Liveness detection issues in face recognition technology
The living body detection problem in face recognition technology requires specific code examples
In recent years, with the rapid development of face recognition technology, face recognition has been widely used It is used in security monitoring, face unlocking, financial transactions and other fields. However, at the same time, counterfeit attack methods such as photos and artificial three-dimensional models are also emerging, which poses certain challenges to the accuracy and security of face recognition. In order to improve the credibility of the face recognition system, live detection has become a necessary link.
Liveness detection is to determine whether a face is a real living body rather than a fake photo or model. Traditional living body detection methods mainly rely on static texture information or two-dimensional features to make judgments, and their accuracy is low. With the rise of deep learning, living body detection methods based on deep learning have gradually become mainstream and have made significant progress.
The following will introduce a living body detection method based on deep learning and give relevant code examples.
First, we need to build a face recognition model. You can use the open source deep learning framework TensorFlow and introduce the face recognition model library facenet based on it. First, install TensorFlow:
pip install tensorflow
Next, we need to download and install the facenet library. Run the following command in the command line:
git clone https://github.com/davidsandberg/facenet.git cd facenet pip install -r requirements.txt
After the download is complete, we can start building the face recognition model. Through the following command, we can download the trained facenet model:
python src/download_and_extract_model.py --model_dir models
Then, we can use the following code to load the model and perform face recognition:
import tensorflow as tf import numpy as np import cv2 from facenet.src.align import detect_face from facenet.src import facenet # 加载facenet模型 sess = tf.Session() facenet.load_model("models") # 获取输入和输出张量 images_placeholder = sess.graph.get_tensor_by_name("input:0") embeddings = sess.graph.get_tensor_by_name("embeddings:0") # 加载人脸检测模型 pnet, rnet, onet = detect_face.create_mtcnn(sess, "facenet/src/align") # 检测人脸及进行活体检测 def detect_faces(image): bounding_boxes, _ = detect_face.detect_face(image, minsize=20, pnet=pnet, rnet=rnet, onet=onet, threshold=[0.6, 0.7, 0.7], factor=0.709) faces = [] for bb in bounding_boxes: x1, y1, x2, y2 = int(bb[0]), int(bb[1]), int(bb[2]), int(bb[3]) face = cv2.resize(image[y1:y2, x1:x2], (160, 160)) face = facenet.prewhiten(face) face = cv2.cvtColor(face, cv2.COLOR_BGR2RGB) faces.append(face) return faces # 加载测试图片 image = cv2.imread("test.jpg") faces = detect_faces(image) # 进行活体检测 for face in faces: face = np.expand_dims(face, axis=0) feed_dict = {images_placeholder: face} face_embeddings = sess.run(embeddings, feed_dict=feed_dict) # 根据face_embeddings进行活体检测算法
Through the above code example, we can Complete face recognition and live body detection based on the facenet model. Of course, in practical applications, we also need to further improve and optimize the living body detection algorithm according to specific scenarios and needs to improve accuracy and reliability.
In short, liveness detection is an indispensable part of face recognition technology and can effectively prevent forgery attacks. By combining deep learning and professional face recognition models, we can quickly and accurately conduct live body detection and apply it in various fields to ensure the safety and credibility of the face recognition system.
The above is the detailed content of Liveness detection issues in face recognition technology. For more information, please follow other related articles on the PHP Chinese website!
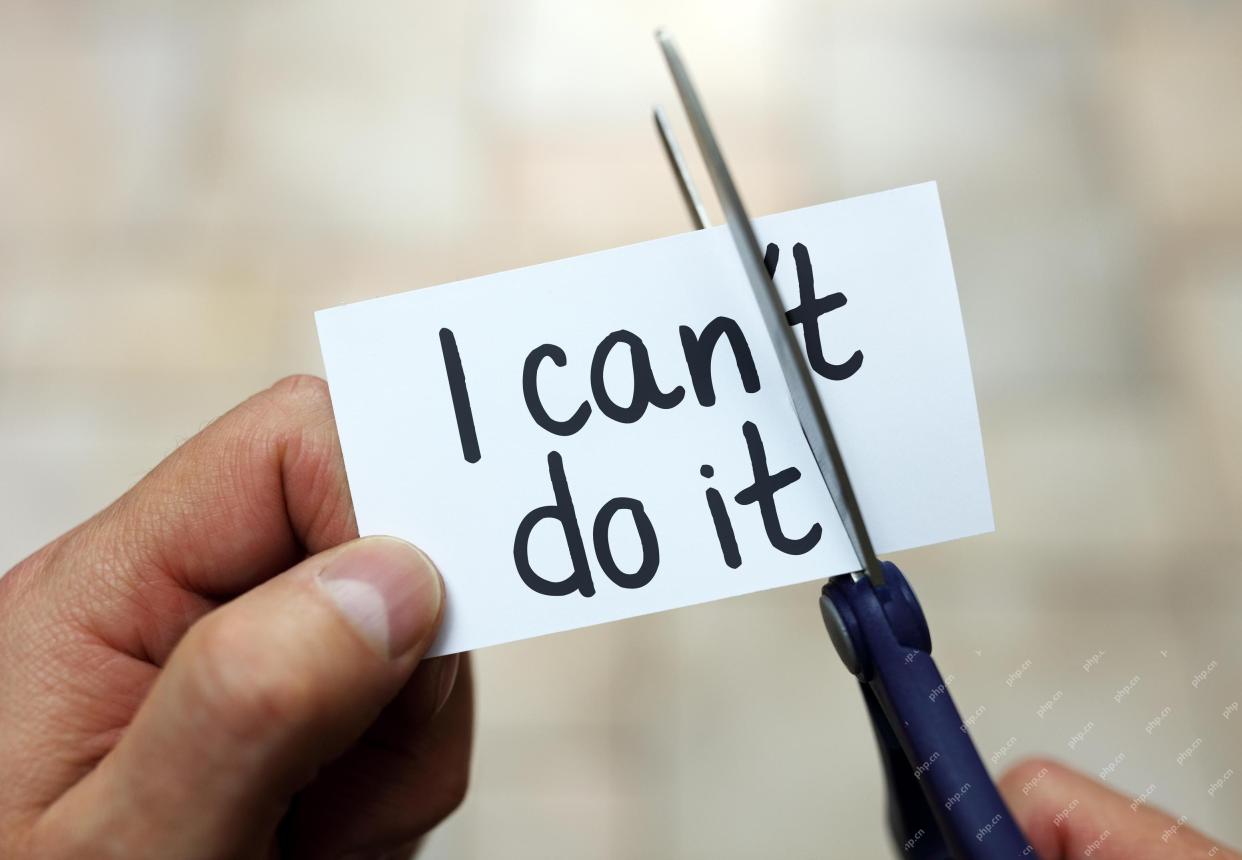
This article explores the growing concern of "AI agency decay"—the gradual decline in our ability to think and decide independently. This is especially crucial for business leaders navigating the increasingly automated world while retainin
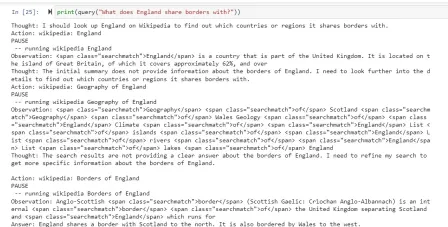
Ever wondered how AI agents like Siri and Alexa work? These intelligent systems are becoming more important in our daily lives. This article introduces the ReAct pattern, a method that enhances AI agents by combining reasoning an
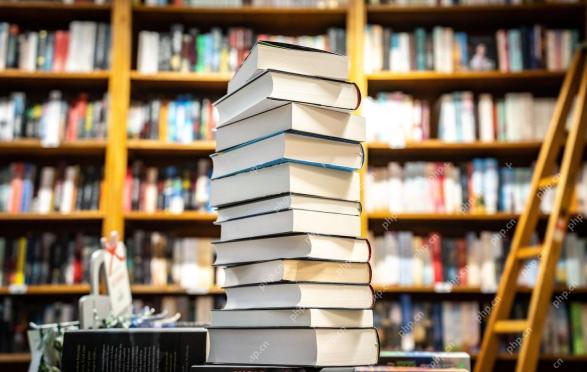
"I think AI tools are changing the learning opportunities for college students. We believe in developing students in core courses, but more and more people also want to get a perspective of computational and statistical thinking," said University of Chicago President Paul Alivisatos in an interview with Deloitte Nitin Mittal at the Davos Forum in January. He believes that people will have to become creators and co-creators of AI, which means that learning and other aspects need to adapt to some major changes. Digital intelligence and critical thinking Professor Alexa Joubin of George Washington University described artificial intelligence as a “heuristic tool” in the humanities and explores how it changes
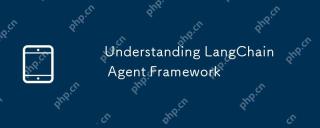
LangChain is a powerful toolkit for building sophisticated AI applications. Its agent architecture is particularly noteworthy, allowing developers to create intelligent systems capable of independent reasoning, decision-making, and action. This expl
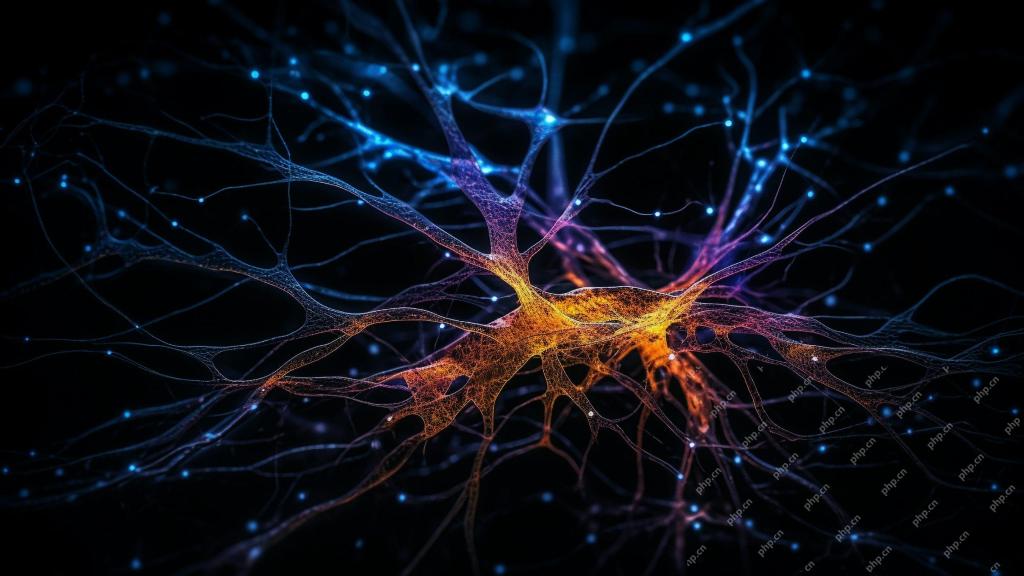
Radial Basis Function Neural Networks (RBFNNs): A Comprehensive Guide Radial Basis Function Neural Networks (RBFNNs) are a powerful type of neural network architecture that leverages radial basis functions for activation. Their unique structure make
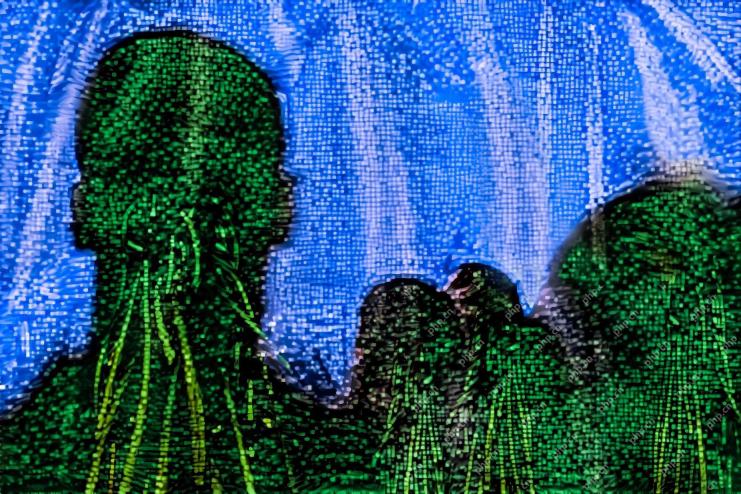
Brain-computer interfaces (BCIs) directly link the brain to external devices, translating brain impulses into actions without physical movement. This technology utilizes implanted sensors to capture brain signals, converting them into digital comman
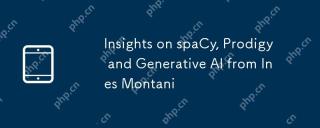
This "Leading with Data" episode features Ines Montani, co-founder and CEO of Explosion AI, and co-developer of spaCy and Prodigy. Ines offers expert insights into the evolution of these tools, Explosion's unique business model, and the tr
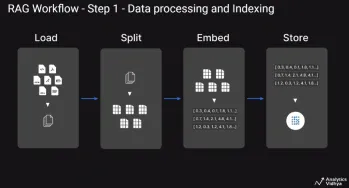
This article explores Retrieval Augmented Generation (RAG) systems and how AI agents can enhance their capabilities. Traditional RAG systems, while useful for leveraging custom enterprise data, suffer from limitations such as a lack of real-time dat


Hot AI Tools

Undresser.AI Undress
AI-powered app for creating realistic nude photos

AI Clothes Remover
Online AI tool for removing clothes from photos.

Undress AI Tool
Undress images for free

Clothoff.io
AI clothes remover

Video Face Swap
Swap faces in any video effortlessly with our completely free AI face swap tool!

Hot Article

Hot Tools

MantisBT
Mantis is an easy-to-deploy web-based defect tracking tool designed to aid in product defect tracking. It requires PHP, MySQL and a web server. Check out our demo and hosting services.

PhpStorm Mac version
The latest (2018.2.1) professional PHP integrated development tool

MinGW - Minimalist GNU for Windows
This project is in the process of being migrated to osdn.net/projects/mingw, you can continue to follow us there. MinGW: A native Windows port of the GNU Compiler Collection (GCC), freely distributable import libraries and header files for building native Windows applications; includes extensions to the MSVC runtime to support C99 functionality. All MinGW software can run on 64-bit Windows platforms.

mPDF
mPDF is a PHP library that can generate PDF files from UTF-8 encoded HTML. The original author, Ian Back, wrote mPDF to output PDF files "on the fly" from his website and handle different languages. It is slower than original scripts like HTML2FPDF and produces larger files when using Unicode fonts, but supports CSS styles etc. and has a lot of enhancements. Supports almost all languages, including RTL (Arabic and Hebrew) and CJK (Chinese, Japanese and Korean). Supports nested block-level elements (such as P, DIV),
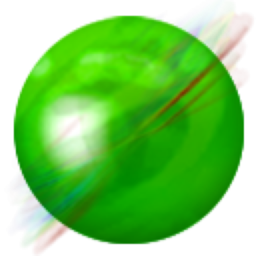
ZendStudio 13.5.1 Mac
Powerful PHP integrated development environment