Haze degree estimation problem in image defogging technology
The problem of haze degree estimation in image dehazing technology
Introduction
With the acceleration of urbanization, the problem of air pollution is becoming increasingly serious, and haze has become a A common phenomenon in urban life. Among them, haze brings challenges to visual tasks such as image acquisition and image processing. In order to improve the image quality degradation problem caused by haze, researchers have proposed various image defogging algorithms. Among these algorithms, accurately estimating the degree of haze in the image is crucial to improving the dehazing effect. This article will discuss the haze degree estimation problem in image dehazing technology and provide specific code examples.
1. The importance of haze degree estimation
Haze degree estimation is an important part of the image dehazing task. By accurately estimating the degree of haze in an image, it can help the dehazing algorithm better understand the mixed haze and scene information in the image, thereby achieving a more accurate dehazing effect. In practical applications, it is often necessary to select appropriate dehazing algorithms and parameters based on the haze level of the image, thereby improving the effect of image processing.
2. Commonly used haze degree estimation methods
- The haze degree estimation method based on single-scale dark channel prior:
The single-scale dark channel prior is based on analysis Dark channel in outdoor images to estimate the extent of haze. This method assumes that the minimum value of the R, G, and B channels of a certain pixel in the image (non-light source point) corresponds to one of the brightest pixels in the image, and estimates the haze level through the depth information of the brightest pixel. degree. The specific calculation formula is:
A = min(R, G, B)
t(x) = 1 - w * min(R/G, R/B, R/A)
where, R , G, and B respectively represent the intensity values of the red, green, and blue channels at the pixel point (x, y), A represents the depth value of the brightest pixel in the image, and w is a fixed weight. - Haze degree estimation method based on image contrast:
This method estimates the degree of haze based on the contrast of the image. Typically, haze images have low contrast, while non-haze images have high contrast. Therefore, the degree of haze can be estimated by comparing the contrast difference between the original image and the dehazed image. A simple calculation method is to calculate the grayscale histogram of the image and calculate the mean square error of the histogram.
3. Code Example
The following is a code example of haze level estimation based on single-scale dark channel prior using Python language:
import cv2 import numpy as np def estimate_haze_level(image): # 计算每个像素点的最小通道值 min_channel = np.min(image, axis=2) # 计算最亮像素点的深度值 A = np.max(min_channel) # 根据公式计算雾霾程度 haze_level = 1 - 0.95 * (min_channel / A) return haze_level # 读取原始图像 image = cv2.imread("input.jpg") # 估计雾霾程度 haze_level = estimate_haze_level(image) # 输出雾霾程度 print("Haze level:", haze_level)
4. Summary
The haze degree estimation problem in image dehazing technology is crucial to improving the dehazing effect. This article introduces the importance of haze level estimation and provides a code example for haze level estimation based on a single-scale dark channel prior. By rationally using image dehazing algorithms and haze degree estimation methods, the problem of image quality degradation caused by haze can be effectively improved and the accuracy and effect of image processing can be improved. As research continues to deepen, it is believed that image defogging technology will be more widely used in the future.
The above is the detailed content of Haze degree estimation problem in image defogging technology. For more information, please follow other related articles on the PHP Chinese website!
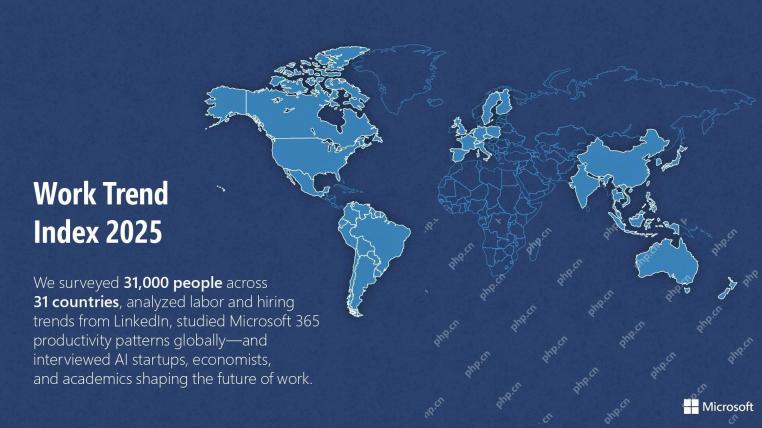
The burgeoning capacity crisis in the workplace, exacerbated by the rapid integration of AI, demands a strategic shift beyond incremental adjustments. This is underscored by the WTI's findings: 68% of employees struggle with workload, leading to bur
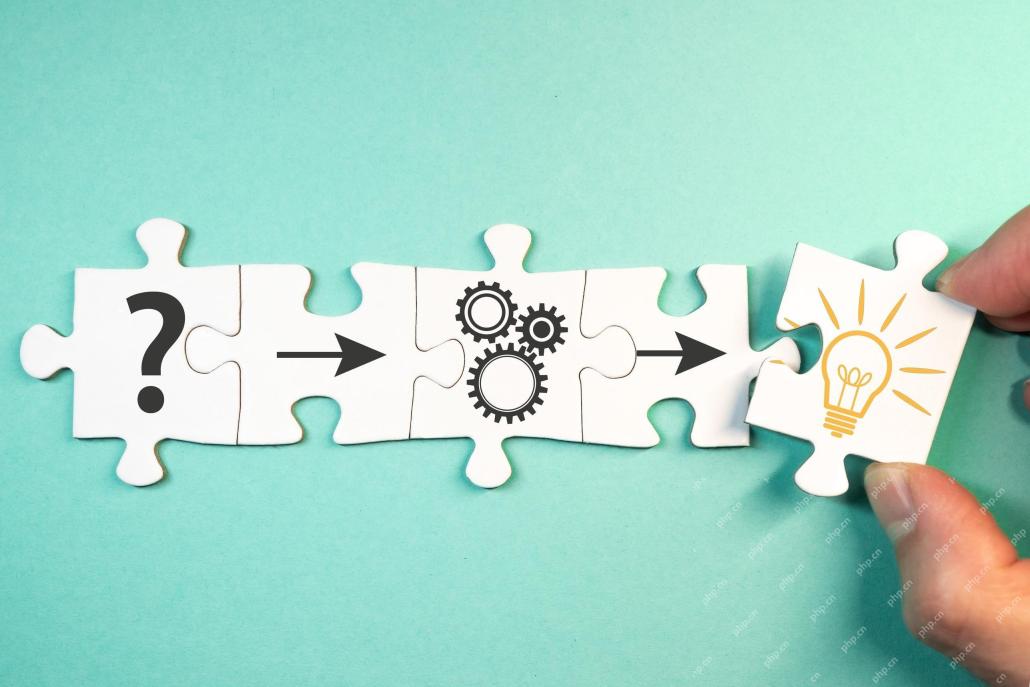
John Searle's Chinese Room Argument: A Challenge to AI Understanding Searle's thought experiment directly questions whether artificial intelligence can genuinely comprehend language or possess true consciousness. Imagine a person, ignorant of Chines
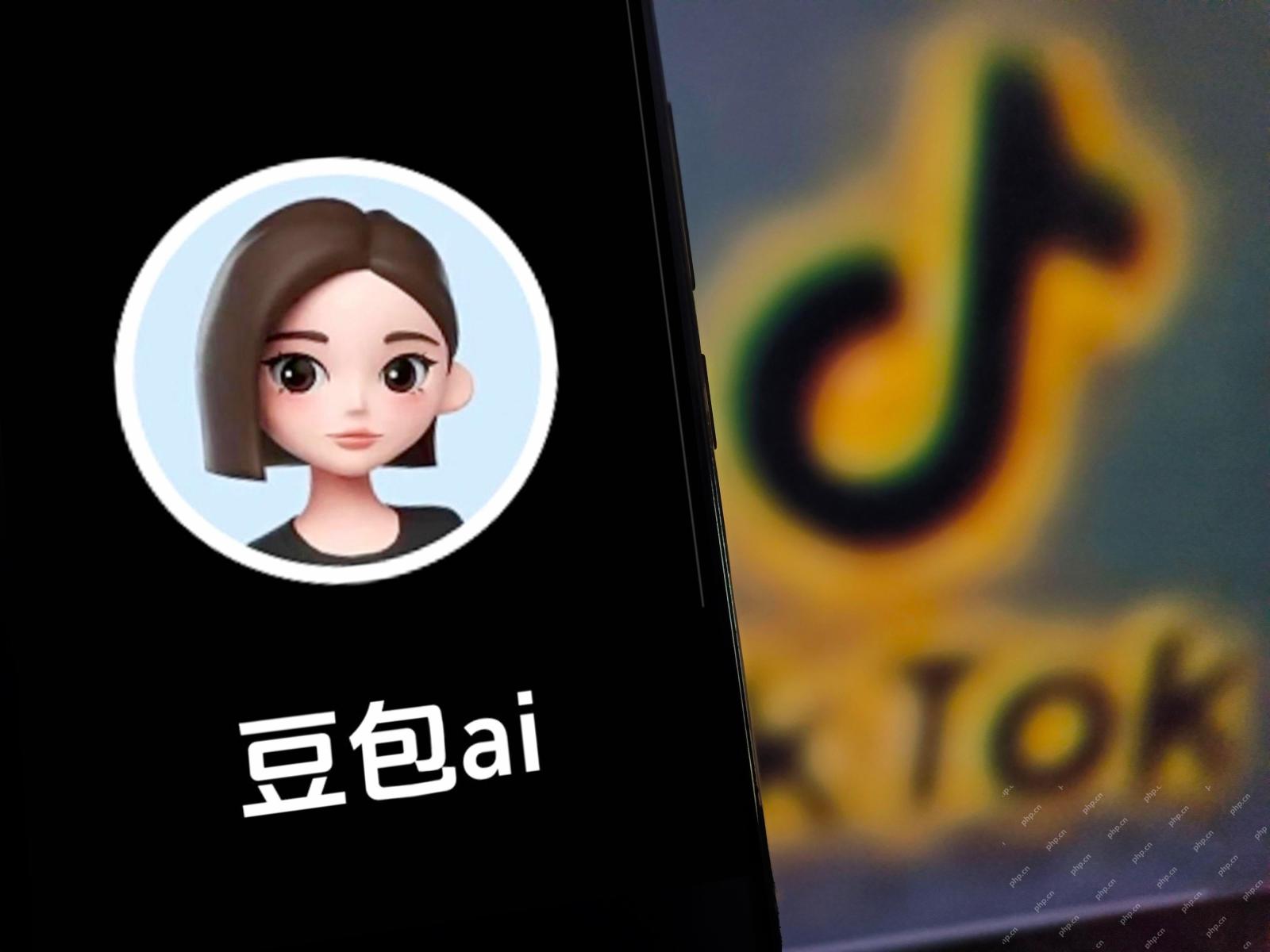
China's tech giants are charting a different course in AI development compared to their Western counterparts. Instead of focusing solely on technical benchmarks and API integrations, they're prioritizing "screen-aware" AI assistants – AI t
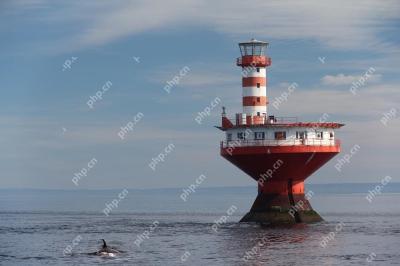
MCP: Empower AI systems to access external tools Model Context Protocol (MCP) enables AI applications to interact with external tools and data sources through standardized interfaces. Developed by Anthropic and supported by major AI providers, MCP allows language models and agents to discover available tools and call them with appropriate parameters. However, there are some challenges in implementing MCP servers, including environmental conflicts, security vulnerabilities, and inconsistent cross-platform behavior. Forbes article "Anthropic's model context protocol is a big step in the development of AI agents" Author: Janakiram MSVDocker solves these problems through containerization. Doc built on Docker Hub infrastructure
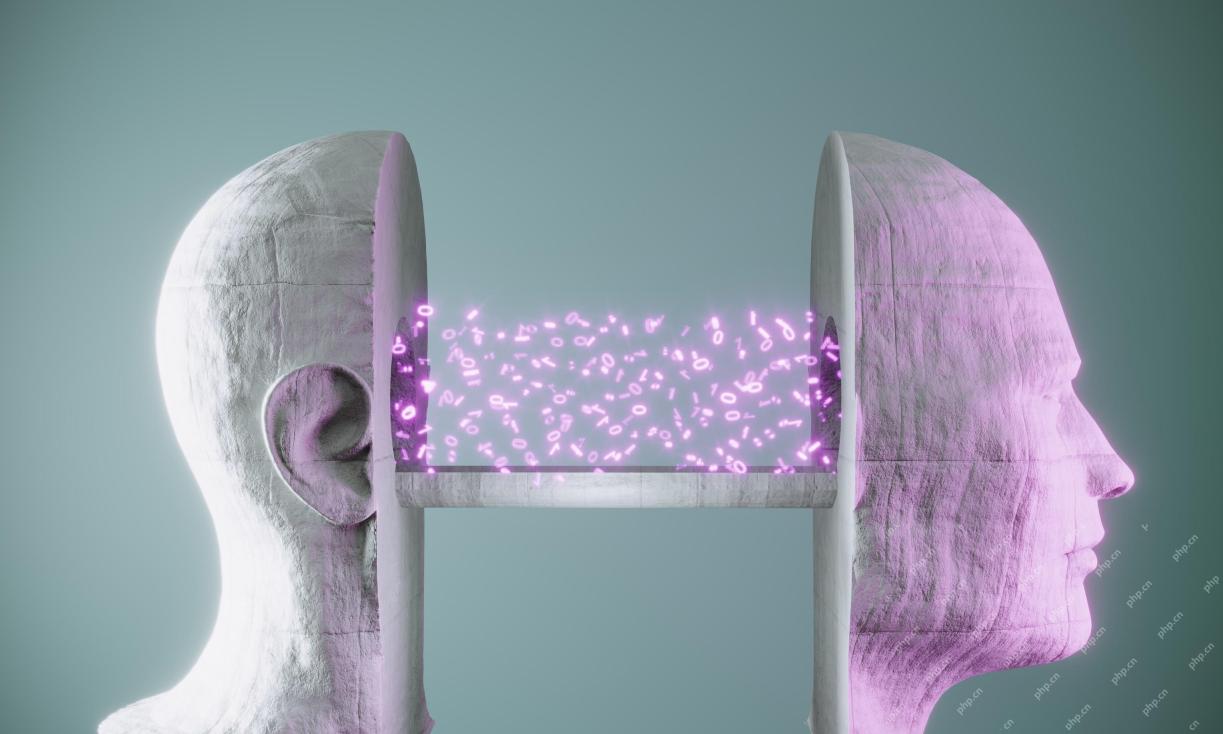
Six strategies employed by visionary entrepreneurs who leveraged cutting-edge technology and shrewd business acumen to create highly profitable, scalable companies while maintaining control. This guide is for aspiring entrepreneurs aiming to build a
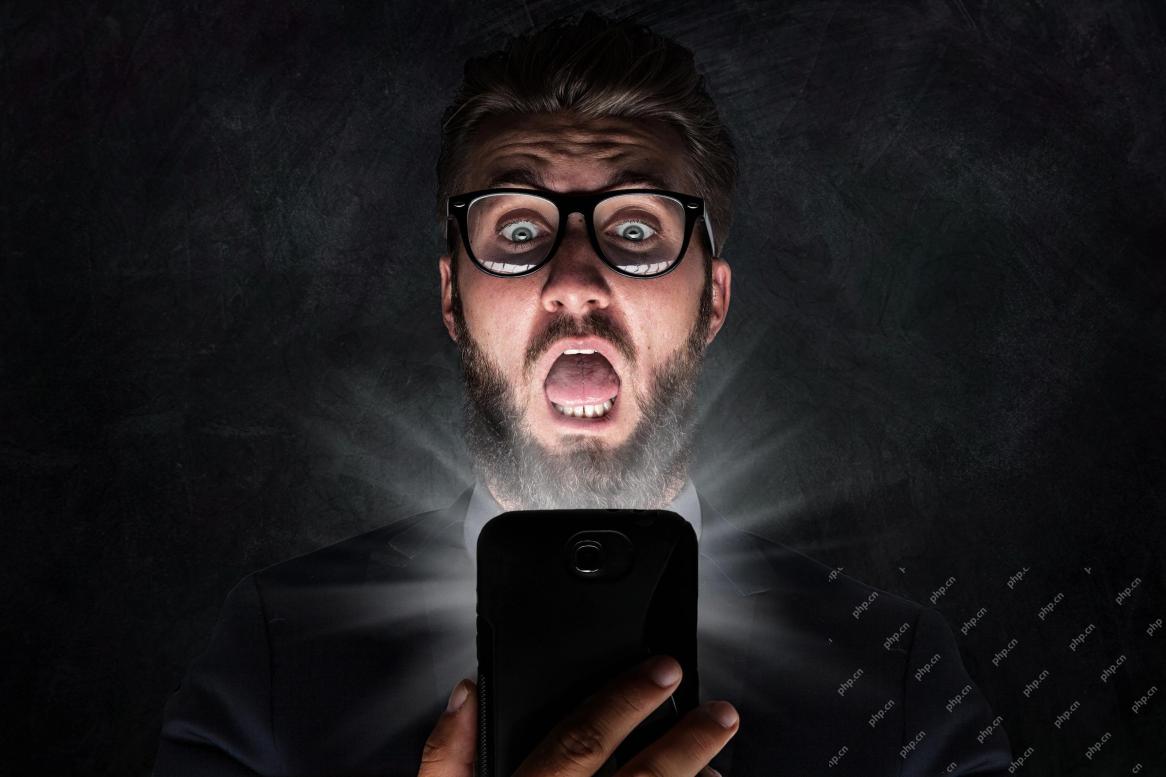
Google Photos' New Ultra HDR Tool: A Game Changer for Image Enhancement Google Photos has introduced a powerful Ultra HDR conversion tool, transforming standard photos into vibrant, high-dynamic-range images. This enhancement benefits photographers a
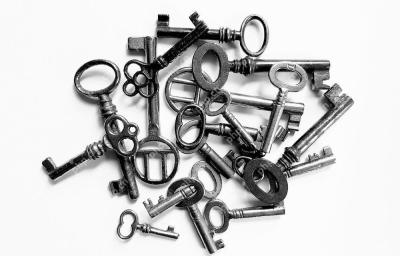
Technical Architecture Solves Emerging Authentication Challenges The Agentic Identity Hub tackles a problem many organizations only discover after beginning AI agent implementation that traditional authentication methods aren’t designed for machine-
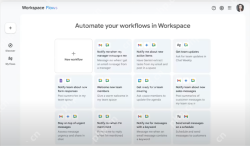
(Note: Google is an advisory client of my firm, Moor Insights & Strategy.) AI: From Experiment to Enterprise Foundation Google Cloud Next 2025 showcased AI's evolution from experimental feature to a core component of enterprise technology, stream


Hot AI Tools

Undresser.AI Undress
AI-powered app for creating realistic nude photos

AI Clothes Remover
Online AI tool for removing clothes from photos.

Undress AI Tool
Undress images for free

Clothoff.io
AI clothes remover

Video Face Swap
Swap faces in any video effortlessly with our completely free AI face swap tool!

Hot Article

Hot Tools

PhpStorm Mac version
The latest (2018.2.1) professional PHP integrated development tool

Notepad++7.3.1
Easy-to-use and free code editor

SublimeText3 Linux new version
SublimeText3 Linux latest version

mPDF
mPDF is a PHP library that can generate PDF files from UTF-8 encoded HTML. The original author, Ian Back, wrote mPDF to output PDF files "on the fly" from his website and handle different languages. It is slower than original scripts like HTML2FPDF and produces larger files when using Unicode fonts, but supports CSS styles etc. and has a lot of enhancements. Supports almost all languages, including RTL (Arabic and Hebrew) and CJK (Chinese, Japanese and Korean). Supports nested block-level elements (such as P, DIV),

SAP NetWeaver Server Adapter for Eclipse
Integrate Eclipse with SAP NetWeaver application server.
