How to write the KNN algorithm in Python?
KNN (K-Nearest Neighbors, K nearest neighbor algorithm) is a simple and commonly used classification algorithm. The idea is to classify test samples into the nearest K neighbors by measuring the distance between different samples. This article will introduce how to write and implement the KNN algorithm using Python and provide specific code examples.
First, we need to prepare some data. Suppose we have a two-dimensional data set, and each sample has two features. We divided the dataset into two categories and plotted them on a two-dimensional plane. The code is as follows:
import numpy as np import matplotlib.pyplot as plt # 生成随机数据 np.random.seed(0) X1 = np.random.randn(100, 2) + np.array([0, 2]) X2 = np.random.randn(100, 2) + np.array([2, 0]) X = np.vstack((X1, X2)) y = np.hstack((np.zeros(100), np.ones(100))) # 绘制数据集 plt.scatter(X[:, 0], X[:, 1], c=y) plt.show()
Next, we need to write the implementation code of the KNN algorithm. First, we define a function to calculate the Euclidean Distance between two samples. The code is as follows:
def euclidean_distance(x1, x2): return np.sqrt(np.sum((x1 - x2)**2))
Then, we write a function to predict the category of a test sample. This function first calculates the distance between the test sample and all training samples, then selects the K closest samples, votes based on the categories of these K neighbors, and finally returns the category with the most votes as the prediction result. The code is as follows:
def knn_predict(X_train, y_train, x_test, k): distances = [euclidean_distance(x_test, x) for x in X_train] k_indices = np.argsort(distances)[:k] k_nearest_labels = [y_train[i] for i in k_indices] return np.argmax(np.bincount(k_nearest_labels))
Finally, we divide the data set into a training set and a test set, and use the KNN algorithm for prediction. The code is as follows:
from sklearn.model_selection import train_test_split # 划分训练集和测试集 X_train, X_test, y_train, y_test = train_test_split(X, y, test_size=0.2) # 对每个测试样本进行预测 predictions = [knn_predict(X_train, y_train, x_test, k=3) for x_test in X_test] # 计算准确率 accuracy = np.mean(predictions == y_test) print("Accuracy:", accuracy)
Through the above code examples, we have completed the writing of the KNN algorithm. It can be seen that using Python to implement the KNN algorithm is relatively simple and has a small amount of code. In practical applications, we can adjust the K value according to specific problems to achieve the best classification effect.
To summarize, this article introduces how to use Python to write the KNN algorithm, including steps such as data preparation, Euclidean distance calculation, algorithm implementation, and accuracy calculation. I hope this article can help readers understand and apply the KNN algorithm.
The above is the detailed content of How to write KNN algorithm in Python?. For more information, please follow other related articles on the PHP Chinese website!
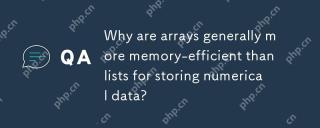
Arraysaregenerallymorememory-efficientthanlistsforstoringnumericaldataduetotheirfixed-sizenatureanddirectmemoryaccess.1)Arraysstoreelementsinacontiguousblock,reducingoverheadfrompointersormetadata.2)Lists,oftenimplementedasdynamicarraysorlinkedstruct
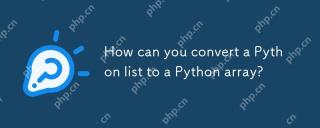
ToconvertaPythonlisttoanarray,usethearraymodule:1)Importthearraymodule,2)Createalist,3)Usearray(typecode,list)toconvertit,specifyingthetypecodelike'i'forintegers.Thisconversionoptimizesmemoryusageforhomogeneousdata,enhancingperformanceinnumericalcomp
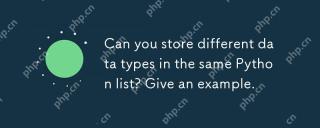
Python lists can store different types of data. The example list contains integers, strings, floating point numbers, booleans, nested lists, and dictionaries. List flexibility is valuable in data processing and prototyping, but it needs to be used with caution to ensure the readability and maintainability of the code.
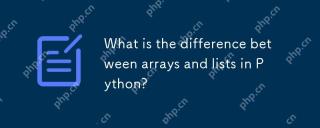
Pythondoesnothavebuilt-inarrays;usethearraymoduleformemory-efficienthomogeneousdatastorage,whilelistsareversatileformixeddatatypes.Arraysareefficientforlargedatasetsofthesametype,whereaslistsofferflexibilityandareeasiertouseformixedorsmallerdatasets.
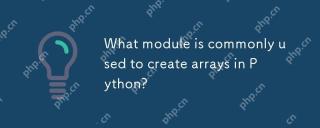
ThemostcommonlyusedmoduleforcreatingarraysinPythonisnumpy.1)Numpyprovidesefficienttoolsforarrayoperations,idealfornumericaldata.2)Arrayscanbecreatedusingnp.array()for1Dand2Dstructures.3)Numpyexcelsinelement-wiseoperationsandcomplexcalculationslikemea
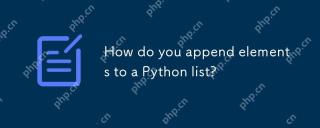
ToappendelementstoaPythonlist,usetheappend()methodforsingleelements,extend()formultipleelements,andinsert()forspecificpositions.1)Useappend()foraddingoneelementattheend.2)Useextend()toaddmultipleelementsefficiently.3)Useinsert()toaddanelementataspeci
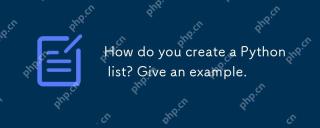
TocreateaPythonlist,usesquarebrackets[]andseparateitemswithcommas.1)Listsaredynamicandcanholdmixeddatatypes.2)Useappend(),remove(),andslicingformanipulation.3)Listcomprehensionsareefficientforcreatinglists.4)Becautiouswithlistreferences;usecopy()orsl
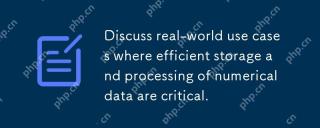
In the fields of finance, scientific research, medical care and AI, it is crucial to efficiently store and process numerical data. 1) In finance, using memory mapped files and NumPy libraries can significantly improve data processing speed. 2) In the field of scientific research, HDF5 files are optimized for data storage and retrieval. 3) In medical care, database optimization technologies such as indexing and partitioning improve data query performance. 4) In AI, data sharding and distributed training accelerate model training. System performance and scalability can be significantly improved by choosing the right tools and technologies and weighing trade-offs between storage and processing speeds.


Hot AI Tools

Undresser.AI Undress
AI-powered app for creating realistic nude photos

AI Clothes Remover
Online AI tool for removing clothes from photos.

Undress AI Tool
Undress images for free

Clothoff.io
AI clothes remover

Video Face Swap
Swap faces in any video effortlessly with our completely free AI face swap tool!

Hot Article

Hot Tools

Notepad++7.3.1
Easy-to-use and free code editor
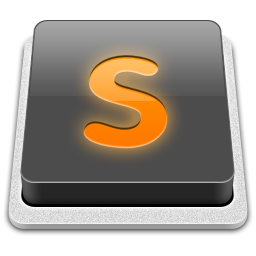
SublimeText3 Mac version
God-level code editing software (SublimeText3)
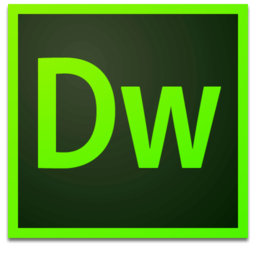
Dreamweaver Mac version
Visual web development tools
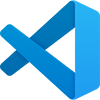
VSCode Windows 64-bit Download
A free and powerful IDE editor launched by Microsoft

SecLists
SecLists is the ultimate security tester's companion. It is a collection of various types of lists that are frequently used during security assessments, all in one place. SecLists helps make security testing more efficient and productive by conveniently providing all the lists a security tester might need. List types include usernames, passwords, URLs, fuzzing payloads, sensitive data patterns, web shells, and more. The tester can simply pull this repository onto a new test machine and he will have access to every type of list he needs.
