To convert a Python list to an array, use the array module: 1) Import the array module, 2) Create a list, 3) Use array(typecode, list) to convert it, specifying the typecode like 'i' for integers. This conversion optimizes memory usage for homogeneous data, enhancing performance in numerical computations, but consider using NumPy arrays for more advanced numerical operations.
Converting a Python list to a Python array might seem straightforward, but there are nuances and best practices to consider. Let's dive into the world of Python data structures and explore this conversion with a touch of personal experience and some deep insights.
When I first started coding in Python, I was fascinated by the flexibility of lists. They're dynamic, easy to use, and incredibly versatile. However, there are times when you need the performance benefits of arrays, especially when dealing with numerical computations. The array
module in Python provides a way to create arrays, which are more memory-efficient for homogeneous data types.
Here's how you can convert a list to an array using the array
module:
from array import array # Let's create a list of integers my_list = [1, 2, 3, 4, 5] # Convert the list to an array of integers my_array = array('i', my_list) # Print the array to verify print(my_array) # Output: array('i', [1, 2, 3, 4, 5])
Now, let's unpack this process and explore some deeper aspects.
Understanding the Conversion
The array
module's array
constructor takes two arguments: the typecode and an iterable. The typecode specifies the type of elements the array will hold. In our example, 'i'
stands for signed integer. You can use different typecodes for different data types, like 'f'
for float, 'd'
for double, etc.
This conversion is not just about changing the data structure; it's about optimizing for specific use cases. Arrays are more compact in memory than lists, especially when dealing with large datasets of the same type. This can lead to performance improvements in numerical computations or when interfacing with C code.
Performance Considerations
When I worked on a project involving large datasets, I noticed that using arrays instead of lists for numerical operations significantly reduced memory usage. However, the conversion itself isn't free. If you're constantly converting between lists and arrays, you might be introducing unnecessary overhead.
Here's a quick benchmark to illustrate the performance difference:
import timeit # List of integers my_list = list(range(1000000)) # Convert to array my_array = array('i', my_list) # Time the sum operation on a list list_time = timeit.timeit(lambda: sum(my_list), number=100) print(f"Time to sum list: {list_time:.6f} seconds") # Time the sum operation on an array array_time = timeit.timeit(lambda: sum(my_array), number=100) print(f"Time to sum array: {array_time:.6f} seconds")
You'll likely see that the array operation is faster, but the difference might be negligible for small datasets. The key is to use arrays when you know you'll be performing operations that benefit from their structure.
Pitfalls and Best Practices
One common pitfall is assuming that arrays are always better than lists. They're not. Arrays are great for homogeneous data, but if you're dealing with mixed types, lists are more flexible. Also, remember that arrays don't support some list methods like append
or extend
. You'll need to use fromlist
to add elements from a list to an array.
Here's an example of how to add elements to an array:
# Create an array my_array = array('i', [1, 2, 3]) # Add elements from a list my_array.fromlist([4, 5, 6]) print(my_array) # Output: array('i', [1, 2, 3, 4, 5, 6])
Another best practice is to consider using NumPy arrays if you're working with numerical data. NumPy arrays are more powerful and flexible than the array
module, offering advanced operations and better performance for large datasets.
import numpy as np # Create a NumPy array from a list my_numpy_array = np.array([1, 2, 3, 4, 5]) print(my_numpy_array) # Output: [1 2 3 4 5]
When to Use Arrays
In my experience, arrays are particularly useful when you're interfacing with C code or when you need to save memory with large datasets of the same type. However, for most general-purpose programming, lists are usually sufficient and more flexible.
Conclusion
Converting a Python list to an array is a simple process, but understanding when and why to do it can significantly impact your code's performance and efficiency. By considering the type of data you're working with and the operations you'll perform, you can make informed decisions about whether to use lists, arrays, or even NumPy arrays. Remember, the best tool depends on the task at hand, and sometimes, the simplest solution is the most effective.
The above is the detailed content of How can you convert a Python list to a Python array?. For more information, please follow other related articles on the PHP Chinese website!
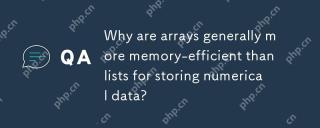
Arraysaregenerallymorememory-efficientthanlistsforstoringnumericaldataduetotheirfixed-sizenatureanddirectmemoryaccess.1)Arraysstoreelementsinacontiguousblock,reducingoverheadfrompointersormetadata.2)Lists,oftenimplementedasdynamicarraysorlinkedstruct
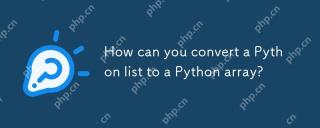
ToconvertaPythonlisttoanarray,usethearraymodule:1)Importthearraymodule,2)Createalist,3)Usearray(typecode,list)toconvertit,specifyingthetypecodelike'i'forintegers.Thisconversionoptimizesmemoryusageforhomogeneousdata,enhancingperformanceinnumericalcomp
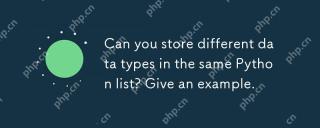
Python lists can store different types of data. The example list contains integers, strings, floating point numbers, booleans, nested lists, and dictionaries. List flexibility is valuable in data processing and prototyping, but it needs to be used with caution to ensure the readability and maintainability of the code.
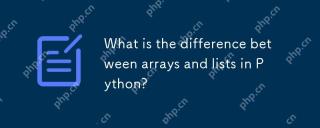
Pythondoesnothavebuilt-inarrays;usethearraymoduleformemory-efficienthomogeneousdatastorage,whilelistsareversatileformixeddatatypes.Arraysareefficientforlargedatasetsofthesametype,whereaslistsofferflexibilityandareeasiertouseformixedorsmallerdatasets.
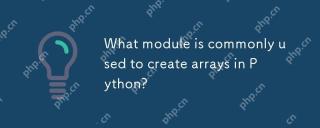
ThemostcommonlyusedmoduleforcreatingarraysinPythonisnumpy.1)Numpyprovidesefficienttoolsforarrayoperations,idealfornumericaldata.2)Arrayscanbecreatedusingnp.array()for1Dand2Dstructures.3)Numpyexcelsinelement-wiseoperationsandcomplexcalculationslikemea
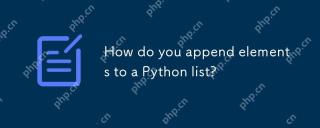
ToappendelementstoaPythonlist,usetheappend()methodforsingleelements,extend()formultipleelements,andinsert()forspecificpositions.1)Useappend()foraddingoneelementattheend.2)Useextend()toaddmultipleelementsefficiently.3)Useinsert()toaddanelementataspeci
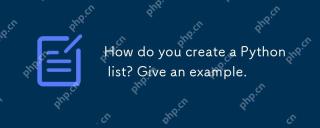
TocreateaPythonlist,usesquarebrackets[]andseparateitemswithcommas.1)Listsaredynamicandcanholdmixeddatatypes.2)Useappend(),remove(),andslicingformanipulation.3)Listcomprehensionsareefficientforcreatinglists.4)Becautiouswithlistreferences;usecopy()orsl
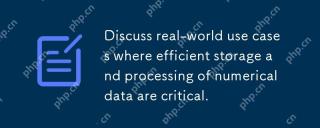
In the fields of finance, scientific research, medical care and AI, it is crucial to efficiently store and process numerical data. 1) In finance, using memory mapped files and NumPy libraries can significantly improve data processing speed. 2) In the field of scientific research, HDF5 files are optimized for data storage and retrieval. 3) In medical care, database optimization technologies such as indexing and partitioning improve data query performance. 4) In AI, data sharding and distributed training accelerate model training. System performance and scalability can be significantly improved by choosing the right tools and technologies and weighing trade-offs between storage and processing speeds.


Hot AI Tools

Undresser.AI Undress
AI-powered app for creating realistic nude photos

AI Clothes Remover
Online AI tool for removing clothes from photos.

Undress AI Tool
Undress images for free

Clothoff.io
AI clothes remover

Video Face Swap
Swap faces in any video effortlessly with our completely free AI face swap tool!

Hot Article

Hot Tools

Atom editor mac version download
The most popular open source editor

Safe Exam Browser
Safe Exam Browser is a secure browser environment for taking online exams securely. This software turns any computer into a secure workstation. It controls access to any utility and prevents students from using unauthorized resources.
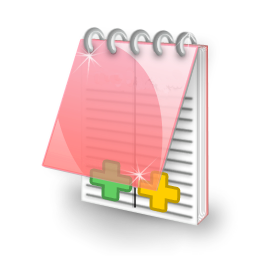
EditPlus Chinese cracked version
Small size, syntax highlighting, does not support code prompt function

mPDF
mPDF is a PHP library that can generate PDF files from UTF-8 encoded HTML. The original author, Ian Back, wrote mPDF to output PDF files "on the fly" from his website and handle different languages. It is slower than original scripts like HTML2FPDF and produces larger files when using Unicode fonts, but supports CSS styles etc. and has a lot of enhancements. Supports almost all languages, including RTL (Arabic and Hebrew) and CJK (Chinese, Japanese and Korean). Supports nested block-level elements (such as P, DIV),

PhpStorm Mac version
The latest (2018.2.1) professional PHP integrated development tool
