University of California, Santa Cruz published a new discovery in a paper that may It can explain the underlying reasons for the performance degradation of GPT-4.
“We found that LLM performed surprisingly better on datasets released before the training data creation date. Datasets released later."
They perform well on "seen" tasks and perform poorly on new tasks. This means that LLM is just a method of imitating intelligence based on approximate retrieval, mainly memorizing things without any level of understanding.
To put it bluntly, LLM’s generalization ability is “not as strong as stated” - the foundation is not solid, and there will always be mistakes in actual combat.
One of the major reasons for this result is "task pollution", which is one form of data pollution. The data pollution we are familiar with before is test data pollution, which is the inclusion of test data examples and labels in the pre-training data. "Task contamination" is the addition of task training examples to pre-training data, making the evaluation in zero-sample or few-sample methods no longer realistic and effective.
The researcher conducted a systematic analysis of the data pollution problem for the first time in the paper:
Paper link: https://arxiv.org/pdf/2312.16337.pdf
After reading the paper, someone said "pessimistically":
This is the fate of all machine learning (ML) models that do not have the ability to continuously learn, that is, ML models The weights are frozen after training, but the input distribution continues to change, and if the model cannot continue to adapt to this change, it will slowly degrade.
This means that as programming languages are constantly updated, LLM-based coding tools will also degrade. This is one of the reasons why you don't have to rely too heavily on such a fragile tool.
The cost of constantly retraining these models is high, and sooner or later someone will give up on these inefficient methods.
No ML model yet can reliably and continuously adapt to changing input distributions without causing severe disruption or performance loss to the previous encoding task.
And this is one of the areas where biological neural networks are good at. Due to the strong generalization ability of biological neural networks, learning different tasks can further improve the performance of the system, because the knowledge gained from one task helps to improve the entire learning process itself, which is called "meta-learning".
How serious is the problem of "task pollution"? Let’s take a look at the content of the paper.
Models and data sets
There are 12 models used in the experiment (as shown in Table 1), 5 of which are proprietary Of the GPT-3 series models, 7 are open models with free access to weights.
Datasets are divided into two categories: published before or after January 1, 2021 Data set, researchers use this partitioning method to analyze the zero-sample or few-sample performance difference between the old data set and the new data set, and use the same partitioning method for all LLMs. Table 1 lists the creation time of each model training data, and Table 2 lists the publication date of each dataset.
The consideration behind the above approach is that zero-shot and few-shot evaluations involve the model making predictions about tasks that it has never seen or only seen a few times during training. The key premise is that the model has no prior exposure to the specific task to be completed. , thereby ensuring a fair assessment of their learning abilities. However, tainted models can give the illusion of competence that they have not been exposed to or have only been exposed to a few times because they have been trained on task examples during pre-training. In a chronological data set, it will be relatively easier to detect such inconsistencies, as any overlaps or anomalies will be obvious.
Measurement methods
The researchers used four methods to measure "task pollution":
- Training data inspection: Search the training data for task training examples.
- Task example extraction: Extract task examples from existing models. Only instruction-tuned models can be extracted. This analysis can also be used for training data or test data extraction. Note that in order to detect task contamination, the extracted task examples do not have to exactly match existing training data examples. Any example that demonstrates a task demonstrates the possible contamination of zero-shot learning and few-shot learning.
- Member Reasoning: This method only applies to build tasks. Checks that the model-generated content for the input instance is exactly the same as the original dataset. If it matches exactly, we can infer that it is a member of the LLM training data. This differs from task example extraction in that the generated output is checked for an exact match. Exact matches on the open-ended generation task strongly suggest that the model saw these examples during training, unless the model is "psychic" and knows the exact wording used in the data. (Note, this can only be used for build tasks.)
- Time series analysis: For a model set where training data was collected during a known time frame, measure its performance on a dataset with a known release date, and use Temporal evidence checks for contamination evidence.
The first three methods have high precision, but low recall rate. If you can find the data in the task's training data, you can be sure that the model has seen the example. However, due to changes in data formats, changes in keywords used to define tasks, and the size of data sets, finding no evidence of contamination using the first three methods does not prove the absence of contamination.
The fourth method, the recall rate of chronological analysis is high, but the precision is low. If performance is high due to task contamination, then chronological analysis has a good chance of spotting it. But other factors may also cause performance to improve over time and therefore be less accurate.
Therefore, the researchers used all four methods to detect task contamination and found strong evidence of task contamination in certain model and dataset combinations.
They first performed timing analysis on all tested models and datasets as it was most likely to find possible contamination; then used training data inspection and task example extraction to find task contamination Further evidence; we next observe the performance of LLM on a pollution-free task, and finally conduct additional analysis using membership inference attacks.
The key conclusions are as follows:
1. The researcher created a data set for each model before its training data was crawled on the Internet. and then analyzed the data set created. It was found that the odds of performing above most baselines were significantly higher for datasets created before collecting LLM training data (Figure 1).
#2. The researcher conducted training data inspection and task example extraction to find possible task contamination. It was found that for classification tasks where task contamination is unlikely, models rarely achieve statistically significant improvements over simple majority baselines across a range of tasks, whether zero- or few-shot (Figure 2).
The researchers also examined the changes in the average performance of the GPT-3 series and open LLM over time, as shown in Figure 3 :
3. As a case study, the researcher also tried to perform semantic parsing tasks on all models in the analysis. Inference attack, found a strong correlation (R=.88) between the number of extracted instances and the accuracy of the model in the final task (Figure 6). This strongly proves that the improvement in zero-shot performance in this task is due to task contamination.
4. The researchers also carefully studied the GPT-3 series models and found that training examples can be extracted from the GPT-3 model, and in each version from davinci to GPT-3.5-turbo, the training examples that can be extracted The number is increasing, which is closely related to the improvement of the zero-sample performance of the GPT-3 model on this task (Figure 2). This strongly proves that the performance improvement of GPT-3 models from davinci to GPT-3.5-turbo on these tasks is due to task contamination.
The above is the detailed content of A new interpretation of the declining intelligence level of GPT-4. For more information, please follow other related articles on the PHP Chinese website!
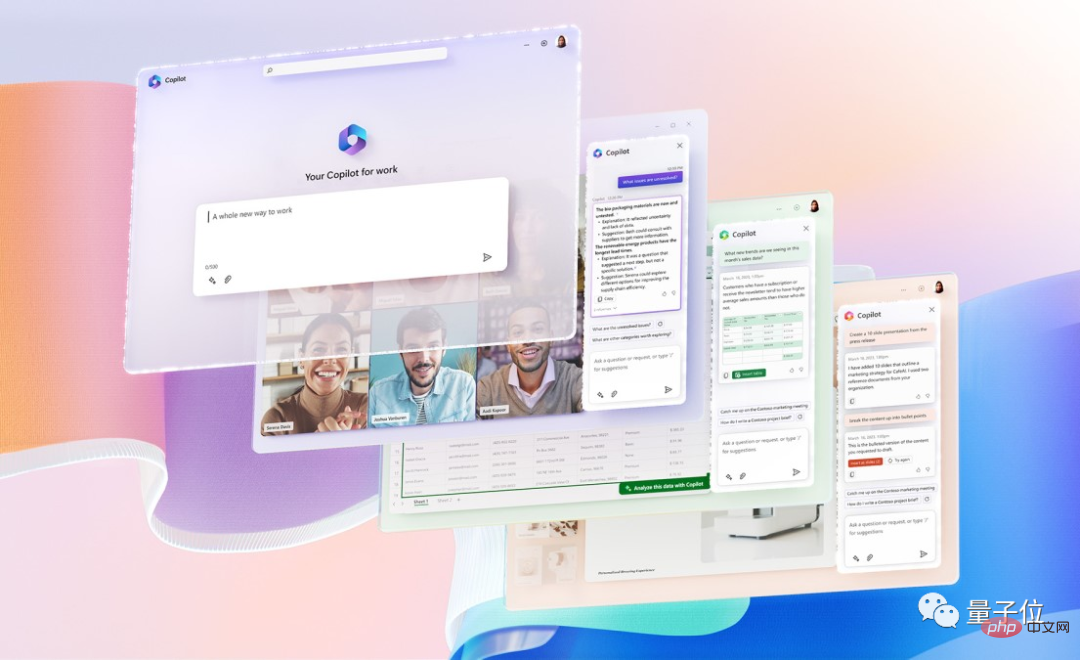
一觉醒来,工作的方式被彻底改变。微软把AI神器GPT-4全面接入Office,这下ChatPPT、ChatWord、ChatExcel一家整整齐齐。CEO纳德拉在发布会上直接放话:今天,进入人机交互的新时代,重新发明生产力。新功能名叫Microsoft 365 Copilot(副驾驶),与改变了程序员的代码助手GitHub Copilot成为一个系列,继续改变更多人。现在AI不光能自动做PPT,而且能根据Word文档的内容一键做出精美排版。甚至连上台时对着每一页PPT应该讲什么话,都给一起安排

集成GPT-4的Github Copilot X还在小范围内测中,而集成GPT-4的Cursor已公开发行。Cursor是一个集成GPT-4的IDE,可以用自然语言编写代码,让编写代码和聊天一样简单。 GPT-4和GPT-3.5在处理和编写代码的能力上差别还是很大的。官网的一份测试报告。前两个是GPT-4,一个采用文本输入,一个采用图像输入;第三个是GPT3.5,可以看出GPT-4的代码能力相较于GPT-3.5有较大能力的提升。集成GPT-4的Github Copilot X还在小范围内测中,而
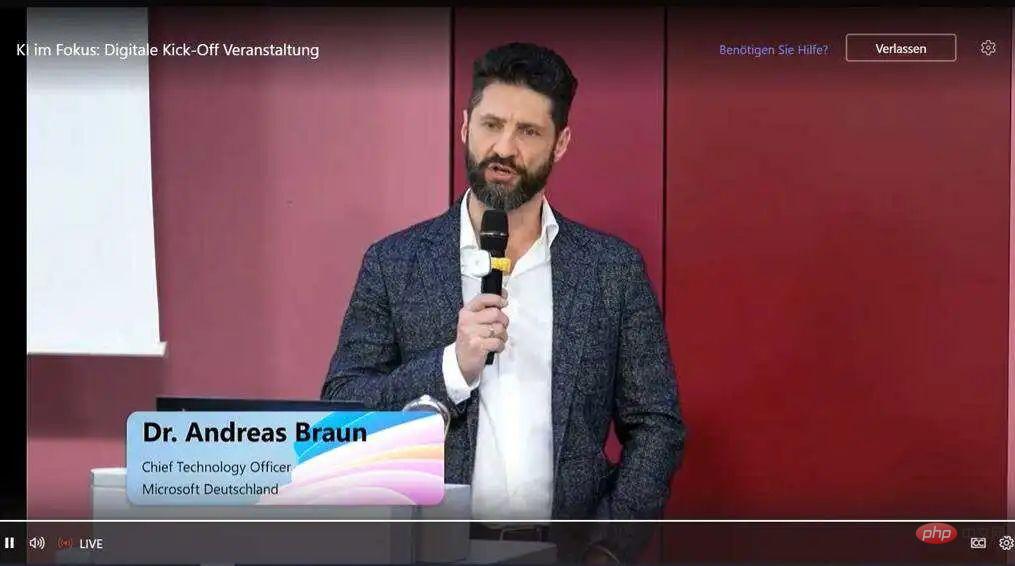
作者 | 云昭3月9日,微软德国CTO Andreas Braun在AI kickoff会议上带来了一个期待已久的消息:“我们将于下周推出GPT-4,届时我们将推出多模式模式,提供完全不同的可能性——例如视频。”言语之中,他将大型语言模型(LLM)比作“游戏改变者”,因为他们教机器理解自然语言,然后机器以统计的方式理解以前只能由人类阅读和理解的东西。与此同时,这项技术已经发展到“适用于所有语言”:你可以用德语提问,也可以用意大利语回答。借助多模态,微软(-OpenAI)将“使模型变得全面”。那
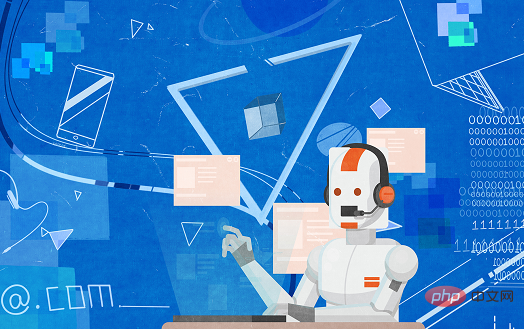
近段时间,人工智能聊天机器人ChatGPT刷爆网络,网友们争先恐后去领略它的超高情商和巨大威力。参加高考、修改代码、构思小说……它在广大网友的“鞭策”下不断突破自我,甚至可以用一整段程序,为你拼接出一只小狗。而这些技能只是基于GPT-3.5开发而来,在3月15日,AI世界再次更新,最新版本的GPT-4也被OpenAI发布了出来。与之前相比,GPT-4不仅展现了更加强大的语言理解能力,还能够处理图像内容,在考试中的得分甚至能超越90%的人类。那么,如此“逆天”的GPT-4还具有哪些能力?它又是如何
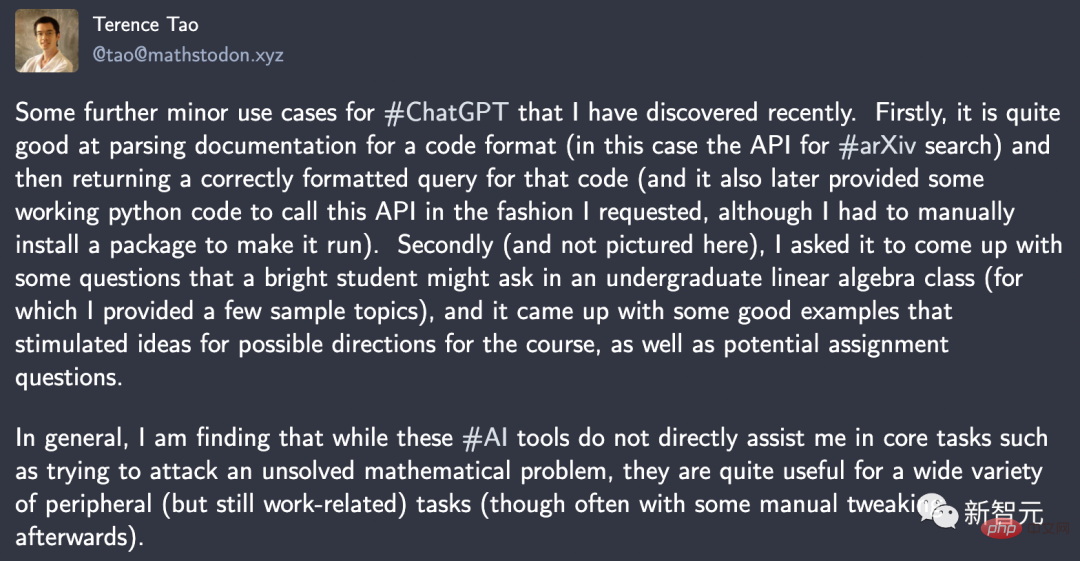
当红炸子鸡ChatGPT,也成为数学天才陶哲轩的研究工具了。近日,他在网上称自己发现了一些ChatGPT的小用例。首先,它很擅长解析代码格式的文档(在这种情况下是#arXiv搜索的API),然后返回一个正确格式的代码查询(后来它还提供了一些工作的python代码,以我要求的方式调用这个API,尽管我不得不手动安装一个包来使它运行)。其次,我让它想出一些,聪明的学生在本科线性代数课上可能会问的问题(为此我提供了一些样本题目),它给出了一些很好的例子,让我对课程可能方向,以及潜在的作业问题有所启发。
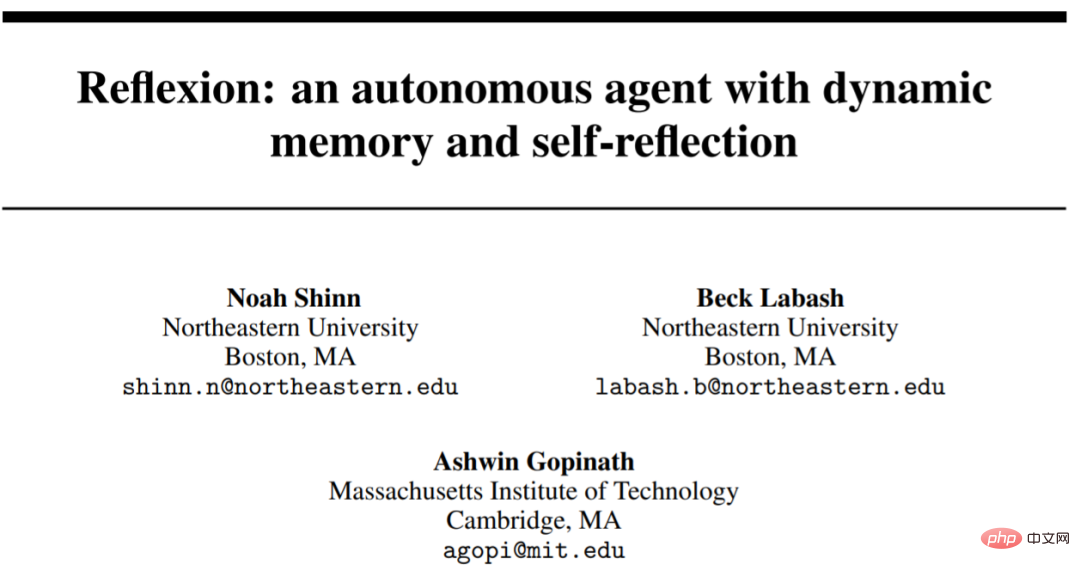
GPT-4 的思考方式,越来越像人了。 人类在做错事时,会反思自己的行为,避免再次出错,如果让 GPT-4 这类大型语言模型也具备反思能力,性能不知道要提高多少了。众所周知,大型语言模型 (LLM) 在各种任务上已经表现出前所未有的性能。然而,这些 SOTA 方法通常需要对已定义的状态空间进行模型微调、策略优化等操作。由于缺乏高质量的训练数据、定义良好的状态空间,优化模型实现起来还是比较难的。此外,模型还不具备人类决策过程所固有的某些品质,特别是从错误中学习的能力。不过现在好了,在最近的一篇论文
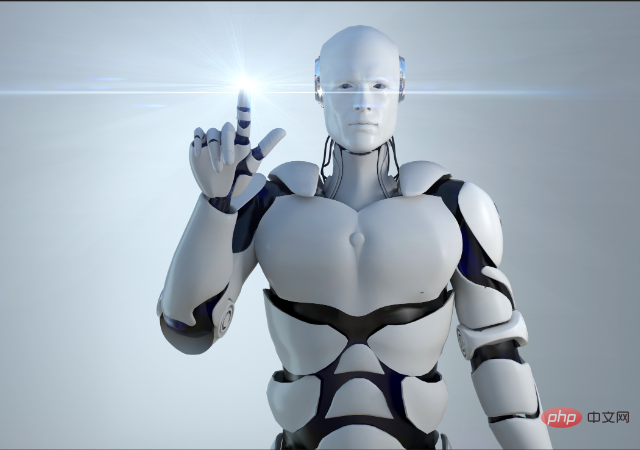
人工智能在过去几十年里发展势头强劲,像GPT-4这样的大型语言模型引起了用户的更多兴趣,他们想知道GPT-4如何支持数字化转型。根据行业媒体的预测,到2024年,GPT-4所基于的ChatGPT深度学习堆栈将产生10亿美元的收入。GPT-4的普及是由于人工智能技术的力量,以及高用户可访问性和广泛的通用性。科技行业的许多不同领域都可以利用GPT-4来自动化和个性化许多任务,使企业员工能够专注于更复杂的任务。以下是GPT-4在几个不同领域促进数字化转型的一些例子。1、个性化员工培训像GPT-4这样的
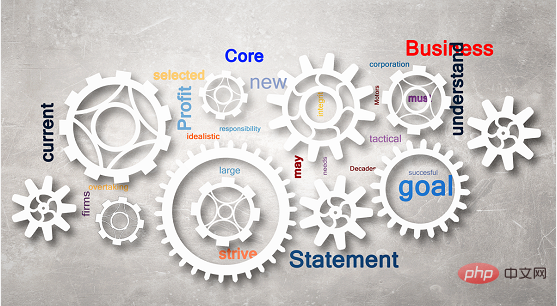
3 月 15 日消息,今天 OpenAI 发布了全新的 GPT-4 大型语言模型,随后微软官方宣布,Bing Chat 此前已经升级使用 OpenAI 的 GPT-4 技术。微软公司副总裁兼消费者首席营销官 Yusuf Mehdi 确认 Bing Chat 聊天机器人 AI 已经在 GPT-4 上运行,ChatGPT 基于最新版本 GPT-4,由 OpenAI 开发 。微软 Bing 博客网站上的一篇帖子进一步证实了这一消息。微软表示,如果用户在过去五周内的任何时间使用过新的 Bing 预览版,


Hot AI Tools

Undresser.AI Undress
AI-powered app for creating realistic nude photos

AI Clothes Remover
Online AI tool for removing clothes from photos.

Undress AI Tool
Undress images for free

Clothoff.io
AI clothes remover

AI Hentai Generator
Generate AI Hentai for free.

Hot Article

Hot Tools
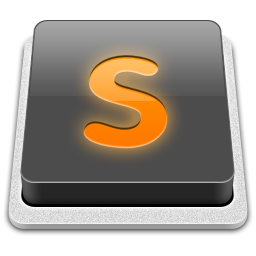
SublimeText3 Mac version
God-level code editing software (SublimeText3)

SAP NetWeaver Server Adapter for Eclipse
Integrate Eclipse with SAP NetWeaver application server.

Atom editor mac version download
The most popular open source editor

mPDF
mPDF is a PHP library that can generate PDF files from UTF-8 encoded HTML. The original author, Ian Back, wrote mPDF to output PDF files "on the fly" from his website and handle different languages. It is slower than original scripts like HTML2FPDF and produces larger files when using Unicode fonts, but supports CSS styles etc. and has a lot of enhancements. Supports almost all languages, including RTL (Arabic and Hebrew) and CJK (Chinese, Japanese and Korean). Supports nested block-level elements (such as P, DIV),

SecLists
SecLists is the ultimate security tester's companion. It is a collection of various types of lists that are frequently used during security assessments, all in one place. SecLists helps make security testing more efficient and productive by conveniently providing all the lists a security tester might need. List types include usernames, passwords, URLs, fuzzing payloads, sensitive data patterns, web shells, and more. The tester can simply pull this repository onto a new test machine and he will have access to every type of list he needs.
